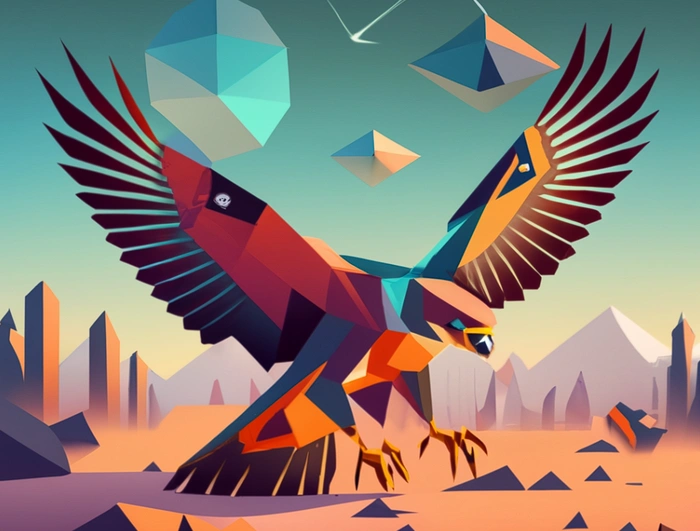
Falcon-40B-Chat-v0.1
Falcon-40B-Chat-v0.1 is a chatbot model for dialogue generation. It was built by fine-tuning Falcon-40B on the OpenAssistant/oasst1 dataset. This repo only includes the LoRA adapters from fine-tuning with 🤗's peft package.
Model Summary
- Model Type: Causal language model (clm)
- Language(s): English
- Base Model: Falcon-40B (License: Apache 2.0)
- Dataset: OpenAssistant/oasst1 (License: Apache 2.0)
- License: Apache 2.0 inherited from "Base Model" and "Dataset"
The model was fine-tuned in 4-bit precision using peft
adapters, transformers
, and bitsandbytes
. Training relied on a method called "Low Rank Adapters" (LoRA), specifically the QLoRA variant. The run took approximately 10 hours and was executed on a workstation with a single A100-SXM NVIDIA GPU with 37 GB of available memory. See attached Colab Notebook for the code and hyperparams used to train the model.
Model Date
May 30, 2023
Open LLM Leaderboard Evaluation Results
Detailed results can be found here
Metric | Value |
---|---|
Avg. | 51.17 |
ARC (25-shot) | 62.63 |
HellaSwag (10-shot) | 85.59 |
MMLU (5-shot) | 57.77 |
TruthfulQA (0-shot) | 51.02 |
Winogrande (5-shot) | 81.45 |
GSM8K (5-shot) | 13.34 |
DROP (3-shot) | 6.36 |
Quick Start
To prompt the chat model, use the following format:
<human>: [Instruction]
<bot>:
Example Dialogue 1
Prompter:
"""<human>: My name is Daniel. Write a short email to my closest friends inviting them to come to my home on Friday for a dinner party, I will make the food but tell them to BYOB.
<bot>:"""
Falcon-40B-Chat-v0.1:
Dear Friends,
I'm excited to invite you to a dinner party at my home on Friday, [date]. I will be making the food, but please bring your own beverages.
I'm looking forward to seeing you and catching up!
Sincerely,
Daniel
Example Dialogue 2
Prompter:
<human>: Create a list of things to do in San Francisco.
<bot>:
Falcon-40B-Chat-v0.1:
Here is a list of things to do in San Francisco:
1. Visit the Golden Gate Bridge
2. Take a cable car ride
3. Visit Alcatraz Island
4. Explore the Fisherman's Wharf
5. Visit the Exploratorium
6. Go to the San Francisco Zoo
7. Visit the California Academy of Sciences
8. Go to the de Young Museum
9. Visit the San Francisco Museum of Modern Art
10. Go to the Asian Art Museum
11. Visit the Conservatory of Flowers
12. Go to the California Historical Society
13. Visit the Walt Disney Family Museum
14. Go to the San Francisco Botanical Garden
15. Visit the San Francisco Museum of Craft and Design
16. Go to the Cartoon Art Museum
17. Visit the Contemporary Jewish Museum
18. Go to the Museum of the African Diaspora
19. Visit the Museum of the City of San Francisco
Direct Use
This model has been finetuned on conversation trees from OpenAssistant/oasst1 and should only be used on data of a similar nature.
Out-of-Scope Use
Production use without adequate assessment of risks and mitigation; any use cases which may be considered irresponsible or harmful.
Bias, Risks, and Limitations
This model is mostly trained on English data, and will not generalize appropriately to other languages. Furthermore, as it is trained on a large-scale corpora representative of the web, it will carry the stereotypes and biases commonly encountered online.
Recommendations
We recommend users of this model to develop guardrails and to take appropriate precautions for any production use.
How to Get Started with the Model
Setup
# Install packages
!pip install -q -U bitsandbytes loralib einops
!pip install -q -U git+https://github.com/huggingface/transformers.git
!pip install -q -U git+https://github.com/huggingface/peft.git
!pip install -q -U git+https://github.com/huggingface/accelerate.git
GPU Inference in 4-bit
This requires a GPU with at least 27GB memory.
First, Load the Model
import torch
from peft import PeftModel, PeftConfig
from transformers import AutoModelForCausalLM, AutoTokenizer, BitsAndBytesConfig
peft_model_id = "dfurman/Falcon-40B-Chat-v0.1"
config = PeftConfig.from_pretrained(peft_model_id)
bnb_config = BitsAndBytesConfig(
load_in_4bit=True,
bnb_4bit_use_double_quant=True,
bnb_4bit_quant_type="nf4",
bnb_4bit_compute_dtype=torch.bfloat16
)
model = AutoModelForCausalLM.from_pretrained(
config.base_model_name_or_path,
return_dict=True,
quantization_config=bnb_config,
device_map={"":0},
trust_remote_code=True,
)
tokenizer = AutoTokenizer.from_pretrained(config.base_model_name_or_path)
tokenizer.pad_token = tokenizer.eos_token
model = PeftModel.from_pretrained(model, peft_model_id)
Next, Run the Model
prompt = """<human>: My name is Daniel. Write a short email to my closest friends inviting them to come to my home on Friday for a dinner party, I will make the food but tell them to BYOB.
<bot>:"""
batch = tokenizer(
prompt,
padding=True,
truncation=True,
return_tensors='pt'
)
batch = batch.to('cuda:0')
with torch.cuda.amp.autocast():
output_tokens = model.generate(
inputs=batch.input_ids,
max_new_tokens=200,
do_sample=False,
use_cache=True,
temperature=1.0,
top_k=50,
top_p=1.0,
num_return_sequences=1,
pad_token_id=tokenizer.eos_token_id,
eos_token_id=tokenizer.eos_token_id,
bos_token_id=tokenizer.eos_token_id,
)
generated_text = tokenizer.decode(output_tokens[0], skip_special_tokens=True)
# Inspect message response in the outputs
print(generated_text.split("<human>: ")[1].split("<bot>: ")[-1])
Reproducibility
See attached Colab Notebook for the code (and hyperparams) used to train the model.
CUDA Info
- CUDA Version: 12.0
- Hardware: 1 A100-SXM
- Max Memory: {0: "37GB"}
- Device Map: {"": 0}
Package Versions Employed
torch
: 2.0.1+cu118transformers
: 4.30.0.dev0peft
: 0.4.0.dev0accelerate
: 0.19.0bitsandbytes
: 0.39.0einops
: 0.6.1
Open LLM Leaderboard Evaluation Results
Detailed results can be found here
Metric | Value |
---|---|
Avg. | 58.63 |
AI2 Reasoning Challenge (25-Shot) | 62.63 |
HellaSwag (10-Shot) | 85.59 |
MMLU (5-Shot) | 57.77 |
TruthfulQA (0-shot) | 51.02 |
Winogrande (5-shot) | 81.45 |
GSM8k (5-shot) | 13.34 |
- Downloads last month
- 27
Model tree for dfurman/Falcon-40B-Chat-v0.1
Base model
tiiuae/falcon-40bDataset used to train dfurman/Falcon-40B-Chat-v0.1
Collection including dfurman/Falcon-40B-Chat-v0.1
Evaluation results
- normalized accuracy on AI2 Reasoning Challenge (25-Shot)test set Open LLM Leaderboard62.630
- normalized accuracy on HellaSwag (10-Shot)validation set Open LLM Leaderboard85.590
- accuracy on MMLU (5-Shot)test set Open LLM Leaderboard57.770
- mc2 on TruthfulQA (0-shot)validation set Open LLM Leaderboard51.020
- accuracy on Winogrande (5-shot)validation set Open LLM Leaderboard81.450
- accuracy on GSM8k (5-shot)test set Open LLM Leaderboard13.340