text
stringlengths 7
318k
| id
stringlengths 14
166
| metadata
dict | __index_level_0__
int64 0
439
|
---|---|---|---|
# coding=utf-8
# Copyright 2022 The HuggingFace Inc. team. All rights reserved.
#
# Licensed under the Apache License, Version 2.0 (the "License");
# you may not use this file except in compliance with the License.
# You may obtain a copy of the License at
#
# http://www.apache.org/licenses/LICENSE-2.0
#
# Unless required by applicable law or agreed to in writing, software
# distributed under the License is distributed on an "AS IS" BASIS,
# WITHOUT WARRANTIES OR CONDITIONS OF ANY KIND, either express or implied.
# See the License for the specific language governing permissions and
# limitations under the License.
"""Image processor class for Flava."""
import math
import random
from functools import lru_cache
from typing import Any, Dict, Iterable, List, Optional, Tuple, Union
import numpy as np
from ...image_processing_utils import BaseImageProcessor, BatchFeature, get_size_dict
from ...image_transforms import resize, to_channel_dimension_format
from ...image_utils import (
OPENAI_CLIP_MEAN,
OPENAI_CLIP_STD,
ChannelDimension,
ImageInput,
PILImageResampling,
infer_channel_dimension_format,
is_scaled_image,
make_list_of_images,
to_numpy_array,
valid_images,
)
from ...utils import TensorType, is_vision_available, logging
if is_vision_available():
import PIL
logger = logging.get_logger(__name__)
# These values are taken from CLIP
FLAVA_IMAGE_MEAN = OPENAI_CLIP_MEAN
FLAVA_IMAGE_STD = OPENAI_CLIP_STD
FLAVA_CODEBOOK_MEAN = [0.0, 0.0, 0.0]
FLAVA_CODEBOOK_STD = [1.0, 1.0, 1.0]
LOGIT_LAPLACE_EPS: float = 0.1
# Inspired from https://github.com/microsoft/unilm/blob/master/beit/masking_generator.py
class FlavaMaskingGenerator:
def __init__(
self,
input_size: Union[int, Tuple[int, int]] = 14,
total_mask_patches: int = 75,
mask_group_max_patches: Optional[int] = None,
mask_group_min_patches: int = 16,
mask_group_min_aspect_ratio: Optional[float] = 0.3,
mask_group_max_aspect_ratio: float = None,
):
if not isinstance(input_size, tuple):
input_size = (input_size,) * 2
self.height, self.width = input_size
self.num_patches = self.height * self.width
self.total_mask_patches = total_mask_patches
self.mask_group_min_patches = mask_group_min_patches
self.mask_group_max_patches = total_mask_patches if mask_group_max_patches is None else mask_group_max_patches
mask_group_max_aspect_ratio = mask_group_max_aspect_ratio or 1 / mask_group_min_aspect_ratio
self.log_aspect_ratio = (math.log(mask_group_min_aspect_ratio), math.log(mask_group_max_aspect_ratio))
def __repr__(self):
repr_str = "MaskingGenerator(%d, %d -> [%d ~ %d], max = %d, %.3f ~ %.3f)" % (
self.height,
self.width,
self.mask_group_min_patches,
self.mask_group_max_patches,
self.total_mask_patches,
self.log_aspect_ratio[0],
self.log_aspect_ratio[1],
)
return repr_str
def get_shape(self):
return self.height, self.width
def _mask(self, mask, max_mask_patches):
delta = 0
for _attempt in range(10):
target_area = random.uniform(self.mask_group_min_patches, max_mask_patches)
aspect_ratio = math.exp(random.uniform(*self.log_aspect_ratio))
height = int(round(math.sqrt(target_area * aspect_ratio)))
width = int(round(math.sqrt(target_area / aspect_ratio)))
if width < self.width and height < self.height:
top = random.randint(0, self.height - height)
left = random.randint(0, self.width - width)
num_masked = mask[top : top + height, left : left + width].sum()
# Overlap
if 0 < height * width - num_masked <= max_mask_patches:
for i in range(top, top + height):
for j in range(left, left + width):
if mask[i, j] == 0:
mask[i, j] = 1
delta += 1
if delta > 0:
break
return delta
def __call__(self):
mask = np.zeros(shape=self.get_shape(), dtype=int)
mask_count = 0
while mask_count < self.total_mask_patches:
max_mask_patches = self.total_mask_patches - mask_count
max_mask_patches = min(max_mask_patches, self.mask_group_max_patches)
delta = self._mask(mask, max_mask_patches)
if delta == 0:
break
else:
mask_count += delta
return mask
class FlavaImageProcessor(BaseImageProcessor):
r"""
Constructs a Flava image processor.
Args:
do_resize (`bool`, *optional*, defaults to `True`):
Whether to resize the image's (height, width) dimensions to the specified `size`. Can be overridden by the
`do_resize` parameter in `preprocess`.
size (`Dict[str, int]` *optional*, defaults to `{"height": 224, "width": 224}`):
Size of the image after resizing. Can be overridden by the `size` parameter in `preprocess`.
resample (`PILImageResampling`, *optional*, defaults to `PILImageResampling.BICUBIC`):
Resampling filter to use if resizing the image. Can be overridden by the `resample` parameter in
`preprocess`.
do_center_crop (`bool`, *optional*, defaults to `True`):
Whether to center crop the images. Can be overridden by the `do_center_crop` parameter in `preprocess`.
crop_size (`Dict[str, int]` *optional*, defaults to `{"height": 224, "width": 224}`):
Size of image after the center crop `(crop_size["height"], crop_size["width"])`. Can be overridden by the
`crop_size` parameter in `preprocess`.
do_rescale (`bool`, *optional*, defaults to `True`):
Whether to rescale the image by the specified scale `rescale_factor`. Can be overridden by the `do_rescale`
parameter in `preprocess`.
rescale_factor (`int` or `float`, *optional*, defaults to `1/255`):
Scale factor to use if rescaling the image. Can be overridden by the `rescale_factor` parameter in
`preprocess`.
do_normalize (`bool`, *optional*, defaults to `True`):
Whether to normalize the image. Can be overridden by the `do_normalize` parameter in `preprocess`.
image_mean (`float` or `List[float]`, *optional*, defaults to `IMAGENET_STANDARD_MEAN`):
Mean to use if normalizing the image. This is a float or list of floats the length of the number of
channels in the image. Can be overridden by the `image_mean` parameter in the `preprocess` method.
image_std (`float` or `List[float]`, *optional*, defaults to `IMAGENET_STANDARD_STD`):
Standard deviation to use if normalizing the image. This is a float or list of floats the length of the
number of channels in the image. Can be overridden by the `image_std` parameter in the `preprocess` method.
return_image_mask (`bool`, *optional*, defaults to `False`):
Whether to return the image mask. Can be overridden by the `return_image_mask` parameter in `preprocess`.
input_size_patches (`int`, *optional*, defaults to 14):
Number of patches in the image in height and width direction. 14x14 = 196 total patches. Can be overridden
by the `input_size_patches` parameter in `preprocess`.
total_mask_patches (`int`, *optional*, defaults to 75):
Total number of patches that should be masked. Can be overridden by the `total_mask_patches` parameter in
`preprocess`.
mask_group_min_patches (`int`, *optional*, defaults to 16):
Minimum number of patches that should be masked. Can be overridden by the `mask_group_min_patches`
parameter in `preprocess`.
mask_group_max_patches (`int`, *optional*):
Maximum number of patches that should be masked. Can be overridden by the `mask_group_max_patches`
parameter in `preprocess`.
mask_group_min_aspect_ratio (`float`, *optional*, defaults to 0.3):
Minimum aspect ratio of the mask window. Can be overridden by the `mask_group_min_aspect_ratio` parameter
in `preprocess`.
mask_group_max_aspect_ratio (`float`, *optional*):
Maximum aspect ratio of the mask window. Can be overridden by the `mask_group_max_aspect_ratio` parameter
in `preprocess`.
codebook_do_resize (`bool`, *optional*, defaults to `True`):
Whether to resize the input for codebook to a certain. Can be overridden by the `codebook_do_resize`
parameter in `preprocess`. `codebook_size`.
codebook_size (`Dict[str, int]`, *optional*, defaults to `{"height": 224, "width": 224}`):
Resize the input for codebook to the given size. Can be overridden by the `codebook_size` parameter in
`preprocess`.
codebook_resample (`PILImageResampling`, *optional*, defaults to `PILImageResampling.LANCZOS`):
Resampling filter to use if resizing the codebook image. Can be overridden by the `codebook_resample`
parameter in `preprocess`.
codebook_do_center_crop (`bool`, *optional*, defaults to `True`):
Whether to crop the input for codebook at the center. If the input size is smaller than
`codebook_crop_size` along any edge, the image is padded with 0's and then center cropped. Can be
overridden by the `codebook_do_center_crop` parameter in `preprocess`.
codebook_crop_size (`Dict[str, int]`, *optional*, defaults to `{"height": 224, "width": 224}`):
Desired output size for codebook input when applying center-cropping. Can be overridden by the
`codebook_crop_size` parameter in `preprocess`.
codebook_do_rescale (`bool`, *optional*, defaults to `True`):
Whether to rescale the input for codebook by the specified scale `codebook_rescale_factor`. Can be
overridden by the `codebook_do_rescale` parameter in `preprocess`.
codebook_rescale_factor (`int` or `float`, *optional*, defaults to `1/255`):
Defines the scale factor to use if rescaling the codebook image. Can be overridden by the
`codebook_rescale_factor` parameter in `preprocess`.
codebook_do_map_pixels (`bool`, *optional*, defaults to `True`):
Whether to map the pixel values of the codebook input to (1 - 2e)x + e. Can be overridden by the
`codebook_do_map_pixels` parameter in `preprocess`.
codebook_do_normalize (`bool`, *optional*, defaults to `True`):
Whether or not to normalize the input for codebook with `codebook_image_mean` and `codebook_image_std`. Can
be overridden by the `codebook_do_normalize` parameter in `preprocess`.
codebook_image_mean (`Optional[Union[float, Iterable[float]]]`, *optional*, defaults to `[0, 0, 0]`):
The sequence of means for each channel, to be used when normalizing images for codebook. Can be overridden
by the `codebook_image_mean` parameter in `preprocess`.
codebook_image_std (`Optional[Union[float, Iterable[float]]]`, *optional*, defaults to `[0.5, 0.5, 0.5]`):
The sequence of standard deviations for each channel, to be used when normalizing images for codebook. Can
be overridden by the `codebook_image_std` parameter in `preprocess`.
"""
model_input_names = ["pixel_values"]
def __init__(
self,
do_resize: bool = True,
size: Dict[str, int] = None,
resample: PILImageResampling = PILImageResampling.BICUBIC,
do_center_crop: bool = True,
crop_size: Dict[str, int] = None,
do_rescale: bool = True,
rescale_factor: Union[int, float] = 1 / 255,
do_normalize: bool = True,
image_mean: Optional[Union[float, Iterable[float]]] = None,
image_std: Optional[Union[float, Iterable[float]]] = None,
# Mask related params
return_image_mask: bool = False,
input_size_patches: int = 14,
total_mask_patches: int = 75,
mask_group_min_patches: int = 16,
mask_group_max_patches: Optional[int] = None,
mask_group_min_aspect_ratio: float = 0.3,
mask_group_max_aspect_ratio: Optional[float] = None,
# Codebook related params
return_codebook_pixels: bool = False,
codebook_do_resize: bool = True,
codebook_size: bool = None,
codebook_resample: int = PILImageResampling.LANCZOS,
codebook_do_center_crop: bool = True,
codebook_crop_size: int = None,
codebook_do_rescale: bool = True,
codebook_rescale_factor: Union[int, float] = 1 / 255,
codebook_do_map_pixels: bool = True,
codebook_do_normalize: bool = True,
codebook_image_mean: Optional[Union[float, Iterable[float]]] = None,
codebook_image_std: Optional[Union[float, Iterable[float]]] = None,
**kwargs,
) -> None:
super().__init__(**kwargs)
size = size if size is not None else {"height": 224, "width": 224}
size = get_size_dict(size)
crop_size = crop_size if crop_size is not None else {"height": 224, "width": 224}
crop_size = get_size_dict(crop_size, param_name="crop_size")
codebook_size = codebook_size if codebook_size is not None else {"height": 112, "width": 112}
codebook_size = get_size_dict(codebook_size, param_name="codebook_size")
codebook_crop_size = codebook_crop_size if codebook_crop_size is not None else {"height": 112, "width": 112}
codebook_crop_size = get_size_dict(codebook_crop_size, param_name="codebook_crop_size")
self.do_resize = do_resize
self.size = size
self.resample = resample
self.do_rescale = do_rescale
self.rescale_factor = rescale_factor
self.do_center_crop = do_center_crop
self.crop_size = crop_size
self.do_normalize = do_normalize
self.image_mean = image_mean if image_mean is not None else FLAVA_IMAGE_MEAN
self.image_std = image_std if image_std is not None else FLAVA_IMAGE_STD
self.return_image_mask = return_image_mask
self.input_size_patches = input_size_patches
self.total_mask_patches = total_mask_patches
self.mask_group_min_patches = mask_group_min_patches
self.mask_group_max_patches = mask_group_max_patches
self.mask_group_min_aspect_ratio = mask_group_min_aspect_ratio
self.mask_group_max_aspect_ratio = mask_group_max_aspect_ratio
self.return_codebook_pixels = return_codebook_pixels
self.codebook_do_resize = codebook_do_resize
self.codebook_size = codebook_size
self.codebook_resample = codebook_resample
self.codebook_do_center_crop = codebook_do_center_crop
self.codebook_crop_size = codebook_crop_size
self.codebook_do_rescale = codebook_do_rescale
self.codebook_rescale_factor = codebook_rescale_factor
self.codebook_do_map_pixels = codebook_do_map_pixels
self.codebook_do_normalize = codebook_do_normalize
self.codebook_image_mean = codebook_image_mean
self.codebook_image_mean = codebook_image_mean if codebook_image_mean is not None else FLAVA_CODEBOOK_MEAN
self.codebook_image_std = codebook_image_std if codebook_image_std is not None else FLAVA_CODEBOOK_STD
@classmethod
def from_dict(cls, image_processor_dict: Dict[str, Any], **kwargs):
"""
Overrides the `from_dict` method from the base class to make sure parameters are updated if image processor is
created using from_dict and kwargs e.g. `FlavaImageProcessor.from_pretrained(checkpoint, codebook_size=600)`
"""
image_processor_dict = image_processor_dict.copy()
if "codebook_size" in kwargs:
image_processor_dict["codebook_size"] = kwargs.pop("codebook_size")
if "codebook_crop_size" in kwargs:
image_processor_dict["codebook_crop_size"] = kwargs.pop("codebook_crop_size")
return super().from_dict(image_processor_dict, **kwargs)
@lru_cache()
def masking_generator(
self,
input_size_patches,
total_mask_patches,
mask_group_min_patches,
mask_group_max_patches,
mask_group_min_aspect_ratio,
mask_group_max_aspect_ratio,
) -> FlavaMaskingGenerator:
return FlavaMaskingGenerator(
input_size=input_size_patches,
total_mask_patches=total_mask_patches,
mask_group_min_patches=mask_group_min_patches,
mask_group_max_patches=mask_group_max_patches,
mask_group_min_aspect_ratio=mask_group_min_aspect_ratio,
mask_group_max_aspect_ratio=mask_group_max_aspect_ratio,
)
# Copied from transformers.models.vit.image_processing_vit.ViTImageProcessor.resize with PILImageResampling.BILINEAR->PILImageResampling.BICUBIC
def resize(
self,
image: np.ndarray,
size: Dict[str, int],
resample: PILImageResampling = PILImageResampling.BICUBIC,
data_format: Optional[Union[str, ChannelDimension]] = None,
input_data_format: Optional[Union[str, ChannelDimension]] = None,
**kwargs,
) -> np.ndarray:
"""
Resize an image to `(size["height"], size["width"])`.
Args:
image (`np.ndarray`):
Image to resize.
size (`Dict[str, int]`):
Dictionary in the format `{"height": int, "width": int}` specifying the size of the output image.
resample (`PILImageResampling`, *optional*, defaults to `PILImageResampling.BICUBIC`):
`PILImageResampling` filter to use when resizing the image e.g. `PILImageResampling.BICUBIC`.
data_format (`ChannelDimension` or `str`, *optional*):
The channel dimension format for the output image. If unset, the channel dimension format of the input
image is used. Can be one of:
- `"channels_first"` or `ChannelDimension.FIRST`: image in (num_channels, height, width) format.
- `"channels_last"` or `ChannelDimension.LAST`: image in (height, width, num_channels) format.
- `"none"` or `ChannelDimension.NONE`: image in (height, width) format.
input_data_format (`ChannelDimension` or `str`, *optional*):
The channel dimension format for the input image. If unset, the channel dimension format is inferred
from the input image. Can be one of:
- `"channels_first"` or `ChannelDimension.FIRST`: image in (num_channels, height, width) format.
- `"channels_last"` or `ChannelDimension.LAST`: image in (height, width, num_channels) format.
- `"none"` or `ChannelDimension.NONE`: image in (height, width) format.
Returns:
`np.ndarray`: The resized image.
"""
size = get_size_dict(size)
if "height" not in size or "width" not in size:
raise ValueError(f"The `size` dictionary must contain the keys `height` and `width`. Got {size.keys()}")
output_size = (size["height"], size["width"])
return resize(
image,
size=output_size,
resample=resample,
data_format=data_format,
input_data_format=input_data_format,
**kwargs,
)
def map_pixels(self, image: np.ndarray) -> np.ndarray:
return (1 - 2 * LOGIT_LAPLACE_EPS) * image + LOGIT_LAPLACE_EPS
def _preprocess_image(
self,
image: ImageInput,
do_resize: bool = None,
size: Dict[str, int] = None,
resample: PILImageResampling = None,
do_center_crop: bool = None,
crop_size: Dict[str, int] = None,
do_rescale: bool = None,
rescale_factor: float = None,
do_normalize: bool = None,
image_mean: Optional[Union[float, List[float]]] = None,
image_std: Optional[Union[float, List[float]]] = None,
do_map_pixels: bool = None,
data_format: Optional[ChannelDimension] = ChannelDimension.FIRST,
input_data_format: Optional[ChannelDimension] = None,
) -> np.ndarray:
"""Preprocesses a single image."""
if do_resize and size is None or resample is None:
raise ValueError("Size and resample must be specified if do_resize is True.")
if do_rescale and rescale_factor is None:
raise ValueError("Rescale factor must be specified if do_rescale is True.")
if do_normalize and (image_mean is None or image_std is None):
raise ValueError("Image mean and std must be specified if do_normalize is True.")
# All transformations expect numpy arrays.
image = to_numpy_array(image)
if is_scaled_image(image) and do_rescale:
logger.warning_once(
"It looks like you are trying to rescale already rescaled images. If the input"
" images have pixel values between 0 and 1, set `do_rescale=False` to avoid rescaling them again."
)
if input_data_format is None:
# We assume that all images have the same channel dimension format.
input_data_format = infer_channel_dimension_format(image)
if do_resize:
image = self.resize(image=image, size=size, resample=resample, input_data_format=input_data_format)
if do_center_crop:
image = self.center_crop(image=image, size=crop_size, input_data_format=input_data_format)
if do_rescale:
image = self.rescale(image=image, scale=rescale_factor, input_data_format=input_data_format)
if do_normalize:
image = self.normalize(image=image, mean=image_mean, std=image_std, input_data_format=input_data_format)
if do_map_pixels:
image = self.map_pixels(image)
if data_format is not None:
image = to_channel_dimension_format(image, data_format, input_channel_dim=input_data_format)
return image
def preprocess(
self,
images: ImageInput,
do_resize: Optional[bool] = None,
size: Dict[str, int] = None,
resample: PILImageResampling = None,
do_center_crop: Optional[bool] = None,
crop_size: Optional[Dict[str, int]] = None,
do_rescale: Optional[bool] = None,
rescale_factor: Optional[float] = None,
do_normalize: Optional[bool] = None,
image_mean: Optional[Union[float, List[float]]] = None,
image_std: Optional[Union[float, List[float]]] = None,
# Mask related params
return_image_mask: Optional[bool] = None,
input_size_patches: Optional[int] = None,
total_mask_patches: Optional[int] = None,
mask_group_min_patches: Optional[int] = None,
mask_group_max_patches: Optional[int] = None,
mask_group_min_aspect_ratio: Optional[float] = None,
mask_group_max_aspect_ratio: Optional[float] = None,
# Codebook related params
return_codebook_pixels: Optional[bool] = None,
codebook_do_resize: Optional[bool] = None,
codebook_size: Optional[Dict[str, int]] = None,
codebook_resample: Optional[int] = None,
codebook_do_center_crop: Optional[bool] = None,
codebook_crop_size: Optional[Dict[str, int]] = None,
codebook_do_rescale: Optional[bool] = None,
codebook_rescale_factor: Optional[float] = None,
codebook_do_map_pixels: Optional[bool] = None,
codebook_do_normalize: Optional[bool] = None,
codebook_image_mean: Optional[Iterable[float]] = None,
codebook_image_std: Optional[Iterable[float]] = None,
return_tensors: Optional[Union[str, TensorType]] = None,
data_format: ChannelDimension = ChannelDimension.FIRST,
input_data_format: Optional[Union[str, ChannelDimension]] = None,
**kwargs,
) -> PIL.Image.Image:
"""
Preprocess an image or batch of images.
Args:
images (`ImageInput`):
Image to preprocess. Expects a single or batch of images with pixel values ranging from 0 to 255. If
passing in images with pixel values between 0 and 1, set `do_rescale=False`.
do_resize (`bool`, *optional*, defaults to `self.do_resize`):
Whether to resize the image.
size (`Dict[str, int]`, *optional*, defaults to `self.size`):
Size of the image.
resample (`int`, *optional*, defaults to `self.resample`):
Resampling filter to use if resizing the image. This can be one of the enum `PILImageResampling`, Only
has an effect if `do_resize` is set to `True`.
do_center_crop (`bool`, *optional*, defaults to `self.do_center_crop`):
Whether to center crop the image.
crop_size (`Dict[str, int]`, *optional*, defaults to `self.crop_size`):
Size of the center crop. Only has an effect if `do_center_crop` is set to `True`.
do_rescale (`bool`, *optional*, defaults to `self.do_rescale`):
Whether to rescale the image values between [0 - 1].
rescale_factor (`float`, *optional*, defaults to `self.rescale_factor`):
Rescale factor to rescale the image by if `do_rescale` is set to `True`.
do_normalize (`bool`, *optional*, defaults to `self.do_normalize`):
Whether to normalize the image.
image_mean (`float` or `List[float]`, *optional*, defaults to `self.image_mean`):
Image mean.
image_std (`float` or `List[float]`, *optional*, defaults to `self.image_std`):
Image standard deviation.
return_image_mask (`bool`, *optional*, defaults to `self.return_image_mask`):
Whether to return the image mask.
input_size_patches (`int`, *optional*, defaults to `self.input_size_patches`):
Size of the patches to extract from the image.
total_mask_patches (`int`, *optional*, defaults to `self.total_mask_patches`):
Total number of patches to extract from the image.
mask_group_min_patches (`int`, *optional*, defaults to `self.mask_group_min_patches`):
Minimum number of patches to extract from the image.
mask_group_max_patches (`int`, *optional*, defaults to `self.mask_group_max_patches`):
Maximum number of patches to extract from the image.
mask_group_min_aspect_ratio (`float`, *optional*, defaults to `self.mask_group_min_aspect_ratio`):
Minimum aspect ratio of the patches to extract from the image.
mask_group_max_aspect_ratio (`float`, *optional*, defaults to `self.mask_group_max_aspect_ratio`):
Maximum aspect ratio of the patches to extract from the image.
return_codebook_pixels (`bool`, *optional*, defaults to `self.return_codebook_pixels`):
Whether to return the codebook pixels.
codebook_do_resize (`bool`, *optional*, defaults to `self.codebook_do_resize`):
Whether to resize the codebook pixels.
codebook_size (`Dict[str, int]`, *optional*, defaults to `self.codebook_size`):
Size of the codebook pixels.
codebook_resample (`int`, *optional*, defaults to `self.codebook_resample`):
Resampling filter to use if resizing the codebook pixels. This can be one of the enum
`PILImageResampling`, Only has an effect if `codebook_do_resize` is set to `True`.
codebook_do_center_crop (`bool`, *optional*, defaults to `self.codebook_do_center_crop`):
Whether to center crop the codebook pixels.
codebook_crop_size (`Dict[str, int]`, *optional*, defaults to `self.codebook_crop_size`):
Size of the center crop of the codebook pixels. Only has an effect if `codebook_do_center_crop` is set
to `True`.
codebook_do_rescale (`bool`, *optional*, defaults to `self.codebook_do_rescale`):
Whether to rescale the codebook pixels values between [0 - 1].
codebook_rescale_factor (`float`, *optional*, defaults to `self.codebook_rescale_factor`):
Rescale factor to rescale the codebook pixels by if `codebook_do_rescale` is set to `True`.
codebook_do_map_pixels (`bool`, *optional*, defaults to `self.codebook_do_map_pixels`):
Whether to map the codebook pixels values.
codebook_do_normalize (`bool`, *optional*, defaults to `self.codebook_do_normalize`):
Whether to normalize the codebook pixels.
codebook_image_mean (`float` or `List[float]`, *optional*, defaults to `self.codebook_image_mean`):
Codebook pixels mean to normalize the codebook pixels by if `codebook_do_normalize` is set to `True`.
codebook_image_std (`float` or `List[float]`, *optional*, defaults to `self.codebook_image_std`):
Codebook pixels standard deviation to normalize the codebook pixels by if `codebook_do_normalize` is
set to `True`.
return_tensors (`str` or `TensorType`, *optional*):
The type of tensors to return. Can be one of:
- Unset: Return a list of `np.ndarray`.
- `TensorType.TENSORFLOW` or `'tf'`: Return a batch of type `tf.Tensor`.
- `TensorType.PYTORCH` or `'pt'`: Return a batch of type `torch.Tensor`.
- `TensorType.NUMPY` or `'np'`: Return a batch of type `np.ndarray`.
- `TensorType.JAX` or `'jax'`: Return a batch of type `jax.numpy.ndarray`.
data_format (`ChannelDimension` or `str`, *optional*, defaults to `ChannelDimension.FIRST`):
The channel dimension format for the output image. Can be one of:
- `ChannelDimension.FIRST`: image in (num_channels, height, width) format.
- `ChannelDimension.LAST`: image in (height, width, num_channels) format.
input_data_format (`ChannelDimension` or `str`, *optional*):
The channel dimension format for the input image. If unset, the channel dimension format is inferred
from the input image. Can be one of:
- `"channels_first"` or `ChannelDimension.FIRST`: image in (num_channels, height, width) format.
- `"channels_last"` or `ChannelDimension.LAST`: image in (height, width, num_channels) format.
- `"none"` or `ChannelDimension.NONE`: image in (height, width) format.
"""
do_resize = do_resize if do_resize is not None else self.do_resize
size = size if size is not None else self.size
size = get_size_dict(size)
resample = resample if resample is not None else self.resample
do_center_crop = do_center_crop if do_center_crop is not None else self.do_center_crop
crop_size = crop_size if crop_size is not None else self.crop_size
crop_size = get_size_dict(crop_size, param_name="crop_size")
do_rescale = do_rescale if do_rescale is not None else self.do_rescale
rescale_factor = rescale_factor if rescale_factor is not None else self.rescale_factor
do_normalize = do_normalize if do_normalize is not None else self.do_normalize
image_mean = image_mean if image_mean is not None else self.image_mean
image_std = image_std if image_std is not None else self.image_std
return_image_mask = return_image_mask if return_image_mask is not None else self.return_image_mask
input_size_patches = input_size_patches if input_size_patches is not None else self.input_size_patches
total_mask_patches = total_mask_patches if total_mask_patches is not None else self.total_mask_patches
mask_group_min_patches = (
mask_group_min_patches if mask_group_min_patches is not None else self.mask_group_min_patches
)
mask_group_max_patches = (
mask_group_max_patches if mask_group_max_patches is not None else self.mask_group_max_patches
)
mask_group_min_aspect_ratio = (
mask_group_min_aspect_ratio
if mask_group_min_aspect_ratio is not None
else self.mask_group_min_aspect_ratio
)
mask_group_max_aspect_ratio = (
mask_group_max_aspect_ratio
if mask_group_max_aspect_ratio is not None
else self.mask_group_max_aspect_ratio
)
return_codebook_pixels = (
return_codebook_pixels if return_codebook_pixels is not None else self.return_codebook_pixels
)
codebook_do_resize = codebook_do_resize if codebook_do_resize is not None else self.codebook_do_resize
codebook_size = codebook_size if codebook_size is not None else self.codebook_size
codebook_size = get_size_dict(codebook_size, param_name="codebook_size")
codebook_resample = codebook_resample if codebook_resample is not None else self.codebook_resample
codebook_do_rescale = codebook_do_rescale if codebook_do_rescale is not None else self.codebook_do_rescale
codebook_rescale_factor = (
codebook_rescale_factor if codebook_rescale_factor is not None else self.codebook_rescale_factor
)
codebook_do_center_crop = (
codebook_do_center_crop if codebook_do_center_crop is not None else self.codebook_do_center_crop
)
codebook_crop_size = codebook_crop_size if codebook_crop_size is not None else self.codebook_crop_size
codebook_crop_size = get_size_dict(codebook_crop_size, param_name="codebook_crop_size")
codebook_do_map_pixels = (
codebook_do_map_pixels if codebook_do_map_pixels is not None else self.codebook_do_map_pixels
)
codebook_do_normalize = (
codebook_do_normalize if codebook_do_normalize is not None else self.codebook_do_normalize
)
codebook_image_mean = codebook_image_mean if codebook_image_mean is not None else self.codebook_image_mean
codebook_image_std = codebook_image_std if codebook_image_std is not None else self.codebook_image_std
images = make_list_of_images(images)
if not valid_images(images):
raise ValueError(
"Invalid image type. Must be of type PIL.Image.Image, numpy.ndarray, "
"torch.Tensor, tf.Tensor or jax.ndarray."
)
processed_images = [
self._preprocess_image(
image=img,
do_resize=do_resize,
size=size,
resample=resample,
do_center_crop=do_center_crop,
crop_size=crop_size,
do_rescale=do_rescale,
rescale_factor=rescale_factor,
do_normalize=do_normalize,
image_mean=image_mean,
image_std=image_std,
do_map_pixels=False,
data_format=data_format,
input_data_format=input_data_format,
)
for img in images
]
data = {"pixel_values": processed_images}
if return_codebook_pixels:
codebook_images = [
self._preprocess_image(
image=img,
do_resize=codebook_do_resize,
size=codebook_size,
resample=codebook_resample,
do_center_crop=codebook_do_center_crop,
crop_size=codebook_crop_size,
do_rescale=codebook_do_rescale,
rescale_factor=codebook_rescale_factor,
do_normalize=codebook_do_normalize,
image_mean=codebook_image_mean,
image_std=codebook_image_std,
do_map_pixels=codebook_do_map_pixels,
data_format=data_format,
input_data_format=input_data_format,
)
for img in images
]
data["codebook_pixel_values"] = codebook_images
if return_image_mask:
mask_generator = self.masking_generator(
input_size_patches=input_size_patches,
total_mask_patches=total_mask_patches,
mask_group_min_patches=mask_group_min_patches,
mask_group_max_patches=mask_group_max_patches,
mask_group_min_aspect_ratio=mask_group_min_aspect_ratio,
mask_group_max_aspect_ratio=mask_group_max_aspect_ratio,
)
masks = [mask_generator() for _ in images]
data["bool_masked_pos"] = masks
return BatchFeature(data=data, tensor_type=return_tensors)
| transformers/src/transformers/models/flava/image_processing_flava.py/0 | {
"file_path": "transformers/src/transformers/models/flava/image_processing_flava.py",
"repo_id": "transformers",
"token_count": 16167
} | 300 |
# coding=utf-8
# Copyright 2020 The Facebook AI Research Team Authors and The HuggingFace Inc. team.
#
# Licensed under the Apache License, Version 2.0 (the "License");
# you may not use this file except in compliance with the License.
# You may obtain a copy of the License at
#
# http://www.apache.org/licenses/LICENSE-2.0
#
# Unless required by applicable law or agreed to in writing, software
# distributed under the License is distributed on an "AS IS" BASIS,
# WITHOUT WARRANTIES OR CONDITIONS OF ANY KIND, either express or implied.
# See the License for the specific language governing permissions and
# limitations under the License.
#
# Original implementation: https://github.com/pytorch/fairseq/tree/master/examples/wmt19
# Authors:
# - @alexeib Alexei Baevski
# - @edunov Sergey Edunov
# - @michaelauli Michael Auli
# - @myleott Myle Ott
# - @nng555 Nathan Ng
# - David Grangier
# - Kyra Yee
#
# Paper: Facebook FAIR's WMT19 News Translation Task Submission https://arxiv.org/abs/1907.06616
#
"""PyTorch Fairseq model, ported from https://github.com/pytorch/fairseq/tree/master/examples/wmt19"""
import math
from typing import Any, Dict, List, Optional, Tuple, Union
import torch
from torch import Tensor, nn
from torch.nn import CrossEntropyLoss, LayerNorm
from ...activations import ACT2FN
from ...integrations.deepspeed import is_deepspeed_zero3_enabled
from ...modeling_outputs import (
BaseModelOutput,
BaseModelOutputWithPastAndCrossAttentions,
Seq2SeqLMOutput,
Seq2SeqModelOutput,
)
from ...modeling_utils import PreTrainedModel
from ...utils import (
add_code_sample_docstrings,
add_end_docstrings,
add_start_docstrings,
add_start_docstrings_to_model_forward,
logging,
replace_return_docstrings,
)
from .configuration_fsmt import FSMTConfig
logger = logging.get_logger(__name__)
_CHECKPOINT_FOR_DOC = "facebook/wmt19-ru-en"
_CONFIG_FOR_DOC = "FSMTConfig"
# See all FSMT models at https://huggingface.co/models?filter=fsmt
# Porting notes:
# this one is modeled after BartModel*
#
# Currently only translation (fairseq also has weights for LM)
#
# fairseq provides weights for ru-en, en-ru and de-en, en-de pairs. All have been ported.
# - ru-en, en-ru use asymmetric vocab
# - de-en, en-de use a merged single vocab (but the code works as if they are separate)
#
# Differences with Bart:
# - not using bos token
# - 2 separate vocabs (src and target)
# - embed weights aren't tied
# - uses a model Ensemble (but that part isn't ported/implemented yet) - so we
# aren't getting as good of a BLEU score
# - uses a projection layer at the end of the decoder
# - doesn't use final_logits_bias
# - beam search: stops as soon as num_beams == len(hypos) (whereas transformers
# is not satisfied there and will continue searching until the next cycles
# aren't promising something better), comparing BLEU scores - the transformers
# algorithm is slightly superior, therefore using the latter. But if you want
# to match fairseq outputs, you need to pass ``early_stopping=True`` to ``generate()``.
#
# SinusoidalPositionalEmbedding is slightly different from Bart's - generates
# different embeddings. This implementation is copied verbatim from fairseq with
# some small changes to make it work here.
#
# Other changes:
# - doesn't support use_cache as Bart's version does
#
#
# FSMTConfig changes with BartConfig
#
# Differences with BART:
# - src/tgt vocabs aren't shared
# - token embeddings aren't shared
# - needs a language pair
# - scale_embedding are True
#
# some unused args were removed too
#
#
# TODO:
# - port model ensemble (fs uses 4 model checkpoints)
# - solve beam search discrepancies
# docstyle-ignore
"""
Here is how to compare BLEU scores against fairseq implementation:
# en-ru
export PAIR=en-ru
export DATA_DIR=data/$PAIR
export SAVE_DIR=data/$PAIR
export BS=8
export NUM_BEAMS=50
mkdir -p $DATA_DIR
sacrebleu -t wmt19 -l $PAIR --echo src > $DATA_DIR/val.source
sacrebleu -t wmt19 -l $PAIR --echo ref > $DATA_DIR/val.target
echo $PAIR
PYTHONPATH="src:examples/seq2seq" python examples/seq2seq/run_eval.py facebook/wmt19-$PAIR $DATA_DIR/val.source $SAVE_DIR/test_translations.txt --reference_path $DATA_DIR/val.target --score_path $SAVE_DIR/test_bleu.json --bs $BS --task translation --num_beams $NUM_BEAMS
# (fairseq BLEU: 36.4 http://matrix.statmt.org/matrix/output/1914?score_id=37605)
# ru-en
export PAIR=ru-en
export DATA_DIR=data/$PAIR
export SAVE_DIR=data/$PAIR
export BS=8
export NUM_BEAMS=50
mkdir -p $DATA_DIR
sacrebleu -t wmt19 -l $PAIR --echo src > $DATA_DIR/val.source
sacrebleu -t wmt19 -l $PAIR --echo ref > $DATA_DIR/val.target
PYTHONPATH="src:examples/seq2seq" python examples/seq2seq/run_eval.py facebook/wmt19-$PAIR $DATA_DIR/val.source $SAVE_DIR/test_translations.txt --reference_path $DATA_DIR/val.target --score_path $SAVE_DIR/test_bleu.json --bs $BS --task translation --num_beams $NUM_BEAMS
# (fairseq BLEU: 41.3 http://matrix.statmt.org/matrix/output/1907?run_id=6937)
# de-en
export PAIR=de-en
export DATA_DIR=data/$PAIR
export SAVE_DIR=data/$PAIR
export BS=8
export NUM_BEAMS=50
mkdir -p $DATA_DIR
sacrebleu -t wmt19 -l $PAIR --echo src > $DATA_DIR/val.source
sacrebleu -t wmt19 -l $PAIR --echo ref > $DATA_DIR/val.target
echo $PAIR
PYTHONPATH="src:examples/seq2seq" python examples/seq2seq/run_eval.py facebook/wmt19-$PAIR $DATA_DIR/val.source $SAVE_DIR/test_translations.txt --reference_path $DATA_DIR/val.target --score_path $SAVE_DIR/test_bleu.json --bs $BS --task translation --num_beams $NUM_BEAMS
# (fairseq BLEU: 42.3 http://matrix.statmt.org/matrix/output/1902?run_id=6750)
# en-de
export PAIR=en-de
export DATA_DIR=data/$PAIR
export SAVE_DIR=data/$PAIR
export BS=8
mkdir -p $DATA_DIR
sacrebleu -t wmt19 -l $PAIR --echo src > $DATA_DIR/val.source
sacrebleu -t wmt19 -l $PAIR --echo ref > $DATA_DIR/val.target
echo $PAIR
PYTHONPATH="src:examples/seq2seq" python examples/seq2seq/run_eval.py facebook/wmt19-$PAIR $DATA_DIR/val.source $SAVE_DIR/test_translations.txt --reference_path $DATA_DIR/val.target --score_path $SAVE_DIR/test_bleu.json --bs $BS --task translation --num_beams $NUM_BEAMS
# (fairseq BLEU: 43.1 http://matrix.statmt.org/matrix/output/1909?run_id=6862)
"""
FSMT_START_DOCSTRING = r"""
This model inherits from [`PreTrainedModel`]. Check the superclass documentation for the generic methods the
library implements for all its model (such as downloading or saving, resizing the input embeddings, pruning heads
etc.)
This model is also a PyTorch [torch.nn.Module](https://pytorch.org/docs/stable/nn.html#torch.nn.Module) subclass.
Use it as a regular PyTorch Module and refer to the PyTorch documentation for all matter related to general usage
and behavior.
Parameters:
config ([`FSMTConfig`]): Model configuration class with all the parameters of the model.
Initializing with a config file does not load the weights associated with the model, only the
configuration. Check out the [`~PreTrainedModel.from_pretrained`] method to load the model weights.
"""
FSMT_GENERATION_EXAMPLE = r"""
Translation example::
```python
>>> from transformers import AutoTokenizer, FSMTForConditionalGeneration
>>> mname = "facebook/wmt19-ru-en"
>>> model = FSMTForConditionalGeneration.from_pretrained(mname)
>>> tokenizer = AutoTokenizer.from_pretrained(mname)
>>> src_text = "Машинное обучение - это здорово, не так ли?"
>>> input_ids = tokenizer(src_text, return_tensors="pt").input_ids
>>> outputs = model.generate(input_ids, num_beams=5, num_return_sequences=3)
>>> tokenizer.decode(outputs[0], skip_special_tokens=True)
"Machine learning is great, isn't it?"
```
"""
FSMT_INPUTS_DOCSTRING = r"""
Args:
input_ids (`torch.LongTensor` of shape `(batch_size, sequence_length)`):
Indices of input sequence tokens in the vocabulary.
Indices can be obtained using [`FSTMTokenizer`]. See [`PreTrainedTokenizer.encode`] and
[`PreTrainedTokenizer.__call__`] for details.
[What are input IDs?](../glossary#input-ids)
attention_mask (`torch.Tensor` of shape `(batch_size, sequence_length)`, *optional*):
Mask to avoid performing attention on padding token indices. Mask values selected in `[0, 1]`:
- 1 for tokens that are **not masked**,
- 0 for tokens that are **masked**.
[What are attention masks?](../glossary#attention-mask)
decoder_input_ids (`torch.LongTensor` of shape `(batch_size, target_sequence_length)`, *optional*):
Indices of decoder input sequence tokens in the vocabulary.
Indices can be obtained using [`AutoTokenizer`]. See [`PreTrainedTokenizer.encode`] and
[`PreTrainedTokenizer.__call__`] for details.
[What are decoder input IDs?](../glossary#decoder-input-ids)
FSMT uses the `eos_token_id` as the starting token for `decoder_input_ids` generation. If `past_key_values`
is used, optionally only the last `decoder_input_ids` have to be input (see `past_key_values`).
decoder_attention_mask (`torch.BoolTensor` of shape `(batch_size, target_sequence_length)`, *optional*):
Default behavior: generate a tensor that ignores pad tokens in `decoder_input_ids`. Causal mask will also
be used by default.
head_mask (`torch.Tensor` of shape `(encoder_layers, encoder_attention_heads)`, *optional*):
Mask to nullify selected heads of the attention modules in the encoder. Mask values selected in `[0, 1]`:
- 1 indicates the head is **not masked**,
- 0 indicates the head is **masked**.
decoder_head_mask (`torch.Tensor` of shape `(decoder_layers, decoder_attention_heads)`, *optional*):
Mask to nullify selected heads of the attention modules in the decoder. Mask values selected in `[0, 1]`:
- 1 indicates the head is **not masked**,
- 0 indicates the head is **masked**.
cross_attn_head_mask (`torch.Tensor` of shape `(decoder_layers, decoder_attention_heads)`, *optional*):
Mask to nullify selected heads of the cross-attention modules in the decoder. Mask values selected in `[0,
1]`:
- 1 indicates the head is **not masked**,
- 0 indicates the head is **masked**.
encoder_outputs (`Tuple(torch.FloatTensor)`, *optional*):
Tuple consists of (`last_hidden_state`, *optional*: `hidden_states`, *optional*: `attentions`)
`last_hidden_state` of shape `(batch_size, sequence_length, hidden_size)` is a sequence of hidden-states at
the output of the last layer of the encoder. Used in the cross-attention of the decoder.
past_key_values (`Tuple(torch.FloatTensor)` of length `config.n_layers` with each tuple having 4 tensors of shape `(batch_size, num_heads, sequence_length - 1, embed_size_per_head)`):
Contains precomputed key and value hidden-states of the attention blocks. Can be used to speed up decoding.
If `past_key_values` are used, the user can optionally input only the last `decoder_input_ids` (those that
don't have their past key value states given to this model) of shape `(batch_size, 1)` instead of all
`decoder_input_ids` of shape `(batch_size, sequence_length)`.
inputs_embeds (`torch.FloatTensor` of shape `(batch_size, sequence_length, hidden_size)`, *optional*):
Optionally, instead of passing `input_ids` you can choose to directly pass an embedded representation. This
is useful if you want more control over how to convert `input_ids` indices into associated vectors than the
model's internal embedding lookup matrix.
decoder_inputs_embeds (`torch.FloatTensor` of shape `(batch_size, target_sequence_length, hidden_size)`, *optional*):
Optionally, instead of passing `decoder_input_ids` you can choose to directly pass an embedded
representation. If `past_key_values` is used, optionally only the last `decoder_inputs_embeds` have to be
input (see `past_key_values`). This is useful if you want more control over how to convert
`decoder_input_ids` indices into associated vectors than the model's internal embedding lookup matrix.
If `decoder_input_ids` and `decoder_inputs_embeds` are both unset, `decoder_inputs_embeds` takes the value
of `inputs_embeds`.
use_cache (`bool`, *optional*, defaults to `True`):
If set to `True`, `past_key_values` key value states are returned and can be used to speed up decoding (see
`past_key_values`).
output_attentions (`bool`, *optional*):
Whether or not to return the attentions tensors of all attention layers. See `attentions` under returned
tensors for more detail.
output_hidden_states (`bool`, *optional*):
Whether or not to return the hidden states of all layers. See `hidden_states` under returned tensors for
more detail.
return_dict (`bool`, *optional*):
Whether or not to return a [`~utils.ModelOutput`] instead of a plain tuple.
"""
def invert_mask(attention_mask):
"""Turns 1->0, 0->1, False->True, True-> False"""
assert attention_mask.dim() == 2
return attention_mask.eq(0)
def triu_onnx(x, diagonal=0):
l = x.shape[0]
arange = torch.arange(l, device=x.device)
mask = arange.expand(l, l)
arange = arange.unsqueeze(-1)
if diagonal:
arange = arange + diagonal
mask = mask >= arange
return x.masked_fill(mask == 0, 0)
def _prepare_fsmt_decoder_inputs(
config,
input_ids,
decoder_input_ids=None,
decoder_padding_mask=None,
causal_mask_dtype=torch.float32,
):
"""
Prepare masks that ignore padding tokens in the decoder and a causal mask for the decoder if none are provided.
This mimics the default behavior in fairseq. To override it pass in masks. Note: this is not called during
generation
"""
pad_token_id = config.pad_token_id
if decoder_input_ids is None:
decoder_input_ids = shift_tokens_right(input_ids, pad_token_id)
bsz, tgt_len = decoder_input_ids.size()
if decoder_padding_mask is None:
decoder_padding_mask = make_padding_mask(decoder_input_ids, pad_token_id)
else:
decoder_padding_mask = invert_mask(decoder_padding_mask)
causal_mask = triu_onnx(fill_with_neg_inf(torch.zeros(tgt_len, tgt_len, dtype=causal_mask_dtype)), 1).to(
device=decoder_input_ids.device
)
return decoder_input_ids, decoder_padding_mask, causal_mask
class PretrainedFSMTModel(PreTrainedModel):
config_class = FSMTConfig
base_model_prefix = "model"
def _init_weights(self, module):
std = self.config.init_std
if isinstance(module, nn.Linear):
module.weight.data.normal_(mean=0.0, std=std)
if module.bias is not None:
module.bias.data.zero_()
elif isinstance(module, SinusoidalPositionalEmbedding):
pass
elif isinstance(module, nn.Embedding):
module.weight.data.normal_(mean=0.0, std=std)
if module.padding_idx is not None:
module.weight.data[module.padding_idx].zero_()
@property
def dummy_inputs(self):
pad_token = self.config.pad_token_id
input_ids = torch.tensor([[0, 6, 10, 4, 2], [0, 8, 12, 2, pad_token]], device=self.device)
dummy_inputs = {
"attention_mask": input_ids.ne(pad_token),
"input_ids": input_ids,
}
return dummy_inputs
def _make_linear_from_emb(emb):
vocab_size, emb_size = emb.weight.shape
lin_layer = nn.Linear(vocab_size, emb_size, bias=False)
lin_layer.weight.data = emb.weight.data
return lin_layer
# Helper Functions, mostly for making masks
def _check_shapes(shape_1, shape2):
if shape_1 != shape2:
raise AssertionError(f"shape mismatch: {shape_1} != {shape2}")
def shift_tokens_right(input_ids, pad_token_id):
"""Shift input ids one token to the right, and wrap the last non pad token (usually <eos>)."""
# replace possible -100 values in labels by `pad_token_id`
input_ids.masked_fill_(input_ids == -100, pad_token_id)
prev_output_tokens = input_ids.clone()
index_of_eos = (input_ids.ne(pad_token_id).sum(dim=1) - 1).unsqueeze(-1)
prev_output_tokens[:, 0] = input_ids.gather(1, index_of_eos).squeeze()
prev_output_tokens[:, 1:] = input_ids[:, :-1]
return prev_output_tokens
def make_padding_mask(input_ids, padding_idx=1):
"""True for pad tokens"""
padding_mask = input_ids.eq(padding_idx)
if not padding_mask.any():
padding_mask = None
return padding_mask
# Helper Modules
class EncoderLayer(nn.Module):
def __init__(self, config: FSMTConfig):
super().__init__()
self.embed_dim = config.d_model
self.self_attn = Attention(self.embed_dim, config.encoder_attention_heads, dropout=config.attention_dropout)
self.self_attn_layer_norm = LayerNorm(self.embed_dim)
self.dropout = config.dropout
self.activation_fn = ACT2FN[config.activation_function]
self.activation_dropout = config.activation_dropout
self.fc1 = nn.Linear(self.embed_dim, config.encoder_ffn_dim)
self.fc2 = nn.Linear(config.encoder_ffn_dim, self.embed_dim)
self.final_layer_norm = LayerNorm(self.embed_dim)
def forward(self, x, encoder_padding_mask, layer_head_mask, output_attentions=False):
"""
Args:
x (`torch.Tensor`): input to the layer of shape *(seq_len, batch, embed_dim)*
encoder_padding_mask (`torch.ByteTensor`): binary ByteTensor of shape
*(batch, src_len)* where padding elements are indicated by `1`.
for t_tgt, t_src is excluded (or masked out), =0 means it is
included in attention
layer_head_mask (`torch.FloatTensor`): mask for attention heads in a given layer of size
*(config.encoder_attention_heads,)*.
Returns:
encoded output of shape *(seq_len, batch, embed_dim)*
"""
residual = x
x, attn_weights = self.self_attn(
query=x,
key=x,
key_padding_mask=encoder_padding_mask,
layer_head_mask=layer_head_mask,
output_attentions=output_attentions,
)
x = nn.functional.dropout(x, p=self.dropout, training=self.training)
x = residual + x
x = self.self_attn_layer_norm(x)
residual = x
x = self.activation_fn(self.fc1(x))
x = nn.functional.dropout(x, p=self.activation_dropout, training=self.training)
x = self.fc2(x)
x = nn.functional.dropout(x, p=self.dropout, training=self.training)
x = residual + x
x = self.final_layer_norm(x)
return x, attn_weights
class FSMTEncoder(nn.Module):
"""
Transformer encoder consisting of *config.encoder_layers* self attention layers. Each layer is a [`EncoderLayer`].
Args:
config: FSMTConfig
"""
def __init__(self, config: FSMTConfig, embed_tokens):
super().__init__()
self.dropout = config.dropout
self.layerdrop = config.encoder_layerdrop
self.padding_idx = embed_tokens.padding_idx
self.embed_tokens = embed_tokens
embed_dim = embed_tokens.embedding_dim
self.embed_scale = math.sqrt(embed_dim) if config.scale_embedding else 1.0
self.embed_positions = SinusoidalPositionalEmbedding(
config.max_position_embeddings + self.padding_idx + 1, embed_dim, self.padding_idx
)
self.layers = nn.ModuleList([EncoderLayer(config) for _ in range(config.encoder_layers)]) # type: List[EncoderLayer]
def forward(
self,
input_ids: torch.Tensor,
attention_mask: Optional[torch.Tensor] = None,
inputs_embeds: torch.Tensor = None,
head_mask: Optional[torch.Tensor] = None,
output_attentions: bool = False,
output_hidden_states: bool = False,
return_dict: bool = True,
):
"""
Args:
input_ids (`torch.LongTensor`): tokens in the source language of shape
*(batch, src_len)*
attention_mask (`torch.LongTensor`): indicating which indices are padding tokens
inputs_embeds (`torch.FloatTensor`):
embedding vectors of shape *(batch, src_len, embed_dim)*
head_mask (`torch.Tensor` of shape `(num_layers, num_heads)`, *optional*):
Mask to nullify selected heads of the attention modules. Mask values selected in `[0, 1]`:
- 1 indicates the head is **not masked**,
- 0 indicates the head is **masked**.
Returns:
BaseModelOutput or Tuple comprised of:
- **x** (`torch.Tensor`): the last encoder layer's output of shape *(src_len, batch, embed_dim)*
- **encoder_states** (`Tuple(torch.FloatTensor`)): all intermediate hidden states of shape *(src_len,
batch, embed_dim)*. Only populated if *output_hidden_states:* is True.
- **all_attentions** (`Tuple(torch.FloatTensor`)): Attention weights for each layer.
During training might not be of length n_layers because of layer dropout.
"""
# check attention mask and invert
if attention_mask is not None:
attention_mask = invert_mask(attention_mask)
if input_ids is not None and inputs_embeds is not None:
raise ValueError("You cannot specify both input_ids and inputs_embeds at the same time")
elif input_ids is not None:
inputs_embeds = self.embed_tokens(input_ids) * self.embed_scale
embed_pos = self.embed_positions(input_ids)
elif inputs_embeds is not None:
inputs_embeds = inputs_embeds * self.embed_scale
# We assume zeros hidden states correspond to padding tokens
# and create `position_ids` where inputs_embeds[:, :, 0] == 0
position_ids = inputs_embeds[:, :, 0].masked_fill(
inputs_embeds[:, :, 0].eq(0), self.embed_positions.padding_idx
)
embed_pos = self.embed_positions(position_ids)
else:
raise ValueError("You have to specify either input_ids or inputs_embeds")
x = inputs_embeds + embed_pos
x = nn.functional.dropout(x, p=self.dropout, training=self.training)
# B x T x C -> T x B x C
x = x.transpose(0, 1)
encoder_states = () if output_hidden_states else None
all_attentions = () if output_attentions else None
# check if head_mask has a correct number of layers specified if desired
if head_mask is not None:
assert head_mask.size()[0] == (
len(self.layers)
), f"The head_mask should be specified for {len(self.layers)} layers, but it is for {head_mask.size()[0]}."
for idx, encoder_layer in enumerate(self.layers):
if output_hidden_states:
x = x.transpose(0, 1) # T x B x C -> B x T x C
encoder_states += (x,)
x = x.transpose(0, 1) # B x T x C -> T x B x C
# add LayerDrop (see https://arxiv.org/abs/1909.11556 for description)
dropout_probability = torch.rand([])
if self.training and (dropout_probability < self.layerdrop): # skip the layer
attn = None
else:
x, attn = encoder_layer(
x,
attention_mask,
layer_head_mask=(head_mask[idx] if head_mask is not None else None),
output_attentions=output_attentions,
)
if output_attentions:
all_attentions = all_attentions + (attn,)
# T x B x C -> B x T x C
x = x.transpose(0, 1)
if output_hidden_states:
encoder_states += (x,)
if not return_dict:
return tuple(v for v in [x, encoder_states, all_attentions] if v is not None)
return BaseModelOutput(last_hidden_state=x, hidden_states=encoder_states, attentions=all_attentions)
class DecoderLayer(nn.Module):
def __init__(self, config: FSMTConfig):
super().__init__()
self.embed_dim = config.d_model
self.self_attn = Attention(
embed_dim=self.embed_dim,
num_heads=config.decoder_attention_heads,
dropout=config.attention_dropout,
)
self.dropout = config.dropout
self.activation_fn = ACT2FN[config.activation_function]
self.activation_dropout = config.activation_dropout
self.self_attn_layer_norm = LayerNorm(self.embed_dim)
self.encoder_attn = Attention(
self.embed_dim,
config.decoder_attention_heads,
dropout=config.attention_dropout,
encoder_decoder_attention=True,
)
self.encoder_attn_layer_norm = LayerNorm(self.embed_dim)
self.fc1 = nn.Linear(self.embed_dim, config.decoder_ffn_dim)
self.fc2 = nn.Linear(config.decoder_ffn_dim, self.embed_dim)
self.final_layer_norm = LayerNorm(self.embed_dim)
def forward(
self,
x,
encoder_hidden_states,
encoder_attn_mask=None,
layer_state=None,
causal_mask=None,
layer_head_mask=None,
cross_attn_layer_head_mask=None,
decoder_padding_mask=None,
output_attentions=False,
):
residual = x
if layer_state is None:
layer_state = {}
# Self Attention
x, self_attn_weights = self.self_attn(
query=x,
key=x,
layer_state=layer_state, # adds keys to layer state
key_padding_mask=decoder_padding_mask,
attn_mask=causal_mask,
layer_head_mask=layer_head_mask,
output_attentions=output_attentions,
)
x = nn.functional.dropout(x, p=self.dropout, training=self.training)
x = residual + x
x = self.self_attn_layer_norm(x)
# Cross attention
residual = x
assert self.encoder_attn.cache_key != self.self_attn.cache_key
x, cross_attn_weights = self.encoder_attn(
query=x,
key=encoder_hidden_states,
key_padding_mask=encoder_attn_mask,
layer_state=layer_state, # mutates layer state
layer_head_mask=cross_attn_layer_head_mask,
output_attentions=output_attentions,
)
x = nn.functional.dropout(x, p=self.dropout, training=self.training)
x = residual + x
x = self.encoder_attn_layer_norm(x)
# Fully Connected
residual = x
x = self.activation_fn(self.fc1(x))
x = nn.functional.dropout(x, p=self.activation_dropout, training=self.training)
x = self.fc2(x)
x = nn.functional.dropout(x, p=self.dropout, training=self.training)
x = residual + x
x = self.final_layer_norm(x)
return (
x,
self_attn_weights,
layer_state,
cross_attn_weights,
) # layer_state = cache for decoding
class FSMTDecoder(nn.Module):
"""
Transformer decoder consisting of *config.decoder_layers* layers. Each layer is a [`DecoderLayer`]
Args:
config: FSMTConfig
embed_tokens (nn.Embedding): output embedding
"""
def __init__(self, config: FSMTConfig, embed_tokens: nn.Embedding):
super().__init__()
self.dropout = config.dropout
self.layerdrop = config.decoder_layerdrop
self.padding_idx = embed_tokens.padding_idx
self.embed_scale = math.sqrt(config.d_model) if config.scale_embedding else 1.0
self.embed_tokens = embed_tokens
embed_dim = embed_tokens.embedding_dim
self.embed_positions = SinusoidalPositionalEmbedding(
config.max_position_embeddings + self.padding_idx + 1, embed_dim, self.padding_idx
)
self.layers = nn.ModuleList([DecoderLayer(config) for _ in range(config.decoder_layers)]) # type: List[DecoderLayer]
if is_deepspeed_zero3_enabled():
import deepspeed
with deepspeed.zero.GatheredParameters(self.embed_tokens.weight, modifier_rank=None):
embed_tokens_weight_shape = self.embed_tokens.weight.shape
else:
embed_tokens_weight_shape = self.embed_tokens.weight.shape
self.output_projection = nn.Linear(embed_tokens_weight_shape[1], embed_tokens_weight_shape[0], bias=False)
self.output_projection.weight = self.embed_tokens.weight
def forward(
self,
input_ids: torch.Tensor,
encoder_hidden_states: torch.Tensor,
encoder_padding_mask: torch.Tensor,
decoder_padding_mask: torch.Tensor,
decoder_causal_mask: torch.Tensor,
head_mask: Optional[torch.Tensor] = None,
inputs_embeds: Optional[torch.Tensor] = None,
cross_attn_head_mask: Optional[torch.Tensor] = None,
past_key_values: Optional[List[torch.FloatTensor]] = None,
use_cache: bool = False,
output_attentions: bool = False,
output_hidden_states: bool = False,
return_dict: bool = True,
):
"""
Includes several features from "Jointly Learning to Align and Translate with Transformer Models" (Garg et al.,
EMNLP 2019).
Args:
input_ids (`torch.LongTensor` of shape `(batch, tgt_len)`):
previous decoder outputs for teacher forcing
encoder_hidden_states: output from the encoder, used for
encoder-side attention
encoder_padding_mask: for ignoring pad tokens
past_key_values (dict or None): dictionary used for storing state during generation
head_mask (`torch.Tensor` of shape `(num_layers, num_heads)`, *optional*):
Mask to nullify selected heads of the attention modules. Mask values selected in `[0, 1]`:
- 1 indicates the head is **not masked**,
- 0 indicates the head is **masked**.
cross_attn_head_mask (`torch.Tensor` of shape `(num_layers, num_heads)`, *optional*):
Mask to nullify selected heads of the cross-attention modules. Mask values selected in `[0, 1]`:
- 1 indicates the head is **not masked**,
- 0 indicates the head is **masked**.
Returns:
BaseModelOutputWithPast or tuple:
- the decoder's features of shape *(batch, tgt_len, embed_dim)*
- the cache
- hidden states
- attentions
"""
# check attention mask and invert
if encoder_padding_mask is not None:
encoder_padding_mask = invert_mask(encoder_padding_mask)
if input_ids is not None and inputs_embeds is not None:
raise ValueError("You cannot specify both decoder_input_ids and decoder_inputs_embeds at the same time")
elif input_ids is not None:
# embed positions
positions = self.embed_positions(input_ids)
if use_cache:
input_ids = input_ids[:, -1:]
positions = positions[:, -1:] # happens after we embed them
x = self.embed_tokens(input_ids) * self.embed_scale
elif inputs_embeds is not None:
# We assume zeros hidden states correspond to padding tokens
# and create `position_ids` where inputs_embeds[:, :, 0] == 0
position_ids = inputs_embeds[:, :, 0].masked_fill(
inputs_embeds[:, :, 0].eq(0), self.embed_positions.padding_idx
)
positions = self.embed_positions(position_ids)
x = inputs_embeds * self.embed_scale
else:
raise ValueError("You have to specify either decoder_input_ids or decoder_inputs_embeds")
x += positions
x = nn.functional.dropout(x, p=self.dropout, training=self.training)
# Convert to FSMT output format: (BS, seq_len, model_dim) -> (seq_len, BS, model_dim)
x = x.transpose(0, 1)
encoder_hidden_states = encoder_hidden_states.transpose(0, 1)
# decoder layers
all_hidden_states = () if output_hidden_states else None
all_self_attns = () if output_attentions else None
all_cross_attns = () if output_attentions else None
next_decoder_cache = []
# check if head_mask has a correct number of layers specified if desired
for attn_mask, mask_name in zip([head_mask, cross_attn_head_mask], ["head_mask", "cross_attn_head_mask"]):
if attn_mask is not None:
assert attn_mask.size()[0] == (len(self.layers)), (
f"The `{mask_name}` should be specified for {len(self.layers)} layers, but it is for"
f" {head_mask.size()[0]}."
)
for idx, decoder_layer in enumerate(self.layers):
# add LayerDrop (see https://arxiv.org/abs/1909.11556 for description)
if output_hidden_states:
x = x.transpose(0, 1)
all_hidden_states += (x,)
x = x.transpose(0, 1)
if self.training:
dropout_probability = torch.rand([])
if dropout_probability < self.layerdrop:
continue
layer_state = past_key_values[idx] if past_key_values is not None else None
x, layer_self_attn, layer_past, layer_cross_attn = decoder_layer(
x,
encoder_hidden_states,
encoder_attn_mask=encoder_padding_mask,
decoder_padding_mask=decoder_padding_mask,
layer_state=layer_state,
causal_mask=decoder_causal_mask,
layer_head_mask=(head_mask[idx] if head_mask is not None else None),
cross_attn_layer_head_mask=(cross_attn_head_mask[idx] if cross_attn_head_mask is not None else None),
output_attentions=output_attentions,
)
if use_cache:
next_decoder_cache.append(layer_past.copy())
if output_attentions:
all_self_attns += (layer_self_attn,)
all_cross_attns += (layer_cross_attn,)
# add hidden states from the last decoder layer
if output_hidden_states:
x = x.transpose(0, 1)
all_hidden_states += (x,)
x = x.transpose(0, 1)
# Convert to standard output format: (seq_len, BS, model_dim) -> (BS, seq_len, model_dim)
x = x.transpose(0, 1)
encoder_hidden_states = encoder_hidden_states.transpose(0, 1)
x = self.output_projection(x)
next_cache = next_decoder_cache if use_cache else None
if not return_dict:
return tuple(
v for v in [x, next_cache, all_hidden_states, all_self_attns, all_cross_attns] if v is not None
)
return BaseModelOutputWithPastAndCrossAttentions(
last_hidden_state=x,
past_key_values=next_cache,
hidden_states=all_hidden_states,
attentions=all_self_attns,
cross_attentions=all_cross_attns,
)
def _reorder_buffer(attn_cache, new_order):
for k, input_buffer_k in attn_cache.items():
if input_buffer_k is not None:
attn_cache[k] = input_buffer_k.index_select(0, new_order)
return attn_cache
class Attention(nn.Module):
"""Multi-headed attention from 'Attention Is All You Need' paper"""
def __init__(
self,
embed_dim,
num_heads,
dropout=0.0,
bias=True,
encoder_decoder_attention=False, # otherwise self_attention
):
super().__init__()
self.embed_dim = embed_dim
self.num_heads = num_heads
self.dropout = dropout
self.head_dim = embed_dim // num_heads
assert self.head_dim * num_heads == self.embed_dim, "embed_dim must be divisible by num_heads"
self.scaling = self.head_dim**-0.5
self.encoder_decoder_attention = encoder_decoder_attention
self.k_proj = nn.Linear(embed_dim, embed_dim, bias=bias)
self.v_proj = nn.Linear(embed_dim, embed_dim, bias=bias)
self.q_proj = nn.Linear(embed_dim, embed_dim, bias=bias)
self.out_proj = nn.Linear(embed_dim, embed_dim, bias=bias)
self.cache_key = "encoder_decoder" if self.encoder_decoder_attention else "self"
def _shape(self, tensor, seq_len, bsz):
return tensor.contiguous().view(seq_len, bsz * self.num_heads, self.head_dim).transpose(0, 1)
def forward(
self,
query,
key: Optional[Tensor],
key_padding_mask: Optional[Tensor] = None,
layer_state: Optional[Dict[str, Optional[Tensor]]] = None,
attn_mask: Optional[Tensor] = None,
layer_head_mask: Optional[Tensor] = None,
output_attentions=False,
) -> Tuple[Tensor, Optional[Tensor]]:
"""Input shape: Time(SeqLen) x Batch x Channel"""
static_kv: bool = self.encoder_decoder_attention
tgt_len, bsz, embed_dim = query.size()
assert embed_dim == self.embed_dim
assert list(query.size()) == [tgt_len, bsz, embed_dim]
# get here for encoder decoder cause of static_kv
if layer_state is not None: # reuse k,v and encoder_padding_mask
saved_state = layer_state.get(self.cache_key, {})
if "prev_key" in saved_state and static_kv:
# previous time steps are cached - no need to recompute key and value if they are static
key = None
else:
saved_state = None
layer_state = {}
q = self.q_proj(query) * self.scaling
if static_kv:
if key is None:
k = v = None
else:
k = self.k_proj(key)
v = self.v_proj(key)
else:
k = self.k_proj(query)
v = self.v_proj(query)
q = self._shape(q, tgt_len, bsz)
if k is not None:
k = self._shape(k, -1, bsz)
if v is not None:
v = self._shape(v, -1, bsz)
if saved_state is not None:
k, v, key_padding_mask = self._use_saved_state(k, v, saved_state, key_padding_mask, static_kv, bsz)
# Update cache
layer_state[self.cache_key] = {
"prev_key": k.view(bsz, self.num_heads, -1, self.head_dim),
"prev_value": v.view(bsz, self.num_heads, -1, self.head_dim),
"prev_key_padding_mask": key_padding_mask if not static_kv else None,
}
assert k is not None
src_len = k.size(1)
attn_weights = torch.bmm(q, k.transpose(1, 2))
assert attn_weights.size() == (bsz * self.num_heads, tgt_len, src_len)
if attn_mask is not None:
attn_weights = attn_weights.view(bsz, self.num_heads, tgt_len, src_len) + attn_mask
attn_weights = attn_weights.view(bsz * self.num_heads, tgt_len, src_len)
# This is part of a workaround to get around fork/join parallelism not supporting Optional types.
if key_padding_mask is not None and key_padding_mask.dim() == 0:
key_padding_mask = None
assert key_padding_mask is None or key_padding_mask.size()[:2] == (
bsz,
src_len,
)
if key_padding_mask is not None: # don't attend to padding symbols
attn_weights = attn_weights.view(bsz, self.num_heads, tgt_len, src_len)
reshaped = key_padding_mask.unsqueeze(1).unsqueeze(2)
attn_weights = attn_weights.masked_fill(reshaped, torch.finfo(attn_weights.dtype).min)
attn_weights = attn_weights.view(bsz * self.num_heads, tgt_len, src_len)
attn_weights = nn.functional.softmax(attn_weights, dim=-1)
if layer_head_mask is not None:
assert layer_head_mask.size() == (
self.num_heads,
), f"Head mask for a single layer should be of size {(self.num_heads,)}, but is {layer_head_mask.size()}"
attn_weights = layer_head_mask.view(1, -1, 1, 1) * attn_weights.view(bsz, self.num_heads, tgt_len, src_len)
attn_weights = attn_weights.view(bsz * self.num_heads, tgt_len, src_len)
if output_attentions:
# make sure that attn_weights are included in graph
attn_weights_reshaped = attn_weights.view(bsz, self.num_heads, tgt_len, src_len)
attn_weights = attn_weights_reshaped.view(bsz * self.num_heads, tgt_len, src_len)
else:
attn_weights_reshaped = None
attn_probs = nn.functional.dropout(
attn_weights,
p=self.dropout,
training=self.training,
)
assert v is not None
attn_output = torch.bmm(attn_probs, v)
assert attn_output.size() == (bsz * self.num_heads, tgt_len, self.head_dim)
attn_output = attn_output.transpose(0, 1).contiguous().view(tgt_len, bsz, embed_dim)
attn_output = self.out_proj(attn_output)
return attn_output, attn_weights_reshaped
def _use_saved_state(self, k, v, saved_state, key_padding_mask, static_kv, bsz):
# saved states are stored with shape (bsz, num_heads, seq_len, head_dim)
if "prev_key" in saved_state:
_prev_key = saved_state["prev_key"]
assert _prev_key is not None
prev_key = _prev_key.view(bsz * self.num_heads, -1, self.head_dim)
if static_kv:
k = prev_key
else:
assert k is not None
k = torch.cat([prev_key, k], dim=1)
if "prev_value" in saved_state:
_prev_value = saved_state["prev_value"]
assert _prev_value is not None
prev_value = _prev_value.view(bsz * self.num_heads, -1, self.head_dim)
if static_kv:
v = prev_value
else:
assert v is not None
v = torch.cat([prev_value, v], dim=1)
assert k is not None and v is not None
prev_key_padding_mask: Optional[Tensor] = saved_state.get("prev_key_padding_mask", None)
if prev_key_padding_mask is not None:
if static_kv:
new_key_padding_mask = prev_key_padding_mask
else:
new_key_padding_mask = torch.cat([prev_key_padding_mask, key_padding_mask], dim=1)
else:
new_key_padding_mask = key_padding_mask
return k, v, new_key_padding_mask
def fill_with_neg_inf(t):
"""FP16-compatible function that fills a input_ids with -inf."""
return t.float().fill_(torch.finfo(t.dtype).min).type_as(t)
# Public API
def _get_shape(t):
return getattr(t, "shape", None)
@add_start_docstrings(
"The bare FSMT Model outputting raw hidden-states without any specific head on top.",
FSMT_START_DOCSTRING,
)
class FSMTModel(PretrainedFSMTModel):
_tied_weights_keys = ["decoder.embed_tokens.weight", "decoder.output_projection.weight"]
def __init__(self, config: FSMTConfig):
super().__init__(config)
padding_idx = config.pad_token_id
encoder_embed_tokens = nn.Embedding(config.src_vocab_size, config.d_model, padding_idx)
decoder_embed_tokens = nn.Embedding(config.tgt_vocab_size, config.d_model, padding_idx)
self.encoder = FSMTEncoder(config, encoder_embed_tokens)
self.decoder = FSMTDecoder(config, decoder_embed_tokens)
# Initialize weights and apply final processing
self.post_init()
def get_encoder(self):
return self.encoder
def get_decoder(self):
return self.decoder
def _tie_weights(self):
if self.config.tie_word_embeddings:
self._tie_or_clone_weights(self.decoder.embed_tokens, self.get_input_embeddings())
self._tie_or_clone_weights(self.decoder.output_projection, self.get_input_embeddings())
@add_start_docstrings_to_model_forward(FSMT_INPUTS_DOCSTRING)
@add_code_sample_docstrings(
checkpoint=_CHECKPOINT_FOR_DOC,
output_type=Seq2SeqModelOutput,
config_class=_CONFIG_FOR_DOC,
)
def forward(
self,
input_ids: torch.LongTensor,
attention_mask: Optional[torch.Tensor] = None,
decoder_input_ids: Optional[torch.LongTensor] = None,
decoder_attention_mask: Optional[torch.BoolTensor] = None,
head_mask: Optional[torch.Tensor] = None,
decoder_head_mask: Optional[torch.Tensor] = None,
cross_attn_head_mask: Optional[torch.Tensor] = None,
encoder_outputs: Optional[Tuple[torch.FloatTensor]] = None,
past_key_values: Optional[Tuple[torch.FloatTensor]] = None,
use_cache: Optional[bool] = None,
output_attentions: Optional[bool] = None,
output_hidden_states: Optional[bool] = None,
inputs_embeds: Optional[torch.FloatTensor] = None,
decoder_inputs_embeds: Optional[torch.FloatTensor] = None,
return_dict: Optional[bool] = None,
) -> Union[Tuple[torch.Tensor], Seq2SeqModelOutput]:
if decoder_input_ids is None:
use_cache = False
output_attentions = output_attentions if output_attentions is not None else self.config.output_attentions
output_hidden_states = (
output_hidden_states if output_hidden_states is not None else self.config.output_hidden_states
)
use_cache = use_cache if use_cache is not None else self.config.use_cache
return_dict = return_dict if return_dict is not None else self.config.use_return_dict
# make masks if user doesn't supply
if not use_cache and input_ids is not None:
decoder_input_ids, decoder_padding_mask, causal_mask = _prepare_fsmt_decoder_inputs(
self.config,
input_ids,
decoder_input_ids=decoder_input_ids,
decoder_padding_mask=decoder_attention_mask,
causal_mask_dtype=self.decoder.embed_tokens.weight.dtype,
)
else:
decoder_padding_mask, causal_mask = None, None
if decoder_input_ids is None and decoder_inputs_embeds is None:
raise ValueError("Make sure that `decoder_input_ids` or `decoder_inputs_embeds` are passed.")
if encoder_outputs is None:
encoder_outputs = self.encoder(
input_ids=input_ids,
attention_mask=attention_mask,
inputs_embeds=inputs_embeds,
head_mask=head_mask,
output_attentions=output_attentions,
output_hidden_states=output_hidden_states,
return_dict=return_dict,
)
# If the user passed a tuple for encoder_outputs, we wrap it in a BaseModelOutput when return_dict=False
elif return_dict and not isinstance(encoder_outputs, BaseModelOutput):
encoder_outputs = BaseModelOutput(
last_hidden_state=encoder_outputs[0],
hidden_states=encoder_outputs[1] if len(encoder_outputs) > 1 else None,
attentions=encoder_outputs[2] if len(encoder_outputs) > 2 else None,
)
# decoder outputs consists of (dec_features, layer_state, dec_hidden, dec_attn)
decoder_outputs = self.decoder(
decoder_input_ids,
encoder_outputs[0],
attention_mask,
decoder_padding_mask,
decoder_causal_mask=causal_mask,
inputs_embeds=decoder_inputs_embeds,
head_mask=decoder_head_mask,
cross_attn_head_mask=cross_attn_head_mask,
past_key_values=past_key_values,
use_cache=use_cache,
output_attentions=output_attentions,
output_hidden_states=output_hidden_states,
return_dict=return_dict,
)
if not return_dict:
return decoder_outputs + encoder_outputs
return Seq2SeqModelOutput(
last_hidden_state=decoder_outputs.last_hidden_state,
past_key_values=decoder_outputs.past_key_values,
decoder_hidden_states=decoder_outputs.hidden_states,
decoder_attentions=decoder_outputs.attentions,
cross_attentions=decoder_outputs.cross_attentions,
encoder_last_hidden_state=encoder_outputs.last_hidden_state,
encoder_hidden_states=encoder_outputs.hidden_states,
encoder_attentions=encoder_outputs.attentions,
)
def get_input_embeddings(self):
return self.encoder.embed_tokens
def set_input_embeddings(self, value):
self.encoder.embed_tokens = value
def get_output_embeddings(self):
return self.decoder.embed_tokens
def set_output_embeddings(self, value):
self.decoder.embed_tokens = value
@add_start_docstrings(
"The FSMT Model with a language modeling head. Can be used for summarization.", FSMT_START_DOCSTRING
)
class FSMTForConditionalGeneration(PretrainedFSMTModel):
base_model_prefix = "model"
_tied_weights_keys = ["decoder.embed_tokens.weight", "decoder.output_projection.weight"]
def __init__(self, config: FSMTConfig):
super().__init__(config)
base_model = FSMTModel(config)
self.model = base_model
# Initialize weights and apply final processing
self.post_init()
@add_start_docstrings_to_model_forward(FSMT_INPUTS_DOCSTRING)
@replace_return_docstrings(output_type=Seq2SeqLMOutput, config_class=_CONFIG_FOR_DOC)
@add_end_docstrings(FSMT_GENERATION_EXAMPLE)
def forward(
self,
input_ids: torch.LongTensor,
attention_mask: Optional[torch.Tensor] = None,
decoder_input_ids: Optional[torch.LongTensor] = None,
decoder_attention_mask: Optional[torch.BoolTensor] = None,
head_mask: Optional[torch.Tensor] = None,
decoder_head_mask: Optional[torch.Tensor] = None,
cross_attn_head_mask: Optional[torch.Tensor] = None,
encoder_outputs: Optional[Tuple[torch.FloatTensor]] = None,
past_key_values: Optional[Tuple[torch.FloatTensor]] = None,
inputs_embeds: Optional[torch.Tensor] = None,
decoder_inputs_embeds: Optional[torch.Tensor] = None,
labels: Optional[torch.LongTensor] = None,
use_cache: Optional[bool] = None,
output_attentions: Optional[bool] = None,
output_hidden_states: Optional[bool] = None,
return_dict: Optional[bool] = None,
) -> Union[Tuple[torch.Tensor], Seq2SeqLMOutput]:
r"""
labels (`torch.LongTensor` of shape `(batch_size, sequence_length)`, *optional*):
Labels for computing the masked language modeling loss. Indices should either be in `[0, ...,
config.vocab_size]` or -100 (see `input_ids` docstring). Tokens with indices set to `-100` are ignored
(masked), the loss is only computed for the tokens with labels in `[0, ..., config.vocab_size]`.
Returns:
"""
return_dict = return_dict if return_dict is not None else self.config.use_return_dict
if labels is not None:
use_cache = False
outputs = self.model(
input_ids,
inputs_embeds=inputs_embeds,
attention_mask=attention_mask,
decoder_input_ids=decoder_input_ids,
decoder_inputs_embeds=decoder_inputs_embeds,
encoder_outputs=encoder_outputs,
decoder_attention_mask=decoder_attention_mask,
head_mask=head_mask,
decoder_head_mask=decoder_head_mask,
cross_attn_head_mask=cross_attn_head_mask,
past_key_values=past_key_values,
use_cache=use_cache,
output_attentions=output_attentions,
output_hidden_states=output_hidden_states,
return_dict=return_dict,
)
lm_logits = outputs[0]
masked_lm_loss = None
if labels is not None:
loss_fct = CrossEntropyLoss()
# TODO(SS): do we need to ignore pad tokens in labels?
masked_lm_loss = loss_fct(lm_logits.view(-1, self.config.tgt_vocab_size), labels.view(-1))
if not return_dict:
output = (lm_logits,) + outputs[1:]
return ((masked_lm_loss,) + output) if masked_lm_loss is not None else output
return Seq2SeqLMOutput(
loss=masked_lm_loss,
logits=lm_logits,
past_key_values=outputs.past_key_values,
decoder_hidden_states=outputs.decoder_hidden_states,
decoder_attentions=outputs.decoder_attentions,
cross_attentions=outputs.cross_attentions,
encoder_last_hidden_state=outputs.encoder_last_hidden_state,
encoder_hidden_states=outputs.encoder_hidden_states,
encoder_attentions=outputs.encoder_attentions,
)
def prepare_inputs_for_generation(
self,
decoder_input_ids,
past_key_values=None,
attention_mask=None,
head_mask=None,
decoder_head_mask=None,
cross_attn_head_mask=None,
use_cache=None,
encoder_outputs=None,
**kwargs,
):
return {
"input_ids": None, # encoder_outputs is defined. input_ids not needed
"encoder_outputs": encoder_outputs,
"past_key_values": past_key_values,
"decoder_input_ids": decoder_input_ids,
"attention_mask": attention_mask,
"head_mask": head_mask,
"decoder_head_mask": decoder_head_mask,
"cross_attn_head_mask": cross_attn_head_mask,
"use_cache": use_cache, # change this to avoid caching (presumably for debugging)
}
def prepare_decoder_input_ids_from_labels(self, labels: torch.Tensor):
return shift_tokens_right(labels, self.config.pad_token_id)
@staticmethod
def _reorder_cache(past_key_values, beam_idx):
reordered_past = []
for layer_past in past_key_values:
# get the correct batch idx from decoder layer's batch dim for cross and self-attn
layer_past_new = {
attn_key: _reorder_buffer(attn_cache, beam_idx) for attn_key, attn_cache in layer_past.items()
}
reordered_past.append(layer_past_new)
return reordered_past
def get_encoder(self):
return self.model.encoder
def get_decoder(self):
return self.model.decoder
def get_output_embeddings(self):
return self.model.decoder.embed_tokens
def set_output_embeddings(self, value):
self.model.decoder.embed_tokens = value
class SinusoidalPositionalEmbedding(nn.Embedding):
"""
This module produces sinusoidal positional embeddings of any length.
We don't want to save the weight of this embedding since it's not trained (deterministic) and it can be huge.
Padding symbols are ignored.
These embeddings get automatically extended in forward if more positions is needed.
"""
def __init__(self, num_positions, embedding_dim, padding_idx):
self.make_weight(num_positions, embedding_dim, padding_idx)
def make_weight(self, num_positions, embedding_dim, padding_idx):
weight = self.get_embedding(num_positions, embedding_dim, padding_idx)
if not hasattr(self, "weight"):
# in ___init__
super().__init__(num_positions, embedding_dim, padding_idx, _weight=weight)
else:
# in forward put the weights on the correct dtype and device of the param
weight = weight.to(dtype=self.weight.dtype, device=self.weight.device)
self.weight = nn.Parameter(weight)
self.weight.detach_()
self.weight.requires_grad = False
@staticmethod
def get_embedding(num_embeddings, embedding_dim, padding_idx):
"""
Build sinusoidal embeddings.
This matches the implementation in tensor2tensor, but differs slightly from the description in Section 3.5 of
"Attention Is All You Need".
"""
half_dim = embedding_dim // 2
emb = math.log(10000) / (half_dim - 1)
emb = torch.exp(torch.arange(half_dim, dtype=torch.int64).float() * -emb)
emb = torch.arange(num_embeddings, dtype=torch.int64).float().unsqueeze(1) * emb.unsqueeze(0)
emb = torch.cat([torch.sin(emb), torch.cos(emb)], dim=1).view(num_embeddings, -1)
if embedding_dim % 2 == 1:
# zero pad
emb = torch.cat([emb, torch.zeros(num_embeddings, 1)], dim=1)
if padding_idx is not None:
emb[padding_idx, :] = 0
return emb
@staticmethod
def make_positions(tensor, padding_idx: int):
"""
Replace non-padding symbols with their position numbers.
Position numbers begin at padding_idx+1. Padding symbols are ignored.
"""
# The series of casts and type-conversions here are carefully
# balanced to both work with ONNX export and XLA. In particular XLA
# prefers ints, cumsum defaults to output longs, and ONNX doesn't know
# how to handle the dtype kwarg in cumsum.
mask = tensor.ne(padding_idx).int()
return (torch.cumsum(mask, dim=1).type_as(mask) * mask).long() + padding_idx
def forward(
self,
input,
incremental_state: Optional[Any] = None,
timestep: Optional[Tensor] = None,
):
"""Input is expected to be of size [bsz x seqlen]."""
bsz, seq_len = input.shape[:2]
max_pos = self.padding_idx + 1 + seq_len
if max_pos > self.weight.size(0):
# expand embeddings if needed
self.make_weight(max_pos, self.embedding_dim, self.padding_idx)
positions = self.make_positions(input, self.padding_idx)
return super().forward(positions)
| transformers/src/transformers/models/fsmt/modeling_fsmt.py/0 | {
"file_path": "transformers/src/transformers/models/fsmt/modeling_fsmt.py",
"repo_id": "transformers",
"token_count": 25668
} | 301 |
# coding=utf-8
# Copyright 2022 The HuggingFace Inc. team. All rights reserved.
#
# Licensed under the Apache License, Version 2.0 (the "License");
# you may not use this file except in compliance with the License.
# You may obtain a copy of the License at
#
# http://www.apache.org/licenses/LICENSE-2.0
#
# Unless required by applicable law or agreed to in writing, software
# distributed under the License is distributed on an "AS IS" BASIS,
# WITHOUT WARRANTIES OR CONDITIONS OF ANY KIND, either express or implied.
# See the License for the specific language governing permissions and
# limitations under the License.
import os
from typing import Union
from ...configuration_utils import PretrainedConfig
from ...utils import logging
logger = logging.get_logger(__name__)
GIT_PRETRAINED_CONFIG_ARCHIVE_MAP = {
"microsoft/git-base": "https://huggingface.co/microsoft/git-base/resolve/main/config.json",
}
class GitVisionConfig(PretrainedConfig):
r"""
This is the configuration class to store the configuration of a [`GitVisionModel`]. It is used to instantiate a GIT
vision encoder according to the specified arguments, defining the model architecture. Instantiating a configuration
with the defaults will yield a similar configuration to that of the vision encoder of the GIT
[microsoft/git-base](https://huggingface.co/microsoft/git-base) architecture.
Configuration objects inherit from [`PretrainedConfig`] and can be used to control the model outputs. Read the
documentation from [`PretrainedConfig`] for more information.
Args:
hidden_size (`int`, *optional*, defaults to 768):
Dimensionality of the encoder layers and the pooler layer.
intermediate_size (`int`, *optional*, defaults to 3072):
Dimensionality of the "intermediate" (i.e., feed-forward) layer in the Transformer encoder.
num_hidden_layers (`int`, *optional*, defaults to 12):
Number of hidden layers in the Transformer encoder.
num_attention_heads (`int`, *optional*, defaults to 12):
Number of attention heads for each attention layer in the Transformer encoder.
image_size (`int`, *optional*, defaults to 224):
The size (resolution) of each image.
patch_size (`int`, *optional*, defaults to 16):
The size (resolution) of each patch.
hidden_act (`str` or `function`, *optional*, defaults to `"quick_gelu"`):
The non-linear activation function (function or string) in the encoder and pooler. If string, `"gelu"`,
`"relu"`, `"selu"` and `"gelu_new"` ``"quick_gelu"` are supported.
layer_norm_eps (`float`, *optional*, defaults to 1e-5):
The epsilon used by the layer normalization layers.
attention_dropout (`float`, *optional*, defaults to 0.0):
The dropout ratio for the attention probabilities.
initializer_range (`float`, *optional*, defaults to 0.02):
The standard deviation of the truncated_normal_initializer for initializing all weight matrices.
Example:
```python
>>> from transformers import GitVisionConfig, GitVisionModel
>>> # Initializing a GitVisionConfig with microsoft/git-base style configuration
>>> configuration = GitVisionConfig()
>>> # Initializing a GitVisionModel (with random weights) from the microsoft/git-base style configuration
>>> model = GitVisionModel(configuration)
>>> # Accessing the model configuration
>>> configuration = model.config
```"""
model_type = "git_vision_model"
def __init__(
self,
hidden_size=768,
intermediate_size=3072,
num_hidden_layers=12,
num_attention_heads=12,
num_channels=3,
image_size=224,
patch_size=16,
hidden_act="quick_gelu",
layer_norm_eps=1e-5,
attention_dropout=0.0,
initializer_range=0.02,
**kwargs,
):
super().__init__(**kwargs)
self.hidden_size = hidden_size
self.intermediate_size = intermediate_size
self.num_hidden_layers = num_hidden_layers
self.num_attention_heads = num_attention_heads
self.num_channels = num_channels
self.patch_size = patch_size
self.image_size = image_size
self.initializer_range = initializer_range
self.attention_dropout = attention_dropout
self.layer_norm_eps = layer_norm_eps
self.hidden_act = hidden_act
@classmethod
def from_pretrained(cls, pretrained_model_name_or_path: Union[str, os.PathLike], **kwargs) -> "PretrainedConfig":
cls._set_token_in_kwargs(kwargs)
config_dict, kwargs = cls.get_config_dict(pretrained_model_name_or_path, **kwargs)
# get the vision config dict if we are loading from GITConfig
if config_dict.get("model_type") == "git":
config_dict = config_dict["vision_config"]
if "model_type" in config_dict and hasattr(cls, "model_type") and config_dict["model_type"] != cls.model_type:
logger.warning(
f"You are using a model of type {config_dict['model_type']} to instantiate a model of type "
f"{cls.model_type}. This is not supported for all configurations of models and can yield errors."
)
return cls.from_dict(config_dict, **kwargs)
class GitConfig(PretrainedConfig):
r"""
This is the configuration class to store the configuration of a [`GitModel`]. It is used to instantiate a GIT model
according to the specified arguments, defining the model architecture. Instantiating a configuration with the
defaults will yield a similar configuration to that of the GIT
[microsoft/git-base](https://huggingface.co/microsoft/git-base) architecture.
Configuration objects inherit from [`PretrainedConfig`] and can be used to control the model outputs. Read the
documentation from [`PretrainedConfig`] for more information.
Args:
vision_config (`dict`, *optional*):
Dictionary of configuration options used to initialize [`GitVisionConfig`].
vocab_size (`int`, *optional*, defaults to 30522):
Vocabulary size of the GIT model. Defines the number of different tokens that can be represented by the
`inputs_ids` passed when calling [`GitModel`].
hidden_size (`int`, *optional*, defaults to 768):
Dimensionality of the encoder layers and the pooler layer.
num_hidden_layers (`int`, *optional*, defaults to 6):
Number of hidden layers in the Transformer encoder.
num_attention_heads (`int`, *optional*, defaults to 12):
Number of attention heads for each attention layer in the Transformer encoder.
intermediate_size (`int`, *optional*, defaults to 3072):
Dimensionality of the "intermediate" (often named feed-forward) layer in the Transformer encoder.
hidden_act (`str` or `Callable`, *optional*, defaults to `"gelu"`):
The non-linear activation function (function or string) in the encoder and pooler. If string, `"gelu"`,
`"relu"`, `"silu"` and `"gelu_new"` are supported.
hidden_dropout_prob (`float`, *optional*, defaults to 0.1):
The dropout probability for all fully connected layers in the embeddings, encoder, and pooler.
attention_probs_dropout_prob (`float`, *optional*, defaults to 0.1):
The dropout ratio for the attention probabilities.
max_position_embeddings (`int`, *optional*, defaults to 1024):
The maximum sequence length that this model might ever be used with. Typically set this to something large
just in case (e.g., 512 or 1024 or 2048).
initializer_range (`float`, *optional*, defaults to 0.02):
The standard deviation of the truncated_normal_initializer for initializing all weight matrices.
layer_norm_eps (`float`, *optional*, defaults to 1e-12):
The epsilon used by the layer normalization layers.
position_embedding_type (`str`, *optional*, defaults to `"absolute"`):
Type of position embedding. Choose one of `"absolute"`, `"relative_key"`, `"relative_key_query"`. For
positional embeddings use `"absolute"`. For more information on `"relative_key"`, please refer to
[Self-Attention with Relative Position Representations (Shaw et al.)](https://arxiv.org/abs/1803.02155).
For more information on `"relative_key_query"`, please refer to *Method 4* in [Improve Transformer Models
with Better Relative Position Embeddings (Huang et al.)](https://arxiv.org/abs/2009.13658).
use_cache (`bool`, *optional*, defaults to `True`):
Whether or not the model should return the last key/values attentions (not used by all models).
num_image_with_embedding (`int`, *optional*):
The number of temporal embeddings to add, in case the model is used for video captioning/VQA.
Examples:
```python
>>> from transformers import GitConfig, GitModel
>>> # Initializing a GIT microsoft/git-base style configuration
>>> configuration = GitConfig()
>>> # Initializing a model (with random weights) from the microsoft/git-base style configuration
>>> model = GitModel(configuration)
>>> # Accessing the model configuration
>>> configuration = model.config
```"""
model_type = "git"
def __init__(
self,
vision_config=None,
vocab_size=30522,
hidden_size=768,
num_hidden_layers=6,
num_attention_heads=12,
intermediate_size=3072,
hidden_act="gelu",
hidden_dropout_prob=0.1,
attention_probs_dropout_prob=0.1,
max_position_embeddings=1024,
initializer_range=0.02,
layer_norm_eps=1e-12,
pad_token_id=0,
position_embedding_type="absolute",
use_cache=True,
tie_word_embeddings=False,
bos_token_id=101,
eos_token_id=102,
num_image_with_embedding=None,
**kwargs,
):
super().__init__(bos_token_id=bos_token_id, eos_token_id=eos_token_id, pad_token_id=pad_token_id, **kwargs)
if vision_config is None:
vision_config = {}
logger.info("vision_config is None. initializing the GitVisionConfig with default values.")
self.vision_config = GitVisionConfig(**vision_config)
self.vocab_size = vocab_size
self.hidden_size = hidden_size
self.num_hidden_layers = num_hidden_layers
self.num_attention_heads = num_attention_heads
self.hidden_act = hidden_act
self.intermediate_size = intermediate_size
self.hidden_dropout_prob = hidden_dropout_prob
self.attention_probs_dropout_prob = attention_probs_dropout_prob
self.max_position_embeddings = max_position_embeddings
self.initializer_range = initializer_range
self.layer_norm_eps = layer_norm_eps
self.position_embedding_type = position_embedding_type
self.use_cache = use_cache
self.tie_word_embeddings = tie_word_embeddings
self.num_image_with_embedding = num_image_with_embedding
self.bos_token_id = bos_token_id
self.eos_token_id = eos_token_id
| transformers/src/transformers/models/git/configuration_git.py/0 | {
"file_path": "transformers/src/transformers/models/git/configuration_git.py",
"repo_id": "transformers",
"token_count": 4236
} | 302 |
# coding=utf-8
# Copyright 2018 The OpenAI Team Authors and HuggingFace Inc. team.
# Copyright (c) 2018, NVIDIA CORPORATION. All rights reserved.
#
# Licensed under the Apache License, Version 2.0 (the "License");
# you may not use this file except in compliance with the License.
# You may obtain a copy of the License at
#
# http://www.apache.org/licenses/LICENSE-2.0
#
# Unless required by applicable law or agreed to in writing, software
# distributed under the License is distributed on an "AS IS" BASIS,
# WITHOUT WARRANTIES OR CONDITIONS OF ANY KIND, either express or implied.
# See the License for the specific language governing permissions and
# limitations under the License.
""" TF 2.0 OpenAI GPT-2 model."""
from __future__ import annotations
from dataclasses import dataclass
from typing import List, Optional, Tuple, Union
import numpy as np
import tensorflow as tf
from ...activations_tf import get_tf_activation
from ...modeling_tf_outputs import (
TFBaseModelOutputWithPastAndCrossAttentions,
TFCausalLMOutputWithCrossAttentions,
TFSequenceClassifierOutputWithPast,
)
from ...modeling_tf_utils import (
TFCausalLanguageModelingLoss,
TFConv1D,
TFModelInputType,
TFPreTrainedModel,
TFSequenceClassificationLoss,
TFSequenceSummary,
get_initializer,
keras,
keras_serializable,
unpack_inputs,
)
from ...tf_utils import check_embeddings_within_bounds, shape_list, stable_softmax
from ...utils import (
ModelOutput,
add_code_sample_docstrings,
add_start_docstrings,
add_start_docstrings_to_model_forward,
logging,
replace_return_docstrings,
)
from .configuration_gpt2 import GPT2Config
logger = logging.get_logger(__name__)
_CHECKPOINT_FOR_DOC = "gpt2"
_CONFIG_FOR_DOC = "GPT2Config"
TF_GPT2_PRETRAINED_MODEL_ARCHIVE_LIST = [
"gpt2",
"gpt2-medium",
"gpt2-large",
"gpt2-xl",
"distilgpt2",
# See all GPT-2 models at https://huggingface.co/models?filter=gpt2
]
class TFAttention(keras.layers.Layer):
def __init__(self, nx, config, scale=False, is_cross_attention=False, **kwargs):
super().__init__(**kwargs)
n_state = nx # in Attention: n_state=768 (nx=n_embd)
# [switch nx => n_state from Block to Attention to keep identical to TF implementation]
assert n_state % config.n_head == 0
self.n_head = config.n_head
self.split_size = n_state
self.scale = scale
self.output_attentions = config.output_attentions
self.is_cross_attention = is_cross_attention
if self.is_cross_attention:
self.c_attn = TFConv1D(n_state * 2, nx, initializer_range=config.initializer_range, name="c_attn")
self.q_attn = TFConv1D(n_state, nx, initializer_range=config.initializer_range, name="q_attn")
else:
self.c_attn = TFConv1D(n_state * 3, nx, initializer_range=config.initializer_range, name="c_attn")
self.c_proj = TFConv1D(n_state, nx, initializer_range=config.initializer_range, name="c_proj")
self.attn_dropout = keras.layers.Dropout(config.attn_pdrop)
self.resid_dropout = keras.layers.Dropout(config.resid_pdrop)
self.pruned_heads = set()
self.embed_dim = n_state
def prune_heads(self, heads):
pass
@staticmethod
def causal_attention_mask(nd, ns, dtype):
"""
1's in the lower triangle, counting from the lower right corner. Same as tf.matrix_band_part(tf.ones([nd, ns]),
-1, ns-nd), but doesn't produce garbage on TPUs.
"""
i = tf.range(nd)[:, None]
j = tf.range(ns)
m = i >= j - ns + nd
return tf.cast(m, dtype)
def _attn(self, q, k, v, attention_mask, head_mask, output_attentions, training=False):
# q, k, v have shape [batch, heads, sequence, features]
w = tf.matmul(q, k, transpose_b=True)
if self.scale:
dk = tf.cast(shape_list(k)[-1], dtype=w.dtype) # scale attention_scores
w = w / tf.math.sqrt(dk)
if not self.is_cross_attention:
# if only "normal" attention layer implements causal mask
# w has shape [batch, heads, dst_sequence, src_sequence], where information flows from src to dst.
_, _, nd, ns = shape_list(w)
b = self.causal_attention_mask(nd, ns, dtype=w.dtype)
b = tf.reshape(b, [1, 1, nd, ns])
w = w * b - 1e4 * (1 - b)
if attention_mask is not None:
# Apply the attention mask
attention_mask = tf.cast(attention_mask, dtype=w.dtype)
w = w + attention_mask
w = stable_softmax(w, axis=-1)
w = self.attn_dropout(w, training=training)
# Mask heads if we want to
if head_mask is not None:
w = w * head_mask
outputs = [tf.matmul(w, v)]
if output_attentions:
outputs.append(w)
return outputs
def merge_heads(self, x):
x = tf.transpose(x, [0, 2, 1, 3])
x_shape = shape_list(x)
new_x_shape = x_shape[:-2] + [x_shape[-2] * x_shape[-1]]
return tf.reshape(x, new_x_shape)
def split_heads(self, x):
x_shape = shape_list(x)
new_x_shape = x_shape[:-1] + [self.n_head, x_shape[-1] // self.n_head]
x = tf.reshape(x, new_x_shape)
return tf.transpose(x, (0, 2, 1, 3)) # (batch, head, seq_length, head_features)
def call(
self,
x,
layer_past,
attention_mask,
head_mask,
encoder_hidden_states,
encoder_attention_mask,
use_cache,
output_attentions,
training=False,
):
if encoder_hidden_states is not None:
if not hasattr(self, "q_attn"):
raise ValueError(
"If class is used as cross attention, the weights `q_attn` have to be defined. "
"Please make sure to instantiate class with `GPT2Attention(..., is_cross_attention=True)`."
)
query = self.q_attn(x)
kv_out = self.c_attn(encoder_hidden_states)
key, value = tf.split(kv_out, 2, axis=2)
attention_mask = encoder_attention_mask
else:
x = self.c_attn(x)
query, key, value = tf.split(x, 3, axis=2)
query = self.split_heads(query)
key = self.split_heads(key)
value = self.split_heads(value)
if layer_past is not None:
past_key, past_value = tf.unstack(layer_past, axis=0, num=2)
key = tf.concat([past_key, key], axis=-2)
value = tf.concat([past_value, value], axis=-2)
# to cope with keras serialization
if use_cache:
present = tf.stack([key, value], axis=0)
else:
present = (None,)
attn_outputs = self._attn(query, key, value, attention_mask, head_mask, output_attentions, training=training)
a = attn_outputs[0]
a = self.merge_heads(a)
a = self.c_proj(a)
a = self.resid_dropout(a, training=training)
outputs = [a, present] + attn_outputs[1:]
return outputs # a, present, (attentions)
def build(self, input_shape=None):
if self.built:
return
self.built = True
if self.is_cross_attention:
c_attn_shape = 2 * self.embed_dim
else:
c_attn_shape = 3 * self.embed_dim
if getattr(self, "c_proj", None) is not None:
with tf.name_scope(self.c_proj.name):
self.c_proj.build([None, None, self.embed_dim])
if getattr(self, "c_attn", None) is not None:
with tf.name_scope(self.c_attn.name):
self.c_attn.build([None, None, c_attn_shape])
if getattr(self, "q_attn", None) is not None:
with tf.name_scope(self.q_attn.name):
self.q_attn.build([None, None, self.embed_dim])
class TFMLP(keras.layers.Layer):
def __init__(self, n_state, config, **kwargs):
super().__init__(**kwargs)
nx = config.n_embd
self.c_fc = TFConv1D(n_state, nx, initializer_range=config.initializer_range, name="c_fc")
self.c_proj = TFConv1D(nx, n_state, initializer_range=config.initializer_range, name="c_proj")
self.act = get_tf_activation(config.activation_function)
self.dropout = keras.layers.Dropout(config.resid_pdrop)
self.intermediate_size = n_state
self.embed_dim = nx
def call(self, x, training=False):
h = self.act(self.c_fc(x))
h2 = self.c_proj(h)
h2 = self.dropout(h2, training=training)
return h2
def build(self, input_shape=None):
if self.built:
return
self.built = True
if getattr(self, "c_fc", None) is not None:
with tf.name_scope(self.c_fc.name):
self.c_fc.build([None, None, self.intermediate_size])
if getattr(self, "c_proj", None) is not None:
with tf.name_scope(self.c_proj.name):
self.c_proj.build([None, None, self.embed_dim])
class TFBlock(keras.layers.Layer):
def __init__(self, config, scale=False, **kwargs):
super().__init__(**kwargs)
nx = config.n_embd
inner_dim = config.n_inner if config.n_inner is not None else 4 * nx
self.ln_1 = keras.layers.LayerNormalization(epsilon=config.layer_norm_epsilon, name="ln_1")
self.attn = TFAttention(nx, config, scale, name="attn")
self.ln_2 = keras.layers.LayerNormalization(epsilon=config.layer_norm_epsilon, name="ln_2")
if config.add_cross_attention:
self.crossattention = TFAttention(nx, config, scale, name="crossattention", is_cross_attention=True)
self.ln_cross_attn = keras.layers.LayerNormalization(
epsilon=config.layer_norm_epsilon, name="ln_cross_attn"
)
self.mlp = TFMLP(inner_dim, config, name="mlp")
self.hidden_size = config.hidden_size
def call(
self,
x,
layer_past,
attention_mask,
head_mask,
encoder_hidden_states,
encoder_attention_mask,
use_cache,
output_attentions,
training=False,
):
a = self.ln_1(x)
output_attn = self.attn(
a,
layer_past=layer_past,
attention_mask=attention_mask,
head_mask=head_mask,
encoder_hidden_states=None,
encoder_attention_mask=None,
use_cache=use_cache,
output_attentions=output_attentions,
training=training,
)
a = output_attn[0] # output_attn: a, present, (attentions)
outputs = output_attn[1:]
x = x + a
# Cross-Attention Block
if encoder_hidden_states is not None:
# add one self-attention block for cross-attention
if not hasattr(self, "crossattention"):
raise ValueError(
f"If `encoder_hidden_states` are passed, {self} has to be instantiated with "
"cross-attention layers by setting `config.add_cross_attention=True`"
)
ca = self.ln_cross_attn(x)
output_cross_attn = self.crossattention(
ca,
layer_past=None,
attention_mask=attention_mask,
head_mask=head_mask,
encoder_hidden_states=encoder_hidden_states,
encoder_attention_mask=encoder_attention_mask,
use_cache=False,
output_attentions=output_attentions,
training=training,
)
ca = output_cross_attn[0] # output_attn: a, present, (cross_attentions)
x = x + ca
outputs = outputs + output_cross_attn[2:] # add cross attentions if we output attention weights
m = self.ln_2(x)
m = self.mlp(m, training=training)
x = x + m
outputs = [x] + outputs
return outputs # x, present, (attentions, cross_attentions)
def build(self, input_shape=None):
if self.built:
return
self.built = True
if getattr(self, "ln_1", None) is not None:
with tf.name_scope(self.ln_1.name):
self.ln_1.build([None, None, self.hidden_size])
if getattr(self, "attn", None) is not None:
with tf.name_scope(self.attn.name):
self.attn.build(None)
if getattr(self, "ln_2", None) is not None:
with tf.name_scope(self.ln_2.name):
self.ln_2.build([None, None, self.hidden_size])
if getattr(self, "mlp", None) is not None:
with tf.name_scope(self.mlp.name):
self.mlp.build(None)
if getattr(self, "crossattention", None) is not None:
with tf.name_scope(self.crossattention.name):
self.crossattention.build(None)
if getattr(self, "ln_cross_attn", None) is not None:
with tf.name_scope(self.ln_cross_attn.name):
self.ln_cross_attn.build([None, None, self.hidden_size])
@keras_serializable
class TFGPT2MainLayer(keras.layers.Layer):
config_class = GPT2Config
def __init__(self, config, *inputs, **kwargs):
super().__init__(*inputs, **kwargs)
self.config = config
self.output_attentions = config.output_attentions
self.output_hidden_states = config.output_hidden_states
self.use_cache = config.use_cache
self.return_dict = config.use_return_dict
self.num_hidden_layers = config.n_layer
self.n_embd = config.n_embd
self.n_positions = config.n_positions
self.initializer_range = config.initializer_range
self.wte = keras.layers.Embedding(
input_dim=config.vocab_size,
output_dim=config.hidden_size,
embeddings_initializer=get_initializer(config.initializer_range),
name="wte",
)
self.wpe = keras.layers.Embedding(
input_dim=config.n_positions,
output_dim=config.n_embd,
embeddings_initializer=get_initializer(config.initializer_range),
name="wpe",
)
self.drop = keras.layers.Dropout(config.embd_pdrop)
self.h = [TFBlock(config, scale=True, name=f"h_._{i}") for i in range(config.n_layer)]
self.ln_f = keras.layers.LayerNormalization(epsilon=config.layer_norm_epsilon, name="ln_f")
self.embed_dim = config.hidden_size
def get_input_embeddings(self):
return self.wte
def set_input_embeddings(self, new_embeddings):
self.wte = new_embeddings
def _prune_heads(self, heads_to_prune):
"""
Prunes heads of the model. heads_to_prune: dict of {layer_num: list of heads to prune in this layer}
"""
raise NotImplementedError
@unpack_inputs
def call(
self,
input_ids: TFModelInputType | None = None,
past_key_values: Optional[Tuple[Tuple[Union[np.ndarray, tf.Tensor]]]] = None,
attention_mask: np.ndarray | tf.Tensor | None = None,
token_type_ids: np.ndarray | tf.Tensor | None = None,
position_ids: np.ndarray | tf.Tensor | None = None,
head_mask: np.ndarray | tf.Tensor | None = None,
inputs_embeds: np.ndarray | tf.Tensor | None = None,
encoder_hidden_states: np.ndarray | tf.Tensor | None = None,
encoder_attention_mask: np.ndarray | tf.Tensor | None = None,
use_cache: Optional[bool] = None,
output_attentions: Optional[bool] = None,
output_hidden_states: Optional[bool] = None,
return_dict: Optional[bool] = None,
training: Optional[bool] = False,
) -> Union[TFBaseModelOutputWithPastAndCrossAttentions, Tuple[tf.Tensor]]:
if input_ids is not None and inputs_embeds is not None:
raise ValueError("You cannot specify both input_ids and inputs_embeds at the same time")
elif input_ids is not None:
input_shape = shape_list(input_ids)
input_ids = tf.reshape(input_ids, [-1, input_shape[-1]])
elif inputs_embeds is not None:
input_shape = shape_list(inputs_embeds)[:-1]
else:
raise ValueError("You have to specify either input_ids or inputs_embeds")
if past_key_values is None:
past_length = 0
past_key_values = [None] * len(self.h)
else:
past_length = shape_list(past_key_values[0][0])[-2]
if position_ids is None:
position_ids = tf.expand_dims(tf.range(past_length, input_shape[-1] + past_length), axis=0)
if attention_mask is not None:
# We create a 3D attention mask from a 2D tensor mask.
# Sizes are [batch_size, 1, 1, to_seq_length]
# So we can broadcast to [batch_size, num_heads, from_seq_length, to_seq_length]
# this attention mask is more simple than the triangular masking of causal attention
# used in OpenAI GPT, we just need to prepare the broadcast dimension here.
attention_mask_shape = shape_list(attention_mask)
attention_mask = tf.reshape(attention_mask, (attention_mask_shape[0], 1, 1, attention_mask_shape[1]))
# Since attention_mask is 1.0 for positions we want to attend and 0.0 for
# masked positions, this operation will create a tensor which is 0.0 for
# positions we want to attend and -10000.0 for masked positions.
# Since we are adding it to the raw scores before the softmax, this is
# effectively the same as removing these entirely.
one_cst = tf.constant(1.0)
attention_mask = tf.cast(attention_mask, dtype=one_cst.dtype)
attention_mask = tf.multiply(tf.subtract(one_cst, attention_mask), tf.constant(-10000.0))
# Copied from `modeling_tf_t5.py` with -1e9 -> -10000
if self.config.add_cross_attention and encoder_attention_mask is not None:
# If a 2D ou 3D attention mask is provided for the cross-attention
# we need to make broadcastable to [batch_size, num_heads, mask_seq_length, mask_seq_length]
# we need to make broadcastable to [batch_size, num_heads, seq_length, seq_length]
encoder_attention_mask = tf.cast(encoder_attention_mask, dtype=encoder_hidden_states.dtype)
num_dims_encoder_attention_mask = len(shape_list(encoder_attention_mask))
if num_dims_encoder_attention_mask == 3:
encoder_extended_attention_mask = encoder_attention_mask[:, None, :, :]
if num_dims_encoder_attention_mask == 2:
encoder_extended_attention_mask = encoder_attention_mask[:, None, None, :]
# T5 has a mask that can compare sequence ids, we can simulate this here with this transposition
# Cf. https://github.com/tensorflow/mesh/blob/8d2465e9bc93129b913b5ccc6a59aa97abd96ec6/mesh_tensorflow/transformer/transformer_layers.py#L270
# encoder_extended_attention_mask = tf.math.equal(encoder_extended_attention_mask,
# tf.transpose(encoder_extended_attention_mask, perm=(-1, -2)))
encoder_extended_attention_mask = (1.0 - encoder_extended_attention_mask) * -10000.0
else:
encoder_extended_attention_mask = None
encoder_attention_mask = encoder_extended_attention_mask
# Prepare head mask if needed
# 1.0 in head_mask indicate we keep the head
# attention_probs has shape bsz x n_heads x N x N
# input head_mask has shape [num_heads] or [num_hidden_layers x num_heads]
# and head_mask is converted to shape [num_hidden_layers x batch x num_heads x seq_length x seq_length]
if head_mask is not None:
raise NotImplementedError
else:
head_mask = [None] * self.num_hidden_layers
# head_mask = tf.constant([0] * self.num_hidden_layers)
position_ids = tf.reshape(position_ids, [-1, shape_list(position_ids)[-1]])
if inputs_embeds is None:
check_embeddings_within_bounds(input_ids, self.config.vocab_size)
inputs_embeds = self.wte(input_ids)
position_embeds = self.wpe(position_ids)
if token_type_ids is not None:
token_type_ids = tf.reshape(token_type_ids, [-1, shape_list(token_type_ids)[-1]])
token_type_embeds = self.wte(token_type_ids)
else:
token_type_embeds = tf.constant(0.0)
position_embeds = tf.cast(position_embeds, dtype=inputs_embeds.dtype)
token_type_embeds = tf.cast(token_type_embeds, dtype=inputs_embeds.dtype)
hidden_states = inputs_embeds + position_embeds + token_type_embeds
hidden_states = self.drop(hidden_states, training=training)
output_shape = input_shape + [shape_list(hidden_states)[-1]]
presents = () if use_cache else None
all_attentions = () if output_attentions else None
all_cross_attentions = () if output_attentions and self.config.add_cross_attention else None
all_hidden_states = () if output_hidden_states else None
for i, (block, layer_past) in enumerate(zip(self.h, past_key_values)):
if output_hidden_states:
all_hidden_states = all_hidden_states + (tf.reshape(hidden_states, output_shape),)
outputs = block(
hidden_states,
layer_past,
attention_mask,
head_mask[i],
encoder_hidden_states,
encoder_attention_mask,
use_cache,
output_attentions,
training=training,
)
hidden_states, present = outputs[:2]
if use_cache:
presents = presents + (present,)
if output_attentions:
all_attentions = all_attentions + (outputs[2],)
if self.config.add_cross_attention and encoder_hidden_states is not None:
all_cross_attentions = all_cross_attentions + (outputs[3],)
hidden_states = self.ln_f(hidden_states)
hidden_states = tf.reshape(hidden_states, output_shape)
# Add last hidden state
if output_hidden_states:
all_hidden_states = all_hidden_states + (hidden_states,)
if output_attentions:
# let the number of heads free (-1) so we can extract attention even after head pruning
attention_output_shape = input_shape[:-1] + [-1] + shape_list(all_attentions[0])[-2:]
all_attentions = tuple(tf.reshape(t, attention_output_shape) for t in all_attentions)
if not return_dict:
return tuple(
v
for v in [hidden_states, presents, all_hidden_states, all_attentions, all_cross_attentions]
if v is not None
)
return TFBaseModelOutputWithPastAndCrossAttentions(
last_hidden_state=hidden_states,
past_key_values=presents,
hidden_states=all_hidden_states,
attentions=all_attentions,
cross_attentions=all_cross_attentions,
)
def build(self, input_shape=None):
if self.built:
return
self.built = True
if getattr(self, "wte", None) is not None:
with tf.name_scope(self.wte.name):
self.wte.build(None)
if getattr(self, "wpe", None) is not None:
with tf.name_scope(self.wpe.name):
self.wpe.build(None)
if getattr(self, "ln_f", None) is not None:
with tf.name_scope(self.ln_f.name):
self.ln_f.build([None, None, self.embed_dim])
if getattr(self, "h", None) is not None:
for layer in self.h:
with tf.name_scope(layer.name):
layer.build(None)
class TFGPT2PreTrainedModel(TFPreTrainedModel):
"""
An abstract class to handle weights initialization and a simple interface for downloading and loading pretrained
models.
"""
config_class = GPT2Config
base_model_prefix = "transformer"
# names with a '.' represents the authorized unexpected/missing layers when a TF model is loaded from a PT model
_keys_to_ignore_on_load_unexpected = [r"h.\d+.attn.bias", r"h.\d+.crossattention.bias"]
@property
def input_signature(self):
# Although GPT-2 supports token_type_ids in theory, in practice they are rarely used, and the implementation
# means that passing token_type_ids=0 yields different outputs from token_type_ids=None.
# Therefore, we remove the token_type_ids argument by default, even though it would usually be included.
return {
"input_ids": tf.TensorSpec((None, None), tf.int32, name="input_ids"),
"attention_mask": tf.TensorSpec((None, None), tf.int32, name="attention_mask"),
}
@dataclass
class TFGPT2DoubleHeadsModelOutput(ModelOutput):
"""
Base class for outputs of models predicting if two sentences are consecutive or not.
Args:
logits (`tf.Tensor` of shape `(batch_size, num_choices, sequence_length, config.vocab_size)`):
Prediction scores of the language modeling head (scores for each vocabulary token before SoftMax).
mc_logits (`tf.Tensor` of shape `(batch_size, num_choices)`):
Prediction scores of the multiple choice classification head (scores for each choice before SoftMax).
past_key_values (`List[tf.Tensor]`, *optional*, returned when `use_cache=True` is passed or when `config.use_cache=True`):
List of `tf.Tensor` of length `config.n_layers`, with each tensor of shape `(2, batch_size, num_heads,
sequence_length, embed_size_per_head)`).
Contains pre-computed hidden-states (key and values in the attention blocks) that can be used (see
`past_key_values` input) to speed up sequential decoding.
hidden_states (`tuple(tf.Tensor)`, *optional*, returned when `output_hidden_states=True` is passed or when `config.output_hidden_states=True`):
Tuple of `tf.Tensor` (one for the output of the embeddings + one for the output of each layer) of shape
`(batch_size, sequence_length, hidden_size)`.
Hidden-states of the model at the output of each layer plus the initial embedding outputs.
attentions (`tuple(tf.Tensor)`, *optional*, returned when `output_attentions=True` is passed or when `config.output_attentions=True`):
Tuple of `tf.Tensor` (one for each layer) of shape `(batch_size, num_heads, sequence_length,
sequence_length)`.
Attentions weights after the attention softmax, used to compute the weighted average in the self-attention
heads.
"""
logits: tf.Tensor = None
mc_logits: tf.Tensor = None
past_key_values: List[tf.Tensor] | None = None
hidden_states: Tuple[tf.Tensor] | None = None
attentions: Tuple[tf.Tensor] | None = None
GPT2_START_DOCSTRING = r"""
This model inherits from [`TFPreTrainedModel`]. Check the superclass documentation for the generic methods the
library implements for all its model (such as downloading or saving, resizing the input embeddings, pruning heads
etc.)
This model is also a [keras.Model](https://www.tensorflow.org/api_docs/python/tf/keras/Model) subclass. Use it
as a regular TF 2.0 Keras Model and refer to the TF 2.0 documentation for all matter related to general usage and
behavior.
<Tip>
TensorFlow models and layers in `transformers` accept two formats as input:
- having all inputs as keyword arguments (like PyTorch models), or
- having all inputs as a list, tuple or dict in the first positional argument.
The reason the second format is supported is that Keras methods prefer this format when passing inputs to models
and layers. Because of this support, when using methods like `model.fit()` things should "just work" for you - just
pass your inputs and labels in any format that `model.fit()` supports! If, however, you want to use the second
format outside of Keras methods like `fit()` and `predict()`, such as when creating your own layers or models with
the Keras `Functional` API, there are three possibilities you can use to gather all the input Tensors in the first
positional argument:
- a single Tensor with `input_ids` only and nothing else: `model(input_ids)`
- a list of varying length with one or several input Tensors IN THE ORDER given in the docstring:
`model([input_ids, attention_mask])` or `model([input_ids, attention_mask, token_type_ids])`
- a dictionary with one or several input Tensors associated to the input names given in the docstring:
`model({"input_ids": input_ids, "token_type_ids": token_type_ids})`
Note that when creating models and layers with
[subclassing](https://keras.io/guides/making_new_layers_and_models_via_subclassing/) then you don't need to worry
about any of this, as you can just pass inputs like you would to any other Python function!
</Tip>
Parameters:
config ([`GPT2Config`]): Model configuration class with all the parameters of the model.
Initializing with a config file does not load the weights associated with the model, only the
configuration. Check out the [`~PreTrainedModel.from_pretrained`] method to load the model weights.
"""
GPT2_INPUTS_DOCSTRING = r"""
Args:
input_ids (`Numpy array` or `tf.Tensor` of shape `(batch_size, input_ids_length)`):
`input_ids_length` = `sequence_length` if `past_key_values` is `None` else `past_key_values[0].shape[-2]`
(`sequence_length` of input past key value states). Indices of input sequence tokens in the vocabulary.
If `past_key_values` is used, only input IDs that do not have their past calculated should be passed as
`input_ids`.
Indices can be obtained using [`AutoTokenizer`]. See [`PreTrainedTokenizer.__call__`] and
[`PreTrainedTokenizer.encode`] for details.
[What are input IDs?](../glossary#input-ids)
past_key_values (`List[tf.Tensor]` of length `config.n_layers`):
Contains pre-computed hidden-states (key and values in the attention blocks) as computed by the model (see
`past_key_values` output below). Can be used to speed up sequential decoding. The token ids which have
their past given to this model should not be passed as input ids as they have already been computed.
attention_mask (`tf.Tensor` or `Numpy array` of shape `(batch_size, sequence_length)`, *optional*):
Mask to avoid performing attention on padding token indices. Mask values selected in `[0, 1]`:
- 1 for tokens that are **not masked**,
- 0 for tokens that are **masked**.
If `past_key_values` is used, `attention_mask` needs to contain the masking strategy that was used for
`past_key_values`. In other words, the `attention_mask` always has to have the length:
`len(past_key_values) + len(input_ids)`
[What are attention masks?](../glossary#attention-mask)
token_type_ids (`tf.Tensor` or `Numpy array` of shape `(batch_size, sequence_length)`, *optional*):
Segment token indices to indicate first and second portions of the inputs. Indices are selected in `[0,
1]`:
- 0 corresponds to a *sentence A* token,
- 1 corresponds to a *sentence B* token.
[What are token type IDs?](../glossary#token-type-ids)
position_ids (`tf.Tensor` or `Numpy array` of shape `(batch_size, sequence_length)`, *optional*):
Indices of positions of each input sequence tokens in the position embeddings. Selected in the range `[0,
config.max_position_embeddings - 1]`.
[What are position IDs?](../glossary#position-ids)
head_mask (`Numpy array` or `tf.Tensor` of shape `(num_heads,)` or `(num_layers, num_heads)`, *optional*):
Mask to nullify selected heads of the self-attention modules. Mask values selected in `[0, 1]`:
- 1 indicates the head is **not masked**,
- 0 indicates the head is **masked**.
inputs_embeds (`tf.Tensor` of shape `(batch_size, sequence_length, hidden_size)`, *optional*):
Optionally, instead of passing `input_ids` you can choose to directly pass an embedded representation. This
is useful if you want more control over how to convert `input_ids` indices into associated vectors than the
model's internal embedding lookup matrix.
output_attentions (`bool`, *optional*):
Whether or not to return the attentions tensors of all attention layers. See `attentions` under returned
tensors for more detail. This argument can be used only in eager mode, in graph mode the value in the
config will be used instead.
output_hidden_states (`bool`, *optional*):
Whether or not to return the hidden states of all layers. See `hidden_states` under returned tensors for
more detail. This argument can be used only in eager mode, in graph mode the value in the config will be
used instead.
return_dict (`bool`, *optional*):
Whether or not to return a [`~utils.ModelOutput`] instead of a plain tuple. This argument can be used in
eager mode, in graph mode the value will always be set to True.
training (`bool`, *optional*, defaults to `False`):
Whether or not to use the model in training mode (some modules like dropout modules have different
behaviors between training and evaluation).
"""
@add_start_docstrings(
"The bare GPT2 Model transformer outputting raw hidden-states without any specific head on top.",
GPT2_START_DOCSTRING,
)
class TFGPT2Model(TFGPT2PreTrainedModel):
def __init__(self, config, *inputs, **kwargs):
super().__init__(config, *inputs, **kwargs)
self.transformer = TFGPT2MainLayer(config, name="transformer")
@unpack_inputs
@add_start_docstrings_to_model_forward(GPT2_INPUTS_DOCSTRING)
@add_code_sample_docstrings(
checkpoint=_CHECKPOINT_FOR_DOC,
output_type=TFBaseModelOutputWithPastAndCrossAttentions,
config_class=_CONFIG_FOR_DOC,
)
def call(
self,
input_ids: TFModelInputType | None = None,
past_key_values: Optional[Tuple[Tuple[Union[np.ndarray, tf.Tensor]]]] = None,
attention_mask: np.ndarray | tf.Tensor | None = None,
token_type_ids: np.ndarray | tf.Tensor | None = None,
position_ids: np.ndarray | tf.Tensor | None = None,
head_mask: np.ndarray | tf.Tensor | None = None,
inputs_embeds: np.ndarray | tf.Tensor | None = None,
encoder_hidden_states: np.ndarray | tf.Tensor | None = None,
encoder_attention_mask: np.ndarray | tf.Tensor | None = None,
use_cache: Optional[bool] = None,
output_attentions: Optional[bool] = None,
output_hidden_states: Optional[bool] = None,
return_dict: Optional[bool] = None,
training: Optional[bool] = False,
) -> Union[TFBaseModelOutputWithPastAndCrossAttentions, Tuple[tf.Tensor]]:
r"""
encoder_hidden_states (`tf.Tensor` of shape `(batch_size, sequence_length, hidden_size)`, *optional*):
Sequence of hidden-states at the output of the last layer of the encoder. Used in the cross-attention if
the model is configured as a decoder.
encoder_attention_mask (`tf.Tensor` of shape `(batch_size, sequence_length)`, *optional*):
Mask to avoid performing attention on the padding token indices of the encoder input. This mask is used in
the cross-attention if the model is configured as a decoder. Mask values selected in `[0, 1]`:
- 1 for tokens that are **not masked**,
- 0 for tokens that are **masked**.
past_key_values (`Tuple[Tuple[tf.Tensor]]` of length `config.n_layers`)
contains precomputed key and value hidden states of the attention blocks. Can be used to speed up decoding.
If `past` are used, the user can optionally input only the last `decoder_input_ids` (those that don't have
their past key value states given to this model) of shape `(batch_size, 1)` instead of all
`decoder_input_ids` of shape `(batch_size, sequence_length)`.
use_cache (`bool`, *optional*, defaults to `True`):
If set to `True`, `past_key_values` key value states are returned and can be used to speed up decoding (see
`past`). Set to `False` during training, `True` during generation
"""
outputs = self.transformer(
input_ids=input_ids,
past_key_values=past_key_values,
attention_mask=attention_mask,
token_type_ids=token_type_ids,
position_ids=position_ids,
head_mask=head_mask,
inputs_embeds=inputs_embeds,
encoder_hidden_states=encoder_hidden_states,
encoder_attention_mask=encoder_attention_mask,
use_cache=use_cache,
output_attentions=output_attentions,
output_hidden_states=output_hidden_states,
return_dict=return_dict,
training=training,
)
return outputs
def build(self, input_shape=None):
if self.built:
return
self.built = True
if getattr(self, "transformer", None) is not None:
with tf.name_scope(self.transformer.name):
self.transformer.build(None)
@add_start_docstrings(
"""
The GPT2 Model transformer with a language modeling head on top (linear layer with weights tied to the input
embeddings).
""",
GPT2_START_DOCSTRING,
)
class TFGPT2LMHeadModel(TFGPT2PreTrainedModel, TFCausalLanguageModelingLoss):
def __init__(self, config, *inputs, **kwargs):
super().__init__(config, *inputs, **kwargs)
self.transformer = TFGPT2MainLayer(config, name="transformer")
def get_output_embeddings(self):
return self.get_input_embeddings()
def set_output_embeddings(self, value):
self.set_input_embeddings(value)
def prepare_inputs_for_generation(self, inputs, past_key_values=None, use_cache=None, **kwargs):
token_type_ids = kwargs.get("token_type_ids", None)
# only last token for inputs_ids if past is defined in kwargs
if past_key_values:
inputs = tf.expand_dims(inputs[:, -1], -1)
if token_type_ids is not None:
token_type_ids = tf.expand_dims(token_type_ids[:, -1], -1)
position_ids = kwargs.get("position_ids", None)
attention_mask = kwargs.get("attention_mask", None)
if attention_mask is not None and position_ids is None:
position_ids = tf.math.cumsum(attention_mask, axis=-1, exclusive=True)
if past_key_values:
position_ids = tf.expand_dims(position_ids[:, -1], -1)
return {
"input_ids": inputs,
"attention_mask": attention_mask,
"position_ids": position_ids,
"past_key_values": past_key_values,
"use_cache": use_cache,
"token_type_ids": token_type_ids,
}
@unpack_inputs
@add_start_docstrings_to_model_forward(GPT2_INPUTS_DOCSTRING)
@add_code_sample_docstrings(
checkpoint=_CHECKPOINT_FOR_DOC,
output_type=TFCausalLMOutputWithCrossAttentions,
config_class=_CONFIG_FOR_DOC,
)
def call(
self,
input_ids: TFModelInputType | None = None,
past_key_values: Optional[Tuple[Tuple[Union[np.ndarray, tf.Tensor]]]] = None,
attention_mask: np.ndarray | tf.Tensor | None = None,
token_type_ids: np.ndarray | tf.Tensor | None = None,
position_ids: np.ndarray | tf.Tensor | None = None,
head_mask: np.ndarray | tf.Tensor | None = None,
inputs_embeds: np.ndarray | tf.Tensor | None = None,
encoder_hidden_states: np.ndarray | tf.Tensor | None = None,
encoder_attention_mask: np.ndarray | tf.Tensor | None = None,
use_cache: Optional[bool] = None,
output_attentions: Optional[bool] = None,
output_hidden_states: Optional[bool] = None,
return_dict: Optional[bool] = None,
labels: np.ndarray | tf.Tensor | None = None,
training: Optional[bool] = False,
) -> Union[TFCausalLMOutputWithCrossAttentions, Tuple[tf.Tensor]]:
r"""
encoder_hidden_states (`tf.Tensor` of shape `(batch_size, sequence_length, hidden_size)`, *optional*):
Sequence of hidden-states at the output of the last layer of the encoder. Used in the cross-attention if
the model is configured as a decoder.
encoder_attention_mask (`tf.Tensor` of shape `(batch_size, sequence_length)`, *optional*):
Mask to avoid performing attention on the padding token indices of the encoder input. This mask is used in
the cross-attention if the model is configured as a decoder. Mask values selected in `[0, 1]`:
- 1 for tokens that are **not masked**,
- 0 for tokens that are **masked**.
past_key_values (`Tuple[Tuple[tf.Tensor]]` of length `config.n_layers`)
contains precomputed key and value hidden states of the attention blocks. Can be used to speed up decoding.
If `past` are used, the user can optionally input only the last `decoder_input_ids` (those that don't have
their past key value states given to this model) of shape `(batch_size, 1)` instead of all
`decoder_input_ids` of shape `(batch_size, sequence_length)`.
use_cache (`bool`, *optional*, defaults to `True`):
If set to `True`, `past_key_values` key value states are returned and can be used to speed up decoding (see
`past`). Set to `False` during training, `True` during generation
labels (`tf.Tensor` of shape `(batch_size, sequence_length)`, *optional*):
Labels for computing the cross entropy classification loss. Indices should be in `[0, ...,
config.vocab_size - 1]`.
"""
transformer_outputs = self.transformer(
input_ids=input_ids,
past_key_values=past_key_values,
attention_mask=attention_mask,
token_type_ids=token_type_ids,
position_ids=position_ids,
head_mask=head_mask,
inputs_embeds=inputs_embeds,
encoder_hidden_states=encoder_hidden_states,
encoder_attention_mask=encoder_attention_mask,
use_cache=use_cache,
output_attentions=output_attentions,
output_hidden_states=output_hidden_states,
return_dict=return_dict,
training=training,
)
hidden_states = transformer_outputs[0]
logits = tf.matmul(hidden_states, self.transformer.wte.weights, transpose_b=True)
loss = None
if labels is not None:
# shift labels to the left and cut last logit token
shifted_logits = logits[:, :-1]
labels = labels[:, 1:]
loss = self.hf_compute_loss(labels, shifted_logits)
if not return_dict:
output = (logits,) + transformer_outputs[1:]
return ((loss,) + output) if loss is not None else output
return TFCausalLMOutputWithCrossAttentions(
loss=loss,
logits=logits,
past_key_values=transformer_outputs.past_key_values,
hidden_states=transformer_outputs.hidden_states,
attentions=transformer_outputs.attentions,
cross_attentions=transformer_outputs.cross_attentions,
)
def build(self, input_shape=None):
if self.built:
return
self.built = True
if getattr(self, "transformer", None) is not None:
with tf.name_scope(self.transformer.name):
self.transformer.build(None)
@add_start_docstrings(
"""
The GPT2 Model transformer with a language modeling and a multiple-choice classification head on top e.g. for
RocStories/SWAG tasks. The two heads are two linear layers. The language modeling head has its weights tied to the
input embeddings, the classification head takes as input the input of a specified classification token index in the
input sequence).
""",
GPT2_START_DOCSTRING,
)
class TFGPT2DoubleHeadsModel(TFGPT2PreTrainedModel):
def __init__(self, config, *inputs, **kwargs):
super().__init__(config, *inputs, **kwargs)
config.num_labels = 1
self.transformer = TFGPT2MainLayer(config, name="transformer")
self.multiple_choice_head = TFSequenceSummary(
config, initializer_range=config.initializer_range, name="multiple_choice_head"
)
@unpack_inputs
@add_start_docstrings_to_model_forward(GPT2_INPUTS_DOCSTRING)
@replace_return_docstrings(output_type=TFGPT2DoubleHeadsModelOutput, config_class=_CONFIG_FOR_DOC)
def call(
self,
input_ids: TFModelInputType | None = None,
past_key_values: Optional[Tuple[Tuple[Union[np.ndarray, tf.Tensor]]]] = None,
attention_mask: np.ndarray | tf.Tensor | None = None,
token_type_ids: np.ndarray | tf.Tensor | None = None,
position_ids: np.ndarray | tf.Tensor | None = None,
head_mask: np.ndarray | tf.Tensor | None = None,
inputs_embeds: np.ndarray | tf.Tensor | None = None,
mc_token_ids: np.ndarray | tf.Tensor | None = None,
use_cache: Optional[bool] = None,
output_attentions: Optional[bool] = None,
output_hidden_states: Optional[bool] = None,
return_dict: Optional[bool] = None,
training: Optional[bool] = False,
) -> Union[TFGPT2DoubleHeadsModelOutput, Tuple[tf.Tensor]]:
r"""
mc_token_ids (`tf.Tensor` or `Numpy array` of shape `(batch_size, num_choices)`, *optional*, default to index of the last token of the input):
Index of the classification token in each input sequence. Selected in the range `[0, input_ids.size(-1) -
1]`.
Return:
Examples:
```python
>>> import tensorflow as tf
>>> from transformers import AutoTokenizer, TFGPT2DoubleHeadsModel
>>> tokenizer = AutoTokenizer.from_pretrained("gpt2")
>>> model = TFGPT2DoubleHeadsModel.from_pretrained("gpt2")
>>> # Add a [CLS] to the vocabulary (we should train it also!)
>>> num_added_tokens = tokenizer.add_special_tokens({"cls_token": "[CLS]"})
>>> embedding_layer = model.resize_token_embeddings(
... len(tokenizer)
... ) # Update the model embeddings with the new vocabulary size
>>> choices = ["Hello, my dog is cute [CLS]", "Hello, my cat is cute [CLS]"]
>>> encoded_choices = [tokenizer.encode(s) for s in choices]
>>> cls_token_location = [tokens.index(tokenizer.cls_token_id) for tokens in encoded_choices]
>>> input_ids = tf.constant(encoded_choices)[None, :] # Batch size: 1, number of choices: 2
>>> mc_token_ids = tf.constant([cls_token_location]) # Batch size: 1
>>> outputs = model(input_ids, mc_token_ids=mc_token_ids)
>>> lm_prediction_scores, mc_prediction_scores = outputs[:2]
```"""
if input_ids is not None:
input_shapes = shape_list(input_ids)
else:
input_shapes = shape_list(inputs_embeds)[:-1]
seq_length = input_shapes[-1]
flat_input_ids = tf.reshape(input_ids, (-1, seq_length)) if input_ids is not None else None
flat_attention_mask = tf.reshape(attention_mask, (-1, seq_length)) if attention_mask is not None else None
flat_token_type_ids = tf.reshape(token_type_ids, (-1, seq_length)) if token_type_ids is not None else None
flat_position_ids = tf.reshape(position_ids, (-1, seq_length)) if position_ids is not None else None
transformer_outputs = self.transformer(
input_ids=flat_input_ids,
past_key_values=past_key_values,
attention_mask=flat_attention_mask,
token_type_ids=flat_token_type_ids,
position_ids=flat_position_ids,
head_mask=head_mask,
inputs_embeds=inputs_embeds,
encoder_hidden_states=None,
encoder_attention_mask=None,
use_cache=use_cache,
output_attentions=output_attentions,
output_hidden_states=output_hidden_states,
return_dict=return_dict,
training=training,
)
hidden_states = transformer_outputs[0]
hidden_states = tf.reshape(hidden_states, input_shapes + shape_list(hidden_states)[-1:])
if return_dict and output_hidden_states:
# We do this to match the slightly odd PT behaviour - the final hidden state is reshaped to rank 4 when the
# input is rank 3, but all other hidden states remain at rank-3 (with the first 2 dims merged)
all_hidden_states = transformer_outputs.hidden_states[:-1] + (hidden_states,)
else:
all_hidden_states = None
lm_logits = tf.matmul(hidden_states, self.transformer.wte.weights, transpose_b=True)
mc_logits = self.multiple_choice_head(hidden_states, mc_token_ids, training=training)
mc_logits = tf.squeeze(mc_logits, axis=-1)
if not return_dict:
return (lm_logits, mc_logits) + transformer_outputs[1:]
return TFGPT2DoubleHeadsModelOutput(
logits=lm_logits,
mc_logits=mc_logits,
past_key_values=transformer_outputs.past_key_values,
hidden_states=all_hidden_states,
attentions=transformer_outputs.attentions,
)
@property
def input_signature(self):
return {
"input_ids": tf.TensorSpec((None, None, None), tf.int32, name="input_ids"),
"attention_mask": tf.TensorSpec((None, None, None), tf.int32, name="attention_mask"),
"mc_token_ids": tf.TensorSpec((None, None), tf.int32, name="mc_token_ids"),
}
def build(self, input_shape=None):
if self.built:
return
self.built = True
if getattr(self, "transformer", None) is not None:
with tf.name_scope(self.transformer.name):
self.transformer.build(None)
if getattr(self, "multiple_choice_head", None) is not None:
with tf.name_scope(self.multiple_choice_head.name):
self.multiple_choice_head.build(None)
@add_start_docstrings(
"""
The GPT2 Model transformer with a sequence classification head on top (linear layer).
[`TFGPT2ForSequenceClassification`] uses the last token in order to do the classification, as other causal models
(e.g. GPT-1) do.
Since it does classification on the last token, it requires to know the position of the last token. If a
`pad_token_id` is defined in the configuration, it finds the last token that is not a padding token in each row. If
no `pad_token_id` is defined, it simply takes the last value in each row of the batch. Since it cannot guess the
padding tokens when `inputs_embeds` are passed instead of `input_ids`, it does the same (take the last value in
each row of the batch).
""",
GPT2_START_DOCSTRING,
)
class TFGPT2ForSequenceClassification(TFGPT2PreTrainedModel, TFSequenceClassificationLoss):
def __init__(self, config, *inputs, **kwargs):
super().__init__(config, *inputs, **kwargs)
self.num_labels = config.num_labels
self.score = keras.layers.Dense(
config.num_labels,
kernel_initializer=get_initializer(config.initializer_range),
name="score",
use_bias=False,
)
self.transformer = TFGPT2MainLayer(config, name="transformer")
self.config = config
@unpack_inputs
@add_start_docstrings_to_model_forward(GPT2_INPUTS_DOCSTRING)
@add_code_sample_docstrings(
checkpoint="microsoft/DialogRPT-updown",
output_type=TFSequenceClassifierOutputWithPast,
config_class=_CONFIG_FOR_DOC,
)
def call(
self,
input_ids: TFModelInputType | None = None,
past_key_values: Optional[Tuple[Tuple[Union[np.ndarray, tf.Tensor]]]] = None,
attention_mask: np.ndarray | tf.Tensor | None = None,
token_type_ids: np.ndarray | tf.Tensor | None = None,
position_ids: np.ndarray | tf.Tensor | None = None,
head_mask: np.ndarray | tf.Tensor | None = None,
inputs_embeds: np.ndarray | tf.Tensor | None = None,
use_cache: Optional[bool] = None,
output_attentions: Optional[bool] = None,
output_hidden_states: Optional[bool] = None,
return_dict: Optional[bool] = None,
labels: np.ndarray | tf.Tensor | None = None,
training: Optional[bool] = False,
) -> Union[TFSequenceClassifierOutputWithPast, Tuple[tf.Tensor]]:
r"""
labels (`tf.Tensor` of shape `(batch_size, sequence_length)`, *optional*):
Labels for computing the cross entropy classification loss. Indices should be in `[0, ...,
config.vocab_size - 1]`.
"""
transformer_outputs = self.transformer(
input_ids=input_ids,
past_key_values=past_key_values,
attention_mask=attention_mask,
token_type_ids=token_type_ids,
position_ids=position_ids,
head_mask=head_mask,
inputs_embeds=inputs_embeds,
use_cache=use_cache,
output_attentions=output_attentions,
output_hidden_states=output_hidden_states,
return_dict=return_dict,
training=training,
)
hidden_states = transformer_outputs[0]
logits = self.score(hidden_states)
logits_shape = shape_list(logits)
in_logits = None
if self.config.pad_token_id is None:
sequence_lengths = -1
else:
if input_ids is not None:
sequence_lengths = (
tf.argmax(tf.cast(tf.math.equal(input_ids, self.config.pad_token_id), input_ids.dtype), axis=-1)
- 1
)
sequence_lengths = tf.where(sequence_lengths >= 0, sequence_lengths, input_ids.shape[-1] - 1)
in_logits = tf.gather(logits, sequence_lengths, batch_dims=1, axis=1)
else:
sequence_lengths = -1
logger.warning(
f"{self.__class__.__name__} will not detect padding tokens in `inputs_embeds`. Results may be "
"unexpected if using padding tokens in conjunction with `inputs_embeds.`"
)
loss = None
if labels is not None:
assert (
self.config.pad_token_id is not None or logits_shape[0] == 1
), "Cannot handle batch sizes > 1 if no padding token is defined."
if not tf.is_tensor(sequence_lengths):
in_logits = logits[0 : logits_shape[0], sequence_lengths]
loss = self.hf_compute_loss(tf.reshape(labels, [-1]), tf.reshape(in_logits, [-1, self.num_labels]))
pooled_logits = in_logits if in_logits is not None else logits
if not return_dict:
output = (pooled_logits,) + transformer_outputs[1:]
return ((loss,) + output) if loss is not None else output
return TFSequenceClassifierOutputWithPast(
loss=loss,
logits=pooled_logits,
past_key_values=transformer_outputs.past_key_values,
hidden_states=transformer_outputs.hidden_states,
attentions=transformer_outputs.attentions,
)
def build(self, input_shape=None):
if self.built:
return
self.built = True
if getattr(self, "score", None) is not None:
with tf.name_scope(self.score.name):
self.score.build([None, None, self.config.n_embd])
if getattr(self, "transformer", None) is not None:
with tf.name_scope(self.transformer.name):
self.transformer.build(None)
| transformers/src/transformers/models/gpt2/modeling_tf_gpt2.py/0 | {
"file_path": "transformers/src/transformers/models/gpt2/modeling_tf_gpt2.py",
"repo_id": "transformers",
"token_count": 24673
} | 303 |
# coding=utf-8
# Copyright 2023 HuggingFace Inc. team. All rights reserved.
#
# Licensed under the Apache License, Version 2.0 (the "License");
# you may not use this file except in compliance with the License.
# You may obtain a copy of the License at
#
# http://www.apache.org/licenses/LICENSE-2.0
#
# Unless required by applicable law or agreed to in writing, software
# distributed under the License is distributed on an "AS IS" BASIS,
# WITHOUT WARRANTIES OR CONDITIONS OF ANY KIND, either express or implied.
# See the License for the specific language governing permissions and
# limitations under the License.
"""Tokenization classes for GPTSANJapanese."""
import collections
import json
import os
import re
from typing import List, Optional, Tuple, Union
import numpy as np
from ...tokenization_utils import PreTrainedTokenizer
from ...tokenization_utils_base import (
BatchEncoding,
PreTokenizedInput,
PreTokenizedInputPair,
TextInput,
TextInputPair,
TruncationStrategy,
)
from ...utils import PaddingStrategy, logging
logger = logging.get_logger(__name__)
VOCAB_FILES_NAMES = {"vocab_file": "vocab.txt", "emoji_file": "emoji.json"}
PRETRAINED_VOCAB_FILES_MAP = {
"vocab_file": {
"Tanrei/GPTSAN-japanese": "https://huggingface.co/Tanrei/GPTSAN-japanese/blob/main/vocab.txt",
},
"emoji_file": {
"Tanrei/GPTSAN-japanese": "https://huggingface.co/Tanrei/GPTSAN-japanese/blob/main/emoji.json",
},
}
PRETRAINED_POSITIONAL_EMBEDDINGS_SIZES = {
"Tanrei/GPTSAN-japanese": 1280,
}
def load_vocab_and_emoji(vocab_file, emoji_file):
"""Loads a vocabulary file and emoji file into a dictionary."""
with open(emoji_file, "r", encoding="utf-8") as f:
emoji = json.loads(f.read())
vocab = collections.OrderedDict()
raw_vocab = collections.OrderedDict()
ids_to_tokens = collections.OrderedDict()
with open(vocab_file, "r", encoding="utf-8") as f:
token = f.readlines()
token = [[t.rstrip("\n")] if (t == ",\n" or "," not in t) else t.rstrip("\n").split(",") for t in token]
for idx, b in enumerate(token):
ids_to_tokens[idx] = b
raw_vocab[",".join(b)] = idx
for wd in b:
vocab[wd] = idx
return vocab, raw_vocab, ids_to_tokens, emoji
class GPTSanJapaneseTokenizer(PreTrainedTokenizer):
"""
This tokenizer is based on GPTNeoXJapaneseTokenizer and has the following modifications
- Decoding byte0~byte255 tokens correctly
- Added bagofword token handling
- Return token_type_ids for Prefix-LM model
The bagofword token represents a repetition of the previous token and is converted to 3 consecutive tokens when
decoding In addition, the original Japanese special Sub-Word-Encoding has been released in this repository
(https://github.com/tanreinama/Japanese-BPEEncoder_V2). The token_type_ids is a mask indicating the prefix input
position of the Prefix-LM model. To specify a prefix position, specify a prefix input for prefix_text, or specify a
sentence of the prefix part and the part after it as a text pair of batch input.
Example:
```python
>>> from transformers import GPTSanJapaneseTokenizer
>>> tokenizer = GPTSanJapaneseTokenizer.from_pretrained("Tanrei/GPTSAN-japanese")
>>> # You can confirm both 慶応 and 慶應 are encoded to 17750
>>> tokenizer("吾輩は猫である🐯。実は慶応(慶應)大学出身")["input_ids"]
[35993, 35998, 34347, 31459, 30647, 31448, 25, 30659, 35729, 35676, 32417, 30647, 17750, 35589, 17750, 35590, 321, 1281]
>>> # Both 慶応 and 慶應 are decoded to 慶応
>>> tokenizer.decode(tokenizer("吾輩は猫である🐯。実は慶応(慶應)大学出身")["input_ids"])
'吾輩は猫である🐯。実は慶応(慶応)大学出身'
```
Example for Prefix-LM:
```python
>>> from transformers import GPTSanJapaneseTokenizer
>>> tokenizer = GPTSanJapaneseTokenizer.from_pretrained("Tanrei/GPTSAN-japanese")
>>> tokenizer("実は慶応(慶應)大学出身", prefix_text="吾輩は猫である🐯。")["input_ids"]
[35993, 34347, 31459, 30647, 31448, 25, 30659, 35729, 35676, 35998, 32417, 30647, 17750, 35589, 17750, 35590, 321, 1281]
>>> # Mask for Prefix-LM inputs
>>> tokenizer("実は慶応(慶應)大学出身", prefix_text="吾輩は猫である🐯。")["token_type_ids"]
[1, 1, 1, 1, 1, 1, 1, 1, 1, 0, 0, 0, 0, 0, 0, 0, 0, 0]
```
Example for batch encode:
```python
>>> from transformers import GPTSanJapaneseTokenizer
>>> tokenizer = GPTSanJapaneseTokenizer.from_pretrained("Tanrei/GPTSAN-japanese")
>>> tokenizer([["武田信玄", "は、"], ["織田信長", "の配下の、"]], padding=True)["input_ids"]
[[35993, 8640, 25948, 35998, 30647, 35675, 35999, 35999], [35993, 10382, 9868, 35998, 30646, 9459, 30646, 35675]]
>>> # Mask for Prefix-LM inputs
>>> tokenizer([["武田信玄", "は、"], ["織田信長", "の配下の、"]], padding=True)["token_type_ids"]
[[1, 1, 1, 0, 0, 0, 0, 0], [1, 1, 1, 0, 0, 0, 0, 0]]
>>> # Mask for padding
>>> tokenizer([["武田信玄", "は、"], ["織田信長", "の配下の、"]], padding=True)["attention_mask"]
[[1, 1, 1, 1, 1, 1, 0, 0], [1, 1, 1, 1, 1, 1, 1, 1]]
```
Args:
vocab_file (`str`):
File containing the vocabulary.
emoji_file (`str`):
File containing the emoji.
unk_token (`str`, *optional*, defaults to `"<|nottoken|>"`):
The token used for unknown charactor
pad_token (`str`, *optional*, defaults to `"<|separator|>"`):
The token used for padding
bos_token (`str`, *optional*, defaults to `"<|startoftext|>"`):
The beginning of sequence token.
eos_token (`str`, *optional*, defaults to `"<|endoftext|>"`):
The end of sequence token.
sep_token (`str`, *optional*, defaults to `"<|segmenter|>"`):
A special token to separate token to prefix part and general input part.
do_clean_text (`bool`, *optional*, defaults to `False`):
Whether or not to clean text for URL, EMAIL, TEL, Japanese DATE and Japanese PRICE.
"""
vocab_files_names = VOCAB_FILES_NAMES
pretrained_vocab_files_map = PRETRAINED_VOCAB_FILES_MAP
max_model_input_sizes = PRETRAINED_POSITIONAL_EMBEDDINGS_SIZES
model_input_names = ["input_ids", "attention_mask", "token_type_ids"]
def __init__(
self,
vocab_file,
emoji_file,
unk_token="<|nottoken|>",
pad_token="<|separator|>",
bos_token="<|startoftext|>",
eos_token="<|endoftext|>",
sep_token="<|segmenter|>",
do_clean_text=False,
**kwargs,
):
if not os.path.isfile(vocab_file):
raise ValueError(
f"Can't find a vocabulary file at path '{vocab_file}'. To load the vocabulary from a Google pretrained"
" model use `tokenizer = GPTSanJapaneseTokenizer.from_pretrained(PRETRAINED_MODEL_NAME)`"
)
if not os.path.isfile(emoji_file):
raise ValueError(
f"Can't find a emoji file at path '{emoji_file}'. To load the emoji information from a Google"
" pretrained model use `tokenizer = GPTSanJapaneseTokenizer.from_pretrained(PRETRAINED_MODEL_NAME)`"
)
self.do_clean_text = do_clean_text
self.vocab, self.raw_vocab, self.ids_to_tokens, self.emoji = load_vocab_and_emoji(vocab_file, emoji_file)
self.subword_tokenizer = SubWordJapaneseTokenizer(
vocab=self.vocab, ids_to_tokens=self.ids_to_tokens, emoji=self.emoji
)
super().__init__(
unk_token=unk_token,
pad_token=pad_token,
bos_token=bos_token,
eos_token=eos_token,
sep_token=sep_token,
do_clean_text=do_clean_text,
**kwargs,
)
@property
# Copied from tokenization_gpt_neox_japanese.GPTNeoXJapaneseTokenizer.vocab_size
def vocab_size(self):
# self.vocab contains support for character fluctuation unique to Japanese, and has a large number of vocab
return len(self.raw_vocab)
# Copied from tokenization_gpt_neox_japanese.GPTNeoXJapaneseTokenizer.get_vocab
def get_vocab(self):
return dict(self.raw_vocab, **self.added_tokens_encoder)
# Copied from tokenization_gpt_neox_japanese.GPTNeoXJapaneseTokenizer._tokenize
def _tokenize(self, text):
return self.subword_tokenizer.tokenize(text, clean=self.do_clean_text)
# Copied from tokenization_gpt_neox_japanese.GPTNeoXJapaneseTokenizer._convert_token_to_id
def _convert_token_to_id(self, token):
"""Converts a token (str) in an id using the vocab."""
return self.vocab.get(token, self.vocab.get(self.unk_token))
# Copied from tokenization_gpt_neox_japanese.GPTNeoXJapaneseTokenizer._convert_id_to_token
def _convert_id_to_token(self, index):
"""Converts an index (integer) in a token (str) using the vocab."""
return self.subword_tokenizer.convert_id_to_token(index)
def convert_tokens_to_string(self, tokens):
"""Converts a sequence of tokens (string) in a single string."""
words = []
byte_tokens = []
for word in tokens:
if word[:6] == "<|byte" and word[-2:] == "|>":
byte_tokens.append(int(word[6:-2]))
else:
if len(byte_tokens) > 0:
words.append(bytearray(byte_tokens).decode("utf-8", errors="replace"))
byte_tokens = []
if word[:7] == "<|emoji" and word[-2:] == "|>":
words.append(self.emoji["emoji_inv"][word])
elif word == "<SP>":
words.append(" ")
elif word == "<BR>":
words.append("\n")
elif word == "<TAB>":
words.append("\t")
elif word == "<BLOCK>":
words.append("▀")
elif word == "<KIGOU>":
words.append("ǀ")
elif word == "<U2000U2BFF>":
words.append("‖")
elif word == "<|bagoftoken|>":
if len(words) > 0:
words.append(words[-1])
words.append(words[-1])
words.append(words[-1])
elif word.startswith("<|") and word.endswith("|>"):
words.append("")
else:
words.append(word)
if len(byte_tokens) > 0:
words.append(bytearray(byte_tokens).decode("utf-8", errors="replace"))
text = "".join(words)
return text
@property
def default_chat_template(self):
"""
A simple chat template that adds standard BOS, SEP and EOS tokens between messages while discarding role
information.
"""
logger.warning_once(
"\nNo chat template is defined for this tokenizer - using the default template "
f"for the {self.__class__.__name__} class. If the default is not appropriate for "
"your model, please set `tokenizer.chat_template` to an appropriate template. "
"See https://huggingface.co/docs/transformers/main/chat_templating for more information.\n"
)
return (
"{% for message in messages %}"
"{% if not loop.first %}{{ bos_token}}{% endif %}"
"{{ sep_token }}{{ message.content }} {{ eos_token }}"
"{% endfor %}"
)
# Copied from tokenization_gpt_neox_japanese.GPTNeoXJapaneseTokenizer.save_vocabulary
def save_vocabulary(self, save_directory: str, filename_prefix: Optional[str] = None) -> Tuple[str]:
index = 0
if os.path.isdir(save_directory):
vocab_file = os.path.join(
save_directory, (filename_prefix + "-" if filename_prefix else "") + VOCAB_FILES_NAMES["vocab_file"]
)
emoji_file = os.path.join(
save_directory, (filename_prefix + "-" if filename_prefix else "") + VOCAB_FILES_NAMES["emoji_file"]
)
else:
vocab_file = (
(filename_prefix + "-" if filename_prefix else "") + save_directory + VOCAB_FILES_NAMES["vocab_file"]
)
emoji_file = (
(filename_prefix + "-" if filename_prefix else "") + save_directory + VOCAB_FILES_NAMES["emoji_file"]
)
with open(vocab_file, "w", encoding="utf-8") as writer:
for token_index, token in self.ids_to_tokens.items():
if index != token_index:
logger.warning(
f"Saving vocabulary to {vocab_file}: vocabulary indices are not consecutive."
" Please check that the vocabulary is not corrupted!"
)
index = token_index
writer.write(",".join(token) + "\n")
index += 1
with open(emoji_file, "w", encoding="utf-8") as writer:
json.dump(self.emoji, writer)
return vocab_file, emoji_file
def create_token_type_ids_from_sequences(
self, token_ids_0: List[int], token_ids_1: Optional[List[int]] = None
) -> List[int]:
# docstyle-ignore
"""
The tokenizer returns token_type_ids as separators between the Prefix part and the rest.
token_type_ids is 1 for the Prefix part and 0 for the rest of the token.
Example:
```python
>>> from transformers import GPTSanJapaneseTokenizer
>>> tokenizer = GPTSanJapaneseTokenizer.from_pretrained("Tanrei/GPTSAN-japanese")
>>> x_token = tokenizer("アイウエ")
>>> # input_ids: | SOT | SEG | ア | イ | ウ | エ |
>>> # token_type_ids: | 1 | 0 | 0 | 0 | 0 | 0 |
>>> x_token = tokenizer("", prefix_text="アイウエ")
>>> # input_ids: | SOT | ア | イ | ウ | エ | SEG |
>>> # token_type_ids: | 1 | 1 | 1 | 1 | 1 | 0 |
>>> x_token = tokenizer("ウエ", prefix_text="アイ")
>>> # input_ids: | SOT | ア | イ | SEG | ウ | エ |
>>> # token_type_ids: | 1 | 1 | 1 | 0 | 0 | 0 |
```"""
prefix_len = 0
if self.sep_token in self.vocab:
segid = self.vocab[self.sep_token]
if segid in token_ids_0:
prefix_len = token_ids_0.index(segid)
if token_ids_1 is None:
total_len = len(token_ids_0)
else:
total_len = len(token_ids_0 + token_ids_1)
return prefix_len * [1] + (total_len - prefix_len) * [0]
def prepare_for_tokenization(self, text, prefix_text=None, add_sep_token=None, **kwargs):
# GPTSAN inserts extra SEP tokens in Prefix-LM in addition to SOT for text generation.
# SOT at the beginning of the text, and SEP at the separator between the Prefix part and the rest.
if add_sep_token is None:
add_sep_token = self.sep_token not in text # If insert un-prefix position explicitly
prepared = self.bos_token if self.bos_token in self.vocab else ""
prepared += prefix_text if prefix_text is not None else ""
if add_sep_token:
prepared += self.sep_token if self.sep_token in self.vocab else ""
prepared += text
return (prepared, kwargs)
def _batch_encode_plus(
self,
batch_text_or_text_pairs: Union[
List[TextInput], List[TextInputPair], List[PreTokenizedInput], List[PreTokenizedInputPair]
],
add_special_tokens: bool = True,
padding_strategy: PaddingStrategy = PaddingStrategy.DO_NOT_PAD,
truncation_strategy: TruncationStrategy = TruncationStrategy.DO_NOT_TRUNCATE,
max_length: Optional[int] = None,
stride: int = 0,
is_split_into_words: bool = False,
pad_to_multiple_of: Optional[int] = None,
return_tensors: Optional[str] = None,
return_token_type_ids: Optional[bool] = None,
return_attention_mask: Optional[bool] = None,
return_overflowing_tokens: bool = False,
return_special_tokens_mask: bool = False,
return_offsets_mapping: bool = False,
return_length: bool = False,
verbose: bool = True,
) -> BatchEncoding:
# This tokenizer converts input text pairs into Prefix input and subsequent input
if isinstance(batch_text_or_text_pairs[0], tuple) or isinstance(tuple(batch_text_or_text_pairs[0]), list):
# As a single text with an explicit un-prefix position
batch_prefix_texts = []
for pref, txt in batch_text_or_text_pairs:
batch_prefix_texts.append(pref + self.sep_token + txt)
batch_text_or_text_pairs = batch_prefix_texts
return super()._batch_encode_plus(
batch_text_or_text_pairs,
add_special_tokens,
padding_strategy,
truncation_strategy,
max_length,
stride,
is_split_into_words,
pad_to_multiple_of,
return_tensors,
return_token_type_ids,
return_attention_mask,
return_overflowing_tokens,
return_special_tokens_mask,
return_offsets_mapping,
return_length,
verbose,
)
class SubWordJapaneseTokenizer(object):
"""
This tokenizer is based on GPTNeoXJapaneseTokenizer and has the following modifications
- Decoding byte0~byte255 tokens correctly
- Added bagofword token handling
https://github.com/tanreinama/Japanese-BPEEncoder_V2 This tokenizer class is under MIT Lisence according to the
original repository.
MIT License
Copyright (c) 2020 tanreinama
Permission is hereby granted, free of charge, to any person obtaining a copy of this software and associated
documentation files (the "Software"), to deal in the Software without restriction, including without limitation the
rights to use, copy, modify, merge, publish, distribute, sublicense, and/or sell copies of the Software, and to
permit persons to whom the Software is furnished to do so, subject to the following conditions:
The above copyright notice and this permission notice shall be included in all copies or substantial portions of
the Software.
THE SOFTWARE IS PROVIDED "AS IS", WITHOUT WARRANTY OF ANY KIND, EXPRESS OR IMPLIED, INCLUDING BUT NOT LIMITED TO
THE WARRANTIES OF MERCHANTABILITY, FITNESS FOR A PARTICULAR PURPOSE AND NONINFRINGEMENT. IN NO EVENT SHALL THE
AUTHORS OR COPYRIGHT HOLDERS BE LIABLE FOR ANY CLAIM, DAMAGES OR OTHER LIABILITY, WHETHER IN AN ACTION OF CONTRACT,
TORT OR OTHERWISE, ARISING FROM, OUT OF OR IN CONNECTION WITH THE SOFTWARE OR THE USE OR OTHER DEALINGS IN THE
SOFTWARE.
"""
# Copied from tokenization_gpt_neox_japanese.SubWordJapaneseTokenizer.__init__
def __init__(self, vocab, ids_to_tokens, emoji):
self.vocab = vocab # same as swe
self.ids_to_tokens = ids_to_tokens # same as bpe
self.emoji = emoji
self.maxlen = np.max([len(w) for w in self.vocab.keys()])
self.content_repatter1 = re.compile(r"(https?|ftp)(:\/\/[-_\.!~*\'()a-zA-Z0-9;\/?:\@&=\+$,%#]+)")
self.content_repatter2 = re.compile(r"[A-Za-z0-9\._+]*@[\-_0-9A-Za-z]+(\.[A-Za-z]+)*")
self.content_repatter3 = re.compile(r"[\(]{0,1}[0-9]{2,4}[\)\-\(]{0,1}[0-9]{2,4}[\)\-]{0,1}[0-9]{3,4}")
self.content_repatter4 = re.compile(
r"([12]\d{3}[/\-年])*(0?[1-9]|1[0-2])[/\-月]((0?[1-9]|[12][0-9]|3[01])日?)*(\d{1,2}|:|\d{1,2}時|\d{1,2}分|\(日\)|\(月\)|\(火\)|\(水\)|\(木\)|\(金\)|\(土\)|㈰|㈪|㈫|㈬|㈭|㈮|㈯)*"
)
self.content_repatter5 = re.compile(
r"(明治|大正|昭和|平成|令和|㍾|㍽|㍼|㍻|\u32ff)\d{1,2}年(0?[1-9]|1[0-2])月(0?[1-9]|[12][0-9]|3[01])日(\d{1,2}|:|\d{1,2}時|\d{1,2}分|\(日\)|\(月\)|\(火\)|\(水\)|\(木\)|\(金\)|\(土\)|㈰|㈪|㈫|㈬|㈭|㈮|㈯)*"
)
self.content_repatter6 = re.compile(
r"((0|[1-9]\d*|[1-9]\d{0,2}(,\d{3})+)*億)*((0|[1-9]\d*|[1-9]\d{0,2}(,\d{3})+)*万)*((0|[1-9]\d*|[1-9]\d{0,2}(,\d{3})+)*千)*(0|[1-9]\d*|[1-9]\d{0,2}(,\d{3})+)*(千円|万円|千万円|円|千ドル|万ドル|千万ドル|ドル|千ユーロ|万ユーロ|千万ユーロ|ユーロ)+(\(税込\)|\(税抜\)|\+tax)*"
)
keisen = "─━│┃┄┅┆┇┈┉┊┋┌┍┎┏┐┑┒┓└┕┖┗┘┙┚┛├┝┞┟┠┡┢┣┤┥┦┧┨┩┪┫┬┭┮┯┰┱┲┳┴┵┶┷┸┹┺┻┼┽┾┿╀╁╂╃╄╅╆╇╈╉╊╋╌╍╎╏═║╒╓╔╕╖╗╘╙╚╛╜╝╞╟╠╡╢╣╤╥╦╧╨╩╪╫╬╭╮╯╰╱╲╳╴╵╶╷╸╹╺╻╼╽╾╿"
blocks = "▀▁▂▃▄▅▆▇█▉▊▋▌▍▎▏▐░▒▓▔▕▖▗▘▙▚▛▜▝▞▟"
self.content_trans1 = str.maketrans({k: "<BLOCK>" for k in keisen + blocks})
# Copied from tokenization_gpt_neox_japanese.SubWordJapaneseTokenizer.__len__
def __len__(self):
return len(self.ids_to_tokens)
# Copied from tokenization_gpt_neox_japanese.SubWordJapaneseTokenizer.clean_text
def clean_text(self, content):
content = self.content_repatter1.sub("<URL>", content)
content = self.content_repatter2.sub("<EMAIL>", content)
content = self.content_repatter3.sub("<TEL>", content)
content = self.content_repatter4.sub("<DATE>", content)
content = self.content_repatter5.sub("<DATE>", content)
content = self.content_repatter6.sub("<PRICE>", content)
content = content.translate(self.content_trans1)
while "<BLOCK><BLOCK>" in content:
content = content.replace("<BLOCK><BLOCK>", "<BLOCK>")
return content
# Copied from tokenization_gpt_neox_japanese.SubWordJapaneseTokenizer.tokenize
def tokenize(self, text, clean=False):
text = text.replace(" ", "<SP>")
text = text.replace(" ", "<SP>")
text = text.replace("\r\n", "<BR>")
text = text.replace("\n", "<BR>")
text = text.replace("\r", "<BR>")
text = text.replace("\t", "<TAB>")
text = text.replace("—", "ー")
text = text.replace("−", "ー")
for k, v in self.emoji["emoji"].items():
if k in text:
text = text.replace(k, v)
if clean:
text = self.clean_text(text)
def check_simbol(x):
e = x.encode()
if len(x) == 1 and len(e) == 2:
c = (int(e[0]) << 8) + int(e[1])
if (
(c >= 0xC2A1 and c <= 0xC2BF)
or (c >= 0xC780 and c <= 0xC783)
or (c >= 0xCAB9 and c <= 0xCBBF)
or (c >= 0xCC80 and c <= 0xCDA2)
):
return True
return False
def checku2e(x):
e = x.encode()
if len(x) == 1 and len(e) == 3:
c = (int(e[0]) << 16) + (int(e[1]) << 8) + int(e[2])
if c >= 0xE28080 and c <= 0xE2B07F:
return True
return False
pos = 0
result = []
while pos < len(text):
end = min(len(text), pos + self.maxlen + 1) if text[pos] == "<" else pos + 3
candidates = [] # (token_id, token, pos)
for e in range(end, pos, -1):
wd = text[pos:e]
if wd in self.vocab:
if wd[0] == "<" and len(wd) > 2:
candidates = [(self.vocab[wd], wd, e)]
break
else:
candidates.append((self.vocab[wd], wd, e))
if len(candidates) > 0:
# the smallest token_id is adopted
_, wd, e = sorted(candidates, key=lambda x: x[0])[0]
result.append(wd)
pos = e
else:
end = pos + 1
wd = text[pos:end]
if check_simbol(wd):
result.append("<KIGOU>")
elif checku2e(wd):
result.append("<U2000U2BFF>")
else:
for i in wd.encode("utf-8"):
result.append("<|byte%d|>" % i)
pos = end
return result
def convert_id_to_token(self, index):
return self.ids_to_tokens[index][0]
| transformers/src/transformers/models/gptsan_japanese/tokenization_gptsan_japanese.py/0 | {
"file_path": "transformers/src/transformers/models/gptsan_japanese/tokenization_gptsan_japanese.py",
"repo_id": "transformers",
"token_count": 11883
} | 304 |
# coding=utf-8
# Copyright 2021 The HuggingFace Inc. team.
#
# Licensed under the Apache License, Version 2.0 (the "License");
# you may not use this file except in compliance with the License.
# You may obtain a copy of the License at
#
# http://www.apache.org/licenses/LICENSE-2.0
#
# Unless required by applicable law or agreed to in writing, software
# distributed under the License is distributed on an "AS IS" BASIS,
# WITHOUT WARRANTIES OR CONDITIONS OF ANY KIND, either express or implied.
# See the License for the specific language governing permissions and
# limitations under the License.
"""Convert Hubert checkpoint."""
import argparse
import torch
from s3prl.hub import distilhubert
from transformers import HubertConfig, HubertModel, Wav2Vec2FeatureExtractor, logging
logging.set_verbosity_info()
logger = logging.get_logger(__name__)
MAPPING = {
"post_extract_proj": "feature_projection.projection",
"encoder.pos_conv.0": "encoder.pos_conv_embed.conv",
"self_attn.k_proj": "encoder.layers.*.attention.k_proj",
"self_attn.v_proj": "encoder.layers.*.attention.v_proj",
"self_attn.q_proj": "encoder.layers.*.attention.q_proj",
"self_attn.out_proj": "encoder.layers.*.attention.out_proj",
"self_attn_layer_norm": "encoder.layers.*.layer_norm",
"fc1": "encoder.layers.*.feed_forward.intermediate_dense",
"fc2": "encoder.layers.*.feed_forward.output_dense",
"final_layer_norm": "encoder.layers.*.final_layer_norm",
"encoder.layer_norm": "encoder.layer_norm",
"mask_emb": "masked_spec_embed",
}
def set_recursively(hf_pointer, key, value, full_name, weight_type):
for attribute in key.split("."):
hf_pointer = getattr(hf_pointer, attribute)
if weight_type is not None:
hf_shape = getattr(hf_pointer, weight_type).shape
else:
hf_shape = hf_pointer.shape
assert hf_shape == value.shape, (
f"Shape of hf {key + '.' + weight_type if weight_type is not None else ''} is {hf_shape}, but should be"
f" {value.shape} for {full_name}"
)
if weight_type == "weight":
hf_pointer.weight.data = value
elif weight_type == "weight_g":
hf_pointer.weight_g.data = value
elif weight_type == "weight_v":
hf_pointer.weight_v.data = value
elif weight_type == "bias":
hf_pointer.bias.data = value
else:
hf_pointer.data = value
logger.info(f"{key + '.' + weight_type if weight_type is not None else ''} was initialized from {full_name}.")
def recursively_load_weights(fairseq_model, hf_model):
unused_weights = []
fairseq_dict = fairseq_model.state_dict()
feature_extractor = hf_model.feature_extractor
for name, value in fairseq_dict.items():
is_used = False
if "conv_layers" in name:
load_conv_layer(
name,
value,
feature_extractor,
unused_weights,
hf_model.config.feat_extract_norm == "group",
)
is_used = True
else:
for key, mapped_key in MAPPING.items():
mapped_key = mapped_key
if key in name:
is_used = True
if "*" in mapped_key:
layer_index = name.split(key)[0].split(".")[-2]
mapped_key = mapped_key.replace("*", layer_index)
if "weight_g" in name:
weight_type = "weight_g"
elif "weight_v" in name:
weight_type = "weight_v"
elif "weight" in name:
weight_type = "weight"
elif "bias" in name:
weight_type = "bias"
else:
weight_type = None
set_recursively(hf_model, mapped_key, value, name, weight_type)
continue
if not is_used:
unused_weights.append(name)
logger.warning(f"Unused weights: {unused_weights}")
def load_conv_layer(full_name, value, feature_extractor, unused_weights, use_group_norm):
name = full_name.split("conv_layers.")[-1]
items = name.split(".")
layer_id = int(items[0])
type_id = int(items[1])
if type_id == 0:
if "bias" in name:
assert value.shape == feature_extractor.conv_layers[layer_id].conv.bias.data.shape, (
f"{full_name} has size {value.shape}, but"
f" {feature_extractor.conv_layers[layer_id].conv.bias.data.shape} was found."
)
feature_extractor.conv_layers[layer_id].conv.bias.data = value
logger.info(f"Feat extract conv layer {layer_id} was initialized from {full_name}.")
elif "weight" in name:
assert value.shape == feature_extractor.conv_layers[layer_id].conv.weight.data.shape, (
f"{full_name} has size {value.shape}, but"
f" {feature_extractor.conv_layers[layer_id].conv.weight.data.shape} was found."
)
feature_extractor.conv_layers[layer_id].conv.weight.data = value
logger.info(f"Feat extract conv layer {layer_id} was initialized from {full_name}.")
elif (type_id == 2 and not use_group_norm) or (type_id == 2 and layer_id == 0 and use_group_norm):
if "bias" in name:
assert value.shape == feature_extractor.conv_layers[layer_id].layer_norm.bias.data.shape, (
f"{full_name} has size {value.shape}, but {feature_extractor[layer_id].layer_norm.bias.data.shape} was"
" found."
)
feature_extractor.conv_layers[layer_id].layer_norm.bias.data = value
logger.info(f"Feat extract layer norm weight of layer {layer_id} was initialized from {full_name}.")
elif "weight" in name:
assert value.shape == feature_extractor.conv_layers[layer_id].layer_norm.weight.data.shape, (
f"{full_name} has size {value.shape}, but"
f" {feature_extractor[layer_id].layer_norm.weight.data.shape} was found."
)
feature_extractor.conv_layers[layer_id].layer_norm.weight.data = value
logger.info(f"Feat extract layer norm weight of layer {layer_id} was initialized from {full_name}.")
else:
unused_weights.append(full_name)
def convert_config(model):
config = HubertConfig()
fs_config = model.config
config.activation_dropout = fs_config.activation_dropout
config.apply_spec_augment = False
config.attention_dropout = fs_config.attention_dropout
config.conv_bias = False
conv_layers = eval(fs_config.extractor_conv_feature_layers)
config.conv_dim = [x[0] for x in conv_layers]
config.conv_kernel = [x[1] for x in conv_layers]
config.conv_stride = [x[2] for x in conv_layers]
config.feat_extract_activation = "gelu"
config.feat_extract_norm = "layer" if fs_config.extractor_mode == "layer_norm" else "group"
config.feat_proj_layer_norm = False
config.feat_proj_dropout = 0.0
config.final_dropout = 0.0
config.hidden_act = fs_config.activation_fn
config.hidden_dropout = fs_config.dropout
config.hidden_size = fs_config.encoder_embed_dim
config.initializer_range = 0.02
config.intermediate_size = fs_config.encoder_ffn_embed_dim
config.layer_norm_eps = 1e-5
config.layerdrop = 0.0
config.num_attention_heads = fs_config.encoder_attention_heads
config.num_conv_pos_embedding_groups = fs_config.conv_pos_groups
config.num_conv_pos_embeddings = fs_config.conv_pos
config.num_feat_extract_layers = len(conv_layers)
config.num_hidden_layers = fs_config.encoder_layers
return config
@torch.no_grad()
def convert_hubert_checkpoint(pytorch_dump_folder_path, config_path=None):
"""
Copy/paste/tweak model's weights to transformers design.
"""
model = distilhubert().model.model
if config_path is not None:
config = HubertConfig.from_pretrained(config_path)
else:
config = convert_config(model)
model = model.eval()
feature_extractor = Wav2Vec2FeatureExtractor(
feature_size=1,
sampling_rate=16000,
padding_value=0,
do_normalize=False,
return_attention_mask=False,
)
hf_model = HubertModel(config)
recursively_load_weights(model, hf_model)
feature_extractor.save_pretrained(pytorch_dump_folder_path)
hf_model.save_pretrained(pytorch_dump_folder_path)
if __name__ == "__main__":
parser = argparse.ArgumentParser()
parser.add_argument("--pytorch_dump_folder_path", default=None, type=str, help="Path to the output PyTorch model.")
parser.add_argument("--config_path", default=None, type=str, help="Path to hf config.json of model to convert")
args = parser.parse_args()
convert_hubert_checkpoint(args.pytorch_dump_folder_path, args.config_path)
| transformers/src/transformers/models/hubert/convert_distilhubert_original_s3prl_checkpoint_to_pytorch.py/0 | {
"file_path": "transformers/src/transformers/models/hubert/convert_distilhubert_original_s3prl_checkpoint_to_pytorch.py",
"repo_id": "transformers",
"token_count": 3907
} | 305 |
# Copyright 2020 The HuggingFace Team. All rights reserved.
#
# Licensed under the Apache License, Version 2.0 (the "License");
# you may not use this file except in compliance with the License.
# You may obtain a copy of the License at
#
# http://www.apache.org/licenses/LICENSE-2.0
#
# Unless required by applicable law or agreed to in writing, software
# distributed under the License is distributed on an "AS IS" BASIS,
# WITHOUT WARRANTIES OR CONDITIONS OF ANY KIND, either express or implied.
# See the License for the specific language governing permissions and
# limitations under the License.
from typing import TYPE_CHECKING
from ...utils import OptionalDependencyNotAvailable, _LazyModule, is_torch_available, is_vision_available
_import_structure = {
"configuration_imagegpt": ["IMAGEGPT_PRETRAINED_CONFIG_ARCHIVE_MAP", "ImageGPTConfig", "ImageGPTOnnxConfig"]
}
try:
if not is_vision_available():
raise OptionalDependencyNotAvailable()
except OptionalDependencyNotAvailable:
pass
else:
_import_structure["feature_extraction_imagegpt"] = ["ImageGPTFeatureExtractor"]
_import_structure["image_processing_imagegpt"] = ["ImageGPTImageProcessor"]
try:
if not is_torch_available():
raise OptionalDependencyNotAvailable()
except OptionalDependencyNotAvailable:
pass
else:
_import_structure["modeling_imagegpt"] = [
"IMAGEGPT_PRETRAINED_MODEL_ARCHIVE_LIST",
"ImageGPTForCausalImageModeling",
"ImageGPTForImageClassification",
"ImageGPTModel",
"ImageGPTPreTrainedModel",
"load_tf_weights_in_imagegpt",
]
if TYPE_CHECKING:
from .configuration_imagegpt import IMAGEGPT_PRETRAINED_CONFIG_ARCHIVE_MAP, ImageGPTConfig, ImageGPTOnnxConfig
try:
if not is_vision_available():
raise OptionalDependencyNotAvailable()
except OptionalDependencyNotAvailable:
pass
else:
from .feature_extraction_imagegpt import ImageGPTFeatureExtractor
from .image_processing_imagegpt import ImageGPTImageProcessor
try:
if not is_torch_available():
raise OptionalDependencyNotAvailable()
except OptionalDependencyNotAvailable:
pass
else:
from .modeling_imagegpt import (
IMAGEGPT_PRETRAINED_MODEL_ARCHIVE_LIST,
ImageGPTForCausalImageModeling,
ImageGPTForImageClassification,
ImageGPTModel,
ImageGPTPreTrainedModel,
load_tf_weights_in_imagegpt,
)
else:
import sys
sys.modules[__name__] = _LazyModule(__name__, globals()["__file__"], _import_structure, module_spec=__spec__)
| transformers/src/transformers/models/imagegpt/__init__.py/0 | {
"file_path": "transformers/src/transformers/models/imagegpt/__init__.py",
"repo_id": "transformers",
"token_count": 1004
} | 306 |
# coding=utf-8
# Copyright 2022 The HuggingFace Inc. team.
#
# Licensed under the Apache License, Version 2.0 (the "License");
# you may not use this file except in compliance with the License.
# You may obtain a copy of the License at
#
# http://www.apache.org/licenses/LICENSE-2.0
#
# Unless required by applicable law or agreed to in writing, software
# distributed under the License is distributed on an "AS IS" BASIS,
# WITHOUT WARRANTIES OR CONDITIONS OF ANY KIND, either express or implied.
# See the License for the specific language governing permissions and
# limitations under the License.
"""Convert Jukebox checkpoints"""
import argparse
import json
import os
from pathlib import Path
import requests
import torch
from transformers import JukeboxConfig, JukeboxModel
from transformers.utils import logging
logging.set_verbosity_info()
logger = logging.get_logger(__name__)
PREFIX = "https://openaipublic.azureedge.net/jukebox/models/"
MODEL_MAPPING = {
"jukebox-1b-lyrics": [
"5b/vqvae.pth.tar",
"5b/prior_level_0.pth.tar",
"5b/prior_level_1.pth.tar",
"1b_lyrics/prior_level_2.pth.tar",
],
"jukebox-5b-lyrics": [
"5b/vqvae.pth.tar",
"5b/prior_level_0.pth.tar",
"5b/prior_level_1.pth.tar",
"5b_lyrics/prior_level_2.pth.tar",
],
}
def replace_key(key):
if key.endswith(".model.1.bias") and len(key.split(".")) > 10:
key = key.replace(".model.1.bias", ".conv1d_1.bias")
elif key.endswith(".model.1.weight") and len(key.split(".")) > 10:
key = key.replace(".model.1.weight", ".conv1d_1.weight")
elif key.endswith(".model.3.bias") and len(key.split(".")) > 10:
key = key.replace(".model.3.bias", ".conv1d_2.bias")
elif key.endswith(".model.3.weight") and len(key.split(".")) > 10:
key = key.replace(".model.3.weight", ".conv1d_2.weight")
if "conditioner_blocks.0." in key:
key = key.replace("conditioner_blocks.0", "conditioner_blocks")
if "prime_prior" in key:
key = key.replace("prime_prior", "encoder")
if ".emb." in key and "total" not in key and "absolute" not in key and "relative" not in key:
key = key.replace(".emb.", ".")
if key.endswith("k"): # replace vqvae.X.k with vqvae.X.codebook
return key.replace(".k", ".codebook")
if "y_emb." in key:
return key.replace("y_emb.", "metadata_embedding.")
if "x_emb.emb." in key:
key = key.replace("0.x_emb.emb", "embed_tokens")
if "prime_state_ln" in key:
return key.replace("prime_state_ln", "encoder.final_layer_norm")
if ".ln" in key:
return key.replace(".ln", ".layer_norm")
if "_ln" in key:
return key.replace("_ln", "_layer_norm")
if "prime_state_proj" in key:
return key.replace("prime_state_proj", "encoder.proj_in")
if "prime_x_out" in key:
return key.replace("prime_x_out", "encoder.lm_head")
if "prior.x_out" in key:
return key.replace("x_out", "fc_proj_out")
if "x_emb" in key:
return key.replace("x_emb", "embed_tokens")
return key
def fix_jukebox_keys(state_dict, model_state_dict, key_prefix, mapping):
new_dict = {}
import re
re_encoder_block_conv_in = re.compile(r"encoders.(\d*).level_blocks.(\d*).model.(\d*).(\d).(bias|weight)")
re_encoder_block_resnet = re.compile(
r"encoders.(\d*).level_blocks.(\d*).model.(\d*).(\d).model.(\d*).model.(\d*).(bias|weight)"
)
re_encoder_block_proj_out = re.compile(r"encoders.(\d*).level_blocks.(\d*).model.(\d*).(bias|weight)")
re_decoder_block_conv_out = re.compile(r"decoders.(\d*).level_blocks.(\d*).model.(\d*).(\d).(bias|weight)")
re_decoder_block_resnet = re.compile(
r"decoders.(\d*).level_blocks.(\d*).model.(\d*).(\d).model.(\d*).model.(\d*).(bias|weight)"
)
re_decoder_block_proj_in = re.compile(r"decoders.(\d*).level_blocks.(\d*).model.(\d*).(bias|weight)")
re_prior_cond_conv_out = re.compile(r"conditioner_blocks.(\d*).cond.model.(\d*).(\d).(bias|weight)")
re_prior_cond_resnet = re.compile(
r"conditioner_blocks.(\d*).cond.model.(\d*).(\d).model.(\d*).model.(\d*).(bias|weight)"
)
re_prior_cond_proj_in = re.compile(r"conditioner_blocks.(\d*).cond.model.(\d*).(bias|weight)")
for original_key, value in state_dict.items():
# rename vqvae.encoder keys
if re_encoder_block_conv_in.fullmatch(original_key):
regex_match = re_encoder_block_conv_in.match(original_key)
groups = regex_match.groups()
block_index = int(groups[2]) * 2 + int(groups[3])
re_new_key = f"encoders.{groups[0]}.level_blocks.{groups[1]}.downsample_block.{block_index}.{groups[-1]}"
key = re_encoder_block_conv_in.sub(re_new_key, original_key)
elif re_encoder_block_resnet.fullmatch(original_key):
regex_match = re_encoder_block_resnet.match(original_key)
groups = regex_match.groups()
block_index = int(groups[2]) * 2 + int(groups[3])
conv_index = {"1": 1, "3": 2}[groups[-2]]
prefix = f"encoders.{groups[0]}.level_blocks.{groups[1]}.downsample_block.{block_index}."
resnet_block = f"resnet_block.{groups[-3]}.conv1d_{conv_index}.{groups[-1]}"
re_new_key = prefix + resnet_block
key = re_encoder_block_resnet.sub(re_new_key, original_key)
elif re_encoder_block_proj_out.fullmatch(original_key):
regex_match = re_encoder_block_proj_out.match(original_key)
groups = regex_match.groups()
re_new_key = f"encoders.{groups[0]}.level_blocks.{groups[1]}.proj_out.{groups[-1]}"
key = re_encoder_block_proj_out.sub(re_new_key, original_key)
# rename vqvae.decoder keys
elif re_decoder_block_conv_out.fullmatch(original_key):
regex_match = re_decoder_block_conv_out.match(original_key)
groups = regex_match.groups()
block_index = int(groups[2]) * 2 + int(groups[3]) - 2
re_new_key = f"decoders.{groups[0]}.level_blocks.{groups[1]}.upsample_block.{block_index}.{groups[-1]}"
key = re_decoder_block_conv_out.sub(re_new_key, original_key)
elif re_decoder_block_resnet.fullmatch(original_key):
regex_match = re_decoder_block_resnet.match(original_key)
groups = regex_match.groups()
block_index = int(groups[2]) * 2 + int(groups[3]) - 2
conv_index = {"1": 1, "3": 2}[groups[-2]]
prefix = f"decoders.{groups[0]}.level_blocks.{groups[1]}.upsample_block.{block_index}."
resnet_block = f"resnet_block.{groups[-3]}.conv1d_{conv_index}.{groups[-1]}"
re_new_key = prefix + resnet_block
key = re_decoder_block_resnet.sub(re_new_key, original_key)
elif re_decoder_block_proj_in.fullmatch(original_key):
regex_match = re_decoder_block_proj_in.match(original_key)
groups = regex_match.groups()
re_new_key = f"decoders.{groups[0]}.level_blocks.{groups[1]}.proj_in.{groups[-1]}"
key = re_decoder_block_proj_in.sub(re_new_key, original_key)
# rename prior cond.model to upsampler.upsample_block and resnet
elif re_prior_cond_conv_out.fullmatch(original_key):
regex_match = re_prior_cond_conv_out.match(original_key)
groups = regex_match.groups()
block_index = int(groups[1]) * 2 + int(groups[2]) - 2
re_new_key = f"conditioner_blocks.upsampler.upsample_block.{block_index}.{groups[-1]}"
key = re_prior_cond_conv_out.sub(re_new_key, original_key)
elif re_prior_cond_resnet.fullmatch(original_key):
regex_match = re_prior_cond_resnet.match(original_key)
groups = regex_match.groups()
block_index = int(groups[1]) * 2 + int(groups[2]) - 2
conv_index = {"1": 1, "3": 2}[groups[-2]]
prefix = f"conditioner_blocks.upsampler.upsample_block.{block_index}."
resnet_block = f"resnet_block.{groups[-3]}.conv1d_{conv_index}.{groups[-1]}"
re_new_key = prefix + resnet_block
key = re_prior_cond_resnet.sub(re_new_key, original_key)
elif re_prior_cond_proj_in.fullmatch(original_key):
regex_match = re_prior_cond_proj_in.match(original_key)
groups = regex_match.groups()
re_new_key = f"conditioner_blocks.upsampler.proj_in.{groups[-1]}"
key = re_prior_cond_proj_in.sub(re_new_key, original_key)
# keep original key
else:
key = original_key
key = replace_key(key)
if f"{key_prefix}.{key}" not in model_state_dict or key is None:
print(f"failed converting {original_key} to {key}, does not match")
# handle missmatched shape
elif value.shape != model_state_dict[f"{key_prefix}.{key}"].shape:
val = model_state_dict[f"{key_prefix}.{key}"]
print(f"{original_key}-> {key} : \nshape {val.shape} and { value.shape}, do not match")
key = original_key
mapping[key] = original_key
new_dict[key] = value
return new_dict
@torch.no_grad()
def convert_openai_checkpoint(model_name=None, pytorch_dump_folder_path=None):
"""
Copy/paste/tweak model's weights to our Jukebox structure.
"""
for file in MODEL_MAPPING[model_name]:
if not os.path.isfile(f"{pytorch_dump_folder_path}/{file.split('/')[-1]}"):
r = requests.get(f"{PREFIX}{file}", allow_redirects=True)
os.makedirs(f"{pytorch_dump_folder_path}/", exist_ok=True)
open(f"{pytorch_dump_folder_path}/{file.split('/')[-1]}", "wb").write(r.content)
model_to_convert = MODEL_MAPPING[model_name.split("/")[-1]]
config = JukeboxConfig.from_pretrained(model_name)
model = JukeboxModel(config)
weight_dict = []
mapping = {}
for i, dict_name in enumerate(model_to_convert):
old_dic = torch.load(f"{pytorch_dump_folder_path}/{dict_name.split('/')[-1]}")["model"]
new_dic = {}
for k in old_dic.keys():
if k.endswith(".b"):
new_dic[k.replace("b", "bias")] = old_dic[k]
elif k.endswith(".w"):
new_dic[k.replace("w", "weight")] = old_dic[k]
elif "level_2" not in dict_name and "cond.model." in k:
new_dic[k.replace(".blocks.", ".model.")] = old_dic[k]
else:
new_dic[k] = old_dic[k]
key_prefix = "vqvae" if i == 0 else f"priors.{3 - i}"
new_dic = fix_jukebox_keys(new_dic, model.state_dict(), key_prefix, mapping)
weight_dict.append(new_dic)
vqvae_state_dict = weight_dict.pop(0)
model.vqvae.load_state_dict(vqvae_state_dict)
for i in range(len(weight_dict)):
model.priors[i].load_state_dict(weight_dict[2 - i])
Path(pytorch_dump_folder_path).mkdir(exist_ok=True)
with open(f"{pytorch_dump_folder_path}/mapping.json", "w") as txtfile:
json.dump(mapping, txtfile)
print(f"Saving model {model_name} to {pytorch_dump_folder_path}")
model.save_pretrained(pytorch_dump_folder_path)
return weight_dict
if __name__ == "__main__":
parser = argparse.ArgumentParser()
# Required parameters
parser.add_argument(
"--model_name",
default="jukebox-5b-lyrics",
type=str,
help="Name of the model you'd like to convert.",
)
parser.add_argument(
"--pytorch_dump_folder_path",
default="jukebox-5b-lyrics-converted",
type=str,
help="Path to the output PyTorch model directory.",
)
args = parser.parse_args()
convert_openai_checkpoint(args.model_name, args.pytorch_dump_folder_path)
| transformers/src/transformers/models/jukebox/convert_jukebox.py/0 | {
"file_path": "transformers/src/transformers/models/jukebox/convert_jukebox.py",
"repo_id": "transformers",
"token_count": 5498
} | 307 |
# coding=utf-8
# Copyright 2022 The HuggingFace Inc. team. All rights reserved.
#
# Licensed under the Apache License, Version 2.0 (the "License");
# you may not use this file except in compliance with the License.
# You may obtain a copy of the License at
#
# http://www.apache.org/licenses/LICENSE-2.0
#
# Unless required by applicable law or agreed to in writing, software
# distributed under the License is distributed on an "AS IS" BASIS,
# WITHOUT WARRANTIES OR CONDITIONS OF ANY KIND, either express or implied.
# See the License for the specific language governing permissions and
# limitations under the License.
""" LiLT configuration"""
from ...configuration_utils import PretrainedConfig
from ...utils import logging
logger = logging.get_logger(__name__)
LILT_PRETRAINED_CONFIG_ARCHIVE_MAP = {
"SCUT-DLVCLab/lilt-roberta-en-base": (
"https://huggingface.co/SCUT-DLVCLab/lilt-roberta-en-base/resolve/main/config.json"
),
}
class LiltConfig(PretrainedConfig):
r"""
This is the configuration class to store the configuration of a [`LiltModel`]. It is used to instantiate a LiLT
model according to the specified arguments, defining the model architecture. Instantiating a configuration with the
defaults will yield a similar configuration to that of the LiLT
[SCUT-DLVCLab/lilt-roberta-en-base](https://huggingface.co/SCUT-DLVCLab/lilt-roberta-en-base) architecture.
Configuration objects inherit from [`PretrainedConfig`] and can be used to control the model outputs. Read the
documentation from [`PretrainedConfig`] for more information.
Args:
vocab_size (`int`, *optional*, defaults to 30522):
Vocabulary size of the LiLT model. Defines the number of different tokens that can be represented by the
`inputs_ids` passed when calling [`LiltModel`].
hidden_size (`int`, *optional*, defaults to 768):
Dimensionality of the encoder layers and the pooler layer. Should be a multiple of 24.
num_hidden_layers (`int`, *optional*, defaults to 12):
Number of hidden layers in the Transformer encoder.
num_attention_heads (`int`, *optional*, defaults to 12):
Number of attention heads for each attention layer in the Transformer encoder.
intermediate_size (`int`, *optional*, defaults to 3072):
Dimensionality of the "intermediate" (often named feed-forward) layer in the Transformer encoder.
hidden_act (`str` or `Callable`, *optional*, defaults to `"gelu"`):
The non-linear activation function (function or string) in the encoder and pooler. If string, `"gelu"`,
`"relu"`, `"silu"` and `"gelu_new"` are supported.
hidden_dropout_prob (`float`, *optional*, defaults to 0.1):
The dropout probability for all fully connected layers in the embeddings, encoder, and pooler.
attention_probs_dropout_prob (`float`, *optional*, defaults to 0.1):
The dropout ratio for the attention probabilities.
max_position_embeddings (`int`, *optional*, defaults to 512):
The maximum sequence length that this model might ever be used with. Typically set this to something large
just in case (e.g., 512 or 1024 or 2048).
type_vocab_size (`int`, *optional*, defaults to 2):
The vocabulary size of the `token_type_ids` passed when calling [`LiltModel`].
initializer_range (`float`, *optional*, defaults to 0.02):
The standard deviation of the truncated_normal_initializer for initializing all weight matrices.
layer_norm_eps (`float`, *optional*, defaults to 1e-12):
The epsilon used by the layer normalization layers.
position_embedding_type (`str`, *optional*, defaults to `"absolute"`):
Type of position embedding. Choose one of `"absolute"`, `"relative_key"`, `"relative_key_query"`. For
positional embeddings use `"absolute"`. For more information on `"relative_key"`, please refer to
[Self-Attention with Relative Position Representations (Shaw et al.)](https://arxiv.org/abs/1803.02155).
For more information on `"relative_key_query"`, please refer to *Method 4* in [Improve Transformer Models
with Better Relative Position Embeddings (Huang et al.)](https://arxiv.org/abs/2009.13658).
classifier_dropout (`float`, *optional*):
The dropout ratio for the classification head.
channel_shrink_ratio (`int`, *optional*, defaults to 4):
The shrink ratio compared to the `hidden_size` for the channel dimension of the layout embeddings.
max_2d_position_embeddings (`int`, *optional*, defaults to 1024):
The maximum value that the 2D position embedding might ever be used with. Typically set this to something
large just in case (e.g., 1024).
Examples:
```python
>>> from transformers import LiltConfig, LiltModel
>>> # Initializing a LiLT SCUT-DLVCLab/lilt-roberta-en-base style configuration
>>> configuration = LiltConfig()
>>> # Randomly initializing a model from the SCUT-DLVCLab/lilt-roberta-en-base style configuration
>>> model = LiltModel(configuration)
>>> # Accessing the model configuration
>>> configuration = model.config
```"""
model_type = "lilt"
def __init__(
self,
vocab_size=30522,
hidden_size=768,
num_hidden_layers=12,
num_attention_heads=12,
intermediate_size=3072,
hidden_act="gelu",
hidden_dropout_prob=0.1,
attention_probs_dropout_prob=0.1,
max_position_embeddings=512,
type_vocab_size=2,
initializer_range=0.02,
layer_norm_eps=1e-12,
pad_token_id=0,
position_embedding_type="absolute",
classifier_dropout=None,
channel_shrink_ratio=4,
max_2d_position_embeddings=1024,
**kwargs,
):
super().__init__(pad_token_id=pad_token_id, **kwargs)
self.vocab_size = vocab_size
self.hidden_size = hidden_size
self.num_hidden_layers = num_hidden_layers
self.num_attention_heads = num_attention_heads
self.hidden_act = hidden_act
self.intermediate_size = intermediate_size
self.hidden_dropout_prob = hidden_dropout_prob
self.attention_probs_dropout_prob = attention_probs_dropout_prob
self.max_position_embeddings = max_position_embeddings
self.type_vocab_size = type_vocab_size
self.initializer_range = initializer_range
self.layer_norm_eps = layer_norm_eps
self.position_embedding_type = position_embedding_type
self.classifier_dropout = classifier_dropout
self.channel_shrink_ratio = channel_shrink_ratio
self.max_2d_position_embeddings = max_2d_position_embeddings
| transformers/src/transformers/models/lilt/configuration_lilt.py/0 | {
"file_path": "transformers/src/transformers/models/lilt/configuration_lilt.py",
"repo_id": "transformers",
"token_count": 2571
} | 308 |
# coding=utf-8
# Copyright 2018 The HuggingFace Inc. team.
#
# Licensed under the Apache License, Version 2.0 (the "License");
# you may not use this file except in compliance with the License.
# You may obtain a copy of the License at
#
# http://www.apache.org/licenses/LICENSE-2.0
#
# Unless required by applicable law or agreed to in writing, software
# distributed under the License is distributed on an "AS IS" BASIS,
# WITHOUT WARRANTIES OR CONDITIONS OF ANY KIND, either express or implied.
# See the License for the specific language governing permissions and
# limitations under the License.
"""Convert RoBERTa checkpoint."""
import argparse
import pytorch_lightning as pl
import torch
from torch import nn
from transformers import LongformerForQuestionAnswering, LongformerModel
class LightningModel(pl.LightningModule):
def __init__(self, model):
super().__init__()
self.model = model
self.num_labels = 2
self.qa_outputs = nn.Linear(self.model.config.hidden_size, self.num_labels)
# implement only because lightning requires to do so
def forward(self):
pass
def convert_longformer_qa_checkpoint_to_pytorch(
longformer_model: str, longformer_question_answering_ckpt_path: str, pytorch_dump_folder_path: str
):
# load longformer model from model identifier
longformer = LongformerModel.from_pretrained(longformer_model)
lightning_model = LightningModel(longformer)
ckpt = torch.load(longformer_question_answering_ckpt_path, map_location=torch.device("cpu"))
lightning_model.load_state_dict(ckpt["state_dict"])
# init longformer question answering model
longformer_for_qa = LongformerForQuestionAnswering.from_pretrained(longformer_model)
# transfer weights
longformer_for_qa.longformer.load_state_dict(lightning_model.model.state_dict())
longformer_for_qa.qa_outputs.load_state_dict(lightning_model.qa_outputs.state_dict())
longformer_for_qa.eval()
# save model
longformer_for_qa.save_pretrained(pytorch_dump_folder_path)
print(f"Conversion successful. Model saved under {pytorch_dump_folder_path}")
if __name__ == "__main__":
parser = argparse.ArgumentParser()
# Required parameters
parser.add_argument(
"--longformer_model",
default=None,
type=str,
required=True,
help="model identifier of longformer. Should be either `longformer-base-4096` or `longformer-large-4096`.",
)
parser.add_argument(
"--longformer_question_answering_ckpt_path",
default=None,
type=str,
required=True,
help="Path the official PyTorch Lightning Checkpoint.",
)
parser.add_argument(
"--pytorch_dump_folder_path", default=None, type=str, required=True, help="Path to the output PyTorch model."
)
args = parser.parse_args()
convert_longformer_qa_checkpoint_to_pytorch(
args.longformer_model, args.longformer_question_answering_ckpt_path, args.pytorch_dump_folder_path
)
| transformers/src/transformers/models/longformer/convert_longformer_original_pytorch_lightning_to_pytorch.py/0 | {
"file_path": "transformers/src/transformers/models/longformer/convert_longformer_original_pytorch_lightning_to_pytorch.py",
"repo_id": "transformers",
"token_count": 1071
} | 309 |
# coding=utf-8
# Copyright 2018, Hao Tan, Mohit Bansal
#
# Licensed under the Apache License, Version 2.0 (the "License");
# you may not use this file except in compliance with the License.
# You may obtain a copy of the License at
#
# http://www.apache.org/licenses/LICENSE-2.0
#
# Unless required by applicable law or agreed to in writing, software
# distributed under the License is distributed on an "AS IS" BASIS,
# WITHOUT WARRANTIES OR CONDITIONS OF ANY KIND, either express or implied.
# See the License for the specific language governing permissions and
# limitations under the License.
""" LXMERT model configuration"""
from ...configuration_utils import PretrainedConfig
from ...utils import logging
logger = logging.get_logger(__name__)
LXMERT_PRETRAINED_CONFIG_ARCHIVE_MAP = {
"unc-nlp/lxmert-base-uncased": "https://huggingface.co/unc-nlp/lxmert-base-uncased/resolve/main/config.json",
}
class LxmertConfig(PretrainedConfig):
r"""
This is the configuration class to store the configuration of a [`LxmertModel`] or a [`TFLxmertModel`]. It is used
to instantiate a LXMERT model according to the specified arguments, defining the model architecture. Instantiating
a configuration with the defaults will yield a similar configuration to that of the Lxmert
[unc-nlp/lxmert-base-uncased](https://huggingface.co/unc-nlp/lxmert-base-uncased) architecture.
Configuration objects inherit from [`PretrainedConfig`] and can be used to control the model outputs. Read the
documentation from [`PretrainedConfig`] for more information.
Args:
vocab_size (`int`, *optional*, defaults to 30522):
Vocabulary size of the LXMERT model. Defines the number of different tokens that can be represented by the
`inputs_ids` passed when calling [`LxmertModel`] or [`TFLxmertModel`].
hidden_size (`int`, *optional*, defaults to 768):
Dimensionality of the encoder layers and the pooler layer.
num_attention_heads (`int`, *optional*, defaults to 12):
Number of attention heads for each attention layer in the Transformer encoder.
num_qa_labels (`int`, *optional*, defaults to 9500):
This represents the total number of different question answering (QA) labels there are. If using more than
one dataset with QA, the user will need to account for the total number of labels that all of the datasets
have in total.
num_object_labels (`int`, *optional*, defaults to 1600):
This represents the total number of semantically unique objects that lxmert will be able to classify a
pooled-object feature as belonging too.
num_attr_labels (`int`, *optional*, defaults to 400):
This represents the total number of semantically unique attributes that lxmert will be able to classify a
pooled-object feature as possessing.
intermediate_size (`int`, *optional*, defaults to 3072):
Dimensionality of the "intermediate" (often named feed-forward) layer in the Transformer encoder.
hidden_act (`str` or `Callable`, *optional*, defaults to `"gelu"`):
The non-linear activation function (function or string) in the encoder and pooler. If string, `"gelu"`,
`"relu"`, `"silu"` and `"gelu_new"` are supported.
hidden_dropout_prob (`float`, *optional*, defaults to 0.1):
The dropout probability for all fully connected layers in the embeddings, encoder, and pooler.
attention_probs_dropout_prob (`float`, *optional*, defaults to 0.1):
The dropout ratio for the attention probabilities.
max_position_embeddings (`int`, *optional*, defaults to 512):
The maximum sequence length that this model might ever be used with. Typically set this to something large
just in case (e.g., 512 or 1024 or 2048).
type_vocab_size (`int`, *optional*, defaults to 2):
The vocabulary size of the *token_type_ids* passed into [`BertModel`].
initializer_range (`float`, *optional*, defaults to 0.02):
The standard deviation of the truncated_normal_initializer for initializing all weight matrices.
layer_norm_eps (`float`, *optional*, defaults to 1e-12):
The epsilon used by the layer normalization layers.
l_layers (`int`, *optional*, defaults to 9):
Number of hidden layers in the Transformer language encoder.
x_layers (`int`, *optional*, defaults to 5):
Number of hidden layers in the Transformer cross modality encoder.
r_layers (`int`, *optional*, defaults to 5):
Number of hidden layers in the Transformer visual encoder.
visual_feat_dim (`int`, *optional*, defaults to 2048):
This represents the last dimension of the pooled-object features used as input for the model, representing
the size of each object feature itself.
visual_pos_dim (`int`, *optional*, defaults to 4):
This represents the number of spacial features that are mixed into the visual features. The default is set
to 4 because most commonly this will represent the location of a bounding box. i.e., (x, y, width, height)
visual_loss_normalizer (`float`, *optional*, defaults to 6.67):
This represents the scaling factor in which each visual loss is multiplied by if during pretraining, one
decided to train with multiple vision-based loss objectives.
task_matched (`bool`, *optional*, defaults to `True`):
This task is used for sentence-image matching. If the sentence correctly describes the image the label will
be 1. If the sentence does not correctly describe the image, the label will be 0.
task_mask_lm (`bool`, *optional*, defaults to `True`):
Whether or not to add masked language modeling (as used in pretraining models such as BERT) to the loss
objective.
task_obj_predict (`bool`, *optional*, defaults to `True`):
Whether or not to add object prediction, attribute prediction and feature regression to the loss objective.
task_qa (`bool`, *optional*, defaults to `True`):
Whether or not to add the question-answering loss to the objective
visual_obj_loss (`bool`, *optional*, defaults to `True`):
Whether or not to calculate the object-prediction loss objective
visual_attr_loss (`bool`, *optional*, defaults to `True`):
Whether or not to calculate the attribute-prediction loss objective
visual_feat_loss (`bool`, *optional*, defaults to `True`):
Whether or not to calculate the feature-regression loss objective
"""
model_type = "lxmert"
attribute_map = {}
def __init__(
self,
vocab_size=30522,
hidden_size=768,
num_attention_heads=12,
num_qa_labels=9500,
num_object_labels=1600,
num_attr_labels=400,
intermediate_size=3072,
hidden_act="gelu",
hidden_dropout_prob=0.1,
attention_probs_dropout_prob=0.1,
max_position_embeddings=512,
type_vocab_size=2,
initializer_range=0.02,
layer_norm_eps=1e-12,
l_layers=9,
x_layers=5,
r_layers=5,
visual_feat_dim=2048,
visual_pos_dim=4,
visual_loss_normalizer=6.67,
task_matched=True,
task_mask_lm=True,
task_obj_predict=True,
task_qa=True,
visual_obj_loss=True,
visual_attr_loss=True,
visual_feat_loss=True,
**kwargs,
):
self.vocab_size = vocab_size
self.hidden_size = hidden_size
self.num_attention_heads = num_attention_heads
self.hidden_act = hidden_act
self.intermediate_size = intermediate_size
self.hidden_dropout_prob = hidden_dropout_prob
self.attention_probs_dropout_prob = attention_probs_dropout_prob
self.max_position_embeddings = max_position_embeddings
self.type_vocab_size = type_vocab_size
self.initializer_range = initializer_range
self.layer_norm_eps = layer_norm_eps
self.num_qa_labels = num_qa_labels
self.num_object_labels = num_object_labels
self.num_attr_labels = num_attr_labels
self.l_layers = l_layers
self.x_layers = x_layers
self.r_layers = r_layers
self.visual_feat_dim = visual_feat_dim
self.visual_pos_dim = visual_pos_dim
self.visual_loss_normalizer = visual_loss_normalizer
self.task_matched = task_matched
self.task_mask_lm = task_mask_lm
self.task_obj_predict = task_obj_predict
self.task_qa = task_qa
self.visual_obj_loss = visual_obj_loss
self.visual_attr_loss = visual_attr_loss
self.visual_feat_loss = visual_feat_loss
self.num_hidden_layers = {"vision": r_layers, "cross_encoder": x_layers, "language": l_layers}
super().__init__(**kwargs)
| transformers/src/transformers/models/lxmert/configuration_lxmert.py/0 | {
"file_path": "transformers/src/transformers/models/lxmert/configuration_lxmert.py",
"repo_id": "transformers",
"token_count": 3447
} | 310 |
# coding=utf-8
# Copyright 2022 Meta Platforms, Inc.and The HuggingFace Inc. team. All rights reserved.
#
# Licensed under the Apache License, Version 2.0 (the "License");
# you may not use this file except in compliance with the License.
# You may obtain a copy of the License at
#
# http://www.apache.org/licenses/LICENSE-2.0
#
# Unless required by applicable law or agreed to in writing, software
# distributed under the License is distributed on an "AS IS" BASIS,
# WITHOUT WARRANTIES OR CONDITIONS OF ANY KIND, either express or implied.
# See the License for the specific language governing permissions and
# limitations under the License.
""" MaskFormer model configuration"""
from typing import Dict, Optional
from ...configuration_utils import PretrainedConfig
from ...utils import logging
from ..auto import CONFIG_MAPPING
from ..detr import DetrConfig
from ..swin import SwinConfig
MASKFORMER_PRETRAINED_CONFIG_ARCHIVE_MAP = {
"facebook/maskformer-swin-base-ade": (
"https://huggingface.co/facebook/maskformer-swin-base-ade/blob/main/config.json"
)
# See all MaskFormer models at https://huggingface.co/models?filter=maskformer
}
logger = logging.get_logger(__name__)
class MaskFormerConfig(PretrainedConfig):
r"""
This is the configuration class to store the configuration of a [`MaskFormerModel`]. It is used to instantiate a
MaskFormer model according to the specified arguments, defining the model architecture. Instantiating a
configuration with the defaults will yield a similar configuration to that of the MaskFormer
[facebook/maskformer-swin-base-ade](https://huggingface.co/facebook/maskformer-swin-base-ade) architecture trained
on [ADE20k-150](https://huggingface.co/datasets/scene_parse_150).
Configuration objects inherit from [`PretrainedConfig`] and can be used to control the model outputs. Read the
documentation from [`PretrainedConfig`] for more information.
Currently, MaskFormer only supports the [Swin Transformer](swin) as backbone.
Args:
mask_feature_size (`int`, *optional*, defaults to 256):
The masks' features size, this value will also be used to specify the Feature Pyramid Network features'
size.
no_object_weight (`float`, *optional*, defaults to 0.1):
Weight to apply to the null (no object) class.
use_auxiliary_loss(`bool`, *optional*, defaults to `False`):
If `True` [`MaskFormerForInstanceSegmentationOutput`] will contain the auxiliary losses computed using the
logits from each decoder's stage.
backbone_config (`Dict`, *optional*):
The configuration passed to the backbone, if unset, the configuration corresponding to
`swin-base-patch4-window12-384` will be used.
backbone (`str`, *optional*):
Name of backbone to use when `backbone_config` is `None`. If `use_pretrained_backbone` is `True`, this
will load the corresponding pretrained weights from the timm or transformers library. If `use_pretrained_backbone`
is `False`, this loads the backbone's config and uses that to initialize the backbone with random weights.
use_pretrained_backbone (`bool`, *optional*, `False`):
Whether to use pretrained weights for the backbone.
use_timm_backbone (`bool`, *optional*, `False`):
Whether to load `backbone` from the timm library. If `False`, the backbone is loaded from the transformers
library.
decoder_config (`Dict`, *optional*):
The configuration passed to the transformer decoder model, if unset the base config for `detr-resnet-50`
will be used.
init_std (`float`, *optional*, defaults to 0.02):
The standard deviation of the truncated_normal_initializer for initializing all weight matrices.
init_xavier_std (`float`, *optional*, defaults to 1):
The scaling factor used for the Xavier initialization gain in the HM Attention map module.
dice_weight (`float`, *optional*, defaults to 1.0):
The weight for the dice loss.
cross_entropy_weight (`float`, *optional*, defaults to 1.0):
The weight for the cross entropy loss.
mask_weight (`float`, *optional*, defaults to 20.0):
The weight for the mask loss.
output_auxiliary_logits (`bool`, *optional*):
Should the model output its `auxiliary_logits` or not.
Raises:
`ValueError`:
Raised if the backbone model type selected is not in `["swin"]` or the decoder model type selected is not
in `["detr"]`
Examples:
```python
>>> from transformers import MaskFormerConfig, MaskFormerModel
>>> # Initializing a MaskFormer facebook/maskformer-swin-base-ade configuration
>>> configuration = MaskFormerConfig()
>>> # Initializing a model (with random weights) from the facebook/maskformer-swin-base-ade style configuration
>>> model = MaskFormerModel(configuration)
>>> # Accessing the model configuration
>>> configuration = model.config
```
"""
model_type = "maskformer"
attribute_map = {"hidden_size": "mask_feature_size"}
backbones_supported = ["resnet", "swin"]
decoders_supported = ["detr"]
def __init__(
self,
fpn_feature_size: int = 256,
mask_feature_size: int = 256,
no_object_weight: float = 0.1,
use_auxiliary_loss: bool = False,
backbone_config: Optional[Dict] = None,
decoder_config: Optional[Dict] = None,
init_std: float = 0.02,
init_xavier_std: float = 1.0,
dice_weight: float = 1.0,
cross_entropy_weight: float = 1.0,
mask_weight: float = 20.0,
output_auxiliary_logits: Optional[bool] = None,
backbone: Optional[str] = None,
use_pretrained_backbone: bool = False,
use_timm_backbone: bool = False,
**kwargs,
):
if use_pretrained_backbone:
raise ValueError("Pretrained backbones are not supported yet.")
if backbone_config is not None and backbone is not None:
raise ValueError("You can't specify both `backbone` and `backbone_config`.")
if backbone_config is None and backbone is None:
# fall back to https://huggingface.co/microsoft/swin-base-patch4-window12-384-in22k
backbone_config = SwinConfig(
image_size=384,
in_channels=3,
patch_size=4,
embed_dim=128,
depths=[2, 2, 18, 2],
num_heads=[4, 8, 16, 32],
window_size=12,
drop_path_rate=0.3,
out_features=["stage1", "stage2", "stage3", "stage4"],
)
if isinstance(backbone_config, dict):
backbone_model_type = backbone_config.pop("model_type")
config_class = CONFIG_MAPPING[backbone_model_type]
backbone_config = config_class.from_dict(backbone_config)
# verify that the backbone is supported
if backbone_config is not None and backbone_config.model_type not in self.backbones_supported:
logger.warning_once(
f"Backbone {backbone_config.model_type} is not a supported model and may not be compatible with MaskFormer. "
f"Supported model types: {','.join(self.backbones_supported)}"
)
if decoder_config is None:
# fall back to https://huggingface.co/facebook/detr-resnet-50
decoder_config = DetrConfig()
else:
# verify that the decoder is supported
decoder_type = (
decoder_config.pop("model_type") if isinstance(decoder_config, dict) else decoder_config.model_type
)
if decoder_type not in self.decoders_supported:
raise ValueError(
f"Transformer Decoder {decoder_type} not supported, please use one of"
f" {','.join(self.decoders_supported)}"
)
if isinstance(decoder_config, dict):
config_class = CONFIG_MAPPING[decoder_type]
decoder_config = config_class.from_dict(decoder_config)
self.backbone_config = backbone_config
self.decoder_config = decoder_config
# main feature dimension for the model
self.fpn_feature_size = fpn_feature_size
self.mask_feature_size = mask_feature_size
# initializer
self.init_std = init_std
self.init_xavier_std = init_xavier_std
# Hungarian matcher && loss
self.cross_entropy_weight = cross_entropy_weight
self.dice_weight = dice_weight
self.mask_weight = mask_weight
self.use_auxiliary_loss = use_auxiliary_loss
self.no_object_weight = no_object_weight
self.output_auxiliary_logits = output_auxiliary_logits
self.num_attention_heads = self.decoder_config.encoder_attention_heads
self.num_hidden_layers = self.decoder_config.num_hidden_layers
self.backbone = backbone
self.use_pretrained_backbone = use_pretrained_backbone
self.use_timm_backbone = use_timm_backbone
super().__init__(**kwargs)
@classmethod
def from_backbone_and_decoder_configs(
cls, backbone_config: PretrainedConfig, decoder_config: PretrainedConfig, **kwargs
):
"""Instantiate a [`MaskFormerConfig`] (or a derived class) from a pre-trained backbone model configuration and DETR model
configuration.
Args:
backbone_config ([`PretrainedConfig`]):
The backbone configuration.
decoder_config ([`PretrainedConfig`]):
The transformer decoder configuration to use.
Returns:
[`MaskFormerConfig`]: An instance of a configuration object
"""
return cls(
backbone_config=backbone_config,
decoder_config=decoder_config,
**kwargs,
)
| transformers/src/transformers/models/maskformer/configuration_maskformer.py/0 | {
"file_path": "transformers/src/transformers/models/maskformer/configuration_maskformer.py",
"repo_id": "transformers",
"token_count": 3990
} | 311 |
# coding=utf-8
# Copyright 2020 The Facebook AI Research Team Authors and The HuggingFace Inc. team.
#
# Licensed under the Apache License, Version 2.0 (the "License");
# you may not use this file except in compliance with the License.
# You may obtain a copy of the License at
#
# http://www.apache.org/licenses/LICENSE-2.0
#
# Unless required by applicable law or agreed to in writing, software
# distributed under the License is distributed on an "AS IS" BASIS,
# WITHOUT WARRANTIES OR CONDITIONS OF ANY KIND, either express or implied.
# See the License for the specific language governing permissions and
# limitations under the License.
import os
from shutil import copyfile
from typing import List, Optional, Tuple
from tokenizers import processors
from ...tokenization_utils import AddedToken, BatchEncoding
from ...tokenization_utils_fast import PreTrainedTokenizerFast
from ...utils import is_sentencepiece_available, logging
if is_sentencepiece_available():
from .tokenization_mbart import MBartTokenizer
else:
MBartTokenizer = None
logger = logging.get_logger(__name__)
VOCAB_FILES_NAMES = {"vocab_file": "sentencepiece.bpe.model", "tokenizer_file": "tokenizer.json"}
PRETRAINED_VOCAB_FILES_MAP = {
"vocab_file": {
"facebook/mbart-large-en-ro": (
"https://huggingface.co/facebook/mbart-large-en-ro/resolve/main/sentencepiece.bpe.model"
),
"facebook/mbart-large-cc25": (
"https://huggingface.co/facebook/mbart-large-cc25/resolve/main/sentencepiece.bpe.model"
),
},
"tokenizer_file": {
"facebook/mbart-large-en-ro": "https://huggingface.co/facebook/mbart-large-en-ro/resolve/main/tokenizer.json",
"facebook/mbart-large-cc25": "https://huggingface.co/facebook/mbart-large-cc25/resolve/main/tokenizer.json",
},
}
PRETRAINED_POSITIONAL_EMBEDDINGS_SIZES = {
"facebook/mbart-large-en-ro": 1024,
"facebook/mbart-large-cc25": 1024,
}
FAIRSEQ_LANGUAGE_CODES = ["ar_AR", "cs_CZ", "de_DE", "en_XX", "es_XX", "et_EE", "fi_FI", "fr_XX", "gu_IN", "hi_IN", "it_IT", "ja_XX", "kk_KZ", "ko_KR", "lt_LT", "lv_LV", "my_MM", "ne_NP", "nl_XX", "ro_RO", "ru_RU", "si_LK", "tr_TR", "vi_VN", "zh_CN"] # fmt: skip
class MBartTokenizerFast(PreTrainedTokenizerFast):
"""
Construct a "fast" MBART tokenizer (backed by HuggingFace's *tokenizers* library). Based on
[BPE](https://huggingface.co/docs/tokenizers/python/latest/components.html?highlight=BPE#models).
This tokenizer inherits from [`PreTrainedTokenizerFast`] which contains most of the main methods. Users should
refer to this superclass for more information regarding those methods.
The tokenization method is `<tokens> <eos> <language code>` for source language documents, and `<language code>
<tokens> <eos>` for target language documents.
Examples:
```python
>>> from transformers import MBartTokenizerFast
>>> tokenizer = MBartTokenizerFast.from_pretrained(
... "facebook/mbart-large-en-ro", src_lang="en_XX", tgt_lang="ro_RO"
... )
>>> example_english_phrase = " UN Chief Says There Is No Military Solution in Syria"
>>> expected_translation_romanian = "Şeful ONU declară că nu există o soluţie militară în Siria"
>>> inputs = tokenizer(example_english_phrase, text_target=expected_translation_romanian, return_tensors="pt")
```"""
vocab_files_names = VOCAB_FILES_NAMES
max_model_input_sizes = PRETRAINED_POSITIONAL_EMBEDDINGS_SIZES
pretrained_vocab_files_map = PRETRAINED_VOCAB_FILES_MAP
model_input_names = ["input_ids", "attention_mask"]
slow_tokenizer_class = MBartTokenizer
prefix_tokens: List[int] = []
suffix_tokens: List[int] = []
def __init__(
self,
vocab_file=None,
tokenizer_file=None,
bos_token="<s>",
eos_token="</s>",
sep_token="</s>",
cls_token="<s>",
unk_token="<unk>",
pad_token="<pad>",
mask_token="<mask>",
src_lang=None,
tgt_lang=None,
additional_special_tokens=None,
**kwargs,
):
# Mask token behave like a normal word, i.e. include the space before it
mask_token = AddedToken(mask_token, lstrip=True, rstrip=False) if isinstance(mask_token, str) else mask_token
_additional_special_tokens = FAIRSEQ_LANGUAGE_CODES.copy()
if additional_special_tokens is not None:
# Only add those special tokens if they are not already there.
_additional_special_tokens.extend(
[t for t in additional_special_tokens if t not in _additional_special_tokens]
)
super().__init__(
vocab_file=vocab_file,
tokenizer_file=tokenizer_file,
bos_token=bos_token,
eos_token=eos_token,
sep_token=sep_token,
cls_token=cls_token,
unk_token=unk_token,
pad_token=pad_token,
mask_token=mask_token,
src_lang=src_lang,
tgt_lang=tgt_lang,
additional_special_tokens=_additional_special_tokens,
**kwargs,
)
self.vocab_file = vocab_file
self.lang_code_to_id = {
lang_code: self.convert_tokens_to_ids(lang_code) for lang_code in FAIRSEQ_LANGUAGE_CODES
}
self._src_lang = src_lang if src_lang is not None else "en_XX"
self.cur_lang_code = self.convert_tokens_to_ids(self._src_lang)
self.tgt_lang = tgt_lang
self.set_src_lang_special_tokens(self._src_lang)
@property
def can_save_slow_tokenizer(self) -> bool:
return os.path.isfile(self.vocab_file) if self.vocab_file else False
@property
def src_lang(self) -> str:
return self._src_lang
@src_lang.setter
def src_lang(self, new_src_lang: str) -> None:
self._src_lang = new_src_lang
self.set_src_lang_special_tokens(self._src_lang)
def build_inputs_with_special_tokens(
self, token_ids_0: List[int], token_ids_1: Optional[List[int]] = None
) -> List[int]:
"""
Build model inputs from a sequence or a pair of sequence for sequence classification tasks by concatenating and
adding special tokens. The special tokens depend on calling set_lang.
An MBART sequence has the following format, where `X` represents the sequence:
- `input_ids` (for encoder) `X [eos, src_lang_code]`
- `decoder_input_ids`: (for decoder) `X [eos, tgt_lang_code]`
BOS is never used. Pairs of sequences are not the expected use case, but they will be handled without a
separator.
Args:
token_ids_0 (`List[int]`):
List of IDs to which the special tokens will be added.
token_ids_1 (`List[int]`, *optional*):
Optional second list of IDs for sequence pairs.
Returns:
`List[int]`: list of [input IDs](../glossary#input-ids) with the appropriate special tokens.
"""
if token_ids_1 is None:
return self.prefix_tokens + token_ids_0 + self.suffix_tokens
# We don't expect to process pairs, but leave the pair logic for API consistency
return self.prefix_tokens + token_ids_0 + token_ids_1 + self.suffix_tokens
def create_token_type_ids_from_sequences(
self, token_ids_0: List[int], token_ids_1: Optional[List[int]] = None
) -> List[int]:
"""
Create a mask from the two sequences passed to be used in a sequence-pair classification task. mBART does not
make use of token type ids, therefore a list of zeros is returned.
Args:
token_ids_0 (`List[int]`):
List of IDs.
token_ids_1 (`List[int]`, *optional*):
Optional second list of IDs for sequence pairs.
Returns:
`List[int]`: List of zeros.
"""
sep = [self.sep_token_id]
cls = [self.cls_token_id]
if token_ids_1 is None:
return len(cls + token_ids_0 + sep) * [0]
return len(cls + token_ids_0 + sep + sep + token_ids_1 + sep) * [0]
def _build_translation_inputs(
self, raw_inputs, return_tensors: str, src_lang: Optional[str], tgt_lang: Optional[str], **extra_kwargs
):
"""Used by translation pipeline, to prepare inputs for the generate function"""
if src_lang is None or tgt_lang is None:
raise ValueError("Translation requires a `src_lang` and a `tgt_lang` for this model")
self.src_lang = src_lang
inputs = self(raw_inputs, add_special_tokens=True, return_tensors=return_tensors, **extra_kwargs)
tgt_lang_id = self.convert_tokens_to_ids(tgt_lang)
inputs["forced_bos_token_id"] = tgt_lang_id
return inputs
def prepare_seq2seq_batch(
self,
src_texts: List[str],
src_lang: str = "en_XX",
tgt_texts: Optional[List[str]] = None,
tgt_lang: str = "ro_RO",
**kwargs,
) -> BatchEncoding:
self.src_lang = src_lang
self.tgt_lang = tgt_lang
return super().prepare_seq2seq_batch(src_texts, tgt_texts, **kwargs)
def _switch_to_input_mode(self):
return self.set_src_lang_special_tokens(self.src_lang)
def _switch_to_target_mode(self):
return self.set_tgt_lang_special_tokens(self.tgt_lang)
def set_src_lang_special_tokens(self, src_lang) -> None:
"""Reset the special tokens to the source lang setting. No prefix and suffix=[eos, src_lang_code]."""
self.cur_lang_code = self.convert_tokens_to_ids(src_lang)
self.prefix_tokens = []
self.suffix_tokens = [self.eos_token_id, self.cur_lang_code]
prefix_tokens_str = self.convert_ids_to_tokens(self.prefix_tokens)
suffix_tokens_str = self.convert_ids_to_tokens(self.suffix_tokens)
self._tokenizer.post_processor = processors.TemplateProcessing(
single=prefix_tokens_str + ["$A"] + suffix_tokens_str,
pair=prefix_tokens_str + ["$A", "$B"] + suffix_tokens_str,
special_tokens=list(zip(prefix_tokens_str + suffix_tokens_str, self.prefix_tokens + self.suffix_tokens)),
)
def set_tgt_lang_special_tokens(self, lang: str) -> None:
"""Reset the special tokens to the target language setting. No prefix and suffix=[eos, tgt_lang_code]."""
self.cur_lang_code = self.convert_tokens_to_ids(lang)
self.prefix_tokens = []
self.suffix_tokens = [self.eos_token_id, self.cur_lang_code]
prefix_tokens_str = self.convert_ids_to_tokens(self.prefix_tokens)
suffix_tokens_str = self.convert_ids_to_tokens(self.suffix_tokens)
self._tokenizer.post_processor = processors.TemplateProcessing(
single=prefix_tokens_str + ["$A"] + suffix_tokens_str,
pair=prefix_tokens_str + ["$A", "$B"] + suffix_tokens_str,
special_tokens=list(zip(prefix_tokens_str + suffix_tokens_str, self.prefix_tokens + self.suffix_tokens)),
)
def save_vocabulary(self, save_directory: str, filename_prefix: Optional[str] = None) -> Tuple[str]:
if not self.can_save_slow_tokenizer:
raise ValueError(
"Your fast tokenizer does not have the necessary information to save the vocabulary for a slow "
"tokenizer."
)
if not os.path.isdir(save_directory):
logger.error(f"Vocabulary path ({save_directory}) should be a directory.")
return
out_vocab_file = os.path.join(
save_directory, (filename_prefix + "-" if filename_prefix else "") + VOCAB_FILES_NAMES["vocab_file"]
)
if os.path.abspath(self.vocab_file) != os.path.abspath(out_vocab_file):
copyfile(self.vocab_file, out_vocab_file)
return (out_vocab_file,)
| transformers/src/transformers/models/mbart/tokenization_mbart_fast.py/0 | {
"file_path": "transformers/src/transformers/models/mbart/tokenization_mbart_fast.py",
"repo_id": "transformers",
"token_count": 5169
} | 312 |
# coding=utf-8
# Copyright 2023 The HuggingFace Inc. team. All rights reserved.
#
# Licensed under the Apache License, Version 2.0 (the "License");
# you may not use this file except in compliance with the License.
# You may obtain a copy of the License at
#
# http://www.apache.org/licenses/LICENSE-2.0
#
# Unless required by applicable law or agreed to in writing, software
# distributed under the License is distributed on an "AS IS" BASIS,
# WITHOUT WARRANTIES OR CONDITIONS OF ANY KIND, either express or implied.
# See the License for the specific language governing permissions and
# limitations under the License.
""" MGP-STR model configuration"""
from ...configuration_utils import PretrainedConfig
from ...utils import logging
logger = logging.get_logger(__name__)
MGP_STR_PRETRAINED_CONFIG_ARCHIVE_MAP = {
"alibaba-damo/mgp-str-base": "https://huggingface.co/alibaba-damo/mgp-str-base/resolve/main/config.json",
}
class MgpstrConfig(PretrainedConfig):
r"""
This is the configuration class to store the configuration of an [`MgpstrModel`]. It is used to instantiate an
MGP-STR model according to the specified arguments, defining the model architecture. Instantiating a configuration
with the defaults will yield a similar configuration to that of the MGP-STR
[alibaba-damo/mgp-str-base](https://huggingface.co/alibaba-damo/mgp-str-base) architecture.
Configuration objects inherit from [`PretrainedConfig`] and can be used to control the model outputs. Read the
documentation from [`PretrainedConfig`] for more information.
Args:
image_size (`List[int]`, *optional*, defaults to `[32, 128]`):
The size (resolution) of each image.
patch_size (`int`, *optional*, defaults to 4):
The size (resolution) of each patch.
num_channels (`int`, *optional*, defaults to 3):
The number of input channels.
max_token_length (`int`, *optional*, defaults to 27):
The max number of output tokens.
num_character_labels (`int`, *optional*, defaults to 38):
The number of classes for character head .
num_bpe_labels (`int`, *optional*, defaults to 50257):
The number of classes for bpe head .
num_wordpiece_labels (`int`, *optional*, defaults to 30522):
The number of classes for wordpiece head .
hidden_size (`int`, *optional*, defaults to 768):
The embedding dimension.
num_hidden_layers (`int`, *optional*, defaults to 12):
Number of hidden layers in the Transformer encoder.
num_attention_heads (`int`, *optional*, defaults to 12):
Number of attention heads for each attention layer in the Transformer encoder.
mlp_ratio (`float`, *optional*, defaults to 4.0):
The ratio of mlp hidden dim to embedding dim.
qkv_bias (`bool`, *optional*, defaults to `True`):
Whether to add a bias to the queries, keys and values.
distilled (`bool`, *optional*, defaults to `False`):
Model includes a distillation token and head as in DeiT models.
layer_norm_eps (`float`, *optional*, defaults to 1e-05):
The epsilon used by the layer normalization layers.
drop_rate (`float`, *optional*, defaults to 0.0):
The dropout probability for all fully connected layers in the embeddings, encoder.
attn_drop_rate (`float`, *optional*, defaults to 0.0):
The dropout ratio for the attention probabilities.
drop_path_rate (`float`, *optional*, defaults to 0.0):
The stochastic depth rate.
output_a3_attentions (`bool`, *optional*, defaults to `False`):
Whether or not the model should returns A^3 module attentions.
initializer_range (`float`, *optional*, defaults to 0.02):
The standard deviation of the truncated_normal_initializer for initializing all weight matrices.
Example:
```python
>>> from transformers import MgpstrConfig, MgpstrForSceneTextRecognition
>>> # Initializing a Mgpstr mgp-str-base style configuration
>>> configuration = MgpstrConfig()
>>> # Initializing a model (with random weights) from the mgp-str-base style configuration
>>> model = MgpstrForSceneTextRecognition(configuration)
>>> # Accessing the model configuration
>>> configuration = model.config
```"""
model_type = "mgp-str"
def __init__(
self,
image_size=[32, 128],
patch_size=4,
num_channels=3,
max_token_length=27,
num_character_labels=38,
num_bpe_labels=50257,
num_wordpiece_labels=30522,
hidden_size=768,
num_hidden_layers=12,
num_attention_heads=12,
mlp_ratio=4.0,
qkv_bias=True,
distilled=False,
layer_norm_eps=1e-5,
drop_rate=0.0,
attn_drop_rate=0.0,
drop_path_rate=0.0,
output_a3_attentions=False,
initializer_range=0.02,
**kwargs,
):
super().__init__(**kwargs)
self.image_size = image_size
self.patch_size = patch_size
self.num_channels = num_channels
self.max_token_length = max_token_length
self.num_character_labels = num_character_labels
self.num_bpe_labels = num_bpe_labels
self.num_wordpiece_labels = num_wordpiece_labels
self.hidden_size = hidden_size
self.num_hidden_layers = num_hidden_layers
self.num_attention_heads = num_attention_heads
self.mlp_ratio = mlp_ratio
self.distilled = distilled
self.layer_norm_eps = layer_norm_eps
self.drop_rate = drop_rate
self.qkv_bias = qkv_bias
self.attn_drop_rate = attn_drop_rate
self.drop_path_rate = drop_path_rate
self.output_a3_attentions = output_a3_attentions
self.initializer_range = initializer_range
| transformers/src/transformers/models/mgp_str/configuration_mgp_str.py/0 | {
"file_path": "transformers/src/transformers/models/mgp_str/configuration_mgp_str.py",
"repo_id": "transformers",
"token_count": 2316
} | 313 |
# coding=utf-8
# Copyright 2018 The HuggingFace Inc. team, Microsoft Corporation.
# Copyright (c) 2018, NVIDIA CORPORATION. All rights reserved.
#
# Licensed under the Apache License, Version 2.0 (the "License");
# you may not use this file except in compliance with the License.
# You may obtain a copy of the License at
#
# http://www.apache.org/licenses/LICENSE-2.0
#
# Unless required by applicable law or agreed to in writing, software
# distributed under the License is distributed on an "AS IS" BASIS,
# WITHOUT WARRANTIES OR CONDITIONS OF ANY KIND, either express or implied.
# See the License for the specific language governing permissions and
# limitations under the License.
"""PyTorch MPNet model."""
import math
from typing import Optional, Tuple, Union
import torch
from torch import nn
from torch.nn import BCEWithLogitsLoss, CrossEntropyLoss, MSELoss
from ...activations import ACT2FN, gelu
from ...modeling_outputs import (
BaseModelOutput,
BaseModelOutputWithPooling,
MaskedLMOutput,
MultipleChoiceModelOutput,
QuestionAnsweringModelOutput,
SequenceClassifierOutput,
TokenClassifierOutput,
)
from ...modeling_utils import PreTrainedModel
from ...pytorch_utils import find_pruneable_heads_and_indices, prune_linear_layer
from ...utils import add_code_sample_docstrings, add_start_docstrings, add_start_docstrings_to_model_forward, logging
from .configuration_mpnet import MPNetConfig
logger = logging.get_logger(__name__)
_CHECKPOINT_FOR_DOC = "microsoft/mpnet-base"
_CONFIG_FOR_DOC = "MPNetConfig"
MPNET_PRETRAINED_MODEL_ARCHIVE_LIST = [
"microsoft/mpnet-base",
]
class MPNetPreTrainedModel(PreTrainedModel):
config_class = MPNetConfig
pretrained_model_archive_map = MPNET_PRETRAINED_MODEL_ARCHIVE_LIST
base_model_prefix = "mpnet"
def _init_weights(self, module):
"""Initialize the weights"""
if isinstance(module, nn.Linear):
# Slightly different from the TF version which uses truncated_normal for initialization
# cf https://github.com/pytorch/pytorch/pull/5617
module.weight.data.normal_(mean=0.0, std=self.config.initializer_range)
if module.bias is not None:
module.bias.data.zero_()
elif isinstance(module, nn.Embedding):
module.weight.data.normal_(mean=0.0, std=self.config.initializer_range)
if module.padding_idx is not None:
module.weight.data[module.padding_idx].zero_()
elif isinstance(module, nn.LayerNorm):
module.bias.data.zero_()
module.weight.data.fill_(1.0)
class MPNetEmbeddings(nn.Module):
def __init__(self, config):
super().__init__()
self.padding_idx = 1
self.word_embeddings = nn.Embedding(config.vocab_size, config.hidden_size, padding_idx=self.padding_idx)
self.position_embeddings = nn.Embedding(
config.max_position_embeddings, config.hidden_size, padding_idx=self.padding_idx
)
self.LayerNorm = nn.LayerNorm(config.hidden_size, eps=config.layer_norm_eps)
self.dropout = nn.Dropout(config.hidden_dropout_prob)
self.register_buffer(
"position_ids", torch.arange(config.max_position_embeddings).expand((1, -1)), persistent=False
)
def forward(self, input_ids=None, position_ids=None, inputs_embeds=None, **kwargs):
if position_ids is None:
if input_ids is not None:
position_ids = create_position_ids_from_input_ids(input_ids, self.padding_idx)
else:
position_ids = self.create_position_ids_from_inputs_embeds(inputs_embeds)
if input_ids is not None:
input_shape = input_ids.size()
else:
input_shape = inputs_embeds.size()[:-1]
seq_length = input_shape[1]
if position_ids is None:
position_ids = self.position_ids[:, :seq_length]
if inputs_embeds is None:
inputs_embeds = self.word_embeddings(input_ids)
position_embeddings = self.position_embeddings(position_ids)
embeddings = inputs_embeds + position_embeddings
embeddings = self.LayerNorm(embeddings)
embeddings = self.dropout(embeddings)
return embeddings
def create_position_ids_from_inputs_embeds(self, inputs_embeds):
"""
We are provided embeddings directly. We cannot infer which are padded so just generate sequential position ids.
Args:
inputs_embeds: torch.Tensor
Returns: torch.Tensor
"""
input_shape = inputs_embeds.size()[:-1]
sequence_length = input_shape[1]
position_ids = torch.arange(
self.padding_idx + 1, sequence_length + self.padding_idx + 1, dtype=torch.long, device=inputs_embeds.device
)
return position_ids.unsqueeze(0).expand(input_shape)
class MPNetSelfAttention(nn.Module):
def __init__(self, config):
super().__init__()
if config.hidden_size % config.num_attention_heads != 0 and not hasattr(config, "embedding_size"):
raise ValueError(
f"The hidden size ({config.hidden_size}) is not a multiple of the number of attention "
f"heads ({config.num_attention_heads})"
)
self.num_attention_heads = config.num_attention_heads
self.attention_head_size = int(config.hidden_size / config.num_attention_heads)
self.all_head_size = self.num_attention_heads * self.attention_head_size
self.q = nn.Linear(config.hidden_size, self.all_head_size)
self.k = nn.Linear(config.hidden_size, self.all_head_size)
self.v = nn.Linear(config.hidden_size, self.all_head_size)
self.o = nn.Linear(config.hidden_size, config.hidden_size)
self.dropout = nn.Dropout(config.attention_probs_dropout_prob)
def transpose_for_scores(self, x):
new_x_shape = x.size()[:-1] + (self.num_attention_heads, self.attention_head_size)
x = x.view(*new_x_shape)
return x.permute(0, 2, 1, 3)
def forward(
self,
hidden_states,
attention_mask=None,
head_mask=None,
position_bias=None,
output_attentions=False,
**kwargs,
):
q = self.q(hidden_states)
k = self.k(hidden_states)
v = self.v(hidden_states)
q = self.transpose_for_scores(q)
k = self.transpose_for_scores(k)
v = self.transpose_for_scores(v)
# Take the dot product between "query" and "key" to get the raw attention scores.
attention_scores = torch.matmul(q, k.transpose(-1, -2))
attention_scores = attention_scores / math.sqrt(self.attention_head_size)
# Apply relative position embedding (precomputed in MPNetEncoder) if provided.
if position_bias is not None:
attention_scores += position_bias
if attention_mask is not None:
attention_scores = attention_scores + attention_mask
# Normalize the attention scores to probabilities.
attention_probs = nn.functional.softmax(attention_scores, dim=-1)
attention_probs = self.dropout(attention_probs)
if head_mask is not None:
attention_probs = attention_probs * head_mask
c = torch.matmul(attention_probs, v)
c = c.permute(0, 2, 1, 3).contiguous()
new_c_shape = c.size()[:-2] + (self.all_head_size,)
c = c.view(*new_c_shape)
o = self.o(c)
outputs = (o, attention_probs) if output_attentions else (o,)
return outputs
class MPNetAttention(nn.Module):
def __init__(self, config):
super().__init__()
self.attn = MPNetSelfAttention(config)
self.LayerNorm = nn.LayerNorm(config.hidden_size, eps=config.layer_norm_eps)
self.dropout = nn.Dropout(config.hidden_dropout_prob)
self.pruned_heads = set()
def prune_heads(self, heads):
if len(heads) == 0:
return
heads, index = find_pruneable_heads_and_indices(
heads, self.attn.num_attention_heads, self.attn.attention_head_size, self.pruned_heads
)
self.attn.q = prune_linear_layer(self.attn.q, index)
self.attn.k = prune_linear_layer(self.attn.k, index)
self.attn.v = prune_linear_layer(self.attn.v, index)
self.attn.o = prune_linear_layer(self.attn.o, index, dim=1)
self.attn.num_attention_heads = self.attn.num_attention_heads - len(heads)
self.attn.all_head_size = self.attn.attention_head_size * self.attn.num_attention_heads
self.pruned_heads = self.pruned_heads.union(heads)
def forward(
self,
hidden_states,
attention_mask=None,
head_mask=None,
position_bias=None,
output_attentions=False,
**kwargs,
):
self_outputs = self.attn(
hidden_states,
attention_mask,
head_mask,
position_bias,
output_attentions=output_attentions,
)
attention_output = self.LayerNorm(self.dropout(self_outputs[0]) + hidden_states)
outputs = (attention_output,) + self_outputs[1:] # add attentions if we output them
return outputs
# Copied from transformers.models.bert.modeling_bert.BertIntermediate
class MPNetIntermediate(nn.Module):
def __init__(self, config):
super().__init__()
self.dense = nn.Linear(config.hidden_size, config.intermediate_size)
if isinstance(config.hidden_act, str):
self.intermediate_act_fn = ACT2FN[config.hidden_act]
else:
self.intermediate_act_fn = config.hidden_act
def forward(self, hidden_states: torch.Tensor) -> torch.Tensor:
hidden_states = self.dense(hidden_states)
hidden_states = self.intermediate_act_fn(hidden_states)
return hidden_states
# Copied from transformers.models.bert.modeling_bert.BertOutput
class MPNetOutput(nn.Module):
def __init__(self, config):
super().__init__()
self.dense = nn.Linear(config.intermediate_size, config.hidden_size)
self.LayerNorm = nn.LayerNorm(config.hidden_size, eps=config.layer_norm_eps)
self.dropout = nn.Dropout(config.hidden_dropout_prob)
def forward(self, hidden_states: torch.Tensor, input_tensor: torch.Tensor) -> torch.Tensor:
hidden_states = self.dense(hidden_states)
hidden_states = self.dropout(hidden_states)
hidden_states = self.LayerNorm(hidden_states + input_tensor)
return hidden_states
class MPNetLayer(nn.Module):
def __init__(self, config):
super().__init__()
self.attention = MPNetAttention(config)
self.intermediate = MPNetIntermediate(config)
self.output = MPNetOutput(config)
def forward(
self,
hidden_states,
attention_mask=None,
head_mask=None,
position_bias=None,
output_attentions=False,
**kwargs,
):
self_attention_outputs = self.attention(
hidden_states,
attention_mask,
head_mask,
position_bias=position_bias,
output_attentions=output_attentions,
)
attention_output = self_attention_outputs[0]
outputs = self_attention_outputs[1:] # add self attentions if we output attention weights
intermediate_output = self.intermediate(attention_output)
layer_output = self.output(intermediate_output, attention_output)
outputs = (layer_output,) + outputs
return outputs
class MPNetEncoder(nn.Module):
def __init__(self, config):
super().__init__()
self.config = config
self.n_heads = config.num_attention_heads
self.layer = nn.ModuleList([MPNetLayer(config) for _ in range(config.num_hidden_layers)])
self.relative_attention_bias = nn.Embedding(config.relative_attention_num_buckets, self.n_heads)
def forward(
self,
hidden_states: torch.Tensor,
attention_mask: Optional[torch.Tensor] = None,
head_mask: Optional[torch.Tensor] = None,
output_attentions: bool = False,
output_hidden_states: bool = False,
return_dict: bool = False,
**kwargs,
):
position_bias = self.compute_position_bias(hidden_states)
all_hidden_states = () if output_hidden_states else None
all_attentions = () if output_attentions else None
for i, layer_module in enumerate(self.layer):
if output_hidden_states:
all_hidden_states = all_hidden_states + (hidden_states,)
layer_outputs = layer_module(
hidden_states,
attention_mask,
head_mask[i],
position_bias,
output_attentions=output_attentions,
**kwargs,
)
hidden_states = layer_outputs[0]
if output_attentions:
all_attentions = all_attentions + (layer_outputs[1],)
# Add last layer
if output_hidden_states:
all_hidden_states = all_hidden_states + (hidden_states,)
if not return_dict:
return tuple(v for v in [hidden_states, all_hidden_states, all_attentions] if v is not None)
return BaseModelOutput(
last_hidden_state=hidden_states,
hidden_states=all_hidden_states,
attentions=all_attentions,
)
def compute_position_bias(self, x, position_ids=None, num_buckets=32):
bsz, qlen, klen = x.size(0), x.size(1), x.size(1)
if position_ids is not None:
context_position = position_ids[:, :, None]
memory_position = position_ids[:, None, :]
else:
context_position = torch.arange(qlen, dtype=torch.long)[:, None]
memory_position = torch.arange(klen, dtype=torch.long)[None, :]
relative_position = memory_position - context_position
rp_bucket = self.relative_position_bucket(relative_position, num_buckets=num_buckets)
rp_bucket = rp_bucket.to(x.device)
values = self.relative_attention_bias(rp_bucket)
values = values.permute([2, 0, 1]).unsqueeze(0)
values = values.expand((bsz, -1, qlen, klen)).contiguous()
return values
@staticmethod
def relative_position_bucket(relative_position, num_buckets=32, max_distance=128):
ret = 0
n = -relative_position
num_buckets //= 2
ret += (n < 0).to(torch.long) * num_buckets
n = torch.abs(n)
max_exact = num_buckets // 2
is_small = n < max_exact
val_if_large = max_exact + (
torch.log(n.float() / max_exact) / math.log(max_distance / max_exact) * (num_buckets - max_exact)
).to(torch.long)
val_if_large = torch.min(val_if_large, torch.full_like(val_if_large, num_buckets - 1))
ret += torch.where(is_small, n, val_if_large)
return ret
# Copied from transformers.models.bert.modeling_bert.BertPooler
class MPNetPooler(nn.Module):
def __init__(self, config):
super().__init__()
self.dense = nn.Linear(config.hidden_size, config.hidden_size)
self.activation = nn.Tanh()
def forward(self, hidden_states: torch.Tensor) -> torch.Tensor:
# We "pool" the model by simply taking the hidden state corresponding
# to the first token.
first_token_tensor = hidden_states[:, 0]
pooled_output = self.dense(first_token_tensor)
pooled_output = self.activation(pooled_output)
return pooled_output
MPNET_START_DOCSTRING = r"""
This model inherits from [`PreTrainedModel`]. Check the superclass documentation for the generic methods the
library implements for all its model (such as downloading or saving, resizing the input embeddings, pruning heads
etc.)
This model is also a PyTorch [torch.nn.Module](https://pytorch.org/docs/stable/nn.html#torch.nn.Module) subclass.
Use it as a regular PyTorch Module and refer to the PyTorch documentation for all matter related to general usage
and behavior.
Parameters:
config ([`MPNetConfig`]): Model configuration class with all the parameters of the model.
Initializing with a config file does not load the weights associated with the model, only the
configuration. Check out the [`~PreTrainedModel.from_pretrained`] method to load the model weights.
"""
MPNET_INPUTS_DOCSTRING = r"""
Args:
input_ids (`torch.LongTensor` of shape `({0})`):
Indices of input sequence tokens in the vocabulary.
Indices can be obtained using [`AutoTokenizer`]. See [`PreTrainedTokenizer.encode`] and
[`PreTrainedTokenizer.__call__`] for details.
[What are input IDs?](../glossary#input-ids)
attention_mask (`torch.FloatTensor` of shape `(batch_size, sequence_length)`, *optional*):
Mask to avoid performing attention on padding token indices. Mask values selected in `[0, 1]`:
- 1 for tokens that are **not masked**,
- 0 for tokens that are **masked**.
[What are attention masks?](../glossary#attention-mask)
position_ids (`torch.LongTensor` of shape `({0})`, *optional*):
Indices of positions of each input sequence tokens in the position embeddings. Selected in the range `[0,
config.max_position_embeddings - 1]`.
[What are position IDs?](../glossary#position-ids)
head_mask (`torch.FloatTensor` of shape `(num_heads,)` or `(num_layers, num_heads)`, *optional*):
Mask to nullify selected heads of the self-attention modules. Mask values selected in `[0, 1]`:
- 1 indicates the head is **not masked**,
- 0 indicates the head is **masked**.
inputs_embeds (`torch.FloatTensor` of shape `({0}, hidden_size)`, *optional*):
Optionally, instead of passing `input_ids` you can choose to directly pass an embedded representation. This
is useful if you want more control over how to convert *input_ids* indices into associated vectors than the
model's internal embedding lookup matrix.
output_attentions (`bool`, *optional*):
Whether or not to return the attentions tensors of all attention layers. See `attentions` under returned
tensors for more detail.
output_hidden_states (`bool`, *optional*):
Whether or not to return the hidden states of all layers. See `hidden_states` under returned tensors for
more detail.
return_dict (`bool`, *optional*):
Whether or not to return a [`~utils.ModelOutput`] instead of a plain tuple.
"""
@add_start_docstrings(
"The bare MPNet Model transformer outputting raw hidden-states without any specific head on top.",
MPNET_START_DOCSTRING,
)
class MPNetModel(MPNetPreTrainedModel):
def __init__(self, config, add_pooling_layer=True):
super().__init__(config)
self.config = config
self.embeddings = MPNetEmbeddings(config)
self.encoder = MPNetEncoder(config)
self.pooler = MPNetPooler(config) if add_pooling_layer else None
# Initialize weights and apply final processing
self.post_init()
def get_input_embeddings(self):
return self.embeddings.word_embeddings
def set_input_embeddings(self, value):
self.embeddings.word_embeddings = value
def _prune_heads(self, heads_to_prune):
"""
Prunes heads of the model. heads_to_prune: dict of {layer_num: list of heads to prune in this layer} See base
class PreTrainedModel
"""
for layer, heads in heads_to_prune.items():
self.encoder.layer[layer].attention.prune_heads(heads)
@add_start_docstrings_to_model_forward(MPNET_INPUTS_DOCSTRING.format("batch_size, sequence_length"))
@add_code_sample_docstrings(
checkpoint=_CHECKPOINT_FOR_DOC,
output_type=BaseModelOutputWithPooling,
config_class=_CONFIG_FOR_DOC,
)
def forward(
self,
input_ids: Optional[torch.LongTensor] = None,
attention_mask: Optional[torch.FloatTensor] = None,
position_ids: Optional[torch.LongTensor] = None,
head_mask: Optional[torch.FloatTensor] = None,
inputs_embeds: Optional[torch.FloatTensor] = None,
output_attentions: Optional[bool] = None,
output_hidden_states: Optional[bool] = None,
return_dict: Optional[bool] = None,
**kwargs,
) -> Union[Tuple[torch.Tensor], BaseModelOutputWithPooling]:
output_attentions = output_attentions if output_attentions is not None else self.config.output_attentions
output_hidden_states = (
output_hidden_states if output_hidden_states is not None else self.config.output_hidden_states
)
return_dict = return_dict if return_dict is not None else self.config.use_return_dict
if input_ids is not None and inputs_embeds is not None:
raise ValueError("You cannot specify both input_ids and inputs_embeds at the same time")
elif input_ids is not None:
self.warn_if_padding_and_no_attention_mask(input_ids, attention_mask)
input_shape = input_ids.size()
elif inputs_embeds is not None:
input_shape = inputs_embeds.size()[:-1]
else:
raise ValueError("You have to specify either input_ids or inputs_embeds")
device = input_ids.device if input_ids is not None else inputs_embeds.device
if attention_mask is None:
attention_mask = torch.ones(input_shape, device=device)
extended_attention_mask: torch.Tensor = self.get_extended_attention_mask(attention_mask, input_shape)
head_mask = self.get_head_mask(head_mask, self.config.num_hidden_layers)
embedding_output = self.embeddings(input_ids=input_ids, position_ids=position_ids, inputs_embeds=inputs_embeds)
encoder_outputs = self.encoder(
embedding_output,
attention_mask=extended_attention_mask,
head_mask=head_mask,
output_attentions=output_attentions,
output_hidden_states=output_hidden_states,
return_dict=return_dict,
)
sequence_output = encoder_outputs[0]
pooled_output = self.pooler(sequence_output) if self.pooler is not None else None
if not return_dict:
return (sequence_output, pooled_output) + encoder_outputs[1:]
return BaseModelOutputWithPooling(
last_hidden_state=sequence_output,
pooler_output=pooled_output,
hidden_states=encoder_outputs.hidden_states,
attentions=encoder_outputs.attentions,
)
class MPNetForMaskedLM(MPNetPreTrainedModel):
_tied_weights_keys = ["lm_head.decoder"]
def __init__(self, config):
super().__init__(config)
self.mpnet = MPNetModel(config, add_pooling_layer=False)
self.lm_head = MPNetLMHead(config)
# Initialize weights and apply final processing
self.post_init()
def get_output_embeddings(self):
return self.lm_head.decoder
def set_output_embeddings(self, new_embeddings):
self.lm_head.decoder = new_embeddings
@add_start_docstrings_to_model_forward(MPNET_INPUTS_DOCSTRING.format("batch_size, sequence_length"))
@add_code_sample_docstrings(
checkpoint=_CHECKPOINT_FOR_DOC,
output_type=MaskedLMOutput,
config_class=_CONFIG_FOR_DOC,
)
def forward(
self,
input_ids: Optional[torch.LongTensor] = None,
attention_mask: Optional[torch.FloatTensor] = None,
position_ids: Optional[torch.LongTensor] = None,
head_mask: Optional[torch.FloatTensor] = None,
inputs_embeds: Optional[torch.FloatTensor] = None,
labels: Optional[torch.LongTensor] = None,
output_attentions: Optional[bool] = None,
output_hidden_states: Optional[bool] = None,
return_dict: Optional[bool] = None,
) -> Union[Tuple[torch.Tensor], MaskedLMOutput]:
r"""
labels (`torch.LongTensor` of shape `(batch_size, sequence_length)`, *optional*):
Labels for computing the masked language modeling loss. Indices should be in `[-100, 0, ...,
config.vocab_size]` (see `input_ids` docstring) Tokens with indices set to `-100` are ignored (masked), the
loss is only computed for the tokens with labels in `[0, ..., config.vocab_size]`
"""
return_dict = return_dict if return_dict is not None else self.config.use_return_dict
outputs = self.mpnet(
input_ids,
attention_mask=attention_mask,
position_ids=position_ids,
head_mask=head_mask,
inputs_embeds=inputs_embeds,
output_attentions=output_attentions,
output_hidden_states=output_hidden_states,
return_dict=return_dict,
)
sequence_output = outputs[0]
prediction_scores = self.lm_head(sequence_output)
masked_lm_loss = None
if labels is not None:
loss_fct = CrossEntropyLoss()
masked_lm_loss = loss_fct(prediction_scores.view(-1, self.config.vocab_size), labels.view(-1))
if not return_dict:
output = (prediction_scores,) + outputs[2:]
return ((masked_lm_loss,) + output) if masked_lm_loss is not None else output
return MaskedLMOutput(
loss=masked_lm_loss,
logits=prediction_scores,
hidden_states=outputs.hidden_states,
attentions=outputs.attentions,
)
class MPNetLMHead(nn.Module):
"""MPNet Head for masked and permuted language modeling."""
def __init__(self, config):
super().__init__()
self.dense = nn.Linear(config.hidden_size, config.hidden_size)
self.layer_norm = nn.LayerNorm(config.hidden_size, eps=config.layer_norm_eps)
self.decoder = nn.Linear(config.hidden_size, config.vocab_size, bias=False)
self.bias = nn.Parameter(torch.zeros(config.vocab_size))
# Need a link between the two variables so that the bias is correctly resized with `resize_token_embeddings`
self.decoder.bias = self.bias
def forward(self, features, **kwargs):
x = self.dense(features)
x = gelu(x)
x = self.layer_norm(x)
# project back to size of vocabulary with bias
x = self.decoder(x)
return x
@add_start_docstrings(
"""
MPNet Model transformer with a sequence classification/regression head on top (a linear layer on top of the pooled
output) e.g. for GLUE tasks.
""",
MPNET_START_DOCSTRING,
)
class MPNetForSequenceClassification(MPNetPreTrainedModel):
def __init__(self, config):
super().__init__(config)
self.num_labels = config.num_labels
self.mpnet = MPNetModel(config, add_pooling_layer=False)
self.classifier = MPNetClassificationHead(config)
# Initialize weights and apply final processing
self.post_init()
@add_start_docstrings_to_model_forward(MPNET_INPUTS_DOCSTRING.format("batch_size, sequence_length"))
@add_code_sample_docstrings(
checkpoint=_CHECKPOINT_FOR_DOC,
output_type=SequenceClassifierOutput,
config_class=_CONFIG_FOR_DOC,
)
def forward(
self,
input_ids: Optional[torch.LongTensor] = None,
attention_mask: Optional[torch.FloatTensor] = None,
position_ids: Optional[torch.LongTensor] = None,
head_mask: Optional[torch.FloatTensor] = None,
inputs_embeds: Optional[torch.FloatTensor] = None,
labels: Optional[torch.LongTensor] = None,
output_attentions: Optional[bool] = None,
output_hidden_states: Optional[bool] = None,
return_dict: Optional[bool] = None,
) -> Union[Tuple[torch.Tensor], SequenceClassifierOutput]:
r"""
labels (`torch.LongTensor` of shape `(batch_size,)`, *optional*):
Labels for computing the sequence classification/regression loss. Indices should be in `[0, ...,
config.num_labels - 1]`. If `config.num_labels == 1` a regression loss is computed (Mean-Square loss), If
`config.num_labels > 1` a classification loss is computed (Cross-Entropy).
"""
return_dict = return_dict if return_dict is not None else self.config.use_return_dict
outputs = self.mpnet(
input_ids,
attention_mask=attention_mask,
position_ids=position_ids,
head_mask=head_mask,
inputs_embeds=inputs_embeds,
output_attentions=output_attentions,
output_hidden_states=output_hidden_states,
return_dict=return_dict,
)
sequence_output = outputs[0]
logits = self.classifier(sequence_output)
loss = None
if labels is not None:
if self.config.problem_type is None:
if self.num_labels == 1:
self.config.problem_type = "regression"
elif self.num_labels > 1 and (labels.dtype == torch.long or labels.dtype == torch.int):
self.config.problem_type = "single_label_classification"
else:
self.config.problem_type = "multi_label_classification"
if self.config.problem_type == "regression":
loss_fct = MSELoss()
if self.num_labels == 1:
loss = loss_fct(logits.squeeze(), labels.squeeze())
else:
loss = loss_fct(logits, labels)
elif self.config.problem_type == "single_label_classification":
loss_fct = CrossEntropyLoss()
loss = loss_fct(logits.view(-1, self.num_labels), labels.view(-1))
elif self.config.problem_type == "multi_label_classification":
loss_fct = BCEWithLogitsLoss()
loss = loss_fct(logits, labels)
if not return_dict:
output = (logits,) + outputs[2:]
return ((loss,) + output) if loss is not None else output
return SequenceClassifierOutput(
loss=loss,
logits=logits,
hidden_states=outputs.hidden_states,
attentions=outputs.attentions,
)
@add_start_docstrings(
"""
MPNet Model with a multiple choice classification head on top (a linear layer on top of the pooled output and a
softmax) e.g. for RocStories/SWAG tasks.
""",
MPNET_START_DOCSTRING,
)
class MPNetForMultipleChoice(MPNetPreTrainedModel):
def __init__(self, config):
super().__init__(config)
self.mpnet = MPNetModel(config)
self.dropout = nn.Dropout(config.hidden_dropout_prob)
self.classifier = nn.Linear(config.hidden_size, 1)
# Initialize weights and apply final processing
self.post_init()
@add_start_docstrings_to_model_forward(MPNET_INPUTS_DOCSTRING.format("batch_size, num_choices, sequence_length"))
@add_code_sample_docstrings(
checkpoint=_CHECKPOINT_FOR_DOC,
output_type=MultipleChoiceModelOutput,
config_class=_CONFIG_FOR_DOC,
)
def forward(
self,
input_ids: Optional[torch.LongTensor] = None,
attention_mask: Optional[torch.FloatTensor] = None,
position_ids: Optional[torch.LongTensor] = None,
head_mask: Optional[torch.FloatTensor] = None,
inputs_embeds: Optional[torch.FloatTensor] = None,
labels: Optional[torch.LongTensor] = None,
output_attentions: Optional[bool] = None,
output_hidden_states: Optional[bool] = None,
return_dict: Optional[bool] = None,
) -> Union[Tuple[torch.Tensor], MultipleChoiceModelOutput]:
r"""
labels (`torch.LongTensor` of shape `(batch_size,)`, *optional*):
Labels for computing the multiple choice classification loss. Indices should be in `[0, ...,
num_choices-1]` where `num_choices` is the size of the second dimension of the input tensors. (See
`input_ids` above)
"""
return_dict = return_dict if return_dict is not None else self.config.use_return_dict
num_choices = input_ids.shape[1] if input_ids is not None else inputs_embeds.shape[1]
flat_input_ids = input_ids.view(-1, input_ids.size(-1)) if input_ids is not None else None
flat_position_ids = position_ids.view(-1, position_ids.size(-1)) if position_ids is not None else None
flat_attention_mask = attention_mask.view(-1, attention_mask.size(-1)) if attention_mask is not None else None
flat_inputs_embeds = (
inputs_embeds.view(-1, inputs_embeds.size(-2), inputs_embeds.size(-1))
if inputs_embeds is not None
else None
)
outputs = self.mpnet(
flat_input_ids,
position_ids=flat_position_ids,
attention_mask=flat_attention_mask,
head_mask=head_mask,
inputs_embeds=flat_inputs_embeds,
output_attentions=output_attentions,
output_hidden_states=output_hidden_states,
return_dict=return_dict,
)
pooled_output = outputs[1]
pooled_output = self.dropout(pooled_output)
logits = self.classifier(pooled_output)
reshaped_logits = logits.view(-1, num_choices)
loss = None
if labels is not None:
loss_fct = CrossEntropyLoss()
loss = loss_fct(reshaped_logits, labels)
if not return_dict:
output = (reshaped_logits,) + outputs[2:]
return ((loss,) + output) if loss is not None else output
return MultipleChoiceModelOutput(
loss=loss,
logits=reshaped_logits,
hidden_states=outputs.hidden_states,
attentions=outputs.attentions,
)
@add_start_docstrings(
"""
MPNet Model with a token classification head on top (a linear layer on top of the hidden-states output) e.g. for
Named-Entity-Recognition (NER) tasks.
""",
MPNET_START_DOCSTRING,
)
class MPNetForTokenClassification(MPNetPreTrainedModel):
def __init__(self, config):
super().__init__(config)
self.num_labels = config.num_labels
self.mpnet = MPNetModel(config, add_pooling_layer=False)
self.dropout = nn.Dropout(config.hidden_dropout_prob)
self.classifier = nn.Linear(config.hidden_size, config.num_labels)
# Initialize weights and apply final processing
self.post_init()
@add_start_docstrings_to_model_forward(MPNET_INPUTS_DOCSTRING.format("batch_size, sequence_length"))
@add_code_sample_docstrings(
checkpoint=_CHECKPOINT_FOR_DOC,
output_type=TokenClassifierOutput,
config_class=_CONFIG_FOR_DOC,
)
def forward(
self,
input_ids: Optional[torch.LongTensor] = None,
attention_mask: Optional[torch.FloatTensor] = None,
position_ids: Optional[torch.LongTensor] = None,
head_mask: Optional[torch.FloatTensor] = None,
inputs_embeds: Optional[torch.FloatTensor] = None,
labels: Optional[torch.LongTensor] = None,
output_attentions: Optional[bool] = None,
output_hidden_states: Optional[bool] = None,
return_dict: Optional[bool] = None,
) -> Union[Tuple[torch.Tensor], TokenClassifierOutput]:
r"""
labels (`torch.LongTensor` of shape `(batch_size, sequence_length)`, *optional*):
Labels for computing the token classification loss. Indices should be in `[0, ..., config.num_labels - 1]`.
"""
return_dict = return_dict if return_dict is not None else self.config.use_return_dict
outputs = self.mpnet(
input_ids,
attention_mask=attention_mask,
position_ids=position_ids,
head_mask=head_mask,
inputs_embeds=inputs_embeds,
output_attentions=output_attentions,
output_hidden_states=output_hidden_states,
return_dict=return_dict,
)
sequence_output = outputs[0]
sequence_output = self.dropout(sequence_output)
logits = self.classifier(sequence_output)
loss = None
if labels is not None:
loss_fct = CrossEntropyLoss()
loss = loss_fct(logits.view(-1, self.num_labels), labels.view(-1))
if not return_dict:
output = (logits,) + outputs[2:]
return ((loss,) + output) if loss is not None else output
return TokenClassifierOutput(
loss=loss,
logits=logits,
hidden_states=outputs.hidden_states,
attentions=outputs.attentions,
)
class MPNetClassificationHead(nn.Module):
"""Head for sentence-level classification tasks."""
def __init__(self, config):
super().__init__()
self.dense = nn.Linear(config.hidden_size, config.hidden_size)
self.dropout = nn.Dropout(config.hidden_dropout_prob)
self.out_proj = nn.Linear(config.hidden_size, config.num_labels)
def forward(self, features, **kwargs):
x = features[:, 0, :] # take <s> token (equiv. to BERT's [CLS] token)
x = self.dropout(x)
x = self.dense(x)
x = torch.tanh(x)
x = self.dropout(x)
x = self.out_proj(x)
return x
@add_start_docstrings(
"""
MPNet Model with a span classification head on top for extractive question-answering tasks like SQuAD (a linear
layers on top of the hidden-states output to compute `span start logits` and `span end logits`).
""",
MPNET_START_DOCSTRING,
)
class MPNetForQuestionAnswering(MPNetPreTrainedModel):
def __init__(self, config):
super().__init__(config)
self.num_labels = config.num_labels
self.mpnet = MPNetModel(config, add_pooling_layer=False)
self.qa_outputs = nn.Linear(config.hidden_size, config.num_labels)
# Initialize weights and apply final processing
self.post_init()
@add_start_docstrings_to_model_forward(MPNET_INPUTS_DOCSTRING.format("batch_size, sequence_length"))
@add_code_sample_docstrings(
checkpoint=_CHECKPOINT_FOR_DOC,
output_type=QuestionAnsweringModelOutput,
config_class=_CONFIG_FOR_DOC,
)
def forward(
self,
input_ids: Optional[torch.LongTensor] = None,
attention_mask: Optional[torch.FloatTensor] = None,
position_ids: Optional[torch.LongTensor] = None,
head_mask: Optional[torch.FloatTensor] = None,
inputs_embeds: Optional[torch.FloatTensor] = None,
start_positions: Optional[torch.LongTensor] = None,
end_positions: Optional[torch.LongTensor] = None,
output_attentions: Optional[bool] = None,
output_hidden_states: Optional[bool] = None,
return_dict: Optional[bool] = None,
) -> Union[Tuple[torch.Tensor], QuestionAnsweringModelOutput]:
r"""
start_positions (`torch.LongTensor` of shape `(batch_size,)`, *optional*):
Labels for position (index) of the start of the labelled span for computing the token classification loss.
Positions are clamped to the length of the sequence (`sequence_length`). Position outside of the sequence
are not taken into account for computing the loss.
end_positions (`torch.LongTensor` of shape `(batch_size,)`, *optional*):
Labels for position (index) of the end of the labelled span for computing the token classification loss.
Positions are clamped to the length of the sequence (`sequence_length`). Position outside of the sequence
are not taken into account for computing the loss.
"""
return_dict = return_dict if return_dict is not None else self.config.use_return_dict
outputs = self.mpnet(
input_ids,
attention_mask=attention_mask,
position_ids=position_ids,
head_mask=head_mask,
inputs_embeds=inputs_embeds,
output_attentions=output_attentions,
output_hidden_states=output_hidden_states,
return_dict=return_dict,
)
sequence_output = outputs[0]
logits = self.qa_outputs(sequence_output)
start_logits, end_logits = logits.split(1, dim=-1)
start_logits = start_logits.squeeze(-1).contiguous()
end_logits = end_logits.squeeze(-1).contiguous()
total_loss = None
if start_positions is not None and end_positions is not None:
# If we are on multi-GPU, split add a dimension
if len(start_positions.size()) > 1:
start_positions = start_positions.squeeze(-1)
if len(end_positions.size()) > 1:
end_positions = end_positions.squeeze(-1)
# sometimes the start/end positions are outside our model inputs, we ignore these terms
ignored_index = start_logits.size(1)
start_positions = start_positions.clamp(0, ignored_index)
end_positions = end_positions.clamp(0, ignored_index)
loss_fct = CrossEntropyLoss(ignore_index=ignored_index)
start_loss = loss_fct(start_logits, start_positions)
end_loss = loss_fct(end_logits, end_positions)
total_loss = (start_loss + end_loss) / 2
if not return_dict:
output = (start_logits, end_logits) + outputs[2:]
return ((total_loss,) + output) if total_loss is not None else output
return QuestionAnsweringModelOutput(
loss=total_loss,
start_logits=start_logits,
end_logits=end_logits,
hidden_states=outputs.hidden_states,
attentions=outputs.attentions,
)
def create_position_ids_from_input_ids(input_ids, padding_idx):
"""
Replace non-padding symbols with their position numbers. Position numbers begin at padding_idx+1. Padding symbols
are ignored. This is modified from fairseq's `utils.make_positions`. :param torch.Tensor x: :return torch.Tensor:
"""
# The series of casts and type-conversions here are carefully balanced to both work with ONNX export and XLA.
mask = input_ids.ne(padding_idx).int()
incremental_indices = torch.cumsum(mask, dim=1).type_as(mask) * mask
return incremental_indices.long() + padding_idx
| transformers/src/transformers/models/mpnet/modeling_mpnet.py/0 | {
"file_path": "transformers/src/transformers/models/mpnet/modeling_mpnet.py",
"repo_id": "transformers",
"token_count": 18262
} | 314 |
# Copyright 2023 The HuggingFace Team. All rights reserved.
#
# Licensed under the Apache License, Version 2.0 (the "License");
# you may not use this file except in compliance with the License.
# You may obtain a copy of the License at
#
# http://www.apache.org/licenses/LICENSE-2.0
#
# Unless required by applicable law or agreed to in writing, software
# distributed under the License is distributed on an "AS IS" BASIS,
# WITHOUT WARRANTIES OR CONDITIONS OF ANY KIND, either express or implied.
# See the License for the specific language governing permissions and
# limitations under the License.
from typing import TYPE_CHECKING
from ...utils import OptionalDependencyNotAvailable, _LazyModule, is_torch_available
_import_structure = {
"configuration_musicgen": [
"MUSICGEN_PRETRAINED_CONFIG_ARCHIVE_MAP",
"MusicgenConfig",
"MusicgenDecoderConfig",
],
"processing_musicgen": ["MusicgenProcessor"],
}
try:
if not is_torch_available():
raise OptionalDependencyNotAvailable()
except OptionalDependencyNotAvailable:
pass
else:
_import_structure["modeling_musicgen"] = [
"MUSICGEN_PRETRAINED_MODEL_ARCHIVE_LIST",
"MusicgenForConditionalGeneration",
"MusicgenForCausalLM",
"MusicgenModel",
"MusicgenPreTrainedModel",
]
if TYPE_CHECKING:
from .configuration_musicgen import (
MUSICGEN_PRETRAINED_CONFIG_ARCHIVE_MAP,
MusicgenConfig,
MusicgenDecoderConfig,
)
from .processing_musicgen import MusicgenProcessor
try:
if not is_torch_available():
raise OptionalDependencyNotAvailable()
except OptionalDependencyNotAvailable:
pass
else:
from .modeling_musicgen import (
MUSICGEN_PRETRAINED_MODEL_ARCHIVE_LIST,
MusicgenForCausalLM,
MusicgenForConditionalGeneration,
MusicgenModel,
MusicgenPreTrainedModel,
)
else:
import sys
sys.modules[__name__] = _LazyModule(__name__, globals()["__file__"], _import_structure, module_spec=__spec__)
| transformers/src/transformers/models/musicgen/__init__.py/0 | {
"file_path": "transformers/src/transformers/models/musicgen/__init__.py",
"repo_id": "transformers",
"token_count": 799
} | 315 |
# coding=utf-8
# Copyright 2022 The Fairseq Authors and The Google Flax Team Authors And The HuggingFace Inc. team. All rights reserved.
#
# Licensed under the Apache License, Version 2.0 (the "License");
# you may not use this file except in compliance with the License.
# You may obtain a copy of the License at
#
# http://www.apache.org/licenses/LICENSE-2.0
#
# Unless required by applicable law or agreed to in writing, software
# distributed under the License is distributed on an "AS IS" BASIS,
# WITHOUT WARRANTIES OR CONDITIONS OF ANY KIND, either express or implied.
# See the License for the specific language governing permissions and
# limitations under the License.
""" Flax OPT model."""
from functools import partial
from typing import Optional, Tuple
import flax.linen as nn
import jax
import jax.numpy as jnp
from flax.core.frozen_dict import FrozenDict, freeze, unfreeze
from flax.linen import combine_masks, make_causal_mask
from flax.linen.attention import dot_product_attention_weights
from flax.traverse_util import flatten_dict, unflatten_dict
from jax import lax
from jax.random import PRNGKey
from ...modeling_flax_outputs import FlaxBaseModelOutput, FlaxMaskedLMOutput
from ...modeling_flax_utils import ACT2FN, FlaxPreTrainedModel, append_call_sample_docstring
from ...utils import add_start_docstrings, logging
from .configuration_opt import OPTConfig
logger = logging.get_logger(__name__)
_CHECKPOINT_FOR_DOC = "facebook/opt-350m"
_CONFIG_FOR_DOC = "OPTConfig"
OPT_START_DOCSTRING = r"""
This model inherits from [`FlaxPreTrainedModel`]. Check the superclass documentation for the generic methods the
library implements for all its model (such as downloading or saving, resizing the input embeddings, pruning heads
etc.)
This model is also a Flax Linen
[flax.nn.Module](https://flax.readthedocs.io/en/latest/_autosummary/flax.nn.module.html) subclass. Use it as a
regular Flax Module and refer to the Flax documentation for all matter related to general usage and behavior.
Finally, this model supports inherent JAX features such as:
- [Just-In-Time (JIT) compilation](https://jax.readthedocs.io/en/latest/jax.html#just-in-time-compilation-jit)
- [Automatic Differentiation](https://jax.readthedocs.io/en/latest/jax.html#automatic-differentiation)
- [Vectorization](https://jax.readthedocs.io/en/latest/jax.html#vectorization-vmap)
- [Parallelization](https://jax.readthedocs.io/en/latest/jax.html#parallelization-pmap)
Parameters:
config ([`OPTConfig`]): Model configuration class with all the parameters of the model.
Initializing with a config file does not load the weights associated with the model, only the
configuration. Check out the [`~FlaxPreTrainedModel.from_pretrained`] method to load the model weights.
dtype (`jax.numpy.dtype`, *optional*, defaults to `jax.numpy.float32`):
The data type of the computation. Can be one of `jax.numpy.float32`, `jax.numpy.float16` (on GPUs) and
`jax.numpy.bfloat16` (on TPUs).
This can be used to enable mixed-precision training or half-precision inference on GPUs or TPUs. If
specified all the computation will be performed with the given `dtype`.
**Note that this only specifies the dtype of the computation and does not influence the dtype of model
parameters.**
If you wish to change the dtype of the model parameters, see [`~FlaxPreTrainedModel.to_fp16`] and
[`~FlaxPreTrainedModel.to_bf16`].
"""
OPT_INPUTS_DOCSTRING = r"""
Args:
input_ids (`jnp.ndarray` of shape `(batch_size, sequence_length)`):
Indices of input sequence tokens in the vocabulary. Padding will be ignored by default should you provide
it.
Indices can be obtained using [`AutoTokenizer`]. See [`PreTrainedTokenizer.encode`] and
[`PreTrainedTokenizer.__call__`] for details.
[What are input IDs?](../glossary#input-ids)
attention_mask (`jnp.ndarray` of shape `(batch_size, sequence_length)`, *optional*):
Mask to avoid performing attention on padding token indices. Mask values selected in `[0, 1]`:
- 1 for tokens that are **not masked**,
- 0 for tokens that are **masked**.
[What are attention masks?](../glossary#attention-mask)
position_ids (`numpy.ndarray` of shape `(batch_size, sequence_length)`, *optional*):
Indices of positions of each input sequence tokens in the position embeddings. Selected in the range `[0,
config.max_position_embeddings - 1]`.
output_attentions (`bool`, *optional*):
Whether or not to return the attentions tensors of all attention layers. See `attentions` under returned
tensors for more detail.
output_hidden_states (`bool`, *optional*):
Whether or not to return the hidden states of all layers. See `hidden_states` under returned tensors for
more detail.
return_dict (`bool`, *optional*):
Whether or not to return a [`~utils.ModelOutput`] instead of a plain tuple.
"""
# Copied from transformers.models.bart.modeling_flax_bart.FlaxBartAttention with Bart->OPT
class FlaxOPTAttention(nn.Module):
config: OPTConfig
embed_dim: int
num_heads: int
dropout: float = 0.0
causal: bool = False
bias: bool = True
dtype: jnp.dtype = jnp.float32 # the dtype of the computation
def setup(self) -> None:
self.head_dim = self.embed_dim // self.num_heads
if self.head_dim * self.num_heads != self.embed_dim:
raise ValueError(
f"embed_dim must be divisible by num_heads (got `embed_dim`: {self.embed_dim}"
f" and `num_heads`: {self.num_heads})."
)
dense = partial(
nn.Dense,
self.embed_dim,
use_bias=self.bias,
dtype=self.dtype,
kernel_init=jax.nn.initializers.normal(self.config.init_std),
)
self.q_proj, self.k_proj, self.v_proj = dense(), dense(), dense()
self.out_proj = dense()
self.dropout_layer = nn.Dropout(rate=self.dropout)
if self.causal:
self.causal_mask = make_causal_mask(
jnp.ones((1, self.config.max_position_embeddings), dtype="bool"), dtype="bool"
)
def _split_heads(self, hidden_states):
return hidden_states.reshape(hidden_states.shape[:2] + (self.num_heads, self.head_dim))
def _merge_heads(self, hidden_states):
return hidden_states.reshape(hidden_states.shape[:2] + (self.embed_dim,))
@nn.compact
def _concatenate_to_cache(self, key, value, query, attention_mask):
"""
This function takes projected key, value states from a single input token and concatenates the states to cached
states from previous steps. This function is slighly adapted from the official Flax repository:
https://github.com/google/flax/blob/491ce18759622506588784b4fca0e4bf05f8c8cd/flax/linen/attention.py#L252
"""
# detect if we're initializing by absence of existing cache data.
is_initialized = self.has_variable("cache", "cached_key")
cached_key = self.variable("cache", "cached_key", jnp.zeros, key.shape, key.dtype)
cached_value = self.variable("cache", "cached_value", jnp.zeros, value.shape, value.dtype)
cache_index = self.variable("cache", "cache_index", lambda: jnp.array(0, dtype=jnp.int32))
if is_initialized:
*batch_dims, max_length, num_heads, depth_per_head = cached_key.value.shape
# update key, value caches with our new 1d spatial slices
cur_index = cache_index.value
indices = (0,) * len(batch_dims) + (cur_index, 0, 0)
key = lax.dynamic_update_slice(cached_key.value, key, indices)
value = lax.dynamic_update_slice(cached_value.value, value, indices)
cached_key.value = key
cached_value.value = value
num_updated_cache_vectors = query.shape[1]
cache_index.value = cache_index.value + num_updated_cache_vectors
# causal mask for cached decoder self-attention: our single query position should only attend to those key positions that have already been generated and cached, not the remaining zero elements.
pad_mask = jnp.broadcast_to(
jnp.arange(max_length) < cur_index + num_updated_cache_vectors,
tuple(batch_dims) + (1, num_updated_cache_vectors, max_length),
)
attention_mask = combine_masks(pad_mask, attention_mask)
return key, value, attention_mask
def __call__(
self,
hidden_states: jnp.ndarray,
key_value_states: Optional[jnp.ndarray] = None,
attention_mask: Optional[jnp.ndarray] = None,
init_cache: bool = False,
deterministic: bool = True,
) -> Tuple[jnp.ndarray]:
"""Input shape: Batch x Time x Channel"""
# if key_value_states are provided this layer is used as a cross-attention layer
# for the decoder
is_cross_attention = key_value_states is not None
batch_size = hidden_states.shape[0]
# get query proj
query_states = self.q_proj(hidden_states)
# get key, value proj
if is_cross_attention:
# cross_attentions
key_states = self.k_proj(key_value_states)
value_states = self.v_proj(key_value_states)
else:
# self_attention
key_states = self.k_proj(hidden_states)
value_states = self.v_proj(hidden_states)
query_states = self._split_heads(query_states)
key_states = self._split_heads(key_states)
value_states = self._split_heads(value_states)
# handle cache prepare causal attention mask
if self.causal:
query_length, key_length = query_states.shape[1], key_states.shape[1]
if self.has_variable("cache", "cached_key"):
mask_shift = self.variables["cache"]["cache_index"]
max_decoder_length = self.variables["cache"]["cached_key"].shape[1]
causal_mask = lax.dynamic_slice(
self.causal_mask, (0, 0, mask_shift, 0), (1, 1, query_length, max_decoder_length)
)
else:
causal_mask = self.causal_mask[:, :, :query_length, :key_length]
causal_mask = jnp.broadcast_to(causal_mask, (batch_size,) + causal_mask.shape[1:])
# combine masks if needed
if attention_mask is not None and self.causal:
attention_mask = jnp.broadcast_to(jnp.expand_dims(attention_mask, axis=(-3, -2)), causal_mask.shape)
attention_mask = combine_masks(attention_mask, causal_mask)
elif self.causal:
attention_mask = causal_mask
elif attention_mask is not None:
attention_mask = jnp.expand_dims(attention_mask, axis=(-3, -2))
# During fast autoregressive decoding, we feed one position at a time,
# and cache the keys and values step by step.
if self.causal and (self.has_variable("cache", "cached_key") or init_cache):
key_states, value_states, attention_mask = self._concatenate_to_cache(
key_states, value_states, query_states, attention_mask
)
# Convert the boolean attention mask to an attention bias.
if attention_mask is not None:
# attention mask in the form of attention bias
attention_bias = lax.select(
attention_mask > 0,
jnp.full(attention_mask.shape, 0.0).astype(self.dtype),
jnp.full(attention_mask.shape, jnp.finfo(self.dtype).min).astype(self.dtype),
)
else:
attention_bias = None
dropout_rng = None
if not deterministic and self.dropout > 0.0:
dropout_rng = self.make_rng("dropout")
attn_weights = dot_product_attention_weights(
query_states,
key_states,
bias=attention_bias,
dropout_rng=dropout_rng,
dropout_rate=self.dropout,
broadcast_dropout=True,
deterministic=deterministic,
dtype=self.dtype,
precision=None,
)
attn_output = jnp.einsum("...hqk,...khd->...qhd", attn_weights, value_states)
attn_output = self._merge_heads(attn_output)
attn_output = self.out_proj(attn_output)
return attn_output, attn_weights
class FlaxOPTDecoderLayer(nn.Module):
config: OPTConfig
dtype: jnp.dtype = jnp.float32
def setup(self) -> None:
self.embed_dim = self.config.hidden_size
self.self_attn = FlaxOPTAttention(
config=self.config,
embed_dim=self.embed_dim,
num_heads=self.config.num_attention_heads,
dropout=self.config.attention_dropout,
causal=True,
dtype=self.dtype,
)
self.do_layer_norm_before = self.config.do_layer_norm_before
self.dropout_layer = nn.Dropout(rate=self.config.dropout)
self.activation_fn = ACT2FN[self.config.activation_function]
self.self_attn_layer_norm = nn.LayerNorm(dtype=self.dtype, epsilon=1e-05)
self.fc1 = nn.Dense(
self.config.ffn_dim,
dtype=self.dtype,
kernel_init=jax.nn.initializers.normal(self.config.init_std),
)
self.fc2 = nn.Dense(
self.embed_dim, dtype=self.dtype, kernel_init=jax.nn.initializers.normal(self.config.init_std)
)
self.final_layer_norm = nn.LayerNorm(dtype=self.dtype, epsilon=1e-05)
def __call__(
self,
hidden_states: jnp.ndarray,
attention_mask: jnp.ndarray,
init_cache: bool = False,
output_attentions: bool = True,
deterministic: bool = True,
) -> Tuple[jnp.ndarray]:
residual = hidden_states
# 125m, 1.7B, ..., 175B applies layer norm BEFORE attention
if self.do_layer_norm_before:
hidden_states = self.self_attn_layer_norm(hidden_states)
# Self Attention
hidden_states, self_attn_weights = self.self_attn(
hidden_states=hidden_states,
attention_mask=attention_mask,
init_cache=init_cache,
deterministic=deterministic,
)
hidden_states = self.dropout_layer(hidden_states, deterministic=deterministic)
hidden_states = residual + hidden_states
# 350m applies layer norm AFTER attention
if not self.do_layer_norm_before:
hidden_states = self.self_attn_layer_norm(hidden_states)
# Fully Connected
hidden_states_shape = hidden_states.shape
hidden_states = hidden_states.reshape(-1, hidden_states.shape[-1])
residual = hidden_states
# 125m, 1.7B, ..., 175B applies layer norm BEFORE attention
if self.do_layer_norm_before:
hidden_states = self.final_layer_norm(hidden_states)
hidden_states = self.fc1(hidden_states)
hidden_states = self.activation_fn(hidden_states)
hidden_states = self.fc2(hidden_states)
hidden_states = self.dropout_layer(hidden_states, deterministic=deterministic)
hidden_states = (residual + hidden_states).reshape(hidden_states_shape)
# 350m applies layer norm AFTER attention
if not self.do_layer_norm_before:
hidden_states = self.final_layer_norm(hidden_states)
outputs = (hidden_states,)
if output_attentions:
outputs += (self_attn_weights,)
return outputs
class FlaxOPTDecoderLayerCollection(nn.Module):
config: OPTConfig
dtype: jnp.dtype = jnp.float32 # the dtype of the computation
def setup(self):
self.layers = [
FlaxOPTDecoderLayer(self.config, name=str(i), dtype=self.dtype)
for i in range(self.config.num_hidden_layers)
]
self.layerdrop = self.config.layerdrop
def __call__(
self,
hidden_states,
attention_mask,
deterministic: bool = True,
init_cache: bool = False,
output_attentions: bool = False,
output_hidden_states: bool = False,
):
# decoder layers
all_hidden_states = () if output_hidden_states else None
all_self_attns = () if output_attentions else None
for decoder_layer in self.layers:
if output_hidden_states:
all_hidden_states += (hidden_states,)
layer_outputs = decoder_layer(
hidden_states,
attention_mask=attention_mask,
init_cache=init_cache,
output_attentions=output_attentions,
deterministic=deterministic,
)
hidden_states = layer_outputs[0]
if output_attentions:
all_self_attns += (layer_outputs[1],)
outputs = [hidden_states, all_hidden_states, all_self_attns]
return outputs
class FlaxOPTLearnedPositionalEmbedding(nn.Embed):
"""
This module learns positional embeddings up to a fixed maximum size.
"""
def setup(self):
self.offset = 2
self.embedding = self.param(
"embedding", self.embedding_init, (self.num_embeddings + self.offset, self.features), self.param_dtype
)
def __call__(self, positions):
"""`input_ids_shape` is expected to be [bsz x seqlen]."""
return super().__call__(positions + self.offset)
class FlaxOPTDecoder(nn.Module):
config: OPTConfig
dtype: jnp.dtype = jnp.float32 # the dtype of the computation
offset: int = 2
def setup(self):
self.dropout_layer = nn.Dropout(rate=self.config.dropout)
embed_dim = self.config.hidden_size
self.padding_idx = self.config.pad_token_id
self.max_target_positions = self.config.max_position_embeddings
self.embed_tokens = nn.Embed(
self.config.vocab_size,
self.config.word_embed_proj_dim,
embedding_init=jax.nn.initializers.normal(self.config.init_std),
dtype=self.dtype,
)
self.embed_positions = FlaxOPTLearnedPositionalEmbedding(
self.config.max_position_embeddings,
embed_dim,
embedding_init=jax.nn.initializers.normal(self.config.init_std),
dtype=self.dtype,
)
if self.config.word_embed_proj_dim != self.config.hidden_size:
self.project_in = nn.Dense(self.config.hidden_size, use_bias=False)
self.project_out = nn.Dense(self.config.word_embed_proj_dim, use_bias=False)
else:
self.project_in = None
self.project_out = None
# Note that the only purpose of `config._remove_final_layer_norm` is to keep backward compatibility
# with checkpoints that have been fine-tuned before transformers v4.20.1
# see https://github.com/facebookresearch/metaseq/pull/164
if self.config.do_layer_norm_before and not self.config._remove_final_layer_norm:
self.final_layer_norm = nn.LayerNorm(dtype=self.dtype, epsilon=1e-05)
else:
self.final_layer_norm = None
self.layers = FlaxOPTDecoderLayerCollection(self.config, self.dtype)
def __call__(
self,
input_ids,
attention_mask,
position_ids,
init_cache: bool = False,
output_attentions: bool = False,
output_hidden_states: bool = False,
return_dict: bool = True,
deterministic: bool = True,
):
input_shape = input_ids.shape
input_ids = input_ids.reshape(-1, input_shape[-1])
inputs_embeds = self.embed_tokens(input_ids)
if self.project_in is not None:
inputs_embeds = self.project_in(inputs_embeds)
positions = self.embed_positions(position_ids)
hidden_states = inputs_embeds + positions
hidden_state, all_hidden_states, attentions = self.layers(
hidden_states,
attention_mask,
deterministic=deterministic,
init_cache=init_cache,
output_attentions=output_attentions,
output_hidden_states=output_hidden_states,
)
if self.final_layer_norm is not None:
hidden_state = self.final_layer_norm(hidden_state)
if self.project_out is not None:
hidden_state = self.project_out(hidden_state)
if output_hidden_states:
all_hidden_states += (hidden_state,)
outputs = [hidden_state, all_hidden_states, attentions]
if not return_dict:
return tuple(v for v in outputs if v is not None)
return FlaxBaseModelOutput(
last_hidden_state=hidden_state,
hidden_states=all_hidden_states,
attentions=attentions,
)
class FlaxOPTPreTrainedModel(FlaxPreTrainedModel):
config_class = OPTConfig
base_model_prefix: str = "model"
module_class: nn.Module = None
def __init__(
self,
config: OPTConfig,
input_shape: Tuple[int] = (1, 1),
seed: int = 0,
dtype: jnp.dtype = jnp.float32,
_do_init: bool = True,
**kwargs,
):
module = self.module_class(config=config, dtype=dtype, **kwargs)
super().__init__(config, module, input_shape=input_shape, seed=seed, dtype=dtype, _do_init=_do_init)
def init_weights(self, rng: jax.random.PRNGKey, input_shape: Tuple, params: FrozenDict = None) -> FrozenDict:
# init input tensors
input_ids = jnp.zeros(input_shape, dtype="i4")
attention_mask = jnp.ones_like(input_ids)
batch_size, sequence_length = input_ids.shape
position_ids = jnp.broadcast_to(jnp.arange(sequence_length)[None, :], (batch_size, sequence_length))
params_rng, dropout_rng = jax.random.split(rng)
rngs = {"params": params_rng, "dropout": dropout_rng}
module_init_outputs = self.module.init(
rngs,
input_ids,
attention_mask,
position_ids,
return_dict=False,
)
random_params = module_init_outputs["params"]
if params is not None:
random_params = flatten_dict(unfreeze(random_params))
params = flatten_dict(unfreeze(params))
for missing_key in self._missing_keys:
params[missing_key] = random_params[missing_key]
self._missing_keys = set()
return freeze(unflatten_dict(params))
else:
return random_params
def init_cache(self, batch_size, max_length):
r"""
Args:
batch_size (`int`):
batch_size used for fast auto-regressive decoding. Defines the batch size of the initialized cache.
max_length (`int`):
maximum possible length for auto-regressive decoding. Defines the sequence length of the initialized
cache.
"""
# init input variables to retrieve cache
input_ids = jnp.ones((batch_size, max_length), dtype="i4")
attention_mask = jnp.ones_like(input_ids, dtype="i4")
position_ids = jnp.broadcast_to(jnp.arange(jnp.atleast_2d(input_ids).shape[-1]), input_ids.shape)
init_variables = self.module.init(
jax.random.PRNGKey(0), input_ids, attention_mask, position_ids, return_dict=False, init_cache=True
)
return unfreeze(init_variables["cache"])
def __call__(
self,
input_ids: jnp.ndarray,
attention_mask: Optional[jnp.ndarray] = None,
position_ids: Optional[jnp.ndarray] = None,
params: dict = None,
past_key_values: dict = None,
output_attentions: Optional[bool] = None,
output_hidden_states: Optional[bool] = None,
return_dict: Optional[bool] = None,
dropout_rng: PRNGKey = None,
deterministic: bool = True,
):
output_attentions = output_attentions if output_attentions is not None else self.config.output_attentions
output_hidden_states = (
output_hidden_states if output_hidden_states is not None else self.config.output_hidden_states
)
return_dict = return_dict if return_dict is not None else self.config.return_dict
if attention_mask is None:
attention_mask = jnp.ones_like(input_ids)
if position_ids is None:
position_ids = (attention_mask.cumsum(axis=1) * attention_mask) - 1
# Handle any PRNG if needed
rngs = {"dropout": dropout_rng} if dropout_rng is not None else {}
inputs = {"params": params or self.params}
# if past_key_values are passed then cache is already initialized a private flag init_cache has to be passed
# down to ensure cache is used. It has to be made sure that cache is marked as mutable so that it can be
# changed by FlaxOPTAttention module
if past_key_values:
inputs["cache"] = past_key_values
mutable = ["cache"]
else:
mutable = False
outputs = self.module.apply(
inputs,
input_ids=jnp.array(input_ids, dtype="i4"),
attention_mask=jnp.array(attention_mask, dtype="i4"),
position_ids=jnp.array(position_ids, dtype="i4"),
output_attentions=output_attentions,
output_hidden_states=output_hidden_states,
return_dict=return_dict,
deterministic=deterministic,
rngs=rngs,
mutable=mutable,
)
# add updated cache to model output
if past_key_values is not None and return_dict:
outputs, past_key_values = outputs
outputs["past_key_values"] = unfreeze(past_key_values["cache"])
return outputs
elif past_key_values is not None and not return_dict:
outputs, past_key_values = outputs
outputs = outputs[:1] + (unfreeze(past_key_values["cache"]),) + outputs[1:]
return outputs
class FlaxOPTModule(nn.Module):
config: OPTConfig
dtype: jnp.dtype = jnp.float32 # the dtype of the computation
def setup(self):
self.decoder = FlaxOPTDecoder(self.config, dtype=self.dtype)
def _get_decoder_module(self):
return self.decoder
def __call__(
self,
input_ids,
attention_mask,
position_ids,
output_attentions: bool = False,
output_hidden_states: bool = False,
return_dict: bool = True,
deterministic: bool = True,
init_cache=False,
):
decoder_outputs = self.decoder(
input_ids=input_ids,
attention_mask=attention_mask,
position_ids=position_ids,
output_attentions=output_attentions,
output_hidden_states=output_hidden_states,
return_dict=return_dict,
deterministic=deterministic,
init_cache=init_cache,
)
if not return_dict:
return decoder_outputs
return FlaxBaseModelOutput(
last_hidden_state=decoder_outputs.last_hidden_state,
hidden_states=decoder_outputs.hidden_states,
attentions=decoder_outputs.attentions,
)
# Copied from transformers.models.bart.modeling_flax_bart.FlaxBartModel with Bart->OPT
class FlaxOPTModel(FlaxOPTPreTrainedModel):
config: OPTConfig
dtype: jnp.dtype = jnp.float32 # the dtype of the computation
module_class = FlaxOPTModule
append_call_sample_docstring(FlaxOPTModel, _CHECKPOINT_FOR_DOC, FlaxBaseModelOutput, _CONFIG_FOR_DOC)
@add_start_docstrings(
"The bare OPT Model transformer outputting raw hidden-states without any specific head on top.",
OPT_START_DOCSTRING,
)
class FlaxOPTForCausalLMModule(nn.Module):
config: OPTConfig
dtype: jnp.dtype = jnp.float32
def setup(self):
self.model = FlaxOPTModule(config=self.config, dtype=self.dtype)
self.lm_head = nn.Dense(
self.config.vocab_size,
use_bias=False,
dtype=self.dtype,
kernel_init=jax.nn.initializers.normal(self.config.init_std),
)
def __call__(
self,
input_ids,
attention_mask,
position_ids,
init_cache: bool = False,
output_attentions: bool = False,
output_hidden_states: bool = False,
return_dict: bool = True,
deterministic: bool = True,
):
outputs = self.model(
input_ids,
attention_mask,
position_ids,
init_cache=init_cache,
output_attentions=output_attentions,
output_hidden_states=output_hidden_states,
return_dict=return_dict,
deterministic=deterministic,
)
hidden_states = outputs[0]
if self.config.tie_word_embeddings:
shared_embedding = self.model.variables["params"]["decoder"]["embed_tokens"]["embedding"]
lm_logits = self.lm_head.apply({"params": {"kernel": shared_embedding.T}}, hidden_states)
else:
lm_logits = self.lm_head(hidden_states)
if not return_dict:
return (lm_logits,) + outputs[1:]
return FlaxMaskedLMOutput(
logits=lm_logits,
hidden_states=outputs.hidden_states,
attentions=outputs.attentions,
)
@add_start_docstrings(
"""
OPT Model with a language modeling head on top (linear layer with weights tied to the input embeddings) e.g for
autoregressive tasks.
""",
OPT_START_DOCSTRING,
)
class FlaxOPTForCausalLM(FlaxOPTPreTrainedModel):
module_class = FlaxOPTForCausalLMModule
def prepare_inputs_for_generation(self, input_ids, max_length, attention_mask: Optional[jax.Array] = None):
# initializing the cache
batch_size, seq_length = input_ids.shape
past_key_values = self.init_cache(batch_size, max_length)
# Note that usually one would have to put 0's in the attention_mask for x > input_ids.shape[-1] and x < cache_length.
# But since the decoder uses a causal mask, those positions are masked anyway.
# Thus, we can create a single static attention_mask here, which is more efficient for compilation
extended_attention_mask = jnp.ones((batch_size, max_length), dtype="i4")
if attention_mask is not None:
position_ids = attention_mask.cumsum(axis=1) - 1
extended_attention_mask = lax.dynamic_update_slice(extended_attention_mask, attention_mask, (0, 0))
else:
position_ids = jnp.broadcast_to(jnp.arange(seq_length, dtype="i4")[None, :], (batch_size, seq_length))
return {
"past_key_values": past_key_values,
"attention_mask": extended_attention_mask,
"position_ids": position_ids,
}
def update_inputs_for_generation(self, model_outputs, model_kwargs):
model_kwargs["past_key_values"] = model_outputs.past_key_values
model_kwargs["position_ids"] = model_kwargs["position_ids"][:, -1:] + 1
return model_kwargs
append_call_sample_docstring(
FlaxOPTForCausalLM,
_CHECKPOINT_FOR_DOC,
FlaxBaseModelOutput,
_CONFIG_FOR_DOC,
)
| transformers/src/transformers/models/opt/modeling_flax_opt.py/0 | {
"file_path": "transformers/src/transformers/models/opt/modeling_flax_opt.py",
"repo_id": "transformers",
"token_count": 13784
} | 316 |
# coding=utf-8
# Copyright 2022, Google and The HuggingFace Inc. team. All rights reserved.
#
# Licensed under the Apache License, Version 2.0 (the "License");
# you may not use this file except in compliance with the License.
# You may obtain a copy of the License at
#
# http://www.apache.org/licenses/LICENSE-2.0
#
# Unless required by applicable law or agreed to in writing, software
# distributed under the License is distributed on an "AS IS" BASIS,
# WITHOUT WARRANTIES OR CONDITIONS OF ANY KIND, either express or implied.
# See the License for the specific language governing permissions and
# limitations under the License.
""" PyTorch PEGASUS-X model."""
import dataclasses
import math
from typing import Optional, Tuple, Union
import numpy as np
import torch
import torch.utils.checkpoint
from torch import nn
from torch.nn import CrossEntropyLoss
from ...activations import ACT2FN
from ...modeling_attn_mask_utils import _prepare_4d_attention_mask, _prepare_4d_causal_attention_mask
from ...modeling_outputs import (
BaseModelOutput,
BaseModelOutputWithPastAndCrossAttentions,
Seq2SeqLMOutput,
Seq2SeqModelOutput,
)
from ...modeling_utils import PreTrainedModel
from ...utils import (
add_end_docstrings,
add_start_docstrings,
add_start_docstrings_to_model_forward,
logging,
replace_return_docstrings,
)
from .configuration_pegasus_x import PegasusXConfig
logger = logging.get_logger(__name__)
_CHECKPOINT_FOR_DOC = "google/pegasus-x-base"
_CONFIG_FOR_DOC = "PegasusXConfig"
PEGASUS_X_PRETRAINED_MODEL_ARCHIVE_LIST = [
"google/pegasus-x-base",
"google/pegasus-x-large",
# See all PEGASUS models at https://huggingface.co/models?filter=pegasus-x
]
@dataclasses.dataclass
class DimensionInfo:
"""Wrapper for dimension info."""
batch_size: int # batch size
seq_len: int # token length
block_size: int # block size
num_heads: int # num heads
hidden_dim: int # hidden dim
dim_per_head: int # dim per head
num_blocks: int # num blocks
global_len: int # global length
padded_seq_len: int # padded token seq length
# Note: Compared to the original Flax implementation, we will pad the token representations to
# a multiple of block size at the start of the encoder layers, so T=P always.
# Copied from transformers.models.bart.modeling_bart.shift_tokens_right
def shift_tokens_right(input_ids: torch.Tensor, pad_token_id: int, decoder_start_token_id: int):
"""
Shift input ids one token to the right.
"""
shifted_input_ids = input_ids.new_zeros(input_ids.shape)
shifted_input_ids[:, 1:] = input_ids[:, :-1].clone()
shifted_input_ids[:, 0] = decoder_start_token_id
if pad_token_id is None:
raise ValueError("self.model.config.pad_token_id has to be defined.")
# replace possible -100 values in labels by `pad_token_id`
shifted_input_ids.masked_fill_(shifted_input_ids == -100, pad_token_id)
return shifted_input_ids
class PegasusXSinusoidalPositionalEmbedding(nn.Module):
"""This module produces sinusoidal positional embeddings of any length."""
def __init__(self, embed_dim, max_scale: int = 10000.0):
super().__init__()
self.embed_dim = embed_dim
self.max_scale = max_scale
@torch.no_grad()
def forward(self, input_embeds: torch.Tensor, past_key_values_length: int = 0) -> torch.Tensor:
"""`input_ids_shape` is expected to be [bsz x seqlen]."""
batch_size, seq_len = input_embeds.shape[:2]
positions = torch.arange(
past_key_values_length, past_key_values_length + seq_len, dtype=torch.long, device=input_embeds.device
)[:, None]
pe = torch.zeros((seq_len, self.embed_dim), device=input_embeds.device, dtype=input_embeds.dtype)
half_d_feature = self.embed_dim // 2
div_term = torch.exp(
torch.arange(half_d_feature, device=input_embeds.device, dtype=torch.int64).type_as(input_embeds)
* -(np.log(float(self.max_scale)) / (half_d_feature - 1))
)
pe[:, :half_d_feature] = torch.sin(positions * div_term)
pe[:, half_d_feature:] = torch.cos(positions * div_term)
return pe[None].expand(batch_size, -1, -1)
# Copied from transformers.models.bart.modeling_bart.BartAttention with Bart->PegasusX
class PegasusXAttention(nn.Module):
"""Multi-headed attention from 'Attention Is All You Need' paper"""
def __init__(
self,
embed_dim: int,
num_heads: int,
dropout: float = 0.0,
is_decoder: bool = False,
bias: bool = True,
is_causal: bool = False,
config: Optional[PegasusXConfig] = None,
):
super().__init__()
self.embed_dim = embed_dim
self.num_heads = num_heads
self.dropout = dropout
self.head_dim = embed_dim // num_heads
self.config = config
if (self.head_dim * num_heads) != self.embed_dim:
raise ValueError(
f"embed_dim must be divisible by num_heads (got `embed_dim`: {self.embed_dim}"
f" and `num_heads`: {num_heads})."
)
self.scaling = self.head_dim**-0.5
self.is_decoder = is_decoder
self.is_causal = is_causal
self.k_proj = nn.Linear(embed_dim, embed_dim, bias=bias)
self.v_proj = nn.Linear(embed_dim, embed_dim, bias=bias)
self.q_proj = nn.Linear(embed_dim, embed_dim, bias=bias)
self.out_proj = nn.Linear(embed_dim, embed_dim, bias=bias)
def _shape(self, tensor: torch.Tensor, seq_len: int, bsz: int):
return tensor.view(bsz, seq_len, self.num_heads, self.head_dim).transpose(1, 2).contiguous()
def forward(
self,
hidden_states: torch.Tensor,
key_value_states: Optional[torch.Tensor] = None,
past_key_value: Optional[Tuple[torch.Tensor]] = None,
attention_mask: Optional[torch.Tensor] = None,
layer_head_mask: Optional[torch.Tensor] = None,
output_attentions: bool = False,
) -> Tuple[torch.Tensor, Optional[torch.Tensor], Optional[Tuple[torch.Tensor]]]:
"""Input shape: Batch x Time x Channel"""
# if key_value_states are provided this layer is used as a cross-attention layer
# for the decoder
is_cross_attention = key_value_states is not None
bsz, tgt_len, _ = hidden_states.size()
# get query proj
query_states = self.q_proj(hidden_states) * self.scaling
# get key, value proj
# `past_key_value[0].shape[2] == key_value_states.shape[1]`
# is checking that the `sequence_length` of the `past_key_value` is the same as
# the provided `key_value_states` to support prefix tuning
if (
is_cross_attention
and past_key_value is not None
and past_key_value[0].shape[2] == key_value_states.shape[1]
):
# reuse k,v, cross_attentions
key_states = past_key_value[0]
value_states = past_key_value[1]
elif is_cross_attention:
# cross_attentions
key_states = self._shape(self.k_proj(key_value_states), -1, bsz)
value_states = self._shape(self.v_proj(key_value_states), -1, bsz)
elif past_key_value is not None:
# reuse k, v, self_attention
key_states = self._shape(self.k_proj(hidden_states), -1, bsz)
value_states = self._shape(self.v_proj(hidden_states), -1, bsz)
key_states = torch.cat([past_key_value[0], key_states], dim=2)
value_states = torch.cat([past_key_value[1], value_states], dim=2)
else:
# self_attention
key_states = self._shape(self.k_proj(hidden_states), -1, bsz)
value_states = self._shape(self.v_proj(hidden_states), -1, bsz)
if self.is_decoder:
# if cross_attention save Tuple(torch.Tensor, torch.Tensor) of all cross attention key/value_states.
# Further calls to cross_attention layer can then reuse all cross-attention
# key/value_states (first "if" case)
# if uni-directional self-attention (decoder) save Tuple(torch.Tensor, torch.Tensor) of
# all previous decoder key/value_states. Further calls to uni-directional self-attention
# can concat previous decoder key/value_states to current projected key/value_states (third "elif" case)
# if encoder bi-directional self-attention `past_key_value` is always `None`
past_key_value = (key_states, value_states)
proj_shape = (bsz * self.num_heads, -1, self.head_dim)
query_states = self._shape(query_states, tgt_len, bsz).view(*proj_shape)
key_states = key_states.reshape(*proj_shape)
value_states = value_states.reshape(*proj_shape)
src_len = key_states.size(1)
attn_weights = torch.bmm(query_states, key_states.transpose(1, 2))
if attn_weights.size() != (bsz * self.num_heads, tgt_len, src_len):
raise ValueError(
f"Attention weights should be of size {(bsz * self.num_heads, tgt_len, src_len)}, but is"
f" {attn_weights.size()}"
)
if attention_mask is not None:
if attention_mask.size() != (bsz, 1, tgt_len, src_len):
raise ValueError(
f"Attention mask should be of size {(bsz, 1, tgt_len, src_len)}, but is {attention_mask.size()}"
)
attn_weights = attn_weights.view(bsz, self.num_heads, tgt_len, src_len) + attention_mask
attn_weights = attn_weights.view(bsz * self.num_heads, tgt_len, src_len)
attn_weights = nn.functional.softmax(attn_weights, dim=-1)
if layer_head_mask is not None:
if layer_head_mask.size() != (self.num_heads,):
raise ValueError(
f"Head mask for a single layer should be of size {(self.num_heads,)}, but is"
f" {layer_head_mask.size()}"
)
attn_weights = layer_head_mask.view(1, -1, 1, 1) * attn_weights.view(bsz, self.num_heads, tgt_len, src_len)
attn_weights = attn_weights.view(bsz * self.num_heads, tgt_len, src_len)
if output_attentions:
# this operation is a bit awkward, but it's required to
# make sure that attn_weights keeps its gradient.
# In order to do so, attn_weights have to be reshaped
# twice and have to be reused in the following
attn_weights_reshaped = attn_weights.view(bsz, self.num_heads, tgt_len, src_len)
attn_weights = attn_weights_reshaped.view(bsz * self.num_heads, tgt_len, src_len)
else:
attn_weights_reshaped = None
attn_probs = nn.functional.dropout(attn_weights, p=self.dropout, training=self.training)
attn_output = torch.bmm(attn_probs, value_states)
if attn_output.size() != (bsz * self.num_heads, tgt_len, self.head_dim):
raise ValueError(
f"`attn_output` should be of size {(bsz * self.num_heads, tgt_len, self.head_dim)}, but is"
f" {attn_output.size()}"
)
attn_output = attn_output.view(bsz, self.num_heads, tgt_len, self.head_dim)
attn_output = attn_output.transpose(1, 2)
# Use the `embed_dim` from the config (stored in the class) rather than `hidden_state` because `attn_output` can be
# partitioned across GPUs when using tensor-parallelism.
attn_output = attn_output.reshape(bsz, tgt_len, self.embed_dim)
attn_output = self.out_proj(attn_output)
return attn_output, attn_weights_reshaped, past_key_value
class PegasusXGlobalLocalAttention(nn.Module):
"""Global + Local attention. For use with Encoder only."""
def __init__(
self,
embed_dim: int,
num_heads: int,
block_size: int,
dropout: float = 0.0,
is_decoder: bool = False,
):
super().__init__()
self.embed_dim = embed_dim
self.num_heads = num_heads
self.block_size = block_size
self.dropout = dropout
self.head_dim = embed_dim // num_heads
if (self.head_dim * num_heads) != self.embed_dim:
raise ValueError(
f"embed_dim must be divisible by num_heads (got `embed_dim`: {self.embed_dim}"
f" and `num_heads`: {num_heads})."
)
self.scaling = self.head_dim**-0.5
self.is_decoder = is_decoder
self.k_proj = nn.Linear(embed_dim, embed_dim, bias=False)
self.v_proj = nn.Linear(embed_dim, embed_dim, bias=False)
self.q_proj = nn.Linear(embed_dim, embed_dim, bias=False)
self.out_proj = nn.Linear(embed_dim, embed_dim, bias=False)
def _shape(self, tensor: torch.Tensor, seq_len: int, bsz: int):
return tensor.view(bsz, seq_len, self.num_heads, self.head_dim).transpose(1, 2).contiguous()
def forward(
self,
token_hidden_states: torch.Tensor,
global_hidden_states: torch.Tensor,
attention_mask: Optional[torch.Tensor] = None,
output_attentions: bool = False,
) -> Tuple[torch.Tensor, torch.Tensor, Optional[torch.Tensor]]:
"""Input shape: Batch x Time x Channel"""
dim = DimensionInfo(
batch_size=token_hidden_states.shape[0],
seq_len=token_hidden_states.shape[1],
block_size=self.block_size,
num_heads=self.num_heads,
hidden_dim=token_hidden_states.shape[2],
dim_per_head=self.head_dim,
num_blocks=token_hidden_states.shape[1] // self.block_size,
global_len=global_hidden_states.shape[1],
padded_seq_len=token_hidden_states.shape[1],
)
# [batch_size, num_heads, padded_seq_len, dim_per_head]
local_q = self._shape(
self.q_proj(token_hidden_states) * self.scaling,
seq_len=dim.padded_seq_len,
bsz=dim.batch_size,
)
local_k = self._shape(
self.k_proj(token_hidden_states),
seq_len=dim.padded_seq_len,
bsz=dim.batch_size,
)
local_v = self._shape(
self.v_proj(token_hidden_states),
seq_len=dim.padded_seq_len,
bsz=dim.batch_size,
)
# [batch_size, num_heads, global_len, dim_per_head]
global_q = self._shape(
self.q_proj(global_hidden_states) * self.scaling,
seq_len=dim.global_len,
bsz=dim.batch_size,
)
global_k = self._shape(
self.k_proj(global_hidden_states),
seq_len=dim.global_len,
bsz=dim.batch_size,
)
global_v = self._shape(
self.v_proj(global_hidden_states),
seq_len=dim.global_len,
bsz=dim.batch_size,
)
global_attn_output, global_attn_probs = self.compute_global_attention_representations(
global_q=global_q,
global_k=global_k,
global_v=global_v,
local_k=local_k,
local_v=local_v,
mask=attention_mask,
dim=dim,
)
local_attn_output, local_attn_probs = self.compute_local_attention_representations(
global_k=global_k,
global_v=global_v,
local_q=local_q,
local_k=local_k,
local_v=local_v,
mask=attention_mask,
dim=dim,
)
# [batch_size, global_len, hidden_dim]
global_attn_output = (
global_attn_output.transpose(1, 2).contiguous().view(dim.batch_size, dim.global_len, dim.hidden_dim)
)
# [batch_size, global_len, hidden_dim]
global_attn_output = self.out_proj(global_attn_output)
# [batch_size, num_heads, block_size, num_heads, dim_per_head]
local_attn_output = local_attn_output.permute(0, 2, 3, 1, 4).contiguous()
# [batch_size, padded_seq_len, hidden_dim]
local_attn_output = local_attn_output.view(dim.batch_size, dim.padded_seq_len, dim.hidden_dim)
# [batch_size, padded_seq_len, hidden_dim]
local_attn_output = self.out_proj(local_attn_output)
if output_attentions:
attn_probs = {"global": global_attn_probs, "local": local_attn_probs}
else:
attn_probs = None
return local_attn_output, global_attn_output, attn_probs
def compute_global_attention_representations(
self, global_q, global_k, global_v, local_k, local_v, mask, dim: DimensionInfo
):
"""Compute attention representations for global tokens.
Global tokens will attend to both global tokens as well as all input sequence tokens. Because the input
sequence tokens are arranged in blocks for local attention, we unblock them and compute attention.
Args:
global_q (`torch.FloatTensor`) of shape [batch_size, num_heads, global_len, dim_per_head]:
query vectors from global tokens
global_k (`torch.FloatTensor`) of shape [batch_size, num_heads, global_len, dim_per_head]:
key vectors from global tokens
global_v (`torch.FloatTensor`) of shape [batch_size, num_heads, global_len, dim_per_head]:
value vectors from global tokens
local_k (`torch.FloatTensor`) of shape [batch_size, num_heads, padded_seq_len, dim_per_head]:
key vectors from local tokens
local_v (`torch.FloatTensor`) of shape [batch_size, num_heads, padded_seq_len, dim_per_head]:
value vectors from local tokens
mask (`torch.FloatTensor`) of shape [batch_size, padded_seq_len]: attention mask
dim (DimensionInfo): DimensionInfo wrapper for dimensions
Returns:
output of shape `[batch_sizes, length, features]`. where length will be padded to a multiple of block_size
"""
# [batch_size, num_heads, global_len+padded_seq_len, dim_per_head]
global_and_local_k = torch.cat([global_k, local_k], dim=2)
# [batch_size, num_heads, global_len+padded_seq_len, dim_per_head]
global_and_local_v = torch.cat([global_v, local_v], dim=2)
# [batch_size, global_len+padded_seq_len]
extended_mask = nn.functional.pad(mask, pad=(dim.global_len, 0), value=0)
# [batch_size, num_heads, global_len, global_len+padded_seq_len]
attn_weights = torch.einsum("BHGF,BHXF->BHGX", global_q, global_and_local_k)
attn_weights = attn_weights + extended_mask[:, None, None, :]
attn_probs = nn.functional.softmax(attn_weights, dim=-1)
attn_probs = nn.functional.dropout(attn_probs, p=self.dropout, training=self.training)
# [batch_size, num_heads, global_len, F]
attn_output = torch.einsum("BHGX,BHXF->BHGF", attn_probs, global_and_local_v)
return attn_output, attn_probs
def compute_local_attention_representations(
self, global_k, global_v, local_q, local_k, local_v, mask, dim: DimensionInfo
):
"""Compute attention representations for local tokens.
Local tokens will attend to both global tokens as well as all other tokens within the same local block. Hence,
we need to tile and concatenate the global tokens to every local block
Args:
global_k (`torch.FloatTensor`) of shape [batch_size, num_heads, global_len, dim_per_head]:
key vectors from global tokens
global_v (`torch.FloatTensor`) of shape [batch_size, num_heads, global_len, dim_per_head]:
value vectors from global tokens
local_q (`torch.FloatTensor`) of shape [batch_size, num_heads, padded_seq_len, dim_per_head]:
query vectors from local tokens
local_k (`torch.FloatTensor`) of shape [batch_size, num_heads, padded_seq_len, dim_per_head]:
key vectors from local tokens
local_v (`torch.FloatTensor`) of shape [batch_size, num_heads, padded_seq_len, dim_per_head]:
value vectors from local tokens
mask (`torch.FloatTensor`) of shape [batch_size, padded_seq_len]: attention mask
dim (DimensionInfo): DimensionInfo wrapper for dimensions
Returns:
output of shape `[batch_sizes, length, features]`. where length will be padded to a multiple of block_size
"""
# [batch_size, num_heads, num_blocks, block_size, dim_per_head]
blocked_local_q = local_q.view(dim.batch_size, dim.num_heads, dim.num_blocks, dim.block_size, dim.dim_per_head)
# [batch_size, num_heads, num_blocks, block_size, dim_per_head]
blocked_local_k = local_k.view(dim.batch_size, dim.num_heads, dim.num_blocks, dim.block_size, dim.dim_per_head)
# [batch_size, num_heads, num_blocks, block_size, dim_per_head]
blocked_local_v = local_v.view(dim.batch_size, dim.num_heads, dim.num_blocks, dim.block_size, dim.dim_per_head)
# [batch_size, num_blocks, global_len+block_size]
extended_mask = nn.functional.pad(
mask.view(dim.batch_size, dim.num_blocks, dim.block_size),
pad=(dim.global_len, 0),
value=0,
)
# [batch_size, num_heads, num_blocks, block_size, global_len]
blocked_local2global = torch.einsum("BHNKF,BHGF->BHNKG", blocked_local_q, global_k)
# [batch_size, num_heads, num_blocks, block_size, block_size]
blocked_local2local = torch.einsum("BHNKF,BHNXF->BHNKX", blocked_local_q, blocked_local_k)
# [batch_size, num_heads, num_blocks, block_size, global_len+block_size]
attn_weights = torch.cat([blocked_local2global, blocked_local2local], dim=-1)
attn_weights = attn_weights + extended_mask[:, None, :, None, :]
attn_probs = nn.functional.softmax(attn_weights, dim=-1)
attn_probs = nn.functional.dropout(attn_probs, p=self.dropout, training=self.training)
# [batch_size, num_heads, num_blocks, block_size, global_len]
local2global_attn_probs = attn_probs[:, :, :, :, : dim.global_len]
# [batch_size, num_heads, num_blocks, block_size, block_size]
local2local_attn_probs = attn_probs[:, :, :, :, dim.global_len :]
# [batch_size, num_heads, num_blocks, block_size, dim_per_head]
local2global_attn_output = torch.einsum("BHNKG,BHGF->BHNKF", local2global_attn_probs, global_v)
# [batch_size, num_heads, num_blocks, block_size, dim_per_head]
local2local_attn_output = torch.einsum("BHNKX,BHNXF->BHNKF", local2local_attn_probs, blocked_local_v)
# [batch_size, num_heads, num_blocks, block_size, dim_per_head]
attn_output = local2global_attn_output + local2local_attn_output
return attn_output, attn_probs
class PegasusXEncoderLayer(nn.Module):
def __init__(self, stagger_blocks_this_layer: bool, config: PegasusXConfig):
super().__init__()
self.embed_dim = config.d_model
self.self_attn = PegasusXGlobalLocalAttention(
embed_dim=self.embed_dim,
num_heads=config.encoder_attention_heads,
block_size=config.block_size,
dropout=config.attention_dropout,
)
self.self_attn_layer_norm = nn.LayerNorm(self.embed_dim)
self.global_self_attn_layer_norm = nn.LayerNorm(self.embed_dim)
self.dropout = config.dropout
self.activation_fn = ACT2FN[config.activation_function]
self.activation_dropout = config.activation_dropout
self.fc1 = nn.Linear(self.embed_dim, config.encoder_ffn_dim)
self.fc2 = nn.Linear(config.encoder_ffn_dim, self.embed_dim)
self.final_layer_norm = nn.LayerNorm(self.embed_dim)
self.stagger_blocks_this_layer = stagger_blocks_this_layer
self.block_size = config.block_size
def forward(
self,
hidden_states: torch.Tensor,
global_hidden_states: torch.Tensor,
attention_mask: torch.Tensor,
output_attentions: bool = False,
) -> torch.Tensor:
"""
Args:
hidden_states (`torch.FloatTensor`): input to the layer of shape *(seq_len, batch, embed_dim)*
global_hidden_states (`torch.FloatTensor`): global token hidden states
*(seq_len, num_global_tokens, embed_dim)*
attention_mask (`torch.FloatTensor`): attention mask of size
*(batch, 1, tgt_len, src_len)* where padding elements are indicated by very large negative values.
output_attentions (`bool`, *optional*):
Whether or not to return the attentions tensors of all attention layers. See `attentions` under
returned tensors for more detail.
"""
residual = hidden_states
global_residual = global_hidden_states
hidden_states = self.self_attn_layer_norm(hidden_states)
global_hidden_states = self.global_self_attn_layer_norm(global_hidden_states)
if self.stagger_blocks_this_layer:
# Pad the blocks to simulate staggering
hidden_states, attention_mask = self.pad_local_tokens(
hidden_states=hidden_states, attention_mask=attention_mask, block_size=self.block_size
)
hidden_states, global_hidden_states, attn_weights = self.self_attn(
token_hidden_states=hidden_states,
global_hidden_states=global_hidden_states,
attention_mask=attention_mask,
output_attentions=output_attentions,
)
if self.stagger_blocks_this_layer:
# Undo the padding
hidden_states = self.unpad_local_tokens(padded_hidden_states=hidden_states, block_size=self.block_size)
hidden_states = nn.functional.dropout(hidden_states, p=self.dropout, training=self.training)
hidden_states = residual + hidden_states
global_hidden_states = nn.functional.dropout(global_hidden_states, p=self.dropout, training=self.training)
global_hidden_states = global_residual + global_hidden_states
residual = hidden_states
hidden_states = self.final_layer_norm(hidden_states)
hidden_states = self.activation_fn(self.fc1(hidden_states))
hidden_states = nn.functional.dropout(hidden_states, p=self.activation_dropout, training=self.training)
hidden_states = self.fc2(hidden_states)
hidden_states = nn.functional.dropout(hidden_states, p=self.dropout, training=self.training)
hidden_states = residual + hidden_states
global_residual = global_hidden_states
global_hidden_states = self.final_layer_norm(global_hidden_states)
global_hidden_states = self.activation_fn(self.fc1(global_hidden_states))
global_hidden_states = nn.functional.dropout(
global_hidden_states, p=self.activation_dropout, training=self.training
)
global_hidden_states = self.fc2(global_hidden_states)
global_hidden_states = nn.functional.dropout(global_hidden_states, p=self.dropout, training=self.training)
global_hidden_states = global_residual + global_hidden_states
outputs = (hidden_states, global_hidden_states)
if output_attentions:
outputs += (attn_weights,)
return outputs
@classmethod
def pad_local_tokens(cls, hidden_states, attention_mask, block_size):
# hidden_states: [batch_size, seq_len, hidden_dim]
pad_size = block_size // 2
mask_min_value = torch.finfo(hidden_states.dtype).min
padded_hidden_states = torch.nn.functional.pad(
hidden_states,
pad=(0, 0, pad_size, pad_size),
)
padded_mask = torch.nn.functional.pad(
attention_mask,
pad=(pad_size, pad_size),
value=mask_min_value,
)
return padded_hidden_states, padded_mask
@classmethod
def unpad_local_tokens(cls, padded_hidden_states, block_size):
# padded_hidden_states: [batch_size, padded seq_len, hidden_dim]
pad_size = block_size // 2
return padded_hidden_states[:, pad_size:-pad_size, :]
class PegasusXDecoderLayer(nn.Module):
def __init__(self, config: PegasusXConfig):
super().__init__()
self.embed_dim = config.d_model
self.self_attn = PegasusXAttention(
embed_dim=self.embed_dim,
num_heads=config.decoder_attention_heads,
dropout=config.attention_dropout,
is_decoder=True,
bias=False,
)
self.dropout = config.dropout
self.activation_fn = ACT2FN[config.activation_function]
self.activation_dropout = config.activation_dropout
self.self_attn_layer_norm = nn.LayerNorm(self.embed_dim)
self.encoder_attn = PegasusXAttention(
self.embed_dim,
config.decoder_attention_heads,
dropout=config.attention_dropout,
is_decoder=True,
bias=False,
)
self.encoder_attn_layer_norm = nn.LayerNorm(self.embed_dim)
self.fc1 = nn.Linear(self.embed_dim, config.decoder_ffn_dim)
self.fc2 = nn.Linear(config.decoder_ffn_dim, self.embed_dim)
self.final_layer_norm = nn.LayerNorm(self.embed_dim)
def forward(
self,
hidden_states: torch.Tensor,
attention_mask: Optional[torch.Tensor] = None,
encoder_hidden_states: Optional[torch.Tensor] = None,
encoder_attention_mask: Optional[torch.Tensor] = None,
past_key_value: Optional[Tuple[torch.Tensor]] = None,
output_attentions: Optional[bool] = False,
use_cache: Optional[bool] = True,
) -> torch.Tensor:
"""
Args:
hidden_states (`torch.FloatTensor`): input to the layer of shape *(seq_len, batch, embed_dim)*
attention_mask (`torch.FloatTensor`): attention mask of size
*(batch, 1, tgt_len, src_len)* where padding elements are indicated by very large negative values.
encoder_hidden_states (`torch.FloatTensor`):
cross attention input to the layer of shape *(seq_len, batch, embed_dim)*
encoder_attention_mask (`torch.FloatTensor`): encoder attention mask of size
*(batch, 1, tgt_len, src_len)* where padding elements are indicated by very large negative values.
past_key_value (`Tuple(torch.FloatTensor)`): cached past key and value projection states
output_attentions (`bool`, *optional*):
Whether or not to return the attentions tensors of all attention layers. See `attentions` under
returned tensors for more detail.
use_cache: Whether to us KV cache for decoding
"""
residual = hidden_states
hidden_states = self.self_attn_layer_norm(hidden_states)
# Self Attention
# decoder uni-directional self-attention cached key/values tuple is at positions 1,2
self_attn_past_key_value = past_key_value[:2] if past_key_value is not None else None
# add present self-attn cache to positions 1,2 of present_key_value tuple
hidden_states, self_attn_weights, present_key_value = self.self_attn(
hidden_states=hidden_states,
past_key_value=self_attn_past_key_value,
attention_mask=attention_mask,
output_attentions=output_attentions,
)
hidden_states = nn.functional.dropout(hidden_states, p=self.dropout, training=self.training)
hidden_states = residual + hidden_states
# Cross-Attention Block
cross_attn_present_key_value = None
cross_attn_weights = None
if encoder_hidden_states is not None:
residual = hidden_states
hidden_states = self.encoder_attn_layer_norm(hidden_states)
# cross_attn cached key/values tuple is at positions 3,4 of present_key_value tuple
cross_attn_past_key_value = past_key_value[-2:] if past_key_value is not None else None
hidden_states, cross_attn_weights, cross_attn_present_key_value = self.encoder_attn(
hidden_states=hidden_states,
key_value_states=encoder_hidden_states,
attention_mask=encoder_attention_mask,
past_key_value=cross_attn_past_key_value,
output_attentions=output_attentions,
)
hidden_states = nn.functional.dropout(hidden_states, p=self.dropout, training=self.training)
hidden_states = residual + hidden_states
# add cross-attn to positions 3,4 of present_key_value tuple
present_key_value = present_key_value + cross_attn_present_key_value
# Fully Connected
residual = hidden_states
hidden_states = self.final_layer_norm(hidden_states)
hidden_states = self.activation_fn(self.fc1(hidden_states))
hidden_states = nn.functional.dropout(hidden_states, p=self.activation_dropout, training=self.training)
hidden_states = self.fc2(hidden_states)
hidden_states = nn.functional.dropout(hidden_states, p=self.dropout, training=self.training)
hidden_states = residual + hidden_states
outputs = (hidden_states,)
if output_attentions:
outputs += (self_attn_weights, cross_attn_weights)
if use_cache:
outputs += (present_key_value,)
return outputs
class PegasusXPreTrainedModel(PreTrainedModel):
config_class = PegasusXConfig
base_model_prefix = "model"
supports_gradient_checkpointing = True
_no_split_modules = [r"PegasusXEncoderLayer", r"PegasusXDecoderLayer"]
def _init_weights(self, module):
std = self.config.init_std
if isinstance(module, nn.Linear):
module.weight.data.normal_(mean=0.0, std=std)
if module.bias is not None:
module.bias.data.zero_()
elif isinstance(module, nn.Embedding):
module.weight.data.normal_(mean=0.0, std=std)
PEGASUS_X_START_DOCSTRING = r"""
This model inherits from [`PreTrainedModel`]. Check the superclass documentation for the generic methods the
library implements for all its model (such as downloading or saving, resizing the input embeddings, pruning heads
etc.)
This model is also a PyTorch [torch.nn.Module](https://pytorch.org/docs/stable/nn.html#torch.nn.Module) subclass.
Use it as a regular PyTorch Module and refer to the PyTorch documentation for all matter related to general usage
and behavior.
Parameters:
config ([`PegasusXConfig`]):
Model configuration class with all the parameters of the model. Initializing with a config file does not
load the weights associated with the model, only the configuration. Check out the
[`~PreTrainedModel.from_pretrained`] method to load the model weights.
"""
PEGASUS_X_GENERATION_EXAMPLE = r"""
Summarization example:
```python
>>> from transformers import AutoTokenizer, PegasusXForConditionalGeneration
>>> model = PegasusXForConditionalGeneration.from_pretrained("google/pegasus-x-base")
>>> tokenizer = AutoTokenizer.from_pretrained("google/pegasus-x-large")
>>> ARTICLE_TO_SUMMARIZE = (
... "PG&E stated it scheduled the blackouts in response to forecasts for high winds "
... "amid dry conditions. The aim is to reduce the risk of wildfires. Nearly 800 thousand customers were "
... "scheduled to be affected by the shutoffs which were expected to last through at least midday tomorrow."
... )
>>> inputs = tokenizer(ARTICLE_TO_SUMMARIZE, max_length=1024, return_tensors="pt")
>>> # Generate Summary
>>> summary_ids = model.generate(inputs["input_ids"])
>>> tokenizer.batch_decode(summary_ids, skip_special_tokens=True, clean_up_tokenization_spaces=False)[0]
"California's largest electricity provider has turned off power to hundreds of thousands of customers."
```
"""
PEGASUS_X_INPUTS_DOCSTRING = r"""
Args:
input_ids (`torch.LongTensor` of shape `(batch_size, sequence_length)`):
Indices of input sequence tokens in the vocabulary. Padding will be ignored by default should you provide
it.
Indices can be obtained using [`AutoTokenizer`]. See [`PreTrainedTokenizer.encode`] and
[`PreTrainedTokenizer.__call__`] for details.
[What are input IDs?](../glossary#input-ids)
inputs_embeds (`torch.FloatTensor` of shape `(batch_size, sequence_length, hidden_size)`, *optional*):
Optionally, instead of passing `input_ids` you can choose to directly pass an embedded representation.
attention_mask (`torch.Tensor` of shape `(batch_size, sequence_length)`, *optional*):
Mask to avoid performing attention on padding token indices. Mask values selected in `[0, 1]`:
- 1 for tokens that are **not masked**,
- 0 for tokens that are **masked**.
[What are attention masks?](../glossary#attention-mask)
decoder_input_ids (`torch.LongTensor` of shape `(batch_size, target_sequence_length)`, *optional*):
Indices of decoder input sequence tokens in the vocabulary.
Indices can be obtained using [`AutoTokenizer`]. See [`PreTrainedTokenizer.encode`] and
[`PreTrainedTokenizer.__call__`] for details.
[What are decoder input IDs?](../glossary#decoder-input-ids)
PEGASUS-X uses the `pad_token_id` as the starting token for `decoder_input_ids` generation. If
`past_key_values` is used, optionally only the last `decoder_input_ids` have to be input (see
`past_key_values`).
decoder_attention_mask (`torch.LongTensor` of shape `(batch_size, target_sequence_length)`, *optional*):
Default behavior: generate a tensor that ignores pad tokens in `decoder_input_ids`. Causal mask will also
be used by default.
encoder_outputs (`tuple(tuple(torch.FloatTensor)`, *optional*):
Tuple consists of (`last_hidden_state`, *optional*: `hidden_states`, *optional*: `attentions`)
`last_hidden_state` of shape `(batch_size, sequence_length, hidden_size)`, *optional*) is a sequence of
hidden-states at the output of the last layer of the encoder. Used in the cross-attention of the decoder.
past_key_values (`tuple(tuple(torch.FloatTensor))`, *optional*, returned when `use_cache=True` is passed or when `config.use_cache=True`):
Tuple of `tuple(torch.FloatTensor)` of length `config.n_layers`, with each tuple having 2 tensors of shape
`(batch_size, num_heads, sequence_length, embed_size_per_head)`) and 2 additional tensors of shape
`(batch_size, num_heads, encoder_sequence_length, embed_size_per_head)`.
Contains pre-computed hidden-states (key and values in the self-attention blocks and in the cross-attention
blocks) that can be used (see `past_key_values` input) to speed up sequential decoding.
If `past_key_values` are used, the user can optionally input only the last `decoder_input_ids` (those that
don't have their past key value states given to this model) of shape `(batch_size, 1)` instead of all
`decoder_input_ids` of shape `(batch_size, sequence_length)`.
inputs_embeds (`torch.FloatTensor` of shape `(batch_size, sequence_length, hidden_size)`, *optional*):
Optionally, instead of passing `input_ids` you can choose to directly pass an embedded representation.
This is useful if you want more control over how to convert `input_ids` indices into associated vectors
than the model's internal embedding lookup matrix.
decoder_inputs_embeds (`torch.FloatTensor` of shape `(batch_size, target_sequence_length, hidden_size)`, *optional*):
Optionally, instead of passing `decoder_input_ids` you can choose to directly pass an embedded
representation. If `past_key_values` is used, optionally only the last `decoder_inputs_embeds` have to be
input (see `past_key_values`). This is useful if you want more control over how to convert
`decoder_input_ids` indices into associated vectors than the model's internal embedding lookup matrix.
If `decoder_input_ids` and `decoder_inputs_embeds` are both unset, `decoder_inputs_embeds` takes the value
of `inputs_embeds`.
use_cache (`bool`, *optional*):
If set to `True`, `past_key_values` key value states are returned and can be used to speed up decoding (see
`past_key_values`).
output_attentions (`bool`, *optional*):
Whether or not to return the attentions tensors of all attention layers. See `attentions` under returned
tensors for more detail.
output_hidden_states (`bool`, *optional*):
Whether or not to return the hidden states of all layers. See `hidden_states` under returned tensors for
more detail.
return_dict (`bool`, *optional*):
Whether or not to return a [`~utils.ModelOutput`] instead of a plain tuple.
"""
class PegasusXEncoder(PegasusXPreTrainedModel):
"""
Transformer encoder consisting of *config.encoder_layers* self attention layers. Each layer is a
[`PegasusXEncoderLayer`].
Args:
config: PegasusXConfig
embed_tokens (nn.Embedding): output embedding
"""
def __init__(self, config: PegasusXConfig, embed_tokens: Optional[nn.Embedding] = None):
super().__init__(config)
self.dropout = config.dropout
self.layerdrop = config.encoder_layerdrop
embed_dim = config.d_model
self.max_source_positions = config.max_position_embeddings
self.embed_scale = math.sqrt(embed_dim) if config.scale_embedding else 1.0
if embed_tokens is not None:
self.embed_tokens = embed_tokens
else:
self.embed_tokens = nn.Embedding(config.vocab_size, embed_dim)
self.embed_global = nn.Embedding(config.num_global_tokens, embed_dim)
self.embed_positions = PegasusXSinusoidalPositionalEmbedding(embed_dim)
self.layers = nn.ModuleList(
[
PegasusXEncoderLayer(
stagger_blocks_this_layer=i % 2 == 1 and config.stagger_local_blocks, config=config
)
for i in range(config.encoder_layers)
]
)
self.layer_norm = nn.LayerNorm(config.d_model)
self.gradient_checkpointing = False
# Initialize weights and apply final processing
self.post_init()
def resize_position_embeddings(self, new_num_position_embeddings: int):
"""
Resizes position embeddings matrix of the model if `new_num_position_embeddings !=
config.max_position_embeddings`.
Arguments:
new_num_position_embeddings (`int`):
The number of new position embeddings. If position embeddings are learned, increasing the size will add
newly initialized vectors at the end, whereas reducing the size will remove vectors from the end. If
position embeddings are not learned (*e.g.* sinusoidal position embeddings), increasing the size will
add correct vectors at the end following the position encoding algorithm, whereas reducing the size
will remove vectors from the end.
"""
logger.info(f"Setting `config.max_position_embeddings={new_num_position_embeddings}`...")
self.config.max_position_embeddings = new_num_position_embeddings
self.embed_positions = PegasusXSinusoidalPositionalEmbedding(self.config.d_model)
self.embed_positions.to(self.device)
def get_position_embeddings(self) -> nn.Embedding:
"""
Returns the position embeddings matrix
"""
return self.embed_positions
def forward(
self,
input_ids=None,
attention_mask=None,
inputs_embeds=None,
output_attentions=None,
output_hidden_states=None,
return_dict=None,
):
r"""
Args:
input_ids (`torch.LongTensor` of shape `(batch_size, sequence_length)`):
Indices of input sequence tokens in the vocabulary. Padding will be ignored by default should you
provide it.
Indices can be obtained using [`AutoTokenizer`]. See [`PreTrainedTokenizer.encode`] and
[`PreTrainedTokenizer.__call__`] for details.
[What are input IDs?](../glossary#input-ids)
attention_mask (`torch.Tensor` of shape `(batch_size, sequence_length)`, *optional*):
Mask to avoid performing attention on padding token indices. Mask values selected in `[0, 1]`:
- 1 for tokens that are **not masked**,
- 0 for tokens that are **masked**.
[What are attention masks?](../glossary#attention-mask)
inputs_embeds (`torch.FloatTensor` of shape `(batch_size, sequence_length, hidden_size)`, *optional*):
Optionally, instead of passing `input_ids` you can choose to directly pass an embedded representation.
This is useful if you want more control over how to convert `input_ids` indices into associated vectors
than the model's internal embedding lookup matrix.
output_attentions (`bool`, *optional*):
Whether or not to return the attentions tensors of all attention layers. See `attentions` under
returned tensors for more detail.
output_hidden_states (`bool`, *optional*):
Whether or not to return the hidden states of all layers. See `hidden_states` under returned tensors
for more detail.
return_dict (`bool`, *optional*):
Whether or not to return a [`~utils.ModelOutput`] instead of a plain tuple.
"""
output_attentions = output_attentions if output_attentions is not None else self.config.output_attentions
output_hidden_states = (
output_hidden_states if output_hidden_states is not None else self.config.output_hidden_states
)
return_dict = return_dict if return_dict is not None else self.config.use_return_dict
# retrieve input_ids and inputs_embeds
if input_ids is not None and inputs_embeds is not None:
raise ValueError("You cannot specify both input_ids and inputs_embeds at the same time")
elif input_ids is not None:
self.warn_if_padding_and_no_attention_mask(input_ids, attention_mask)
input_shape = input_ids.size()
input_ids = input_ids.view(-1, input_shape[-1])
elif inputs_embeds is not None:
input_shape = inputs_embeds.size()[:-1]
else:
raise ValueError("You have to specify either input_ids or inputs_embeds")
if inputs_embeds is None:
inputs_embeds = self.embed_tokens(input_ids) * self.embed_scale
embed_pos = self.embed_positions(inputs_embeds)
hidden_states = inputs_embeds + embed_pos
hidden_states = nn.functional.dropout(hidden_states, p=self.dropout, training=self.training)
batch_size, seq_len, _ = hidden_states.shape
# Setup mask
if attention_mask is None:
attention_mask = torch.ones(*input_shape, dtype=inputs_embeds.dtype, device=inputs_embeds.device)
attention_mask = attention_mask.to(dtype=hidden_states.dtype)
mask_min_value = torch.finfo(hidden_states.dtype).min
inverted_mask = 1.0 - attention_mask
attention_mask = inverted_mask.masked_fill(
inverted_mask.to(torch.bool),
mask_min_value,
)
# padding to block_size
if seq_len % self.config.block_size != 0:
pad_len = self.config.block_size - seq_len % self.config.block_size
hidden_states = nn.functional.pad(hidden_states, pad=(0, 0, 0, pad_len), value=0)
attention_mask = nn.functional.pad(attention_mask, pad=(0, pad_len), value=mask_min_value)
# Global tokens
global_hidden_states = self.embed_global(
torch.arange(self.config.num_global_tokens, device=hidden_states.device)[None].expand(batch_size, -1)
)
encoder_states = () if output_hidden_states else None
all_attentions = () if output_attentions else None
for idx, encoder_layer in enumerate(self.layers):
if output_hidden_states:
encoder_states = encoder_states + (hidden_states,)
# add LayerDrop (see https://arxiv.org/abs/1909.11556 for description)
to_drop = False
if self.training:
dropout_probability = torch.rand([])
if dropout_probability < self.layerdrop: # skip the layer
to_drop = True
if to_drop:
layer_outputs = (None, None)
else:
if self.gradient_checkpointing and self.training:
layer_outputs = self._gradient_checkpointing_func(
encoder_layer.__call__,
hidden_states,
global_hidden_states,
attention_mask,
output_attentions,
)
else:
layer_outputs = encoder_layer(
hidden_states,
global_hidden_states,
attention_mask,
output_attentions=output_attentions,
)
hidden_states = layer_outputs[0]
global_hidden_states = layer_outputs[1]
if output_attentions:
all_attentions = all_attentions + (layer_outputs[2],)
# Undo padding-to-block-size
hidden_states = hidden_states[:, :seq_len]
hidden_states = self.layer_norm(hidden_states)
if output_hidden_states:
encoder_states = encoder_states + ((hidden_states, global_hidden_states),)
if not return_dict:
return tuple(v for v in [hidden_states, encoder_states, all_attentions] if v is not None)
return BaseModelOutput(
last_hidden_state=hidden_states, hidden_states=encoder_states, attentions=all_attentions
)
class PegasusXDecoder(PegasusXPreTrainedModel):
"""
Transformer decoder consisting of *config.decoder_layers* layers. Each layer is a [`PegasusDecoderLayer`]
Args:
config: PegasusXConfig
embed_tokens (nn.Embedding): output embedding
"""
def __init__(self, config: PegasusXConfig, embed_tokens: Optional[nn.Embedding] = None):
super().__init__(config)
self.dropout = config.dropout
self.layerdrop = config.decoder_layerdrop
self.max_target_positions = config.max_position_embeddings
self.embed_scale = math.sqrt(config.d_model) if config.scale_embedding else 1.0
if embed_tokens is not None:
self.embed_tokens = embed_tokens
else:
self.embed_tokens = nn.Embedding(config.vocab_size, config.d_model)
self.embed_positions = PegasusXSinusoidalPositionalEmbedding(config.d_model)
self.layers = nn.ModuleList([PegasusXDecoderLayer(config) for _ in range(config.decoder_layers)])
self.layer_norm = nn.LayerNorm(config.d_model)
self.gradient_checkpointing = False
# Initialize weights and apply final processing
self.post_init()
def get_input_embeddings(self):
return self.embed_tokens
def set_input_embeddings(self, value):
self.embed_tokens = value
def forward(
self,
input_ids=None,
attention_mask=None,
encoder_hidden_states=None,
encoder_attention_mask=None,
past_key_values=None,
inputs_embeds=None,
use_cache=None,
output_attentions=None,
output_hidden_states=None,
return_dict=None,
):
r"""
Args:
input_ids (`torch.LongTensor` of shape `(batch_size, sequence_length)`):
Indices of input sequence tokens in the vocabulary. Padding will be ignored by default should you
provide it.
Indices can be obtained using [`AutoTokenizer`]. See [`PreTrainedTokenizer.encode`] and
[`PreTrainedTokenizer.__call__`] for details.
[What are input IDs?](../glossary#input-ids)
attention_mask (`torch.Tensor` of shape `(batch_size, sequence_length)`, *optional*):
Mask to avoid performing attention on padding token indices. Mask values selected in `[0, 1]`:
- 1 for tokens that are **not masked**,
- 0 for tokens that are **masked**.
[What are attention masks?](../glossary#attention-mask)
encoder_hidden_states (`torch.FloatTensor` of shape `(batch_size, encoder_sequence_length, hidden_size)`, *optional*):
Sequence of hidden-states at the output of the last layer of the encoder. Used in the cross-attention
of the decoder.
encoder_attention_mask (`torch.LongTensor` of shape `(batch_size, encoder_sequence_length)`, *optional*):
Mask to avoid performing cross-attention on padding tokens indices of encoder input_ids. Mask values
selected in `[0, 1]`:
- 1 for tokens that are **not masked**,
- 0 for tokens that are **masked**.
[What are attention masks?](../glossary#attention-mask)
past_key_values (`tuple(tuple(torch.FloatTensor))`, *optional*, returned when `use_cache=True` is passed or when `config.use_cache=True`):
Tuple of `tuple(torch.FloatTensor)` of length `config.n_layers`, with each tuple having 2 tensors of
shape `(batch_size, num_heads, sequence_length, embed_size_per_head)`) and 2 additional tensors of
shape `(batch_size, num_heads, encoder_sequence_length, embed_size_per_head)`.
Contains pre-computed hidden-states (key and values in the self-attention blocks and in the
cross-attention blocks) that can be used (see `past_key_values` input) to speed up sequential decoding.
If `past_key_values` are used, the user can optionally input only the last `decoder_input_ids` (those
that don't have their past key value states given to this model) of shape `(batch_size, 1)` instead of
all `decoder_input_ids` of shape `(batch_size, sequence_length)`.
inputs_embeds (`torch.FloatTensor` of
shape `(batch_size, sequence_length, hidden_size)`, *optional*): Optionally, instead of passing
`input_ids` you can choose to directly pass an embedded representation. This is useful if you want more
control over how to convert `input_ids` indices into associated vectors than the model's internal
embedding lookup matrix.
output_attentions (`bool`, *optional*):
Whether or not to return the attentions tensors of all attention layers. See `attentions` under
returned tensors for more detail.
output_hidden_states (`bool`, *optional*):
Whether or not to return the hidden states of all layers. See `hidden_states` under returned tensors
for more detail.
return_dict (`bool`, *optional*):
Whether or not to return a [`~utils.ModelOutput`] instead of a plain tuple.
"""
output_attentions = output_attentions if output_attentions is not None else self.config.output_attentions
output_hidden_states = (
output_hidden_states if output_hidden_states is not None else self.config.output_hidden_states
)
use_cache = use_cache if use_cache is not None else self.config.use_cache
return_dict = return_dict if return_dict is not None else self.config.use_return_dict
# retrieve input_ids and inputs_embeds
if input_ids is not None and inputs_embeds is not None:
raise ValueError("You cannot specify both decoder_input_ids and decoder_inputs_embeds at the same time")
elif input_ids is not None:
input_shape = input_ids.size()
input_ids = input_ids.view(-1, input_shape[-1])
elif inputs_embeds is not None:
input_shape = inputs_embeds.size()[:-1]
else:
raise ValueError("You have to specify either decoder_input_ids or decoder_inputs_embeds")
# past_key_values_length
past_key_values_length = past_key_values[0][0].shape[2] if past_key_values is not None else 0
if inputs_embeds is None:
inputs_embeds = self.embed_tokens(input_ids) * self.embed_scale
attention_mask = _prepare_4d_causal_attention_mask(
attention_mask, input_shape, inputs_embeds, past_key_values_length
)
# expand encoder attention mask
if encoder_hidden_states is not None and encoder_attention_mask is not None:
# [bsz, seq_len] -> [bsz, 1, tgt_seq_len, src_seq_len]
encoder_attention_mask = _prepare_4d_attention_mask(
encoder_attention_mask, inputs_embeds.dtype, tgt_len=input_shape[-1]
)
# embed positions
positions = self.embed_positions(inputs_embeds, past_key_values_length)
positions = positions.to(inputs_embeds.device)
hidden_states = inputs_embeds + positions
hidden_states = nn.functional.dropout(hidden_states, p=self.dropout, training=self.training)
if self.gradient_checkpointing and self.training:
if use_cache:
logger.warning_once(
"`use_cache=True` is incompatible with gradient checkpointing. Setting `use_cache=False`..."
)
use_cache = False
# decoder layers
all_hidden_states = () if output_hidden_states else None
all_self_attns = () if output_attentions else None
all_cross_attentions = () if (output_attentions and encoder_hidden_states is not None) else None
next_decoder_cache = () if use_cache else None
for idx, decoder_layer in enumerate(self.layers):
# add LayerDrop (see https://arxiv.org/abs/1909.11556 for description)
if output_hidden_states:
all_hidden_states += (hidden_states,)
if self.training:
dropout_probability = torch.rand([])
if dropout_probability < self.layerdrop:
continue
past_key_value = past_key_values[idx] if past_key_values is not None else None
if self.gradient_checkpointing and self.training:
layer_outputs = self._gradient_checkpointing_func(
decoder_layer.__call__,
hidden_states,
attention_mask,
encoder_hidden_states,
encoder_attention_mask,
None,
output_attentions,
use_cache,
)
else:
layer_outputs = decoder_layer(
hidden_states,
attention_mask=attention_mask,
encoder_hidden_states=encoder_hidden_states,
encoder_attention_mask=encoder_attention_mask,
past_key_value=past_key_value,
output_attentions=output_attentions,
use_cache=use_cache,
)
hidden_states = layer_outputs[0]
if use_cache:
next_decoder_cache += (layer_outputs[3 if output_attentions else 1],)
if output_attentions:
all_self_attns += (layer_outputs[1],)
if encoder_hidden_states is not None:
all_cross_attentions += (layer_outputs[2],)
hidden_states = self.layer_norm(hidden_states)
# add hidden states from the last decoder layer
if output_hidden_states:
all_hidden_states += (hidden_states,)
next_cache = next_decoder_cache if use_cache else None
if not return_dict:
return tuple(
v
for v in [hidden_states, next_cache, all_hidden_states, all_self_attns, all_cross_attentions]
if v is not None
)
return BaseModelOutputWithPastAndCrossAttentions(
last_hidden_state=hidden_states,
past_key_values=next_cache,
hidden_states=all_hidden_states,
attentions=all_self_attns,
cross_attentions=all_cross_attentions,
)
@add_start_docstrings(
"The bare PEGASUS-X Model outputting raw hidden-states without any specific head on top.",
PEGASUS_X_START_DOCSTRING,
)
class PegasusXModel(PegasusXPreTrainedModel):
_tied_weights_keys = ["encoder.embed_tokens.weight", "decoder.embed_tokens.weight"]
def __init__(self, config: PegasusXConfig):
super().__init__(config)
vocab_size = config.vocab_size
self.shared = nn.Embedding(vocab_size, config.d_model)
self.encoder = PegasusXEncoder(config, self.shared)
self.decoder = PegasusXDecoder(config, self.shared)
# Initialize weights and apply final processing
self.post_init()
def get_input_embeddings(self):
return self.shared
def set_input_embeddings(self, value):
self.shared = value
self.encoder.embed_tokens = self.shared
self.decoder.embed_tokens = self.shared
def get_encoder(self):
return self.encoder
def get_decoder(self):
return self.decoder
def resize_position_embeddings(self, new_num_position_embeddings: int):
"""
Resizes position embeddings matrix of the model if `new_num_position_embeddings !=
config.max_position_embeddings`.
Arguments:
new_num_position_embeddings (`int`):
The number of new position embeddings. If position embeddings are learned, increasing the size will add
newly initialized vectors at the end, whereas reducing the size will remove vectors from the end. If
position embeddings are not learned (*e.g.* sinusoidal position embeddings), increasing the size will
add correct vectors at the end following the position encoding algorithm, whereas reducing the size
will remove vectors from the end.
"""
self.config.max_position_embeddings = new_num_position_embeddings
self.encoder.resize_position_embeddings(new_num_position_embeddings)
self.decoder.resize_position_embeddings(new_num_position_embeddings)
def get_position_embeddings(self) -> Tuple[nn.Embedding]:
"""
Returns the position embeddings matrix
"""
return (self.encoder.get_position_embeddings(), self.decoder.get_position_embeddings())
@add_start_docstrings_to_model_forward(PEGASUS_X_INPUTS_DOCSTRING)
@replace_return_docstrings(output_type=Seq2SeqModelOutput, config_class=_CONFIG_FOR_DOC)
def forward(
self,
input_ids: Optional[torch.Tensor] = None,
attention_mask: Optional[torch.Tensor] = None,
decoder_input_ids: Optional[torch.Tensor] = None,
decoder_attention_mask: Optional[torch.Tensor] = None,
encoder_outputs: Optional[Tuple[torch.FloatTensor]] = None,
past_key_values: Optional[Tuple[torch.FloatTensor]] = None,
inputs_embeds: Optional[torch.Tensor] = None,
decoder_inputs_embeds: Optional[torch.Tensor] = None,
use_cache: Optional[bool] = None,
output_attentions: Optional[bool] = None,
output_hidden_states: Optional[bool] = None,
return_dict: Optional[bool] = None,
) -> Union[Tuple, Seq2SeqModelOutput]:
r"""
Returns:
Example:
```python
>>> from transformers import AutoTokenizer, PegasusModel
>>> tokenizer = AutoTokenizer.from_pretrained("google/pegasus-x-large")
>>> model = PegasusModel.from_pretrained("google/pegasus-x-large")
>>> inputs = tokenizer("Studies have been shown that owning a dog is good for you", return_tensors="pt")
>>> decoder_inputs = tokenizer("Studies show that", return_tensors="pt")
>>> outputs = model(input_ids=inputs.input_ids, decoder_input_ids=decoder_inputs.input_ids)
>>> last_hidden_states = outputs.last_hidden_state
>>> list(last_hidden_states.shape)
[1, 4, 1024]
```"""
output_attentions = output_attentions if output_attentions is not None else self.config.output_attentions
output_hidden_states = (
output_hidden_states if output_hidden_states is not None else self.config.output_hidden_states
)
use_cache = use_cache if use_cache is not None else self.config.use_cache
return_dict = return_dict if return_dict is not None else self.config.use_return_dict
if encoder_outputs is None:
encoder_outputs = self.encoder(
input_ids=input_ids,
attention_mask=attention_mask,
inputs_embeds=inputs_embeds,
output_attentions=output_attentions,
output_hidden_states=output_hidden_states,
return_dict=return_dict,
)
# If the user passed a tuple for encoder_outputs, we wrap it in a BaseModelOutput when return_dict=True
elif return_dict and not isinstance(encoder_outputs, BaseModelOutput):
encoder_outputs = BaseModelOutput(
last_hidden_state=encoder_outputs[0],
hidden_states=encoder_outputs[1] if len(encoder_outputs) > 1 else None,
attentions=encoder_outputs[2] if len(encoder_outputs) > 2 else None,
)
# decoder outputs consists of (dec_features, past_key_value, dec_hidden, dec_attn)
decoder_outputs = self.decoder(
input_ids=decoder_input_ids,
attention_mask=decoder_attention_mask,
encoder_hidden_states=encoder_outputs[0],
encoder_attention_mask=attention_mask,
past_key_values=past_key_values,
inputs_embeds=decoder_inputs_embeds,
use_cache=use_cache,
output_attentions=output_attentions,
output_hidden_states=output_hidden_states,
return_dict=return_dict,
)
if not return_dict:
return decoder_outputs + encoder_outputs
return Seq2SeqModelOutput(
last_hidden_state=decoder_outputs.last_hidden_state,
past_key_values=decoder_outputs.past_key_values,
decoder_hidden_states=decoder_outputs.hidden_states,
decoder_attentions=decoder_outputs.attentions,
cross_attentions=decoder_outputs.cross_attentions,
encoder_last_hidden_state=encoder_outputs.last_hidden_state,
encoder_hidden_states=encoder_outputs.hidden_states,
encoder_attentions=encoder_outputs.attentions,
)
@add_start_docstrings("The PEGASUS-X for conditional generation (e.g. summarization).", PEGASUS_X_START_DOCSTRING)
class PegasusXForConditionalGeneration(PegasusXPreTrainedModel):
base_model_prefix = "model"
_tied_weights_keys = ["encoder.embed_tokens.weight", "decoder.embed_tokens.weight", "lm_head.weight"]
def __init__(self, config: PegasusXConfig):
super().__init__(config)
self.model = PegasusXModel(config)
self.lm_head = nn.Linear(config.d_model, self.model.shared.num_embeddings, bias=False)
# Initialize weights and apply final processing
self.post_init()
def get_encoder(self):
return self.model.get_encoder()
def get_decoder(self):
return self.model.get_decoder()
def get_output_embeddings(self):
return self.lm_head
def set_output_embeddings(self, new_embeddings):
self.lm_head = new_embeddings
def resize_position_embeddings(self, new_num_position_embeddings: int):
"""
Resizes position embeddings matrix of the model if `new_num_position_embeddings !=
config.max_position_embeddings`.
Arguments:
new_num_position_embeddings (`int`):
The number of new position embeddings. If position embeddings are learned, increasing the size will add
newly initialized vectors at the end, whereas reducing the size will remove vectors from the end. If
position embeddings are not learned (*e.g.* sinusoidal position embeddings), increasing the size will
add correct vectors at the end following the position encoding algorithm, whereas reducing the size
will remove vectors from the end.
"""
self.config.max_position_embeddings = new_num_position_embeddings
self.model.encoder.resize_position_embeddings(new_num_position_embeddings)
self.model.decoder.resize_position_embeddings(new_num_position_embeddings)
def get_position_embeddings(self) -> Tuple[nn.Embedding]:
"""
Returns the position embeddings matrix
"""
return (self.model.encoder.get_position_embeddings(), self.model.decoder.get_position_embeddings())
@add_start_docstrings_to_model_forward(PEGASUS_X_INPUTS_DOCSTRING)
@replace_return_docstrings(output_type=Seq2SeqLMOutput, config_class=_CONFIG_FOR_DOC)
@add_end_docstrings(PEGASUS_X_GENERATION_EXAMPLE)
def forward(
self,
input_ids: Optional[torch.Tensor] = None,
attention_mask: Optional[torch.Tensor] = None,
decoder_input_ids: Optional[torch.Tensor] = None,
decoder_attention_mask: Optional[torch.Tensor] = None,
encoder_outputs: Optional[Tuple[torch.FloatTensor]] = None,
past_key_values: Optional[Tuple[torch.FloatTensor]] = None,
inputs_embeds: Optional[torch.Tensor] = None,
decoder_inputs_embeds: Optional[torch.Tensor] = None,
labels: Optional[torch.Tensor] = None,
use_cache: Optional[bool] = None,
output_attentions: Optional[bool] = None,
output_hidden_states: Optional[bool] = None,
return_dict: Optional[bool] = None,
) -> Union[Tuple, Seq2SeqLMOutput]:
r"""
labels (`torch.LongTensor` of shape `(batch_size, sequence_length)`, *optional*):
Labels for computing the masked language modeling loss. Indices should either be in `[0, ...,
config.vocab_size]` or -100 (see `input_ids` docstring). Tokens with indices set to `-100` are ignored
(masked), the loss is only computed for the tokens with labels in `[0, ..., config.vocab_size]`.
Returns:
"""
return_dict = return_dict if return_dict is not None else self.config.use_return_dict
if labels is not None:
if use_cache:
logger.warning("The `use_cache` argument is changed to `False` since `labels` is provided.")
use_cache = False
if decoder_input_ids is None and decoder_inputs_embeds is None:
decoder_input_ids = shift_tokens_right(
labels, self.config.pad_token_id, self.config.decoder_start_token_id
)
outputs = self.model(
input_ids,
attention_mask=attention_mask,
decoder_input_ids=decoder_input_ids,
encoder_outputs=encoder_outputs,
decoder_attention_mask=decoder_attention_mask,
past_key_values=past_key_values,
inputs_embeds=inputs_embeds,
decoder_inputs_embeds=decoder_inputs_embeds,
use_cache=use_cache,
output_attentions=output_attentions,
output_hidden_states=output_hidden_states,
return_dict=return_dict,
)
lm_logits = self.lm_head(outputs[0])
masked_lm_loss = None
if labels is not None:
loss_fct = CrossEntropyLoss()
masked_lm_loss = loss_fct(lm_logits.view(-1, self.config.vocab_size), labels.view(-1))
if not return_dict:
output = (lm_logits,) + outputs[1:]
return ((masked_lm_loss,) + output) if masked_lm_loss is not None else output
return Seq2SeqLMOutput(
loss=masked_lm_loss,
logits=lm_logits,
past_key_values=outputs.past_key_values,
decoder_hidden_states=outputs.decoder_hidden_states,
decoder_attentions=outputs.decoder_attentions,
cross_attentions=outputs.cross_attentions,
encoder_last_hidden_state=outputs.encoder_last_hidden_state,
encoder_hidden_states=outputs.encoder_hidden_states,
encoder_attentions=outputs.encoder_attentions,
)
def prepare_inputs_for_generation(
self,
decoder_input_ids,
past_key_values=None,
attention_mask=None,
use_cache=None,
encoder_outputs=None,
**kwargs,
):
# cut decoder_input_ids if past is used
if past_key_values is not None:
past_length = past_key_values[0][0].shape[2]
# Some generation methods already pass only the last input ID
if decoder_input_ids.shape[1] > past_length:
remove_prefix_length = past_length
else:
# Default to old behavior: keep only final ID
remove_prefix_length = decoder_input_ids.shape[1] - 1
decoder_input_ids = decoder_input_ids[:, remove_prefix_length:]
return {
"input_ids": None, # encoder_outputs is defined. input_ids not needed
"encoder_outputs": encoder_outputs,
"past_key_values": past_key_values,
"decoder_input_ids": decoder_input_ids,
"attention_mask": attention_mask,
"use_cache": use_cache, # change this to avoid caching (presumably for debugging)
}
def prepare_decoder_input_ids_from_labels(self, labels: torch.Tensor):
return shift_tokens_right(labels, self.config.pad_token_id, self.config.decoder_start_token_id)
@staticmethod
def _reorder_cache(past_key_values, beam_idx):
reordered_past = ()
for layer_past in past_key_values:
# cached cross_attention states don't have to be reordered -> they are always the same
reordered_past += (
tuple(past_state.index_select(0, beam_idx.to(past_state.device)) for past_state in layer_past[:2])
+ layer_past[2:],
)
return reordered_past
# Copied from transformers.models.bart.modeling_bart.BartDecoderWrapper with Bart->PegasusX
class PegasusXDecoderWrapper(PegasusXPreTrainedModel):
"""
This wrapper class is a helper class to correctly load pretrained checkpoints when the causal language model is
used in combination with the [`EncoderDecoderModel`] framework.
"""
def __init__(self, config):
super().__init__(config)
self.decoder = PegasusXDecoder(config)
def forward(self, *args, **kwargs):
return self.decoder(*args, **kwargs)
| transformers/src/transformers/models/pegasus_x/modeling_pegasus_x.py/0 | {
"file_path": "transformers/src/transformers/models/pegasus_x/modeling_pegasus_x.py",
"repo_id": "transformers",
"token_count": 32980
} | 317 |
# coding=utf-8
# Copyright 2023 Authors: Wenhai Wang, Enze Xie, Xiang Li, Deng-Ping Fan,
# Kaitao Song, Ding Liang, Tong Lu, Ping Luo, Ling Shao and The HuggingFace Inc. team.
# All rights reserved.
#
# Licensed under the Apache License, Version 2.0 (the "License");
# you may not use this file except in compliance with the License.
# You may obtain a copy of the License at
#
# http://www.apache.org/licenses/LICENSE-2.0
#
# Unless required by applicable law or agreed to in writing, software
# distributed under the License is distributed on an "AS IS" BASIS,
# WITHOUT WARRANTIES OR CONDITIONS OF ANY KIND, either express or implied.
# See the License for the specific language governing permissions and
# limitations under the License.
""" Pvt model configuration"""
from collections import OrderedDict
from typing import Callable, List, Mapping
from packaging import version
from ...configuration_utils import PretrainedConfig
from ...onnx import OnnxConfig
from ...utils import logging
logger = logging.get_logger(__name__)
PVT_PRETRAINED_CONFIG_ARCHIVE_MAP = {
"pvt-tiny-224": "https://huggingface.co/Zetatech/pvt-tiny-224",
# See all PVT models at https://huggingface.co/models?filter=pvt
}
class PvtConfig(PretrainedConfig):
r"""
This is the configuration class to store the configuration of a [`PvtModel`]. It is used to instantiate an Pvt
model according to the specified arguments, defining the model architecture. Instantiating a configuration with the
defaults will yield a similar configuration to that of the Pvt
[Xrenya/pvt-tiny-224](https://huggingface.co/Xrenya/pvt-tiny-224) architecture.
Configuration objects inherit from [`PretrainedConfig`] and can be used to control the model outputs. Read the
documentation from [`PretrainedConfig`] for more information.
Args:
image_size (`int`, *optional*, defaults to 224):
The input image size
num_channels (`int`, *optional*, defaults to 3):
The number of input channels.
num_encoder_blocks (`int`, *optional*, defaults to 4):
The number of encoder blocks (i.e. stages in the Mix Transformer encoder).
depths (`List[int]`, *optional*, defaults to `[2, 2, 2, 2]`):
The number of layers in each encoder block.
sequence_reduction_ratios (`List[int]`, *optional*, defaults to `[8, 4, 2, 1]`):
Sequence reduction ratios in each encoder block.
hidden_sizes (`List[int]`, *optional*, defaults to `[64, 128, 320, 512]`):
Dimension of each of the encoder blocks.
patch_sizes (`List[int]`, *optional*, defaults to `[4, 2, 2, 2]`):
Patch size before each encoder block.
strides (`List[int]`, *optional*, defaults to `[4, 2, 2, 2]`):
Stride before each encoder block.
num_attention_heads (`List[int]`, *optional*, defaults to `[1, 2, 5, 8]`):
Number of attention heads for each attention layer in each block of the Transformer encoder.
mlp_ratios (`List[int]`, *optional*, defaults to `[8, 8, 4, 4]`):
Ratio of the size of the hidden layer compared to the size of the input layer of the Mix FFNs in the
encoder blocks.
hidden_act (`str` or `function`, *optional*, defaults to `"gelu"`):
The non-linear activation function (function or string) in the encoder and pooler. If string, `"gelu"`,
`"relu"`, `"selu"` and `"gelu_new"` are supported.
hidden_dropout_prob (`float`, *optional*, defaults to 0.0):
The dropout probability for all fully connected layers in the embeddings, encoder, and pooler.
attention_probs_dropout_prob (`float`, *optional*, defaults to 0.0):
The dropout ratio for the attention probabilities.
initializer_range (`float`, *optional*, defaults to 0.02):
The standard deviation of the truncated_normal_initializer for initializing all weight matrices.
drop_path_rate (`float`, *optional*, defaults to 0.0):
The dropout probability for stochastic depth, used in the blocks of the Transformer encoder.
layer_norm_eps (`float`, *optional*, defaults to 1e-06):
The epsilon used by the layer normalization layers.
qkv_bias (`bool`, *optional*, defaults to `True`):
Whether or not a learnable bias should be added to the queries, keys and values.
num_labels ('int', *optional*, defaults to 1000):
The number of classes.
Example:
```python
>>> from transformers import PvtModel, PvtConfig
>>> # Initializing a PVT Xrenya/pvt-tiny-224 style configuration
>>> configuration = PvtConfig()
>>> # Initializing a model from the Xrenya/pvt-tiny-224 style configuration
>>> model = PvtModel(configuration)
>>> # Accessing the model configuration
>>> configuration = model.config
```"""
model_type = "pvt"
def __init__(
self,
image_size: int = 224,
num_channels: int = 3,
num_encoder_blocks: int = 4,
depths: List[int] = [2, 2, 2, 2],
sequence_reduction_ratios: List[int] = [8, 4, 2, 1],
hidden_sizes: List[int] = [64, 128, 320, 512],
patch_sizes: List[int] = [4, 2, 2, 2],
strides: List[int] = [4, 2, 2, 2],
num_attention_heads: List[int] = [1, 2, 5, 8],
mlp_ratios: List[int] = [8, 8, 4, 4],
hidden_act: Mapping[str, Callable] = "gelu",
hidden_dropout_prob: float = 0.0,
attention_probs_dropout_prob: float = 0.0,
initializer_range: float = 0.02,
drop_path_rate: float = 0.0,
layer_norm_eps: float = 1e-6,
qkv_bias: bool = True,
num_labels: int = 1000,
**kwargs,
):
super().__init__(**kwargs)
self.image_size = image_size
self.num_channels = num_channels
self.num_encoder_blocks = num_encoder_blocks
self.depths = depths
self.sequence_reduction_ratios = sequence_reduction_ratios
self.hidden_sizes = hidden_sizes
self.patch_sizes = patch_sizes
self.strides = strides
self.mlp_ratios = mlp_ratios
self.num_attention_heads = num_attention_heads
self.hidden_act = hidden_act
self.hidden_dropout_prob = hidden_dropout_prob
self.attention_probs_dropout_prob = attention_probs_dropout_prob
self.initializer_range = initializer_range
self.drop_path_rate = drop_path_rate
self.layer_norm_eps = layer_norm_eps
self.num_labels = num_labels
self.qkv_bias = qkv_bias
class PvtOnnxConfig(OnnxConfig):
torch_onnx_minimum_version = version.parse("1.11")
@property
def inputs(self) -> Mapping[str, Mapping[int, str]]:
return OrderedDict(
[
("pixel_values", {0: "batch", 1: "num_channels", 2: "height", 3: "width"}),
]
)
@property
def atol_for_validation(self) -> float:
return 1e-4
@property
def default_onnx_opset(self) -> int:
return 12
| transformers/src/transformers/models/pvt/configuration_pvt.py/0 | {
"file_path": "transformers/src/transformers/models/pvt/configuration_pvt.py",
"repo_id": "transformers",
"token_count": 2810
} | 318 |
# coding=utf-8
# Copyright 2020, The RAG Authors and The HuggingFace Inc. team.
#
# Licensed under the Apache License, Version 2.0 (the "License");
# you may not use this file except in compliance with the License.
# You may obtain a copy of the License at
#
# http://www.apache.org/licenses/LICENSE-2.0
#
# Unless required by applicable law or agreed to in writing, software
# distributed under the License is distributed on an "AS IS" BASIS,
# WITHOUT WARRANTIES OR CONDITIONS OF ANY KIND, either express or implied.
# See the License for the specific language governing permissions and
# limitations under the License.
"""RAG Retriever model implementation."""
import os
import pickle
import time
from typing import Iterable, List, Optional, Tuple
import numpy as np
from ...tokenization_utils import PreTrainedTokenizer
from ...tokenization_utils_base import BatchEncoding
from ...utils import cached_file, is_datasets_available, is_faiss_available, logging, requires_backends, strtobool
from .configuration_rag import RagConfig
from .tokenization_rag import RagTokenizer
if is_datasets_available():
from datasets import Dataset, load_dataset, load_from_disk
if is_faiss_available():
import faiss
logger = logging.get_logger(__name__)
LEGACY_INDEX_PATH = "https://storage.googleapis.com/huggingface-nlp/datasets/wiki_dpr/"
class Index:
"""
A base class for the Indices encapsulated by the [`RagRetriever`].
"""
def get_doc_dicts(self, doc_ids: np.ndarray) -> List[dict]:
"""
Returns a list of dictionaries, containing titles and text of the retrieved documents.
Args:
doc_ids (`np.ndarray` of shape `(batch_size, n_docs)`):
A tensor of document indices.
"""
raise NotImplementedError
def get_top_docs(self, question_hidden_states: np.ndarray, n_docs=5) -> Tuple[np.ndarray, np.ndarray]:
"""
For each query in the batch, retrieves `n_docs` documents.
Args:
question_hidden_states (`np.ndarray` of shape `(batch_size, vector_size)`):
An array of query vectors.
n_docs (`int`):
The number of docs retrieved per query.
Returns:
`np.ndarray` of shape `(batch_size, n_docs)`: A tensor of indices of retrieved documents. `np.ndarray` of
shape `(batch_size, vector_size)`: A tensor of vector representations of retrieved documents.
"""
raise NotImplementedError
def is_initialized(self):
"""
Returns `True` if index is already initialized.
"""
raise NotImplementedError
def init_index(self):
"""
A function responsible for loading the index into memory. Should be called only once per training run of a RAG
model. E.g. if the model is trained on multiple GPUs in a distributed setup, only one of the workers will load
the index.
"""
raise NotImplementedError
class LegacyIndex(Index):
"""
An index which can be deserialized from the files built using https://github.com/facebookresearch/DPR. We use
default faiss index parameters as specified in that repository.
Args:
vector_size (`int`):
The dimension of indexed vectors.
index_path (`str`):
A path to a *directory* containing index files compatible with [`~models.rag.retrieval_rag.LegacyIndex`]
"""
INDEX_FILENAME = "hf_bert_base.hnswSQ8_correct_phi_128.c_index"
PASSAGE_FILENAME = "psgs_w100.tsv.pkl"
def __init__(self, vector_size, index_path):
self.index_id_to_db_id = []
self.index_path = index_path
self.passages = self._load_passages()
self.vector_size = vector_size
self.index = None
self._index_initialized = False
def _resolve_path(self, index_path, filename):
is_local = os.path.isdir(index_path)
try:
# Load from URL or cache if already cached
resolved_archive_file = cached_file(index_path, filename)
except EnvironmentError:
msg = (
f"Can't load '{filename}'. Make sure that:\n\n"
f"- '{index_path}' is a correct remote path to a directory containing a file named {filename}\n\n"
f"- or '{index_path}' is the correct path to a directory containing a file named {filename}.\n\n"
)
raise EnvironmentError(msg)
if is_local:
logger.info(f"loading file {resolved_archive_file}")
else:
logger.info(f"loading file {filename} from cache at {resolved_archive_file}")
return resolved_archive_file
def _load_passages(self):
logger.info(f"Loading passages from {self.index_path}")
passages_path = self._resolve_path(self.index_path, self.PASSAGE_FILENAME)
if not strtobool(os.environ.get("TRUST_REMOTE_CODE", "False")):
raise ValueError(
"This part uses `pickle.load` which is insecure and will execute arbitrary code that is potentially "
"malicious. It's recommended to never unpickle data that could have come from an untrusted source, or "
"that could have been tampered with. If you already verified the pickle data and decided to use it, "
"you can set the environment variable `TRUST_REMOTE_CODE` to `True` to allow it."
)
with open(passages_path, "rb") as passages_file:
passages = pickle.load(passages_file)
return passages
def _deserialize_index(self):
logger.info(f"Loading index from {self.index_path}")
resolved_index_path = self._resolve_path(self.index_path, self.INDEX_FILENAME + ".index.dpr")
self.index = faiss.read_index(resolved_index_path)
resolved_meta_path = self._resolve_path(self.index_path, self.INDEX_FILENAME + ".index_meta.dpr")
if not strtobool(os.environ.get("TRUST_REMOTE_CODE", "False")):
raise ValueError(
"This part uses `pickle.load` which is insecure and will execute arbitrary code that is potentially "
"malicious. It's recommended to never unpickle data that could have come from an untrusted source, or "
"that could have been tampered with. If you already verified the pickle data and decided to use it, "
"you can set the environment variable `TRUST_REMOTE_CODE` to `True` to allow it."
)
with open(resolved_meta_path, "rb") as metadata_file:
self.index_id_to_db_id = pickle.load(metadata_file)
assert (
len(self.index_id_to_db_id) == self.index.ntotal
), "Deserialized index_id_to_db_id should match faiss index size"
def is_initialized(self):
return self._index_initialized
def init_index(self):
index = faiss.IndexHNSWFlat(self.vector_size + 1, 512)
index.hnsw.efSearch = 128
index.hnsw.efConstruction = 200
self.index = index
self._deserialize_index()
self._index_initialized = True
def get_doc_dicts(self, doc_ids: np.array):
doc_list = []
for doc_ids_i in doc_ids:
ids = [str(int(doc_id)) for doc_id in doc_ids_i]
docs = [self.passages[doc_id] for doc_id in ids]
doc_list.append(docs)
doc_dicts = []
for docs in doc_list:
doc_dict = {}
doc_dict["title"] = [doc[1] for doc in docs]
doc_dict["text"] = [doc[0] for doc in docs]
doc_dicts.append(doc_dict)
return doc_dicts
def get_top_docs(self, question_hidden_states: np.ndarray, n_docs=5) -> Tuple[np.ndarray, np.ndarray]:
aux_dim = np.zeros(len(question_hidden_states), dtype="float32").reshape(-1, 1)
query_nhsw_vectors = np.hstack((question_hidden_states, aux_dim))
_, docs_ids = self.index.search(query_nhsw_vectors, n_docs)
vectors = [[self.index.reconstruct(int(doc_id))[:-1] for doc_id in doc_ids] for doc_ids in docs_ids]
ids = [[int(self.index_id_to_db_id[doc_id]) for doc_id in doc_ids] for doc_ids in docs_ids]
return np.array(ids), np.array(vectors)
class HFIndexBase(Index):
def __init__(self, vector_size, dataset, index_initialized=False):
self.vector_size = vector_size
self.dataset = dataset
self._index_initialized = index_initialized
self._check_dataset_format(with_index=index_initialized)
dataset.set_format("numpy", columns=["embeddings"], output_all_columns=True, dtype="float32")
def _check_dataset_format(self, with_index: bool):
if not isinstance(self.dataset, Dataset):
raise ValueError(f"Dataset should be a datasets.Dataset object, but got {type(self.dataset)}")
if len({"title", "text", "embeddings"} - set(self.dataset.column_names)) > 0:
raise ValueError(
"Dataset should be a dataset with the following columns: "
"title (str), text (str) and embeddings (arrays of dimension vector_size), "
f"but got columns {self.dataset.column_names}"
)
if with_index and "embeddings" not in self.dataset.list_indexes():
raise ValueError(
"Missing faiss index in the dataset. Make sure you called `dataset.add_faiss_index` to compute it "
"or `dataset.load_faiss_index` to load one from the disk."
)
def init_index(self):
raise NotImplementedError()
def is_initialized(self):
return self._index_initialized
def get_doc_dicts(self, doc_ids: np.ndarray) -> List[dict]:
return [self.dataset[doc_ids[i].tolist()] for i in range(doc_ids.shape[0])]
def get_top_docs(self, question_hidden_states: np.ndarray, n_docs=5) -> Tuple[np.ndarray, np.ndarray]:
_, ids = self.dataset.search_batch("embeddings", question_hidden_states, n_docs)
docs = [self.dataset[[i for i in indices if i >= 0]] for indices in ids]
vectors = [doc["embeddings"] for doc in docs]
for i in range(len(vectors)):
if len(vectors[i]) < n_docs:
vectors[i] = np.vstack([vectors[i], np.zeros((n_docs - len(vectors[i]), self.vector_size))])
return np.array(ids), np.array(vectors) # shapes (batch_size, n_docs) and (batch_size, n_docs, d)
class CanonicalHFIndex(HFIndexBase):
"""
A wrapper around an instance of [`~datasets.Datasets`]. If `index_path` is set to `None`, we load the pre-computed
index available with the [`~datasets.arrow_dataset.Dataset`], otherwise, we load the index from the indicated path
on disk.
Args:
vector_size (`int`): the dimension of the passages embeddings used by the index
dataset_name (`str`, optional, defaults to `wiki_dpr`):
A dataset identifier of the indexed dataset on HuggingFace AWS bucket (list all available datasets and ids
with `datasets.list_datasets()`).
dataset_split (`str`, optional, defaults to `train`)
Which split of the `dataset` to load.
index_name (`str`, optional, defaults to `train`)
The index_name of the index associated with the `dataset`. The index loaded from `index_path` will be saved
under this name.
index_path (`str`, optional, defaults to `None`)
The path to the serialized faiss index on disk.
use_dummy_dataset (`bool`, optional, defaults to `False`):
If True, use the dummy configuration of the dataset for tests.
"""
def __init__(
self,
vector_size: int,
dataset_name: str = "wiki_dpr",
dataset_split: str = "train",
index_name: Optional[str] = None,
index_path: Optional[str] = None,
use_dummy_dataset=False,
):
if int(index_path is None) + int(index_name is None) != 1:
raise ValueError("Please provide `index_name` or `index_path`.")
self.dataset_name = dataset_name
self.dataset_split = dataset_split
self.index_name = index_name
self.index_path = index_path
self.use_dummy_dataset = use_dummy_dataset
logger.info(f"Loading passages from {self.dataset_name}")
dataset = load_dataset(
self.dataset_name, with_index=False, split=self.dataset_split, dummy=self.use_dummy_dataset
)
super().__init__(vector_size, dataset, index_initialized=False)
def init_index(self):
if self.index_path is not None:
logger.info(f"Loading index from {self.index_path}")
self.dataset.load_faiss_index("embeddings", file=self.index_path)
else:
logger.info(f"Loading index from {self.dataset_name} with index name {self.index_name}")
self.dataset = load_dataset(
self.dataset_name,
with_embeddings=True,
with_index=True,
split=self.dataset_split,
index_name=self.index_name,
dummy=self.use_dummy_dataset,
)
self.dataset.set_format("numpy", columns=["embeddings"], output_all_columns=True)
self._index_initialized = True
class CustomHFIndex(HFIndexBase):
"""
A wrapper around an instance of [`~datasets.Datasets`]. The dataset and the index are both loaded from the
indicated paths on disk.
Args:
vector_size (`int`): the dimension of the passages embeddings used by the index
dataset_path (`str`):
The path to the serialized dataset on disk. The dataset should have 3 columns: title (str), text (str) and
embeddings (arrays of dimension vector_size)
index_path (`str`)
The path to the serialized faiss index on disk.
"""
def __init__(self, vector_size: int, dataset, index_path=None):
super().__init__(vector_size, dataset, index_initialized=index_path is None)
self.index_path = index_path
@classmethod
def load_from_disk(cls, vector_size, dataset_path, index_path):
logger.info(f"Loading passages from {dataset_path}")
if dataset_path is None or index_path is None:
raise ValueError(
"Please provide `dataset_path` and `index_path` after calling `dataset.save_to_disk(dataset_path)` "
"and `dataset.get_index('embeddings').save(index_path)`."
)
dataset = load_from_disk(dataset_path)
return cls(vector_size=vector_size, dataset=dataset, index_path=index_path)
def init_index(self):
if not self.is_initialized():
logger.info(f"Loading index from {self.index_path}")
self.dataset.load_faiss_index("embeddings", file=self.index_path)
self._index_initialized = True
class RagRetriever:
"""
Retriever used to get documents from vector queries. It retrieves the documents embeddings as well as the documents
contents, and it formats them to be used with a RagModel.
Args:
config ([`RagConfig`]):
The configuration of the RAG model this Retriever is used with. Contains parameters indicating which
`Index` to build. You can load your own custom dataset with `config.index_name="custom"` or use a canonical
one (default) from the datasets library with `config.index_name="wiki_dpr"` for example.
question_encoder_tokenizer ([`PreTrainedTokenizer`]):
The tokenizer that was used to tokenize the question. It is used to decode the question and then use the
generator_tokenizer.
generator_tokenizer ([`PreTrainedTokenizer`]):
The tokenizer used for the generator part of the RagModel.
index ([`~models.rag.retrieval_rag.Index`], optional, defaults to the one defined by the configuration):
If specified, use this index instead of the one built using the configuration
Examples:
```python
>>> # To load the default "wiki_dpr" dataset with 21M passages from wikipedia (index name is 'compressed' or 'exact')
>>> from transformers import RagRetriever
>>> retriever = RagRetriever.from_pretrained(
... "facebook/dpr-ctx_encoder-single-nq-base", dataset="wiki_dpr", index_name="compressed"
... )
>>> # To load your own indexed dataset built with the datasets library. More info on how to build the indexed dataset in examples/rag/use_own_knowledge_dataset.py
>>> from transformers import RagRetriever
>>> dataset = (
... ...
... ) # dataset must be a datasets.Datasets object with columns "title", "text" and "embeddings", and it must have a faiss index
>>> retriever = RagRetriever.from_pretrained("facebook/dpr-ctx_encoder-single-nq-base", indexed_dataset=dataset)
>>> # To load your own indexed dataset built with the datasets library that was saved on disk. More info in examples/rag/use_own_knowledge_dataset.py
>>> from transformers import RagRetriever
>>> dataset_path = "path/to/my/dataset" # dataset saved via *dataset.save_to_disk(...)*
>>> index_path = "path/to/my/index.faiss" # faiss index saved via *dataset.get_index("embeddings").save(...)*
>>> retriever = RagRetriever.from_pretrained(
... "facebook/dpr-ctx_encoder-single-nq-base",
... index_name="custom",
... passages_path=dataset_path,
... index_path=index_path,
... )
>>> # To load the legacy index built originally for Rag's paper
>>> from transformers import RagRetriever
>>> retriever = RagRetriever.from_pretrained("facebook/dpr-ctx_encoder-single-nq-base", index_name="legacy")
```"""
def __init__(self, config, question_encoder_tokenizer, generator_tokenizer, index=None, init_retrieval=True):
self._init_retrieval = init_retrieval
requires_backends(self, ["datasets", "faiss"])
super().__init__()
self.index = index or self._build_index(config)
self.generator_tokenizer = generator_tokenizer
self.question_encoder_tokenizer = question_encoder_tokenizer
self.n_docs = config.n_docs
self.batch_size = config.retrieval_batch_size
self.config = config
if self._init_retrieval:
self.init_retrieval()
self.ctx_encoder_tokenizer = None
self.return_tokenized_docs = False
@staticmethod
def _build_index(config):
if config.index_name == "legacy":
return LegacyIndex(
config.retrieval_vector_size,
config.index_path or LEGACY_INDEX_PATH,
)
elif config.index_name == "custom":
return CustomHFIndex.load_from_disk(
vector_size=config.retrieval_vector_size,
dataset_path=config.passages_path,
index_path=config.index_path,
)
else:
return CanonicalHFIndex(
vector_size=config.retrieval_vector_size,
dataset_name=config.dataset,
dataset_split=config.dataset_split,
index_name=config.index_name,
index_path=config.index_path,
use_dummy_dataset=config.use_dummy_dataset,
)
@classmethod
def from_pretrained(cls, retriever_name_or_path, indexed_dataset=None, **kwargs):
requires_backends(cls, ["datasets", "faiss"])
config = kwargs.pop("config", None) or RagConfig.from_pretrained(retriever_name_or_path, **kwargs)
rag_tokenizer = RagTokenizer.from_pretrained(retriever_name_or_path, config=config)
question_encoder_tokenizer = rag_tokenizer.question_encoder
generator_tokenizer = rag_tokenizer.generator
if indexed_dataset is not None:
config.index_name = "custom"
index = CustomHFIndex(config.retrieval_vector_size, indexed_dataset)
else:
index = cls._build_index(config)
return cls(
config,
question_encoder_tokenizer=question_encoder_tokenizer,
generator_tokenizer=generator_tokenizer,
index=index,
)
def save_pretrained(self, save_directory):
if isinstance(self.index, CustomHFIndex):
if self.config.index_path is None:
index_path = os.path.join(save_directory, "hf_dataset_index.faiss")
self.index.dataset.get_index("embeddings").save(index_path)
self.config.index_path = index_path
if self.config.passages_path is None:
passages_path = os.path.join(save_directory, "hf_dataset")
# datasets don't support save_to_disk with indexes right now
faiss_index = self.index.dataset._indexes.pop("embeddings")
self.index.dataset.save_to_disk(passages_path)
self.index.dataset._indexes["embeddings"] = faiss_index
self.config.passages_path = passages_path
self.config.save_pretrained(save_directory)
rag_tokenizer = RagTokenizer(
question_encoder=self.question_encoder_tokenizer,
generator=self.generator_tokenizer,
)
rag_tokenizer.save_pretrained(save_directory)
def init_retrieval(self):
"""
Retriever initialization function. It loads the index into memory.
"""
logger.info("initializing retrieval")
self.index.init_index()
def postprocess_docs(self, docs, input_strings, prefix, n_docs, return_tensors=None):
r"""
Postprocessing retrieved `docs` and combining them with `input_strings`.
Args:
docs (`dict`):
Retrieved documents.
input_strings (`str`):
Input strings decoded by `preprocess_query`.
prefix (`str`):
Prefix added at the beginning of each input, typically used with T5-based models.
Return:
`tuple(tensors)`: a tuple consisting of two elements: contextualized `input_ids` and a compatible
`attention_mask`.
"""
def cat_input_and_doc(doc_title, doc_text, input_string, prefix):
# TODO(Patrick): if we train more RAG models, I want to put the input first to take advantage of effortless truncation
# TODO(piktus): better handling of truncation
if doc_title.startswith('"'):
doc_title = doc_title[1:]
if doc_title.endswith('"'):
doc_title = doc_title[:-1]
if prefix is None:
prefix = ""
out = (prefix + doc_title + self.config.title_sep + doc_text + self.config.doc_sep + input_string).replace(
" ", " "
)
return out
rag_input_strings = [
cat_input_and_doc(
docs[i]["title"][j],
docs[i]["text"][j],
input_strings[i],
prefix,
)
for i in range(len(docs))
for j in range(n_docs)
]
contextualized_inputs = self.generator_tokenizer.batch_encode_plus(
rag_input_strings,
max_length=self.config.max_combined_length,
return_tensors=return_tensors,
padding="max_length",
truncation=True,
)
return contextualized_inputs["input_ids"], contextualized_inputs["attention_mask"]
def _chunk_tensor(self, t: Iterable, chunk_size: int) -> List[Iterable]:
return [t[i : i + chunk_size] for i in range(0, len(t), chunk_size)]
def _main_retrieve(self, question_hidden_states: np.ndarray, n_docs: int) -> Tuple[np.ndarray, np.ndarray]:
question_hidden_states_batched = self._chunk_tensor(question_hidden_states, self.batch_size)
ids_batched = []
vectors_batched = []
for question_hidden_states in question_hidden_states_batched:
start_time = time.time()
ids, vectors = self.index.get_top_docs(question_hidden_states, n_docs)
logger.debug(
f"index search time: {time.time() - start_time} sec, batch size {question_hidden_states.shape}"
)
ids_batched.extend(ids)
vectors_batched.extend(vectors)
return (
np.array(ids_batched),
np.array(vectors_batched),
) # shapes (batch_size, n_docs) and (batch_size, n_docs, d)
def retrieve(self, question_hidden_states: np.ndarray, n_docs: int) -> Tuple[np.ndarray, List[dict]]:
"""
Retrieves documents for specified `question_hidden_states`.
Args:
question_hidden_states (`np.ndarray` of shape `(batch_size, vector_size)`):
A batch of query vectors to retrieve with.
n_docs (`int`):
The number of docs retrieved per query.
Return:
`Tuple[np.ndarray, np.ndarray, List[dict]]`: A tuple with the following objects:
- **retrieved_doc_embeds** (`np.ndarray` of shape `(batch_size, n_docs, dim)`) -- The retrieval embeddings
of the retrieved docs per query.
- **doc_ids** (`np.ndarray` of shape `(batch_size, n_docs)`) -- The ids of the documents in the index
- **doc_dicts** (`List[dict]`): The `retrieved_doc_embeds` examples per query.
"""
doc_ids, retrieved_doc_embeds = self._main_retrieve(question_hidden_states, n_docs)
return retrieved_doc_embeds, doc_ids, self.index.get_doc_dicts(doc_ids)
def set_ctx_encoder_tokenizer(self, ctx_encoder_tokenizer: PreTrainedTokenizer):
# used in end2end retriever training
self.ctx_encoder_tokenizer = ctx_encoder_tokenizer
self.return_tokenized_docs = True
def __call__(
self,
question_input_ids: List[List[int]],
question_hidden_states: np.ndarray,
prefix=None,
n_docs=None,
return_tensors=None,
) -> BatchEncoding:
"""
Retrieves documents for specified `question_hidden_states`.
Args:
question_input_ids (`List[List[int]]`) batch of input ids
question_hidden_states (`np.ndarray` of shape `(batch_size, vector_size)`:
A batch of query vectors to retrieve with.
prefix (`str`, *optional*):
The prefix used by the generator's tokenizer.
n_docs (`int`, *optional*):
The number of docs retrieved per query.
return_tensors (`str` or [`~utils.TensorType`], *optional*, defaults to "pt"):
If set, will return tensors instead of list of python integers. Acceptable values are:
- `'tf'`: Return TensorFlow `tf.constant` objects.
- `'pt'`: Return PyTorch `torch.Tensor` objects.
- `'np'`: Return Numpy `np.ndarray` objects.
Returns: [`BatchEncoding`]: A [`BatchEncoding`] with the following fields:
- **context_input_ids** -- List of token ids to be fed to a model.
[What are input IDs?](../glossary#input-ids)
- **context_attention_mask** -- List of indices specifying which tokens should be attended to by the model
(when `return_attention_mask=True` or if *"attention_mask"* is in `self.model_input_names`).
[What are attention masks?](../glossary#attention-mask)
- **retrieved_doc_embeds** -- List of embeddings of the retrieved documents
- **doc_ids** -- List of ids of the retrieved documents
"""
n_docs = n_docs if n_docs is not None else self.n_docs
prefix = prefix if prefix is not None else self.config.generator.prefix
retrieved_doc_embeds, doc_ids, docs = self.retrieve(question_hidden_states, n_docs)
input_strings = self.question_encoder_tokenizer.batch_decode(question_input_ids, skip_special_tokens=True)
context_input_ids, context_attention_mask = self.postprocess_docs(
docs, input_strings, prefix, n_docs, return_tensors=return_tensors
)
if self.return_tokenized_docs:
retrieved_doc_text = []
retrieved_doc_title = []
for b_idx in range(len(docs)):
for doc_idx in range(n_docs):
retrieved_doc_text.append(docs[b_idx]["text"][doc_idx])
retrieved_doc_title.append(docs[b_idx]["title"][doc_idx])
tokenized_docs = self.ctx_encoder_tokenizer(
retrieved_doc_title,
retrieved_doc_text,
truncation=True,
padding="longest",
return_tensors=return_tensors,
)
return BatchEncoding(
{
"context_input_ids": context_input_ids,
"context_attention_mask": context_attention_mask,
"retrieved_doc_embeds": retrieved_doc_embeds,
"doc_ids": doc_ids,
"tokenized_doc_ids": tokenized_docs["input_ids"],
"tokenized_doc_attention_mask": tokenized_docs["attention_mask"],
},
tensor_type=return_tensors,
)
else:
return BatchEncoding(
{
"context_input_ids": context_input_ids,
"context_attention_mask": context_attention_mask,
"retrieved_doc_embeds": retrieved_doc_embeds,
"doc_ids": doc_ids,
},
tensor_type=return_tensors,
)
| transformers/src/transformers/models/rag/retrieval_rag.py/0 | {
"file_path": "transformers/src/transformers/models/rag/retrieval_rag.py",
"repo_id": "transformers",
"token_count": 12868
} | 319 |
# coding=utf-8
# Copyright 2022 The HuggingFace Inc. team.
#
# Licensed under the Apache License, Version 2.0 (the "License");
# you may not use this file except in compliance with the License.
# You may obtain a copy of the License at
#
# http://www.apache.org/licenses/LICENSE-2.0
#
# Unless required by applicable law or agreed to in writing, software
# distributed under the License is distributed on an "AS IS" BASIS,
# WITHOUT WARRANTIES OR CONDITIONS OF ANY KIND, either express or implied.
# See the License for the specific language governing permissions and
# limitations under the License.
"""Convert RegNet 10B checkpoints vissl."""
# You need to install a specific version of classy vision
# pip install git+https://github.com/FrancescoSaverioZuppichini/ClassyVision.git@convert_weights
import argparse
import json
import os
import re
from collections import OrderedDict
from dataclasses import dataclass, field
from functools import partial
from pathlib import Path
from pprint import pprint
from typing import Dict, List, Tuple
import torch
import torch.nn as nn
from classy_vision.models.regnet import RegNet, RegNetParams
from huggingface_hub import cached_download, hf_hub_url
from torch import Tensor
from vissl.models.model_helpers import get_trunk_forward_outputs
from transformers import AutoImageProcessor, RegNetConfig, RegNetForImageClassification, RegNetModel
from transformers.modeling_utils import PreTrainedModel
from transformers.utils import logging
logging.set_verbosity_info()
logger = logging.get_logger()
@dataclass
class Tracker:
module: nn.Module
traced: List[nn.Module] = field(default_factory=list)
handles: list = field(default_factory=list)
name2module: Dict[str, nn.Module] = field(default_factory=OrderedDict)
def _forward_hook(self, m, inputs: Tensor, outputs: Tensor, name: str):
has_not_submodules = len(list(m.modules())) == 1 or isinstance(m, nn.Conv2d) or isinstance(m, nn.BatchNorm2d)
if has_not_submodules:
self.traced.append(m)
self.name2module[name] = m
def __call__(self, x: Tensor):
for name, m in self.module.named_modules():
self.handles.append(m.register_forward_hook(partial(self._forward_hook, name=name)))
self.module(x)
[x.remove() for x in self.handles]
return self
@property
def parametrized(self):
# check the len of the state_dict keys to see if we have learnable params
return {k: v for k, v in self.name2module.items() if len(list(v.state_dict().keys())) > 0}
class FakeRegNetVisslWrapper(nn.Module):
"""
Fake wrapper for RegNet that mimics what vissl does without the need to pass a config file.
"""
def __init__(self, model: nn.Module):
super().__init__()
feature_blocks: List[Tuple[str, nn.Module]] = []
# - get the stem
feature_blocks.append(("conv1", model.stem))
# - get all the feature blocks
for k, v in model.trunk_output.named_children():
assert k.startswith("block"), f"Unexpected layer name {k}"
block_index = len(feature_blocks) + 1
feature_blocks.append((f"res{block_index}", v))
self._feature_blocks = nn.ModuleDict(feature_blocks)
def forward(self, x: Tensor):
return get_trunk_forward_outputs(
x,
out_feat_keys=None,
feature_blocks=self._feature_blocks,
)
class FakeRegNetParams(RegNetParams):
"""
Used to instantiace a RegNet model from classy vision with the same depth as the 10B one but with super small
parameters, so we can trace it in memory.
"""
def get_expanded_params(self):
return [(8, 2, 2, 8, 1.0), (8, 2, 7, 8, 1.0), (8, 2, 17, 8, 1.0), (8, 2, 1, 8, 1.0)]
def get_from_to_our_keys(model_name: str) -> Dict[str, str]:
"""
Returns a dictionary that maps from original model's key -> our implementation's keys
"""
# create our model (with small weights)
our_config = RegNetConfig(depths=[2, 7, 17, 1], hidden_sizes=[8, 8, 8, 8], groups_width=8)
if "in1k" in model_name:
our_model = RegNetForImageClassification(our_config)
else:
our_model = RegNetModel(our_config)
# create from model (with small weights)
from_model = FakeRegNetVisslWrapper(
RegNet(FakeRegNetParams(depth=27, group_width=1010, w_0=1744, w_a=620.83, w_m=2.52))
)
with torch.no_grad():
from_model = from_model.eval()
our_model = our_model.eval()
x = torch.randn((1, 3, 32, 32))
# trace both
dest_tracker = Tracker(our_model)
dest_traced = dest_tracker(x).parametrized
pprint(dest_tracker.name2module)
src_tracker = Tracker(from_model)
src_traced = src_tracker(x).parametrized
# convert the keys -> module dict to keys -> params
def to_params_dict(dict_with_modules):
params_dict = OrderedDict()
for name, module in dict_with_modules.items():
for param_name, param in module.state_dict().items():
params_dict[f"{name}.{param_name}"] = param
return params_dict
from_to_ours_keys = {}
src_state_dict = to_params_dict(src_traced)
dst_state_dict = to_params_dict(dest_traced)
for (src_key, src_param), (dest_key, dest_param) in zip(src_state_dict.items(), dst_state_dict.items()):
from_to_ours_keys[src_key] = dest_key
logger.info(f"{src_key} -> {dest_key}")
# if "in1k" was in the model_name it means it must have a classification head (was finetuned)
if "in1k" in model_name:
from_to_ours_keys["0.clf.0.weight"] = "classifier.1.weight"
from_to_ours_keys["0.clf.0.bias"] = "classifier.1.bias"
return from_to_ours_keys
def convert_weights_and_push(save_directory: Path, model_name: str = None, push_to_hub: bool = True):
filename = "imagenet-1k-id2label.json"
num_labels = 1000
repo_id = "huggingface/label-files"
num_labels = num_labels
id2label = json.load(open(cached_download(hf_hub_url(repo_id, filename, repo_type="dataset")), "r"))
id2label = {int(k): v for k, v in id2label.items()}
id2label = id2label
label2id = {v: k for k, v in id2label.items()}
ImageNetPreTrainedConfig = partial(RegNetConfig, num_labels=num_labels, id2label=id2label, label2id=label2id)
names_to_config = {
"regnet-y-10b-seer": ImageNetPreTrainedConfig(
depths=[2, 7, 17, 1], hidden_sizes=[2020, 4040, 11110, 28280], groups_width=1010
),
# finetuned on imagenet
"regnet-y-10b-seer-in1k": ImageNetPreTrainedConfig(
depths=[2, 7, 17, 1], hidden_sizes=[2020, 4040, 11110, 28280], groups_width=1010
),
}
# add seer weights logic
def load_using_classy_vision(checkpoint_url: str) -> Tuple[Dict, Dict]:
files = torch.hub.load_state_dict_from_url(checkpoint_url, model_dir=str(save_directory), map_location="cpu")
# check if we have a head, if yes add it
model_state_dict = files["classy_state_dict"]["base_model"]["model"]
return model_state_dict["trunk"], model_state_dict["heads"]
names_to_from_model = {
"regnet-y-10b-seer": partial(
load_using_classy_vision,
"https://dl.fbaipublicfiles.com/vissl/model_zoo/seer_regnet10B/model_iteration124500_conso.torch",
),
"regnet-y-10b-seer-in1k": partial(
load_using_classy_vision,
"https://dl.fbaipublicfiles.com/vissl/model_zoo/seer_finetuned/seer_10b_finetuned_in1k_model_phase28_conso.torch",
),
}
from_to_ours_keys = get_from_to_our_keys(model_name)
if not (save_directory / f"{model_name}.pth").exists():
logger.info("Loading original state_dict.")
from_state_dict_trunk, from_state_dict_head = names_to_from_model[model_name]()
from_state_dict = from_state_dict_trunk
if "in1k" in model_name:
# add the head
from_state_dict = {**from_state_dict_trunk, **from_state_dict_head}
logger.info("Done!")
converted_state_dict = {}
not_used_keys = list(from_state_dict.keys())
regex = r"\.block.-part."
# this is "interesting", so the original checkpoints have `block[0,1]-part` in each key name, we remove it
for key in from_state_dict.keys():
# remove the weird "block[0,1]-part" from the key
src_key = re.sub(regex, "", key)
# now src_key from the model checkpoints is the one we got from the original model after tracing, so use it to get the correct destination key
dest_key = from_to_ours_keys[src_key]
# store the parameter with our key
converted_state_dict[dest_key] = from_state_dict[key]
not_used_keys.remove(key)
# check that all keys have been updated
assert len(not_used_keys) == 0, f"Some keys where not used {','.join(not_used_keys)}"
logger.info(f"The following keys were not used: {','.join(not_used_keys)}")
# save our state dict to disk
torch.save(converted_state_dict, save_directory / f"{model_name}.pth")
del converted_state_dict
else:
logger.info("The state_dict was already stored on disk.")
if push_to_hub:
logger.info(f"Token is {os.environ['HF_TOKEN']}")
logger.info("Loading our model.")
# create our model
our_config = names_to_config[model_name]
our_model_func = RegNetModel
if "in1k" in model_name:
our_model_func = RegNetForImageClassification
our_model = our_model_func(our_config)
# place our model to the meta device (so remove all the weights)
our_model.to(torch.device("meta"))
logger.info("Loading state_dict in our model.")
# load state dict
state_dict_keys = our_model.state_dict().keys()
PreTrainedModel._load_pretrained_model_low_mem(
our_model, state_dict_keys, [save_directory / f"{model_name}.pth"]
)
logger.info("Finally, pushing!")
# push it to hub
our_model.push_to_hub(
repo_path_or_name=save_directory / model_name,
commit_message="Add model",
output_dir=save_directory / model_name,
)
size = 384
# we can use the convnext one
image_processor = AutoImageProcessor.from_pretrained("facebook/convnext-base-224-22k-1k", size=size)
image_processor.push_to_hub(
repo_path_or_name=save_directory / model_name,
commit_message="Add image processor",
output_dir=save_directory / model_name,
)
if __name__ == "__main__":
parser = argparse.ArgumentParser()
# Required parameters
parser.add_argument(
"--model_name",
default=None,
type=str,
help=(
"The name of the model you wish to convert, it must be one of the supported regnet* architecture,"
" currently: regnetx-*, regnety-*. If `None`, all of them will the converted."
),
)
parser.add_argument(
"--pytorch_dump_folder_path",
default=None,
type=Path,
required=True,
help="Path to the output PyTorch model directory.",
)
parser.add_argument(
"--push_to_hub",
default=True,
type=bool,
required=False,
help="If True, push model and image processor to the hub.",
)
args = parser.parse_args()
pytorch_dump_folder_path: Path = args.pytorch_dump_folder_path
pytorch_dump_folder_path.mkdir(exist_ok=True, parents=True)
convert_weights_and_push(pytorch_dump_folder_path, args.model_name, args.push_to_hub)
| transformers/src/transformers/models/regnet/convert_regnet_seer_10b_to_pytorch.py/0 | {
"file_path": "transformers/src/transformers/models/regnet/convert_regnet_seer_10b_to_pytorch.py",
"repo_id": "transformers",
"token_count": 4917
} | 320 |
# coding=utf-8
# Copyright 2022 Microsoft Research, Inc. and The HuggingFace Inc. team. All rights reserved.
#
# Licensed under the Apache License, Version 2.0 (the "License");
# you may not use this file except in compliance with the License.
# You may obtain a copy of the License at
#
# http://www.apache.org/licenses/LICENSE-2.0
#
# Unless required by applicable law or agreed to in writing, software
# distributed under the License is distributed on an "AS IS" BASIS,
# WITHOUT WARRANTIES OR CONDITIONS OF ANY KIND, either express or implied.
# See the License for the specific language governing permissions and
# limitations under the License.
""" PyTorch ResNet model."""
from typing import Optional
import torch
import torch.utils.checkpoint
from torch import Tensor, nn
from torch.nn import BCEWithLogitsLoss, CrossEntropyLoss, MSELoss
from ...activations import ACT2FN
from ...modeling_outputs import (
BackboneOutput,
BaseModelOutputWithNoAttention,
BaseModelOutputWithPoolingAndNoAttention,
ImageClassifierOutputWithNoAttention,
)
from ...modeling_utils import PreTrainedModel
from ...utils import (
add_code_sample_docstrings,
add_start_docstrings,
add_start_docstrings_to_model_forward,
logging,
replace_return_docstrings,
)
from ...utils.backbone_utils import BackboneMixin
from .configuration_resnet import ResNetConfig
logger = logging.get_logger(__name__)
# General docstring
_CONFIG_FOR_DOC = "ResNetConfig"
# Base docstring
_CHECKPOINT_FOR_DOC = "microsoft/resnet-50"
_EXPECTED_OUTPUT_SHAPE = [1, 2048, 7, 7]
# Image classification docstring
_IMAGE_CLASS_CHECKPOINT = "microsoft/resnet-50"
_IMAGE_CLASS_EXPECTED_OUTPUT = "tiger cat"
RESNET_PRETRAINED_MODEL_ARCHIVE_LIST = [
"microsoft/resnet-50",
# See all resnet models at https://huggingface.co/models?filter=resnet
]
class ResNetConvLayer(nn.Module):
def __init__(
self, in_channels: int, out_channels: int, kernel_size: int = 3, stride: int = 1, activation: str = "relu"
):
super().__init__()
self.convolution = nn.Conv2d(
in_channels, out_channels, kernel_size=kernel_size, stride=stride, padding=kernel_size // 2, bias=False
)
self.normalization = nn.BatchNorm2d(out_channels)
self.activation = ACT2FN[activation] if activation is not None else nn.Identity()
def forward(self, input: Tensor) -> Tensor:
hidden_state = self.convolution(input)
hidden_state = self.normalization(hidden_state)
hidden_state = self.activation(hidden_state)
return hidden_state
class ResNetEmbeddings(nn.Module):
"""
ResNet Embeddings (stem) composed of a single aggressive convolution.
"""
def __init__(self, config: ResNetConfig):
super().__init__()
self.embedder = ResNetConvLayer(
config.num_channels, config.embedding_size, kernel_size=7, stride=2, activation=config.hidden_act
)
self.pooler = nn.MaxPool2d(kernel_size=3, stride=2, padding=1)
self.num_channels = config.num_channels
def forward(self, pixel_values: Tensor) -> Tensor:
num_channels = pixel_values.shape[1]
if num_channels != self.num_channels:
raise ValueError(
"Make sure that the channel dimension of the pixel values match with the one set in the configuration."
)
embedding = self.embedder(pixel_values)
embedding = self.pooler(embedding)
return embedding
class ResNetShortCut(nn.Module):
"""
ResNet shortcut, used to project the residual features to the correct size. If needed, it is also used to
downsample the input using `stride=2`.
"""
def __init__(self, in_channels: int, out_channels: int, stride: int = 2):
super().__init__()
self.convolution = nn.Conv2d(in_channels, out_channels, kernel_size=1, stride=stride, bias=False)
self.normalization = nn.BatchNorm2d(out_channels)
def forward(self, input: Tensor) -> Tensor:
hidden_state = self.convolution(input)
hidden_state = self.normalization(hidden_state)
return hidden_state
class ResNetBasicLayer(nn.Module):
"""
A classic ResNet's residual layer composed by two `3x3` convolutions.
"""
def __init__(self, in_channels: int, out_channels: int, stride: int = 1, activation: str = "relu"):
super().__init__()
should_apply_shortcut = in_channels != out_channels or stride != 1
self.shortcut = (
ResNetShortCut(in_channels, out_channels, stride=stride) if should_apply_shortcut else nn.Identity()
)
self.layer = nn.Sequential(
ResNetConvLayer(in_channels, out_channels, stride=stride),
ResNetConvLayer(out_channels, out_channels, activation=None),
)
self.activation = ACT2FN[activation]
def forward(self, hidden_state):
residual = hidden_state
hidden_state = self.layer(hidden_state)
residual = self.shortcut(residual)
hidden_state += residual
hidden_state = self.activation(hidden_state)
return hidden_state
class ResNetBottleNeckLayer(nn.Module):
"""
A classic ResNet's bottleneck layer composed by three `3x3` convolutions.
The first `1x1` convolution reduces the input by a factor of `reduction` in order to make the second `3x3`
convolution faster. The last `1x1` convolution remaps the reduced features to `out_channels`. If
`downsample_in_bottleneck` is true, downsample will be in the first layer instead of the second layer.
"""
def __init__(
self,
in_channels: int,
out_channels: int,
stride: int = 1,
activation: str = "relu",
reduction: int = 4,
downsample_in_bottleneck: bool = False,
):
super().__init__()
should_apply_shortcut = in_channels != out_channels or stride != 1
reduces_channels = out_channels // reduction
self.shortcut = (
ResNetShortCut(in_channels, out_channels, stride=stride) if should_apply_shortcut else nn.Identity()
)
self.layer = nn.Sequential(
ResNetConvLayer(
in_channels, reduces_channels, kernel_size=1, stride=stride if downsample_in_bottleneck else 1
),
ResNetConvLayer(reduces_channels, reduces_channels, stride=stride if not downsample_in_bottleneck else 1),
ResNetConvLayer(reduces_channels, out_channels, kernel_size=1, activation=None),
)
self.activation = ACT2FN[activation]
def forward(self, hidden_state):
residual = hidden_state
hidden_state = self.layer(hidden_state)
residual = self.shortcut(residual)
hidden_state += residual
hidden_state = self.activation(hidden_state)
return hidden_state
class ResNetStage(nn.Module):
"""
A ResNet stage composed by stacked layers.
"""
def __init__(
self,
config: ResNetConfig,
in_channels: int,
out_channels: int,
stride: int = 2,
depth: int = 2,
):
super().__init__()
layer = ResNetBottleNeckLayer if config.layer_type == "bottleneck" else ResNetBasicLayer
if config.layer_type == "bottleneck":
first_layer = layer(
in_channels,
out_channels,
stride=stride,
activation=config.hidden_act,
downsample_in_bottleneck=config.downsample_in_bottleneck,
)
else:
first_layer = layer(in_channels, out_channels, stride=stride, activation=config.hidden_act)
self.layers = nn.Sequential(
first_layer, *[layer(out_channels, out_channels, activation=config.hidden_act) for _ in range(depth - 1)]
)
def forward(self, input: Tensor) -> Tensor:
hidden_state = input
for layer in self.layers:
hidden_state = layer(hidden_state)
return hidden_state
class ResNetEncoder(nn.Module):
def __init__(self, config: ResNetConfig):
super().__init__()
self.stages = nn.ModuleList([])
# based on `downsample_in_first_stage` the first layer of the first stage may or may not downsample the input
self.stages.append(
ResNetStage(
config,
config.embedding_size,
config.hidden_sizes[0],
stride=2 if config.downsample_in_first_stage else 1,
depth=config.depths[0],
)
)
in_out_channels = zip(config.hidden_sizes, config.hidden_sizes[1:])
for (in_channels, out_channels), depth in zip(in_out_channels, config.depths[1:]):
self.stages.append(ResNetStage(config, in_channels, out_channels, depth=depth))
def forward(
self, hidden_state: Tensor, output_hidden_states: bool = False, return_dict: bool = True
) -> BaseModelOutputWithNoAttention:
hidden_states = () if output_hidden_states else None
for stage_module in self.stages:
if output_hidden_states:
hidden_states = hidden_states + (hidden_state,)
hidden_state = stage_module(hidden_state)
if output_hidden_states:
hidden_states = hidden_states + (hidden_state,)
if not return_dict:
return tuple(v for v in [hidden_state, hidden_states] if v is not None)
return BaseModelOutputWithNoAttention(
last_hidden_state=hidden_state,
hidden_states=hidden_states,
)
class ResNetPreTrainedModel(PreTrainedModel):
"""
An abstract class to handle weights initialization and a simple interface for downloading and loading pretrained
models.
"""
config_class = ResNetConfig
base_model_prefix = "resnet"
main_input_name = "pixel_values"
def _init_weights(self, module):
if isinstance(module, nn.Conv2d):
nn.init.kaiming_normal_(module.weight, mode="fan_out", nonlinearity="relu")
elif isinstance(module, (nn.BatchNorm2d, nn.GroupNorm)):
nn.init.constant_(module.weight, 1)
nn.init.constant_(module.bias, 0)
RESNET_START_DOCSTRING = r"""
This model is a PyTorch [torch.nn.Module](https://pytorch.org/docs/stable/nn.html#torch.nn.Module) subclass. Use it
as a regular PyTorch Module and refer to the PyTorch documentation for all matter related to general usage and
behavior.
Parameters:
config ([`ResNetConfig`]): Model configuration class with all the parameters of the model.
Initializing with a config file does not load the weights associated with the model, only the
configuration. Check out the [`~PreTrainedModel.from_pretrained`] method to load the model weights.
"""
RESNET_INPUTS_DOCSTRING = r"""
Args:
pixel_values (`torch.FloatTensor` of shape `(batch_size, num_channels, height, width)`):
Pixel values. Pixel values can be obtained using [`AutoImageProcessor`]. See
[`ConvNextImageProcessor.__call__`] for details.
output_hidden_states (`bool`, *optional*):
Whether or not to return the hidden states of all layers. See `hidden_states` under returned tensors for
more detail.
return_dict (`bool`, *optional*):
Whether or not to return a [`~utils.ModelOutput`] instead of a plain tuple.
"""
@add_start_docstrings(
"The bare ResNet model outputting raw features without any specific head on top.",
RESNET_START_DOCSTRING,
)
class ResNetModel(ResNetPreTrainedModel):
def __init__(self, config):
super().__init__(config)
self.config = config
self.embedder = ResNetEmbeddings(config)
self.encoder = ResNetEncoder(config)
self.pooler = nn.AdaptiveAvgPool2d((1, 1))
# Initialize weights and apply final processing
self.post_init()
@add_start_docstrings_to_model_forward(RESNET_INPUTS_DOCSTRING)
@add_code_sample_docstrings(
checkpoint=_CHECKPOINT_FOR_DOC,
output_type=BaseModelOutputWithPoolingAndNoAttention,
config_class=_CONFIG_FOR_DOC,
modality="vision",
expected_output=_EXPECTED_OUTPUT_SHAPE,
)
def forward(
self, pixel_values: Tensor, output_hidden_states: Optional[bool] = None, return_dict: Optional[bool] = None
) -> BaseModelOutputWithPoolingAndNoAttention:
output_hidden_states = (
output_hidden_states if output_hidden_states is not None else self.config.output_hidden_states
)
return_dict = return_dict if return_dict is not None else self.config.use_return_dict
embedding_output = self.embedder(pixel_values)
encoder_outputs = self.encoder(
embedding_output, output_hidden_states=output_hidden_states, return_dict=return_dict
)
last_hidden_state = encoder_outputs[0]
pooled_output = self.pooler(last_hidden_state)
if not return_dict:
return (last_hidden_state, pooled_output) + encoder_outputs[1:]
return BaseModelOutputWithPoolingAndNoAttention(
last_hidden_state=last_hidden_state,
pooler_output=pooled_output,
hidden_states=encoder_outputs.hidden_states,
)
@add_start_docstrings(
"""
ResNet Model with an image classification head on top (a linear layer on top of the pooled features), e.g. for
ImageNet.
""",
RESNET_START_DOCSTRING,
)
class ResNetForImageClassification(ResNetPreTrainedModel):
def __init__(self, config):
super().__init__(config)
self.num_labels = config.num_labels
self.resnet = ResNetModel(config)
# classification head
self.classifier = nn.Sequential(
nn.Flatten(),
nn.Linear(config.hidden_sizes[-1], config.num_labels) if config.num_labels > 0 else nn.Identity(),
)
# initialize weights and apply final processing
self.post_init()
@add_start_docstrings_to_model_forward(RESNET_INPUTS_DOCSTRING)
@add_code_sample_docstrings(
checkpoint=_IMAGE_CLASS_CHECKPOINT,
output_type=ImageClassifierOutputWithNoAttention,
config_class=_CONFIG_FOR_DOC,
expected_output=_IMAGE_CLASS_EXPECTED_OUTPUT,
)
def forward(
self,
pixel_values: Optional[torch.FloatTensor] = None,
labels: Optional[torch.LongTensor] = None,
output_hidden_states: Optional[bool] = None,
return_dict: Optional[bool] = None,
) -> ImageClassifierOutputWithNoAttention:
r"""
labels (`torch.LongTensor` of shape `(batch_size,)`, *optional*):
Labels for computing the image classification/regression loss. Indices should be in `[0, ...,
config.num_labels - 1]`. If `config.num_labels > 1` a classification loss is computed (Cross-Entropy).
"""
return_dict = return_dict if return_dict is not None else self.config.use_return_dict
outputs = self.resnet(pixel_values, output_hidden_states=output_hidden_states, return_dict=return_dict)
pooled_output = outputs.pooler_output if return_dict else outputs[1]
logits = self.classifier(pooled_output)
loss = None
if labels is not None:
if self.config.problem_type is None:
if self.num_labels == 1:
self.config.problem_type = "regression"
elif self.num_labels > 1 and (labels.dtype == torch.long or labels.dtype == torch.int):
self.config.problem_type = "single_label_classification"
else:
self.config.problem_type = "multi_label_classification"
if self.config.problem_type == "regression":
loss_fct = MSELoss()
if self.num_labels == 1:
loss = loss_fct(logits.squeeze(), labels.squeeze())
else:
loss = loss_fct(logits, labels)
elif self.config.problem_type == "single_label_classification":
loss_fct = CrossEntropyLoss()
loss = loss_fct(logits.view(-1, self.num_labels), labels.view(-1))
elif self.config.problem_type == "multi_label_classification":
loss_fct = BCEWithLogitsLoss()
loss = loss_fct(logits, labels)
if not return_dict:
output = (logits,) + outputs[2:]
return (loss,) + output if loss is not None else output
return ImageClassifierOutputWithNoAttention(loss=loss, logits=logits, hidden_states=outputs.hidden_states)
@add_start_docstrings(
"""
ResNet backbone, to be used with frameworks like DETR and MaskFormer.
""",
RESNET_START_DOCSTRING,
)
class ResNetBackbone(ResNetPreTrainedModel, BackboneMixin):
def __init__(self, config):
super().__init__(config)
super()._init_backbone(config)
self.num_features = [config.embedding_size] + config.hidden_sizes
self.embedder = ResNetEmbeddings(config)
self.encoder = ResNetEncoder(config)
# initialize weights and apply final processing
self.post_init()
@add_start_docstrings_to_model_forward(RESNET_INPUTS_DOCSTRING)
@replace_return_docstrings(output_type=BackboneOutput, config_class=_CONFIG_FOR_DOC)
def forward(
self, pixel_values: Tensor, output_hidden_states: Optional[bool] = None, return_dict: Optional[bool] = None
) -> BackboneOutput:
"""
Returns:
Examples:
```python
>>> from transformers import AutoImageProcessor, AutoBackbone
>>> import torch
>>> from PIL import Image
>>> import requests
>>> url = "http://images.cocodataset.org/val2017/000000039769.jpg"
>>> image = Image.open(requests.get(url, stream=True).raw)
>>> processor = AutoImageProcessor.from_pretrained("microsoft/resnet-50")
>>> model = AutoBackbone.from_pretrained(
... "microsoft/resnet-50", out_features=["stage1", "stage2", "stage3", "stage4"]
... )
>>> inputs = processor(image, return_tensors="pt")
>>> outputs = model(**inputs)
>>> feature_maps = outputs.feature_maps
>>> list(feature_maps[-1].shape)
[1, 2048, 7, 7]
```"""
return_dict = return_dict if return_dict is not None else self.config.use_return_dict
output_hidden_states = (
output_hidden_states if output_hidden_states is not None else self.config.output_hidden_states
)
embedding_output = self.embedder(pixel_values)
outputs = self.encoder(embedding_output, output_hidden_states=True, return_dict=True)
hidden_states = outputs.hidden_states
feature_maps = ()
for idx, stage in enumerate(self.stage_names):
if stage in self.out_features:
feature_maps += (hidden_states[idx],)
if not return_dict:
output = (feature_maps,)
if output_hidden_states:
output += (outputs.hidden_states,)
return output
return BackboneOutput(
feature_maps=feature_maps,
hidden_states=outputs.hidden_states if output_hidden_states else None,
attentions=None,
)
| transformers/src/transformers/models/resnet/modeling_resnet.py/0 | {
"file_path": "transformers/src/transformers/models/resnet/modeling_resnet.py",
"repo_id": "transformers",
"token_count": 8017
} | 321 |
# coding=utf-8
# Copyright 2023 Bo Peng and HuggingFace Inc. team.
# Copyright (c) 2018, NVIDIA CORPORATION. All rights reserved.
#
# Licensed under the Apache License, Version 2.0 (the "License");
# you may not use this file except in compliance with the License.
# You may obtain a copy of the License at
#
# http://www.apache.org/licenses/LICENSE-2.0
#
# Unless required by applicable law or agreed to in writing, software
# distributed under the License is distributed on an "AS IS" BASIS,
# WITHOUT WARRANTIES OR CONDITIONS OF ANY KIND, either express or implied.
# See the License for the specific language governing permissions and
# limitations under the License.
"""PyTorch RWKV model."""
import math
from dataclasses import dataclass
from pathlib import Path
from typing import List, Optional, Tuple, Union
import torch
import torch.utils.checkpoint
from torch import nn
from torch.nn import CrossEntropyLoss
from ...modeling_utils import PreTrainedModel
from ...utils import (
ModelOutput,
add_code_sample_docstrings,
add_start_docstrings,
add_start_docstrings_to_model_forward,
is_bitsandbytes_available,
is_ninja_available,
is_torch_cuda_available,
logging,
)
from .configuration_rwkv import RwkvConfig
logger = logging.get_logger(__name__)
_CHECKPOINT_FOR_DOC = "RWKV/rwkv-4-169m-pile"
_CONFIG_FOR_DOC = "RwkvConfig"
RWKV_PRETRAINED_MODEL_ARCHIVE_LIST = [
"RWKV/rwkv-4-169m-pile",
"RWKV/rwkv-4-430m-pile",
"RWKV/rwkv-4-1b5-pile",
"RWKV/rwkv-4-3b-pile",
"RWKV/rwkv-4-7b-pile",
"RWKV/rwkv-4-14b-pile",
"RWKV/rwkv-raven-1b5",
"RWKV/rwkv-raven-3b",
"RWKV/rwkv-raven-7b",
"RWKV/rwkv-raven-14b",
# See all RWKV models at https://huggingface.co/models?filter=rwkv
]
rwkv_cuda_kernel = None
def load_wkv_cuda_kernel(context_length):
from torch.utils.cpp_extension import load as load_kernel
global rwkv_cuda_kernel
kernel_folder = Path(__file__).resolve().parent.parent.parent / "kernels" / "rwkv"
cuda_kernel_files = [kernel_folder / f for f in ["wkv_op.cpp", "wkv_cuda.cu", "wkv_cuda_bf16.cu"]]
# Only load the kernel if it's not been loaded yet or if we changed the context length
if rwkv_cuda_kernel is not None and rwkv_cuda_kernel.max_seq_length == context_length:
return
logger.info(f"Loading CUDA kernel for RWKV at context length of {context_length}.")
flags = [
"-res-usage",
"--maxrregcount 60",
"--use_fast_math",
"-O3",
"-Xptxas -O3",
"--extra-device-vectorization",
f"-DTmax={context_length}",
]
rwkv_cuda_kernel = load_kernel(
name=f"wkv_{context_length}",
sources=cuda_kernel_files,
verbose=(logging.get_verbosity() == logging.DEBUG),
extra_cuda_cflags=flags,
)
rwkv_cuda_kernel.max_seq_length = context_length
class RwkvLinearAttention(torch.autograd.Function):
@staticmethod
def forward(ctx, time_decay, time_first, key, value, state=None, return_state=False):
batch_size, seq_len, hidden_size = key.size()
if seq_len > rwkv_cuda_kernel.max_seq_length:
raise ValueError(
f"Cannot process a batch with {seq_len} tokens at the same time, use a maximum of "
f"{rwkv_cuda_kernel.max_seq_length} with this model."
)
if batch_size * hidden_size % min(hidden_size, 32) != 0:
raise ValueError(
f"The product of batch size ({batch_size}) and hidden size ({hidden_size}) needs to be a round "
f"multiple of {min(hidden_size, 32)}."
)
ctx.input_dtype = key.dtype
if (
time_decay.device.type != "cuda"
or time_first.device.type != "cuda"
or key.device.type != "cuda"
or value.device.type != "cuda"
):
raise ValueError("Calling the CUDA kernel for wkv attention requires all tensors to be on CUDA devices.")
time_decay = -torch.exp(time_decay.float().contiguous())
if key.dtype == torch.float16:
time_first = time_first.float()
key = key.float()
value = value.float()
time_first = time_first.contiguous()
key = key.contiguous()
value = value.contiguous()
# The CUDA kernel will fill this tensor.
output = torch.empty_like(key, memory_format=torch.contiguous_format)
if return_state or state is not None:
if state is None:
state = torch.zeros(
batch_size,
hidden_size,
3,
dtype=torch.float32,
device=key.device,
memory_format=torch.contiguous_format,
)
state[:, :, 2] -= 1e38
else:
state = torch.cat([s.unsqueeze(2) for s in state], dim=2).contiguous()
if key.dtype == torch.bfloat16:
forward_func = rwkv_cuda_kernel.forward_with_state_bf16
else:
forward_func = rwkv_cuda_kernel.forward_with_state
forward_func(time_decay, time_first, key, value, output, state)
else:
forward_func = rwkv_cuda_kernel.forward_bf16 if key.dtype == torch.bfloat16 else rwkv_cuda_kernel.forward
forward_func(time_decay, time_first, key, value, output)
ctx.save_for_backward(time_decay, time_first, key, value, output)
if state is not None:
state = [s.squeeze(2) for s in torch.chunk(state, 3, dim=2)]
return output.to(ctx.input_dtype), state
@staticmethod
# g stands for grad
def backward(ctx, g_output, g_state=None):
input_dtype = ctx.input_dtype
time_decay, time_first, key, value, output = ctx.saved_tensors
# The CUDA kernel will fill those tensors.
g_time_decay = torch.empty_like(
time_decay,
memory_format=torch.contiguous_format,
dtype=torch.bfloat16 if input_dtype == torch.bfloat16 else torch.float32,
)
g_time_first = torch.empty_like(time_first, memory_format=torch.contiguous_format)
g_key = torch.empty_like(key, memory_format=torch.contiguous_format)
g_value = torch.empty_like(value, memory_format=torch.contiguous_format)
if input_dtype == torch.float16:
g_output = g_output.float()
backward_func = rwkv_cuda_kernel.backward_bf16 if input_dtype == torch.bfloat16 else rwkv_cuda_kernel.backward
backward_func(
time_decay,
time_first,
key,
value,
output,
g_output.contiguous(),
g_time_decay,
g_time_first,
g_key,
g_value,
)
return (
g_time_decay.to(input_dtype),
g_time_first.to(input_dtype),
g_key.to(input_dtype),
g_value.to(input_dtype),
None,
None,
)
def rwkv_linear_attention_cpu(time_decay, time_first, key, value, state=None, return_state=False):
# For CPU fallback. Will be slower and probably take more memory than the custom CUDA kernel if not executed
# within a torch.no_grad.
_, seq_length, _ = key.size()
output = torch.zeros_like(key)
if state is None:
num_state = torch.zeros_like(key[:, 0], dtype=torch.float32)
den_state = torch.zeros_like(key[:, 0], dtype=torch.float32)
max_state = torch.zeros_like(key[:, 0], dtype=torch.float32) - 1e38
else:
num_state, den_state, max_state = state
# For numerical stability
# real_numerator_state = num_state * torch.exp(max_state)
# real_denominator_state = den_state * torch.exp(max_state)
time_decay = -torch.exp(time_decay)
for current_index in range(seq_length):
current_key = key[:, current_index].float()
current_value = value[:, current_index]
# wkv computation at time t
max_for_output = torch.maximum(max_state, current_key + time_first)
e1 = torch.exp(max_state - max_for_output)
e2 = torch.exp(current_key + time_first - max_for_output)
numerator = e1 * num_state + e2 * current_value
denominator = e1 * den_state + e2
output[:, current_index] = (numerator / denominator).to(output.dtype)
# Update state for next iteration
max_for_state = torch.maximum(max_state + time_decay, current_key)
e1 = torch.exp(max_state + time_decay - max_for_state)
e2 = torch.exp(current_key - max_for_state)
num_state = e1 * num_state + e2 * current_value
den_state = e1 * den_state + e2
max_state = max_for_state
if return_state or state is not None:
state = [num_state, den_state, max_state]
return output, state
def rwkv_linear_attention(time_decay, time_first, key, value, state=None, return_state=False):
no_cuda = any(t.device.type != "cuda" for t in [time_decay, time_first, key, value])
# Launching the CUDA kernel for just one token will actually be slower (there is no for loop in the CPU version
# in this case).
one_token = key.size(1) == 1
if rwkv_cuda_kernel is None or no_cuda or one_token:
return rwkv_linear_attention_cpu(time_decay, time_first, key, value, state=state, return_state=return_state)
else:
return RwkvLinearAttention.apply(time_decay, time_first, key, value, state, return_state)
class RwkvSelfAttention(nn.Module):
def __init__(self, config, layer_id=0):
super().__init__()
self.config = config
kernel_loaded = rwkv_cuda_kernel is not None and rwkv_cuda_kernel.max_seq_length == config.context_length
if is_ninja_available() and is_torch_cuda_available() and not kernel_loaded:
try:
load_wkv_cuda_kernel(config.context_length)
except Exception:
logger.info("Could not load the custom CUDA kernel for RWKV attention.")
self.layer_id = layer_id
hidden_size = config.hidden_size
attention_hidden_size = (
config.attention_hidden_size if config.attention_hidden_size is not None else hidden_size
)
self.attention_hidden_size = attention_hidden_size
self.time_decay = nn.Parameter(torch.empty(attention_hidden_size))
self.time_first = nn.Parameter(torch.empty(attention_hidden_size))
self.time_mix_key = nn.Parameter(torch.empty(1, 1, hidden_size))
self.time_mix_value = nn.Parameter(torch.empty(1, 1, hidden_size))
self.time_mix_receptance = nn.Parameter(torch.empty(1, 1, hidden_size))
self.time_shift = nn.ZeroPad2d((0, 0, 1, -1))
self.key = nn.Linear(hidden_size, attention_hidden_size, bias=False)
self.value = nn.Linear(hidden_size, attention_hidden_size, bias=False)
self.receptance = nn.Linear(hidden_size, attention_hidden_size, bias=False)
self.output = nn.Linear(attention_hidden_size, hidden_size, bias=False)
# TODO: maybe jit, otherwise move inside forward
def extract_key_value(self, hidden, state=None):
# Mix hidden with the previous timestep to produce key, value, receptance
if hidden.size(1) == 1 and state is not None:
shifted = state[1][:, :, self.layer_id]
else:
shifted = self.time_shift(hidden)
if state is not None:
shifted[:, 0] = state[1][:, :, self.layer_id]
key = hidden * self.time_mix_key + shifted * (1 - self.time_mix_key)
value = hidden * self.time_mix_value + shifted * (1 - self.time_mix_value)
receptance = hidden * self.time_mix_receptance + shifted * (1 - self.time_mix_receptance)
key = self.key(key)
value = self.value(value)
receptance = torch.sigmoid(self.receptance(receptance))
if state is not None:
state[1][:, :, self.layer_id] = hidden[:, -1]
return receptance, key, value, state
def forward(self, hidden, state=None, use_cache=False):
receptance, key, value, state = self.extract_key_value(hidden, state=state)
layer_state = tuple(s[:, :, self.layer_id] for s in state[2:]) if state is not None else None
rwkv, layer_state = rwkv_linear_attention(
self.time_decay,
self.time_first,
key,
value,
state=layer_state,
return_state=use_cache,
)
if layer_state is not None:
state[2][:, :, self.layer_id] = layer_state[0]
state[3][:, :, self.layer_id] = layer_state[1]
state[4][:, :, self.layer_id] = layer_state[2]
return self.output(receptance * rwkv), state
class RwkvFeedForward(nn.Module):
def __init__(self, config, layer_id=0):
super().__init__()
self.config = config
self.layer_id = layer_id
hidden_size = config.hidden_size
intermediate_size = (
config.intermediate_size if config.intermediate_size is not None else 4 * config.hidden_size
)
self.time_shift = nn.ZeroPad2d((0, 0, 1, -1))
self.time_mix_key = nn.Parameter(torch.empty(1, 1, hidden_size))
self.time_mix_receptance = nn.Parameter(torch.empty(1, 1, hidden_size))
self.key = nn.Linear(hidden_size, intermediate_size, bias=False)
self.receptance = nn.Linear(hidden_size, hidden_size, bias=False)
self.value = nn.Linear(intermediate_size, hidden_size, bias=False)
def forward(self, hidden, state=None):
if hidden.size(1) == 1 and state is not None:
shifted = state[0][:, :, self.layer_id]
else:
shifted = self.time_shift(hidden)
if state is not None:
shifted[:, 0] = state[0][:, :, self.layer_id]
key = hidden * self.time_mix_key + shifted * (1 - self.time_mix_key)
receptance = hidden * self.time_mix_receptance + shifted * (1 - self.time_mix_receptance)
key = torch.square(torch.relu(self.key(key)))
value = self.value(key)
receptance = torch.sigmoid(self.receptance(receptance))
if state is not None:
state[0][:, :, self.layer_id] = hidden[:, -1]
return receptance * value, state
class RwkvBlock(nn.Module):
def __init__(self, config, layer_id):
super().__init__()
self.config = config
self.layer_id = layer_id
if layer_id == 0:
self.pre_ln = nn.LayerNorm(config.hidden_size, eps=config.layer_norm_epsilon)
self.ln1 = nn.LayerNorm(config.hidden_size, eps=config.layer_norm_epsilon)
self.ln2 = nn.LayerNorm(config.hidden_size, eps=config.layer_norm_epsilon)
self.attention = RwkvSelfAttention(config, layer_id)
self.feed_forward = RwkvFeedForward(config, layer_id)
def forward(self, hidden, state=None, use_cache=False, output_attentions=False):
if self.layer_id == 0:
hidden = self.pre_ln(hidden)
attention, state = self.attention(self.ln1(hidden), state=state, use_cache=use_cache)
hidden = hidden + attention
feed_forward, state = self.feed_forward(self.ln2(hidden), state=state)
hidden = hidden + feed_forward
outputs = (hidden, state)
if output_attentions:
outputs += (attention,)
else:
outputs += (None,)
return outputs
class RwkvPreTrainedModel(PreTrainedModel):
"""
An abstract class to handle weights initialization and a simple interface for downloading and loading pretrained
models.
"""
config_class = RwkvConfig
base_model_prefix = "rwkv"
_no_split_modules = ["RwkvBlock"]
_keep_in_fp32_modules = ["time_decay", "time_first"]
supports_gradient_checkpointing = True
def _init_weights(self, module):
"""Initialize the weights."""
if isinstance(module, RwkvSelfAttention):
layer_id = module.layer_id
num_hidden_layers = module.config.num_hidden_layers
hidden_size = module.config.hidden_size
attention_hidden_size = module.attention_hidden_size
ratio_0_to_1 = layer_id / (num_hidden_layers - 1) # 0 to 1
ratio_1_to_almost0 = 1.0 - (layer_id / num_hidden_layers) # 1 to ~0
time_weight = torch.tensor(
[i / hidden_size for i in range(hidden_size)],
dtype=module.time_mix_key.dtype,
device=module.time_mix_key.device,
)
time_weight = time_weight[None, None, :]
decay_speed = [
-5 + 8 * (h / (attention_hidden_size - 1)) ** (0.7 + 1.3 * ratio_0_to_1)
for h in range(attention_hidden_size)
]
decay_speed = torch.tensor(decay_speed, dtype=module.time_decay.dtype, device=module.time_decay.device)
zigzag = (
torch.tensor(
[(i + 1) % 3 - 1 for i in range(attention_hidden_size)],
dtype=module.time_first.dtype,
device=module.time_first.device,
)
* 0.5
)
with torch.no_grad():
module.time_decay.data = decay_speed
module.time_first.data = torch.ones_like(module.time_first * math.log(0.3) + zigzag)
module.time_mix_key.data = torch.pow(time_weight, ratio_1_to_almost0)
module.time_mix_value.data = torch.pow(time_weight, ratio_1_to_almost0) + 0.3 * ratio_0_to_1
module.time_mix_receptance.data = torch.pow(time_weight, 0.5 * ratio_1_to_almost0)
elif isinstance(module, RwkvFeedForward):
layer_id = module.layer_id
num_hidden_layers = module.config.num_hidden_layers
hidden_size = module.config.hidden_size
ratio_1_to_almost0 = 1.0 - (layer_id / num_hidden_layers) # 1 to ~0
time_weight = torch.tensor(
[i / hidden_size for i in range(hidden_size)],
dtype=module.time_mix_key.dtype,
device=module.time_mix_key.device,
)
time_weight = time_weight[None, None, :]
with torch.no_grad():
module.time_mix_key.data = torch.pow(time_weight, ratio_1_to_almost0)
module.time_mix_receptance.data = torch.pow(time_weight, ratio_1_to_almost0)
@dataclass
class RwkvOutput(ModelOutput):
"""
Class for the RWKV model outputs.
Args:
last_hidden_state (`torch.FloatTensor` of shape `(batch_size, sequence_length, hidden_size)`):
Sequence of hidden-states at the output of the last layer of the model.
state (list of five `torch.FloatTensor` of shape `(batch_size, hidden_size, num_hidden_layers)`):
The state of the model at the last time step. Can be used in a forward method with the next `input_ids` to
avoid providing the old `input_ids`.
hidden_states (`tuple(torch.FloatTensor)`, *optional*, returned when `output_hidden_states=True` is passed or when `config.output_hidden_states=True`):
Tuple of `torch.FloatTensor` (one for the output of the embeddings, if the model has an embedding layer, +
one for the output of each layer) of shape `(batch_size, sequence_length, hidden_size)`.
Hidden-states of the model at the output of each layer plus the optional initial embedding outputs.
attentions (`tuple(torch.FloatTensor)`, *optional*, returned when `output_attentions=True` is passed or when `config.output_attentions=True`):
Tuple of `torch.FloatTensor` (one for each layer) of shape `(batch_size, num_heads, sequence_length,
sequence_length)`.
Attentions weights after the attention softmax, used to compute the weighted average in the self-attention
heads.
"""
last_hidden_state: torch.FloatTensor = None
state: Optional[List[torch.FloatTensor]] = None
hidden_states: Optional[Tuple[torch.FloatTensor, ...]] = None
attentions: Optional[Tuple[torch.FloatTensor, ...]] = None
@dataclass
class RwkvCausalLMOutput(ModelOutput):
"""
Base class for causal language model (or autoregressive) outputs.
Args:
loss (`torch.FloatTensor` of shape `(1,)`, *optional*, returned when `labels` is provided):
Language modeling loss (for next-token prediction).
logits (`torch.FloatTensor` of shape `(batch_size, sequence_length, config.vocab_size)`):
Prediction scores of the language modeling head (scores for each vocabulary token before SoftMax).
state (list of five `torch.FloatTensor` of shape `(batch_size, hidden_size, num_hidden_layers)`):
The state of the model at the last time step. Can be used in a forward method with the next `input_ids` to
avoid providing the old `input_ids`.
hidden_states (`tuple(torch.FloatTensor)`, *optional*, returned when `output_hidden_states=True` is passed or when `config.output_hidden_states=True`):
Tuple of `torch.FloatTensor` (one for the output of the embeddings, if the model has an embedding layer, +
one for the output of each layer) of shape `(batch_size, sequence_length, hidden_size)`.
Hidden-states of the model at the output of each layer plus the optional initial embedding outputs.
attentions (`tuple(torch.FloatTensor)`, *optional*, returned when `output_attentions=True` is passed or when `config.output_attentions=True`):
Tuple of `torch.FloatTensor` (one for each layer) of shape `(batch_size, num_heads, sequence_length,
sequence_length)`.
Attentions weights after the attention softmax, used to compute the weighted average in the self-attention
heads.
"""
loss: Optional[torch.FloatTensor] = None
logits: torch.FloatTensor = None
state: Optional[List[torch.FloatTensor]] = None
hidden_states: Optional[Tuple[torch.FloatTensor, ...]] = None
attentions: Optional[Tuple[torch.FloatTensor, ...]] = None
RWKV_START_DOCSTRING = r"""
This model inherits from [`PreTrainedModel`]. Check the superclass documentation for the generic methods the
library implements for all its model (such as downloading or saving, resizing the input embeddings, pruning heads
etc.)
This model is also a PyTorch [torch.nn.Module](https://pytorch.org/docs/stable/nn.html#torch.nn.Module) subclass.
Use it as a regular PyTorch Module and refer to the PyTorch documentation for all matter related to general usage
and behavior.
Parameters:
config ([`RwkvConfig`]): Model configuration class with all the parameters of the model.
Initializing with a config file does not load the weights associated with the model, only the
configuration. Check out the [`~PreTrainedModel.from_pretrained`] method to load the model weights.
"""
RWKV_INPUTS_DOCSTRING = r"""
Args:
input_ids (`torch.LongTensor` of shape `(batch_size, input_ids_length)`):
`input_ids_length` = `sequence_length` if `past_key_values` is `None` else
`past_key_values[0][0].shape[-2]` (`sequence_length` of input past key value states). Indices of input
sequence tokens in the vocabulary.
If `past_key_values` is used, only `input_ids` that do not have their past calculated should be passed as
`input_ids`.
Indices can be obtained using [`AutoTokenizer`]. See [`PreTrainedTokenizer.encode`] and
[`PreTrainedTokenizer.__call__`] for details.
[What are input IDs?](../glossary#input-ids)
attention_mask (`torch.LongTensor` of shape `(batch_size, input_ids_length)`, *optional*):
Mask to avoid performing attention on padding token indices. Mask values selected in `[0, 1]`:
- 1 for tokens that are **not masked**,
- 0 for tokens that are **masked**.
This is currently not used by `RwkvModel`, but will be supported in the future.
[What are attention masks?](../glossary#attention-mask)
inputs_embeds (`torch.FloatTensor` of shape `(batch_size, sequence_length, hidden_size)`, *optional*):
Optionally, instead of passing `input_ids` you can choose to directly pass an embedded representation. This
is useful if you want more control over how to convert `input_ids` indices into associated vectors than the
model's internal embedding lookup matrix.
state (tuple of five `torch.FloatTensor` of shape `(batch_size, hidden_size, num_hidden_layers)`, *optional*):
If passed along, the model uses the previous state in all the blocks (which will give the output for the
`input_ids` provided as if the model add `state_input_ids + input_ids` as context).
use_cache (`bool`, *optional*):
If set to `True`, the last state is returned and can be used to quickly generate the next logits.
output_attentions (`bool`, *optional*):
Whether or not to return the attentions tensors of all attention layers. See `attentions` under returned
tensors for more detail.
output_hidden_states (`bool`, *optional*):
Whether or not to return the hidden states of all layers. See `hidden_states` under returned tensors for
more detail.
return_dict (`bool`, *optional*):
Whether or not to return a [`~utils.ModelOutput`] instead of a plain tuple.
"""
@add_start_docstrings(
"The bare RWKV Model transformer outputting raw hidden-states without any specific head on top.",
RWKV_START_DOCSTRING,
)
class RwkvModel(RwkvPreTrainedModel):
def __init__(self, config):
super().__init__(config)
self.embeddings = nn.Embedding(config.vocab_size, config.hidden_size)
self.blocks = nn.ModuleList([RwkvBlock(config, layer_id=idx) for idx in range(config.num_hidden_layers)])
self.ln_out = nn.LayerNorm(config.hidden_size)
self.layers_are_rescaled = False
self.gradient_checkpointing = False
# Initialize weights and apply final processing
self.post_init()
def get_input_embeddings(self):
return self.embeddings
def set_input_embeddings(self, new_embeddings):
self.embeddings = new_embeddings
@add_start_docstrings_to_model_forward(RWKV_INPUTS_DOCSTRING)
@add_code_sample_docstrings(
checkpoint=_CHECKPOINT_FOR_DOC,
output_type=RwkvOutput,
config_class=_CONFIG_FOR_DOC,
)
def forward(
self,
input_ids: Optional[torch.LongTensor] = None,
attention_mask: Optional[torch.LongTensor] = None, # noqa
inputs_embeds: Optional[torch.FloatTensor] = None,
state: Optional[List[torch.FloatTensor]] = None,
use_cache: Optional[bool] = None,
output_attentions: Optional[bool] = None,
output_hidden_states: Optional[bool] = None,
return_dict: Optional[bool] = None,
) -> Union[Tuple, RwkvOutput]:
output_attentions = output_attentions if output_attentions is not None else self.config.output_attentions
output_hidden_states = (
output_hidden_states if output_hidden_states is not None else self.config.output_hidden_states
)
use_cache = use_cache if use_cache is not None else (self.config.use_cache if not self.training else False)
return_dict = return_dict if return_dict is not None else self.config.use_return_dict
if self.training == self.layers_are_rescaled:
self._rescale_layers()
if input_ids is not None and inputs_embeds is not None:
raise ValueError("You cannot specify both input_ids and inputs_embeds at the same time")
elif input_ids is None and inputs_embeds is None:
raise ValueError("You have to specify either input_ids or inputs_embeds")
if inputs_embeds is None:
inputs_embeds = self.embeddings(input_ids)
if use_cache and state is None:
shape = (inputs_embeds.size(0), self.config.hidden_size, self.config.num_hidden_layers)
state = [
torch.zeros(
*shape, dtype=inputs_embeds.dtype if i <= 1 else torch.float32, device=inputs_embeds.device
)
for i in range(5)
]
state[4] -= 1e30
if self.gradient_checkpointing and self.training:
if use_cache:
logger.warning_once(
"`use_cache=True` is incompatible with gradient checkpointing. Setting `use_cache=False`..."
)
use_cache = False
hidden_states = inputs_embeds
all_self_attentions = () if output_attentions else None
all_hidden_states = () if output_hidden_states else None
for idx, block in enumerate(self.blocks):
if self.gradient_checkpointing and self.training:
hidden_states, state, attentions = self._gradient_checkpointing_func(
block.__call__, hidden_states, state, use_cache, output_attentions
)
else:
hidden_states, state, attentions = block(
hidden_states, state=state, use_cache=use_cache, output_attentions=output_attentions
)
if (
self.layers_are_rescaled
and self.config.rescale_every > 0
and (idx + 1) % self.config.rescale_every == 0
):
hidden_states = hidden_states / 2
if output_hidden_states:
all_hidden_states = all_hidden_states + (hidden_states,)
if output_attentions:
all_self_attentions = all_self_attentions + (attentions,)
hidden_states = self.ln_out(hidden_states)
if output_hidden_states:
all_hidden_states = all_hidden_states + (hidden_states,)
if not return_dict:
return tuple(x for x in [hidden_states, state, all_hidden_states, all_self_attentions] if x is not None)
return RwkvOutput(
last_hidden_state=hidden_states,
state=state,
hidden_states=all_hidden_states,
attentions=all_self_attentions,
)
def _rescale_layers(self):
# Layers should be rescaled for inference only.
if self.layers_are_rescaled == (not self.training):
return
if self.config.rescale_every > 0:
with torch.no_grad():
for block_id, block in enumerate(self.blocks):
if self.training:
block.attention.output.weight.mul_(2 ** int(block_id // self.config.rescale_every))
block.feed_forward.value.weight.mul_(2 ** int(block_id // self.config.rescale_every))
else:
# Deal with quantization statistics
if hasattr(block.attention.output.weight, "SCB"):
block.attention.output.weight.SCB.div_(2 ** int(block_id // self.config.rescale_every))
block.feed_forward.value.weight.SCB.div_(2 ** int(block_id // self.config.rescale_every))
elif hasattr(block.attention.output.weight, "quant_state"):
self._bnb_4bit_dequantize_and_rescale(block.attention.output, block_id)
self._bnb_4bit_dequantize_and_rescale(block.feed_forward.value, block_id)
else:
block.attention.output.weight.div_(2 ** int(block_id // self.config.rescale_every))
block.feed_forward.value.weight.div_(2 ** int(block_id // self.config.rescale_every))
self.layers_are_rescaled = not self.training
def _bnb_4bit_dequantize_and_rescale(self, target_layer, block_id):
r"""
Perform the dequantization and rescaling of the weights of a given layer. After that operation the layer will
be quantized again.
"""
if not is_bitsandbytes_available():
raise ImportError("Please install bitsandbytes to use this method.")
import bitsandbytes as bnb
dequant_weights = bnb.functional.dequantize_4bit(target_layer.weight.data, target_layer.weight.quant_state)
dequant_weights.div_(2 ** int(block_id // self.config.rescale_every))
# re-quantize the model:
# we need to put it first on CPU then back to the device
# this will create an overhead :/
# We set requires_grad=False as we cannot compute gradients on top of 4bit parameters anyway and to avoid
# bugs with bnb
quant_weight = bnb.nn.Params4bit(dequant_weights.to("cpu"), requires_grad=False).to(dequant_weights.device)
setattr(target_layer, "weight", quant_weight)
@add_start_docstrings(
"""
The RWKV Model transformer with a language modeling head on top (linear layer with weights tied to the input
embeddings).
""",
RWKV_START_DOCSTRING,
)
class RwkvForCausalLM(RwkvPreTrainedModel):
_tied_weights_keys = ["head.weight"]
def __init__(self, config):
super().__init__(config)
self.rwkv = RwkvModel(config)
self.head = nn.Linear(config.hidden_size, config.vocab_size, bias=False)
# Initialize weights and apply final processing
self.post_init()
def get_output_embeddings(self):
return self.head
def set_output_embeddings(self, new_embeddings):
self.head = new_embeddings
def generate(self, *args, **kwargs):
# Thin wrapper to raise exceptions when trying to generate with methods that manipulate `past_key_values`.
# RWKV is one of the few models that don't have it (it has `state` instead, which has different properties and
# usage).
try:
gen_output = super().generate(*args, **kwargs)
except AttributeError as exc:
# Expected exception: "AttributeError: '(object name)' object has no attribute 'past_key_values'"
if "past_key_values" in str(exc):
raise AttributeError(
"You tried to call `generate` with a decoding strategy that manipulates `past_key_values`. RWKV "
"doesn't have that attribute, try another generation strategy instead. For the available "
"generation strategies, check this doc: https://huggingface.co/docs/transformers/en/generation_strategies#decoding-strategies"
)
else:
raise exc
return gen_output
def prepare_inputs_for_generation(self, input_ids, state=None, inputs_embeds=None, **kwargs):
# only last token for inputs_ids if the state is passed along.
if state is not None:
input_ids = input_ids[:, -1].unsqueeze(-1)
# if `inputs_embeds` are passed, we only want to use them in the 1st generation step
if inputs_embeds is not None and state is None:
model_inputs = {"inputs_embeds": inputs_embeds}
else:
model_inputs = {"input_ids": input_ids}
model_inputs["state"] = state
return model_inputs
@add_start_docstrings_to_model_forward(RWKV_INPUTS_DOCSTRING)
@add_code_sample_docstrings(
checkpoint=_CHECKPOINT_FOR_DOC,
output_type=RwkvCausalLMOutput,
config_class=_CONFIG_FOR_DOC,
)
def forward(
self,
input_ids: Optional[torch.LongTensor] = None,
attention_mask: Optional[torch.LongTensor] = None, # noqa
inputs_embeds: Optional[torch.FloatTensor] = None,
state: Optional[List[torch.FloatTensor]] = None,
labels: Optional[torch.LongTensor] = None,
use_cache: Optional[bool] = None,
output_attentions: Optional[bool] = None,
output_hidden_states: Optional[bool] = None,
return_dict: Optional[bool] = None,
) -> Union[Tuple, RwkvCausalLMOutput]:
r"""
labels (`torch.LongTensor` of shape `(batch_size, sequence_length)`, *optional*):
Labels for language modeling. Note that the labels **are shifted** inside the model, i.e. you can set
`labels = input_ids` Indices are selected in `[-100, 0, ..., config.vocab_size]` All labels set to `-100`
are ignored (masked), the loss is only computed for labels in `[0, ..., config.vocab_size]`
"""
return_dict = return_dict if return_dict is not None else self.config.use_return_dict
rwkv_outputs = self.rwkv(
input_ids,
inputs_embeds=inputs_embeds,
state=state,
use_cache=use_cache,
output_attentions=output_attentions,
output_hidden_states=output_hidden_states,
return_dict=return_dict,
)
hidden_states = rwkv_outputs[0]
logits = self.head(hidden_states)
loss = None
if labels is not None:
# move labels to correct device to enable model parallelism
labels = labels.to(logits.device)
# Shift so that tokens < n predict n
shift_logits = logits[..., :-1, :].contiguous()
shift_labels = labels[..., 1:].contiguous()
# Flatten the tokens
loss_fct = CrossEntropyLoss()
loss = loss_fct(shift_logits.view(-1, shift_logits.size(-1)), shift_labels.view(-1))
if not return_dict:
output = (logits,) + rwkv_outputs[1:]
return ((loss,) + output) if loss is not None else output
return RwkvCausalLMOutput(
loss=loss,
logits=logits,
state=rwkv_outputs.state,
hidden_states=rwkv_outputs.hidden_states,
attentions=rwkv_outputs.attentions,
)
| transformers/src/transformers/models/rwkv/modeling_rwkv.py/0 | {
"file_path": "transformers/src/transformers/models/rwkv/modeling_rwkv.py",
"repo_id": "transformers",
"token_count": 16622
} | 322 |
# Copyright 2021 The HuggingFace Team. All rights reserved.
#
# Licensed under the Apache License, Version 2.0 (the "License");
# you may not use this file except in compliance with the License.
# You may obtain a copy of the License at
#
# http://www.apache.org/licenses/LICENSE-2.0
#
# Unless required by applicable law or agreed to in writing, software
# distributed under the License is distributed on an "AS IS" BASIS,
# WITHOUT WARRANTIES OR CONDITIONS OF ANY KIND, either express or implied.
# See the License for the specific language governing permissions and
# limitations under the License.
from typing import TYPE_CHECKING
from ...utils import (
OptionalDependencyNotAvailable,
_LazyModule,
is_sentencepiece_available,
is_tf_available,
is_torch_available,
)
_import_structure = {
"configuration_speech_to_text": ["SPEECH_TO_TEXT_PRETRAINED_CONFIG_ARCHIVE_MAP", "Speech2TextConfig"],
"feature_extraction_speech_to_text": ["Speech2TextFeatureExtractor"],
"processing_speech_to_text": ["Speech2TextProcessor"],
}
try:
if not is_sentencepiece_available():
raise OptionalDependencyNotAvailable()
except OptionalDependencyNotAvailable:
pass
else:
_import_structure["tokenization_speech_to_text"] = ["Speech2TextTokenizer"]
try:
if not is_tf_available():
raise OptionalDependencyNotAvailable()
except OptionalDependencyNotAvailable:
pass
else:
_import_structure["modeling_tf_speech_to_text"] = [
"TF_SPEECH_TO_TEXT_PRETRAINED_MODEL_ARCHIVE_LIST",
"TFSpeech2TextForConditionalGeneration",
"TFSpeech2TextModel",
"TFSpeech2TextPreTrainedModel",
]
try:
if not is_torch_available():
raise OptionalDependencyNotAvailable()
except OptionalDependencyNotAvailable:
pass
else:
_import_structure["modeling_speech_to_text"] = [
"SPEECH_TO_TEXT_PRETRAINED_MODEL_ARCHIVE_LIST",
"Speech2TextForConditionalGeneration",
"Speech2TextModel",
"Speech2TextPreTrainedModel",
]
if TYPE_CHECKING:
from .configuration_speech_to_text import SPEECH_TO_TEXT_PRETRAINED_CONFIG_ARCHIVE_MAP, Speech2TextConfig
from .feature_extraction_speech_to_text import Speech2TextFeatureExtractor
from .processing_speech_to_text import Speech2TextProcessor
try:
if not is_sentencepiece_available():
raise OptionalDependencyNotAvailable()
except OptionalDependencyNotAvailable:
pass
else:
from .tokenization_speech_to_text import Speech2TextTokenizer
try:
if not is_tf_available():
raise OptionalDependencyNotAvailable()
except OptionalDependencyNotAvailable:
pass
else:
from .modeling_tf_speech_to_text import (
TF_SPEECH_TO_TEXT_PRETRAINED_MODEL_ARCHIVE_LIST,
TFSpeech2TextForConditionalGeneration,
TFSpeech2TextModel,
TFSpeech2TextPreTrainedModel,
)
try:
if not is_torch_available():
raise OptionalDependencyNotAvailable()
except OptionalDependencyNotAvailable:
pass
else:
from .modeling_speech_to_text import (
SPEECH_TO_TEXT_PRETRAINED_MODEL_ARCHIVE_LIST,
Speech2TextForConditionalGeneration,
Speech2TextModel,
Speech2TextPreTrainedModel,
)
else:
import sys
sys.modules[__name__] = _LazyModule(__name__, globals()["__file__"], _import_structure, module_spec=__spec__)
| transformers/src/transformers/models/speech_to_text/__init__.py/0 | {
"file_path": "transformers/src/transformers/models/speech_to_text/__init__.py",
"repo_id": "transformers",
"token_count": 1361
} | 323 |
# coding=utf-8
# Copyright 2023 The HuggingFace Inc. team. All rights reserved.
#
# Licensed under the Apache License, Version 2.0 (the "License");
# you may not use this file except in compliance with the License.
# You may obtain a copy of the License at
#
# http://www.apache.org/licenses/LICENSE-2.0
#
# Unless required by applicable law or agreed to in writing, software
# distributed under the License is distributed on an "AS IS" BASIS,
# WITHOUT WARRANTIES OR CONDITIONS OF ANY KIND, either express or implied.
# See the License for the specific language governing permissions and
# limitations under the License.
"""Convert SpeechT5 checkpoint."""
import argparse
import torch
from transformers import (
SpeechT5Config,
SpeechT5FeatureExtractor,
SpeechT5ForSpeechToSpeech,
SpeechT5ForSpeechToText,
SpeechT5ForTextToSpeech,
SpeechT5Processor,
SpeechT5Tokenizer,
logging,
)
from transformers.tokenization_utils import AddedToken
logging.set_verbosity_info()
logger = logging.get_logger("transformers.models.speecht5")
MAPPING_SPEECH_ENCODER_PRENET = {
"speech_encoder_prenet.layer_norm": "speecht5.encoder.prenet.feature_projection.layer_norm",
"speech_encoder_prenet.post_extract_proj": "speecht5.encoder.prenet.feature_projection.projection",
"speech_encoder_prenet.pos_conv.0": "speecht5.encoder.prenet.pos_conv_embed.conv",
"speech_encoder_prenet.mask_emb": "speecht5.encoder.prenet.masked_spec_embed",
}
MAPPING_TEXT_ENCODER_PRENET = {
"text_encoder_prenet.encoder_prenet.0": "speecht5.encoder.prenet.embed_tokens",
"text_encoder_prenet.encoder_prenet.1.alpha": "speecht5.encoder.prenet.encode_positions.alpha",
}
MAPPING_SPEECH_DECODER_PRENET = {
"speech_decoder_prenet.decoder_prenet.0.0.prenet.0.0": "speecht5.decoder.prenet.layers.0",
"speech_decoder_prenet.decoder_prenet.0.0.prenet.1.0": "speecht5.decoder.prenet.layers.1",
"speech_decoder_prenet.decoder_prenet.0.1": "speecht5.decoder.prenet.final_layer",
"speech_decoder_prenet.decoder_prenet.1.alpha": "speecht5.decoder.prenet.encode_positions.alpha",
"speech_decoder_prenet.spkembs_layer.0": "speecht5.decoder.prenet.speaker_embeds_layer",
}
MAPPING_SPEECH_DECODER_POSTNET = {
"speech_decoder_postnet.feat_out": "speech_decoder_postnet.feat_out",
"speech_decoder_postnet.prob_out": "speech_decoder_postnet.prob_out",
"speech_decoder_postnet.postnet.postnet.0.0": "speech_decoder_postnet.layers.0.conv",
"speech_decoder_postnet.postnet.postnet.0.1": "speech_decoder_postnet.layers.0.batch_norm",
"speech_decoder_postnet.postnet.postnet.1.0": "speech_decoder_postnet.layers.1.conv",
"speech_decoder_postnet.postnet.postnet.1.1": "speech_decoder_postnet.layers.1.batch_norm",
"speech_decoder_postnet.postnet.postnet.2.0": "speech_decoder_postnet.layers.2.conv",
"speech_decoder_postnet.postnet.postnet.2.1": "speech_decoder_postnet.layers.2.batch_norm",
"speech_decoder_postnet.postnet.postnet.3.0": "speech_decoder_postnet.layers.3.conv",
"speech_decoder_postnet.postnet.postnet.3.1": "speech_decoder_postnet.layers.3.batch_norm",
"speech_decoder_postnet.postnet.postnet.4.0": "speech_decoder_postnet.layers.4.conv",
"speech_decoder_postnet.postnet.postnet.4.1": "speech_decoder_postnet.layers.4.batch_norm",
}
MAPPING_TEXT_DECODER_PRENET = {
"text_decoder_prenet.embed_tokens": "speecht5.decoder.prenet.embed_tokens",
}
MAPPING_TEXT_DECODER_POSTNET = {
"text_decoder_postnet.output_projection": "text_decoder_postnet.lm_head",
}
MAPPING_ENCODER = {
"encoder.layers.*.self_attn.k_proj": "speecht5.encoder.wrapped_encoder.layers.*.attention.k_proj",
"encoder.layers.*.self_attn.v_proj": "speecht5.encoder.wrapped_encoder.layers.*.attention.v_proj",
"encoder.layers.*.self_attn.q_proj": "speecht5.encoder.wrapped_encoder.layers.*.attention.q_proj",
"encoder.layers.*.self_attn.out_proj": "speecht5.encoder.wrapped_encoder.layers.*.attention.out_proj",
"encoder.layers.*.self_attn_layer_norm": "speecht5.encoder.wrapped_encoder.layers.*.layer_norm",
"encoder.layers.*.fc1": "speecht5.encoder.wrapped_encoder.layers.*.feed_forward.intermediate_dense",
"encoder.layers.*.fc2": "speecht5.encoder.wrapped_encoder.layers.*.feed_forward.output_dense",
"encoder.layers.*.final_layer_norm": "speecht5.encoder.wrapped_encoder.layers.*.final_layer_norm",
"encoder.layer_norm": "speecht5.encoder.wrapped_encoder.layer_norm",
"encoder.pos_emb.pe_k": "speecht5.encoder.wrapped_encoder.embed_positions.pe_k",
}
MAPPING_DECODER = {
"decoder.layers.*.self_attn.k_proj": "speecht5.decoder.wrapped_decoder.layers.*.self_attn.k_proj",
"decoder.layers.*.self_attn.v_proj": "speecht5.decoder.wrapped_decoder.layers.*.self_attn.v_proj",
"decoder.layers.*.self_attn.q_proj": "speecht5.decoder.wrapped_decoder.layers.*.self_attn.q_proj",
"decoder.layers.*.self_attn.out_proj": "speecht5.decoder.wrapped_decoder.layers.*.self_attn.out_proj",
"decoder.layers.*.self_attn_layer_norm": "speecht5.decoder.wrapped_decoder.layers.*.self_attn_layer_norm",
"decoder.layers.*.encoder_attn.k_proj": "speecht5.decoder.wrapped_decoder.layers.*.encoder_attn.k_proj",
"decoder.layers.*.encoder_attn.v_proj": "speecht5.decoder.wrapped_decoder.layers.*.encoder_attn.v_proj",
"decoder.layers.*.encoder_attn.q_proj": "speecht5.decoder.wrapped_decoder.layers.*.encoder_attn.q_proj",
"decoder.layers.*.encoder_attn.out_proj": "speecht5.decoder.wrapped_decoder.layers.*.encoder_attn.out_proj",
"decoder.layers.*.encoder_attn_layer_norm": "speecht5.decoder.wrapped_decoder.layers.*.encoder_attn_layer_norm",
"decoder.layers.*.fc1": "speecht5.decoder.wrapped_decoder.layers.*.feed_forward.intermediate_dense",
"decoder.layers.*.fc2": "speecht5.decoder.wrapped_decoder.layers.*.feed_forward.output_dense",
"decoder.layers.*.final_layer_norm": "speecht5.decoder.wrapped_decoder.layers.*.final_layer_norm",
}
MAPPING_S2T = {
**MAPPING_SPEECH_ENCODER_PRENET,
**MAPPING_ENCODER,
**MAPPING_DECODER,
**MAPPING_TEXT_DECODER_PRENET,
**MAPPING_TEXT_DECODER_POSTNET,
}
MAPPING_T2S = {
**MAPPING_TEXT_ENCODER_PRENET,
**MAPPING_ENCODER,
**MAPPING_DECODER,
**MAPPING_SPEECH_DECODER_PRENET,
**MAPPING_SPEECH_DECODER_POSTNET,
}
MAPPING_S2S = {
**MAPPING_SPEECH_ENCODER_PRENET,
**MAPPING_ENCODER,
**MAPPING_DECODER,
**MAPPING_SPEECH_DECODER_PRENET,
**MAPPING_SPEECH_DECODER_POSTNET,
}
TOP_LEVEL_KEYS = []
IGNORE_KEYS = [
"encoder.version",
"encoder.layers.*.norm_k.weight",
"encoder.layers.*.norm_k.bias",
"decoder.version",
"decoder.layers.*.norm_k.weight",
"decoder.layers.*.norm_k.bias",
"decoder.pos_emb.pe_k",
"speech_encoder_prenet.embed_positions._float_tensor",
"text_decoder_prenet.embed_positions._float_tensor",
]
IGNORE_KEYS_S2T = IGNORE_KEYS + [
"encoder.proj",
"text_encoder_prenet.*",
"speech_decoder_prenet.*",
"speech_decoder_postnet.*",
]
IGNORE_KEYS_T2S = IGNORE_KEYS + [
"encoder.proj",
"speech_encoder_prenet.*",
"text_decoder_prenet.*",
"text_decoder_postnet.*",
]
IGNORE_KEYS_S2S = IGNORE_KEYS + [
"encoder.proj",
"text_encoder_prenet.*",
"text_decoder_prenet.*",
"text_decoder_postnet.*",
]
def set_recursively(hf_pointer, key, value, full_name, weight_type):
for attribute in key.split("."):
hf_pointer = getattr(hf_pointer, attribute)
if weight_type is not None:
hf_shape = getattr(hf_pointer, weight_type).shape
else:
hf_shape = hf_pointer.shape
if hf_shape != value.shape:
raise ValueError(
f"Shape of hf {key + '.' + weight_type if weight_type is not None else ''} is {hf_shape}, but should be"
f" {value.shape} for {full_name}"
)
if weight_type == "weight":
hf_pointer.weight.data = value
elif weight_type == "weight_g":
hf_pointer.weight_g.data = value
elif weight_type == "weight_v":
hf_pointer.weight_v.data = value
elif weight_type == "bias":
hf_pointer.bias.data = value
elif weight_type == "running_mean":
hf_pointer.running_mean.data = value
elif weight_type == "running_var":
hf_pointer.running_var.data = value
elif weight_type == "num_batches_tracked":
hf_pointer.num_batches_tracked.data = value
else:
hf_pointer.data = value
logger.info(f"{key + ('.' + weight_type if weight_type is not None else '')} was initialized from {full_name}.")
def should_ignore(name, ignore_keys):
for key in ignore_keys:
if key.endswith(".*"):
if name.startswith(key[:-1]):
return True
elif ".*." in key:
prefix, suffix = key.split(".*.")
if prefix in name and suffix in name:
return True
elif key in name:
return True
return False
def recursively_load_weights(fairseq_dict, hf_model, task):
unused_weights = []
if task == "s2t":
feature_encoder = hf_model.speecht5.encoder.prenet.feature_encoder
MAPPING = MAPPING_S2T
IGNORE_KEYS = IGNORE_KEYS_S2T
elif task == "t2s":
feature_encoder = None
MAPPING = MAPPING_T2S
IGNORE_KEYS = IGNORE_KEYS_T2S
elif task == "s2s":
feature_encoder = hf_model.speecht5.encoder.prenet.feature_encoder
MAPPING = MAPPING_S2S
IGNORE_KEYS = IGNORE_KEYS_S2S
else:
raise ValueError(f"Unsupported task: {task}")
for name, value in fairseq_dict.items():
if should_ignore(name, IGNORE_KEYS):
logger.info(f"{name} was ignored")
continue
is_used = False
if "conv_layers" in name:
load_conv_layer(
name,
value,
feature_encoder,
unused_weights,
hf_model.config.feat_extract_norm == "group",
)
is_used = True
else:
for key, mapped_key in MAPPING.items():
# mapped_key = "speecht5." + mapped_key if mapped_key not in TOP_LEVEL_KEYS else mapped_key
if "*" in key:
prefix, suffix = key.split(".*.")
if prefix in name and suffix in name:
key = suffix
# if key in name or key.split("w2v_model.")[-1] == name.split(".")[0]:
if key in name:
is_used = True
if "*" in mapped_key:
layer_index = name.split(key)[0].split(".")[-2]
mapped_key = mapped_key.replace("*", layer_index)
if "weight_g" in name:
weight_type = "weight_g"
elif "weight_v" in name:
weight_type = "weight_v"
elif "bias" in name:
weight_type = "bias"
elif "weight" in name:
weight_type = "weight"
elif "running_mean" in name:
weight_type = "running_mean"
elif "running_var" in name:
weight_type = "running_var"
elif "num_batches_tracked" in name:
weight_type = "num_batches_tracked"
else:
weight_type = None
set_recursively(hf_model, mapped_key, value, name, weight_type)
continue
if not is_used:
unused_weights.append(name)
logger.warning(f"Unused weights: {unused_weights}")
def load_conv_layer(full_name, value, feature_extractor, unused_weights, use_group_norm):
name = full_name.split("conv_layers.")[-1]
items = name.split(".")
layer_id = int(items[0])
type_id = int(items[1])
if type_id == 0:
if "bias" in name:
if value.shape != feature_extractor.conv_layers[layer_id].conv.bias.data.shape:
raise ValueError(
f"{full_name} has size {value.shape}, but"
f" {feature_extractor.conv_layers[layer_id].conv.bias.data.shape} was found."
)
feature_extractor.conv_layers[layer_id].conv.bias.data = value
logger.info(f"Feat extract conv layer {layer_id} was initialized from {full_name}.")
elif "weight" in name:
if value.shape != feature_extractor.conv_layers[layer_id].conv.weight.data.shape:
raise ValueError(
f"{full_name} has size {value.shape}, but"
f" {feature_extractor.conv_layers[layer_id].conv.weight.data.shape} was found."
)
feature_extractor.conv_layers[layer_id].conv.weight.data = value
logger.info(f"Feat extract conv layer {layer_id} was initialized from {full_name}.")
elif (type_id == 2 and not use_group_norm) or (type_id == 2 and layer_id == 0 and use_group_norm):
if "bias" in name:
if value.shape != feature_extractor.conv_layers[layer_id].layer_norm.bias.data.shape:
raise ValueError(
f"{full_name} has size {value.shape}, but"
f" {feature_extractor.conv_layers[layer_id].layer_norm.bias.data.shape} was found."
)
feature_extractor.conv_layers[layer_id].layer_norm.bias.data = value
logger.info(f"Feat extract layer norm weight of layer {layer_id} was initialized from {full_name}.")
elif "weight" in name:
if value.shape != feature_extractor.conv_layers[layer_id].layer_norm.weight.data.shape:
raise ValueError(
f"{full_name} has size {value.shape}, but"
f" {feature_extractor.conv_layers[layer_id].layer_norm.weight.data.shape} was found."
)
feature_extractor.conv_layers[layer_id].layer_norm.weight.data = value
logger.info(f"Feat extract layer norm weight of layer {layer_id} was initialized from {full_name}.")
else:
unused_weights.append(full_name)
@torch.no_grad()
def convert_speecht5_checkpoint(
task,
checkpoint_path,
pytorch_dump_folder_path,
config_path=None,
vocab_path=None,
repo_id=None,
):
"""
Copy/paste/tweak model's weights to transformers design.
"""
if config_path is not None:
config = SpeechT5Config.from_pretrained(config_path)
else:
config = SpeechT5Config()
if task == "s2t":
config.max_length = config.max_text_positions
model = SpeechT5ForSpeechToText(config)
elif task == "t2s":
config.max_speech_positions = 1876
config.max_text_positions = 600
config.max_length = config.max_speech_positions
model = SpeechT5ForTextToSpeech(config)
elif task == "s2s":
config.max_speech_positions = 1876
config.max_length = config.max_speech_positions
model = SpeechT5ForSpeechToSpeech(config)
else:
raise ValueError(f"Unknown task name: {task}")
if vocab_path:
tokenizer = SpeechT5Tokenizer(vocab_path, model_max_length=config.max_text_positions)
# Mask token behaves like a normal word, i.e. include the space before it
mask_token = AddedToken("<mask>", lstrip=True, rstrip=False)
tokenizer.mask_token = mask_token
tokenizer.add_special_tokens({"mask_token": mask_token})
tokenizer.add_tokens(["<ctc_blank>"])
feature_extractor = SpeechT5FeatureExtractor()
processor = SpeechT5Processor(tokenizer=tokenizer, feature_extractor=feature_extractor)
processor.save_pretrained(pytorch_dump_folder_path)
fairseq_checkpoint = torch.load(checkpoint_path)
recursively_load_weights(fairseq_checkpoint["model"], model, task)
model.save_pretrained(pytorch_dump_folder_path)
if repo_id:
print("Pushing to the hub...")
processor.push_to_hub(repo_id)
model.push_to_hub(repo_id)
if __name__ == "__main__":
parser = argparse.ArgumentParser()
parser.add_argument(
"--task",
default="s2t",
type=str,
help="Type of the SpeechT5 model you'd like to convert. Should be one of 's2t', 't2s', 's2s'.",
)
parser.add_argument("--checkpoint_path", required=True, default=None, type=str, help="Path to fairseq checkpoint")
parser.add_argument("--vocab_path", default=None, type=str, help="Path to SentencePiece model")
parser.add_argument("--config_path", default=None, type=str, help="Path to hf config.json of model to convert")
parser.add_argument(
"--pytorch_dump_folder_path", required=True, default=None, type=str, help="Path to the output PyTorch model."
)
parser.add_argument(
"--push_to_hub", default=None, type=str, help="Where to upload the converted model on the 🤗 hub."
)
args = parser.parse_args()
convert_speecht5_checkpoint(
args.task,
args.checkpoint_path,
args.pytorch_dump_folder_path,
args.config_path,
args.vocab_path,
args.push_to_hub,
)
| transformers/src/transformers/models/speecht5/convert_speecht5_original_pytorch_checkpoint_to_pytorch.py/0 | {
"file_path": "transformers/src/transformers/models/speecht5/convert_speecht5_original_pytorch_checkpoint_to_pytorch.py",
"repo_id": "transformers",
"token_count": 7959
} | 324 |
# coding=utf-8
# Copyright 2022 The HuggingFace Inc. team. All rights reserved.
#
# Licensed under the Apache License, Version 2.0 (the "License");
# you may not use this file except in compliance with the License.
# You may obtain a copy of the License at
#
# http://www.apache.org/licenses/LICENSE-2.0
#
# Unless required by applicable law or agreed to in writing, software
# distributed under the License is distributed on an "AS IS" BASIS,
# WITHOUT WARRANTIES OR CONDITIONS OF ANY KIND, either express or implied.
# See the License for the specific language governing permissions and
# limitations under the License.
""" Swinv2 Transformer model configuration"""
from ...configuration_utils import PretrainedConfig
from ...utils import logging
from ...utils.backbone_utils import BackboneConfigMixin, get_aligned_output_features_output_indices
logger = logging.get_logger(__name__)
SWINV2_PRETRAINED_CONFIG_ARCHIVE_MAP = {
"microsoft/swinv2-tiny-patch4-window8-256": (
"https://huggingface.co/microsoft/swinv2-tiny-patch4-window8-256/resolve/main/config.json"
),
}
class Swinv2Config(BackboneConfigMixin, PretrainedConfig):
r"""
This is the configuration class to store the configuration of a [`Swinv2Model`]. It is used to instantiate a Swin
Transformer v2 model according to the specified arguments, defining the model architecture. Instantiating a
configuration with the defaults will yield a similar configuration to that of the Swin Transformer v2
[microsoft/swinv2-tiny-patch4-window8-256](https://huggingface.co/microsoft/swinv2-tiny-patch4-window8-256)
architecture.
Configuration objects inherit from [`PretrainedConfig`] and can be used to control the model outputs. Read the
documentation from [`PretrainedConfig`] for more information.
Args:
image_size (`int`, *optional*, defaults to 224):
The size (resolution) of each image.
patch_size (`int`, *optional*, defaults to 4):
The size (resolution) of each patch.
num_channels (`int`, *optional*, defaults to 3):
The number of input channels.
embed_dim (`int`, *optional*, defaults to 96):
Dimensionality of patch embedding.
depths (`list(int)`, *optional*, defaults to `[2, 2, 6, 2]`):
Depth of each layer in the Transformer encoder.
num_heads (`list(int)`, *optional*, defaults to `[3, 6, 12, 24]`):
Number of attention heads in each layer of the Transformer encoder.
window_size (`int`, *optional*, defaults to 7):
Size of windows.
pretrained_window_sizes (`list(int)`, *optional*, defaults to `[0, 0, 0, 0]`):
Size of windows during pretraining.
mlp_ratio (`float`, *optional*, defaults to 4.0):
Ratio of MLP hidden dimensionality to embedding dimensionality.
qkv_bias (`bool`, *optional*, defaults to `True`):
Whether or not a learnable bias should be added to the queries, keys and values.
hidden_dropout_prob (`float`, *optional*, defaults to 0.0):
The dropout probability for all fully connected layers in the embeddings and encoder.
attention_probs_dropout_prob (`float`, *optional*, defaults to 0.0):
The dropout ratio for the attention probabilities.
drop_path_rate (`float`, *optional*, defaults to 0.1):
Stochastic depth rate.
hidden_act (`str` or `function`, *optional*, defaults to `"gelu"`):
The non-linear activation function (function or string) in the encoder. If string, `"gelu"`, `"relu"`,
`"selu"` and `"gelu_new"` are supported.
use_absolute_embeddings (`bool`, *optional*, defaults to `False`):
Whether or not to add absolute position embeddings to the patch embeddings.
initializer_range (`float`, *optional*, defaults to 0.02):
The standard deviation of the truncated_normal_initializer for initializing all weight matrices.
layer_norm_eps (`float`, *optional*, defaults to 1e-05):
The epsilon used by the layer normalization layers.
encoder_stride (`int`, *optional*, defaults to 32):
Factor to increase the spatial resolution by in the decoder head for masked image modeling.
out_features (`List[str]`, *optional*):
If used as backbone, list of features to output. Can be any of `"stem"`, `"stage1"`, `"stage2"`, etc.
(depending on how many stages the model has). If unset and `out_indices` is set, will default to the
corresponding stages. If unset and `out_indices` is unset, will default to the last stage.
out_indices (`List[int]`, *optional*):
If used as backbone, list of indices of features to output. Can be any of 0, 1, 2, etc. (depending on how
many stages the model has). If unset and `out_features` is set, will default to the corresponding stages.
If unset and `out_features` is unset, will default to the last stage.
Example:
```python
>>> from transformers import Swinv2Config, Swinv2Model
>>> # Initializing a Swinv2 microsoft/swinv2-tiny-patch4-window8-256 style configuration
>>> configuration = Swinv2Config()
>>> # Initializing a model (with random weights) from the microsoft/swinv2-tiny-patch4-window8-256 style configuration
>>> model = Swinv2Model(configuration)
>>> # Accessing the model configuration
>>> configuration = model.config
```"""
model_type = "swinv2"
attribute_map = {
"num_attention_heads": "num_heads",
"num_hidden_layers": "num_layers",
}
def __init__(
self,
image_size=224,
patch_size=4,
num_channels=3,
embed_dim=96,
depths=[2, 2, 6, 2],
num_heads=[3, 6, 12, 24],
window_size=7,
pretrained_window_sizes=[0, 0, 0, 0],
mlp_ratio=4.0,
qkv_bias=True,
hidden_dropout_prob=0.0,
attention_probs_dropout_prob=0.0,
drop_path_rate=0.1,
hidden_act="gelu",
use_absolute_embeddings=False,
initializer_range=0.02,
layer_norm_eps=1e-5,
encoder_stride=32,
out_features=None,
out_indices=None,
**kwargs,
):
super().__init__(**kwargs)
self.image_size = image_size
self.patch_size = patch_size
self.num_channels = num_channels
self.embed_dim = embed_dim
self.depths = depths
self.num_layers = len(depths)
self.num_heads = num_heads
self.window_size = window_size
self.pretrained_window_sizes = pretrained_window_sizes
self.mlp_ratio = mlp_ratio
self.qkv_bias = qkv_bias
self.hidden_dropout_prob = hidden_dropout_prob
self.attention_probs_dropout_prob = attention_probs_dropout_prob
self.drop_path_rate = drop_path_rate
self.hidden_act = hidden_act
self.use_absolute_embeddings = use_absolute_embeddings
self.layer_norm_eps = layer_norm_eps
self.initializer_range = initializer_range
self.encoder_stride = encoder_stride
self.stage_names = ["stem"] + [f"stage{idx}" for idx in range(1, len(depths) + 1)]
self._out_features, self._out_indices = get_aligned_output_features_output_indices(
out_features=out_features, out_indices=out_indices, stage_names=self.stage_names
)
# we set the hidden_size attribute in order to make Swinv2 work with VisionEncoderDecoderModel
# this indicates the channel dimension after the last stage of the model
self.hidden_size = int(embed_dim * 2 ** (len(depths) - 1))
| transformers/src/transformers/models/swinv2/configuration_swinv2.py/0 | {
"file_path": "transformers/src/transformers/models/swinv2/configuration_swinv2.py",
"repo_id": "transformers",
"token_count": 2994
} | 325 |
# coding=utf-8
# Copyright 2020 T5 Authors and The HuggingFace Inc. team.
# Copyright (c) 2018, NVIDIA CORPORATION. All rights reserved.
#
# Licensed under the Apache License, Version 2.0 (the "License");
# you may not use this file except in compliance with the License.
# You may obtain a copy of the License at
#
# http://www.apache.org/licenses/LICENSE-2.0
#
# Unless required by applicable law or agreed to in writing, software
# distributed under the License is distributed on an "AS IS" BASIS,
# WITHOUT WARRANTIES OR CONDITIONS OF ANY KIND, either express or implied.
# See the License for the specific language governing permissions and
# limitations under the License.
""" TF 2.0 T5 model."""
from __future__ import annotations
import copy
import itertools
import math
import warnings
from typing import Optional, Tuple, Union
import numpy as np
import tensorflow as tf
from tensorflow.compiler.tf2xla.python.xla import dynamic_slice
from ...activations_tf import get_tf_activation
from ...modeling_tf_outputs import (
TFBaseModelOutput,
TFBaseModelOutputWithPastAndCrossAttentions,
TFSeq2SeqLMOutput,
TFSeq2SeqModelOutput,
)
from ...modeling_tf_utils import (
TFCausalLanguageModelingLoss,
TFModelInputType,
TFPreTrainedModel,
get_initializer,
keras,
keras_serializable,
unpack_inputs,
)
from ...tf_utils import check_embeddings_within_bounds, shape_list, stable_softmax
from ...utils import (
add_start_docstrings,
add_start_docstrings_to_model_forward,
logging,
replace_return_docstrings,
)
from .configuration_t5 import T5Config
logger = logging.get_logger(__name__)
_CONFIG_FOR_DOC = "T5Config"
TF_T5_PRETRAINED_MODEL_ARCHIVE_LIST = [
"t5-small",
"t5-base",
"t5-large",
"t5-3b",
"t5-11b",
# See all T5 models at https://huggingface.co/models?filter=t5
]
####################################################
# TF 2.0 Models are constructed using Keras imperative API by sub-classing
# - keras.layers.Layer for the layers and
# - TFPreTrainedModel for the models (it-self a sub-class of keras.Model)
####################################################
class TFT5LayerNorm(keras.layers.Layer):
def __init__(self, hidden_size, epsilon=1e-6, **kwargs):
"""
Construct a layernorm module in the T5 style No bias and no subtraction of mean.
"""
super().__init__(**kwargs)
self.variance_epsilon = epsilon
self.hidden_size = hidden_size
def build(self, input_shape):
"""Build shared word embedding layer"""
self.weight = self.add_weight("weight", shape=(self.hidden_size,), initializer="ones")
super().build(input_shape)
def call(self, hidden_states):
variance = tf.math.reduce_mean(tf.math.square(hidden_states), axis=-1, keepdims=True)
hidden_states = hidden_states * tf.math.rsqrt(variance + self.variance_epsilon)
return self.weight * hidden_states
class TFT5DenseActDense(keras.layers.Layer):
def __init__(self, config, **kwargs):
super().__init__(**kwargs)
wi_initializer = keras.initializers.RandomNormal(
mean=0, stddev=config.initializer_factor * (config.d_model**-0.5)
)
wo_initializer = keras.initializers.RandomNormal(
mean=0, stddev=config.initializer_factor * (config.d_ff**-0.5)
)
self.wi = keras.layers.Dense(
config.d_ff, use_bias=False, name="wi", kernel_initializer=wi_initializer
) # Update init weights as in flax
self.wo = keras.layers.Dense(
config.d_model, use_bias=False, name="wo", kernel_initializer=wo_initializer
) # Update init weights as in flax
self.dropout = keras.layers.Dropout(config.dropout_rate)
self.act = get_tf_activation(config.dense_act_fn)
self.config = config
def call(self, hidden_states, training=False):
hidden_states = self.wi(hidden_states)
hidden_states = self.act(hidden_states)
hidden_states = self.dropout(hidden_states, training=training)
hidden_states = self.wo(hidden_states)
return hidden_states
def build(self, input_shape=None):
if self.built:
return
self.built = True
if getattr(self, "wi", None) is not None:
with tf.name_scope(self.wi.name):
self.wi.build([None, None, self.config.d_model])
if getattr(self, "wo", None) is not None:
with tf.name_scope(self.wo.name):
self.wo.build([None, None, self.config.d_ff])
class TFT5DenseGatedActDense(keras.layers.Layer):
def __init__(self, config, **kwargs):
super().__init__(**kwargs)
wi_initializer = keras.initializers.RandomNormal(
mean=0, stddev=config.initializer_factor * (config.d_model**-0.5)
)
wo_initializer = keras.initializers.RandomNormal(
mean=0, stddev=config.initializer_factor * (config.d_ff**-0.5)
)
self.wi_0 = keras.layers.Dense(
config.d_ff, use_bias=False, name="wi_0", kernel_initializer=wi_initializer
) # Update init weights as in flax
self.wi_1 = keras.layers.Dense(
config.d_ff, use_bias=False, name="wi_1", kernel_initializer=wi_initializer
) # Update init weights as in flax
self.wo = keras.layers.Dense(
config.d_model, use_bias=False, name="wo", kernel_initializer=wo_initializer
) # Update init weights as in flax
self.dropout = keras.layers.Dropout(config.dropout_rate)
self.act = get_tf_activation(config.dense_act_fn)
self.config = config
def call(self, hidden_states, training=False):
hidden_gelu = self.act(self.wi_0(hidden_states))
hidden_linear = self.wi_1(hidden_states)
hidden_states = hidden_gelu * hidden_linear
hidden_states = self.dropout(hidden_states, training=training)
hidden_states = self.wo(hidden_states)
return hidden_states
def build(self, input_shape=None):
if self.built:
return
self.built = True
if getattr(self, "wi_0", None) is not None:
with tf.name_scope(self.wi_0.name):
self.wi_0.build([None, None, self.config.d_model])
if getattr(self, "wi_1", None) is not None:
with tf.name_scope(self.wi_1.name):
self.wi_1.build([None, None, self.config.d_model])
if getattr(self, "wo", None) is not None:
with tf.name_scope(self.wo.name):
self.wo.build([None, None, self.config.d_ff])
class TFT5LayerFF(keras.layers.Layer):
def __init__(self, config, **kwargs):
super().__init__(**kwargs)
if config.is_gated_act:
self.DenseReluDense = TFT5DenseGatedActDense(config, name="DenseReluDense")
else:
self.DenseReluDense = TFT5DenseActDense(config, name="DenseReluDense")
self.layer_norm = TFT5LayerNorm(config.d_model, epsilon=config.layer_norm_epsilon, name="layer_norm")
self.dropout = keras.layers.Dropout(config.dropout_rate)
def call(self, hidden_states, training=False):
normed_hidden_states = self.layer_norm(hidden_states)
dense_output = self.DenseReluDense(normed_hidden_states, training=training)
hidden_states = hidden_states + self.dropout(dense_output, training=training)
return hidden_states
def build(self, input_shape=None):
if self.built:
return
self.built = True
if getattr(self, "layer_norm", None) is not None:
with tf.name_scope(self.layer_norm.name):
self.layer_norm.build(None)
if getattr(self, "DenseReluDense", None) is not None:
with tf.name_scope(self.DenseReluDense.name):
self.DenseReluDense.build(None)
class TFT5Attention(keras.layers.Layer):
NEW_ID = itertools.count()
def __init__(self, config, has_relative_attention_bias=False, **kwargs):
super().__init__(**kwargs)
self.layer_id = next(TFT5Attention.NEW_ID)
self.is_decoder = config.is_decoder
self.use_cache = config.use_cache
self.has_relative_attention_bias = has_relative_attention_bias
self.output_attentions = config.output_attentions
self.relative_attention_num_buckets = config.relative_attention_num_buckets
self.relative_attention_max_distance = config.relative_attention_max_distance
self.d_model = config.d_model
self.key_value_proj_dim = config.d_kv
self.n_heads = config.num_heads
self.inner_dim = self.n_heads * self.key_value_proj_dim
# Mesh TensorFlow initialization to avoid scaling before softmax
q_initializer = keras.initializers.RandomNormal(
mean=0, stddev=config.initializer_factor * ((self.inner_dim * self.key_value_proj_dim) ** -0.5)
)
k_initializer = keras.initializers.RandomNormal(
mean=0, stddev=config.initializer_factor * (self.inner_dim**-0.5)
)
v_initializer = keras.initializers.RandomNormal(
mean=0, stddev=config.initializer_factor * (self.inner_dim**-0.5)
)
o_initializer = keras.initializers.RandomNormal(
mean=0, stddev=config.initializer_factor * (self.inner_dim**-0.5)
)
self.relative_attention_bias_initializer = keras.initializers.RandomNormal(
mean=0, stddev=config.initializer_factor * (self.inner_dim**-0.5)
)
self.q = keras.layers.Dense(
self.inner_dim, use_bias=False, name="q", kernel_initializer=q_initializer
) # Update init weights as in flax
self.k = keras.layers.Dense(
self.inner_dim, use_bias=False, name="k", kernel_initializer=k_initializer
) # Update init weights as in flax
self.v = keras.layers.Dense(
self.inner_dim, use_bias=False, name="v", kernel_initializer=v_initializer
) # Update init weights as in flax
self.o = keras.layers.Dense(
self.d_model, use_bias=False, name="o", kernel_initializer=o_initializer
) # Update init weights as in flax
self.dropout = keras.layers.Dropout(config.dropout_rate)
self.pruned_heads = set()
def build(self, input_shape=None):
if self.built:
return
self.built = True
if self.has_relative_attention_bias:
with tf.name_scope("relative_attention_bias"):
self.relative_attention_bias = self.add_weight(
name="embeddings",
shape=[self.relative_attention_num_buckets, self.n_heads],
initializer=self.relative_attention_bias_initializer, # Add initializer
)
if getattr(self, "q", None) is not None:
with tf.name_scope(self.q.name):
self.q.build([None, None, self.d_model])
if getattr(self, "k", None) is not None:
with tf.name_scope(self.k.name):
self.k.build([None, None, self.d_model])
if getattr(self, "v", None) is not None:
with tf.name_scope(self.v.name):
self.v.build([None, None, self.d_model])
if getattr(self, "o", None) is not None:
with tf.name_scope(self.o.name):
self.o.build([None, None, self.inner_dim])
def prune_heads(self, heads):
raise NotImplementedError
@staticmethod
def _relative_position_bucket(relative_position, bidirectional=True, num_buckets=32, max_distance=128):
"""
Adapted from Mesh Tensorflow:
https://github.com/tensorflow/mesh/blob/0cb87fe07da627bf0b7e60475d59f95ed6b5be3d/mesh_tensorflow/transformer/transformer_layers.py#L593
Translate relative position to a bucket number for relative attention. The relative position is defined as
memory_position - query_position, i.e. the distance in tokens from the attending position to the attended-to
position. If bidirectional=False, then positive relative positions are invalid. We use smaller buckets for
small absolute relative_position and larger buckets for larger absolute relative_positions. All relative
positions >=max_distance map to the same bucket. All relative positions <=-max_distance map to the same bucket.
This should allow for more graceful generalization to longer sequences than the model has been trained on
Args:
relative_position: an int32 Tensor
bidirectional: a boolean - whether the attention is bidirectional
num_buckets: an integer
max_distance: an integer
Returns:
a Tensor with the same shape as relative_position, containing int32 values in the range [0, num_buckets)
"""
relative_buckets = 0
# n = -relative_position
if bidirectional:
num_buckets //= 2
relative_buckets += (
tf.cast(tf.math.greater(relative_position, 0), dtype=relative_position.dtype) * num_buckets
)
relative_position = tf.math.abs(relative_position)
else:
relative_position = -tf.math.minimum(relative_position, 0)
# now n is in the range [0, inf)
max_exact = num_buckets // 2
is_small = tf.math.less(relative_position, max_exact)
relative_position_if_large = max_exact + tf.cast(
tf.math.log(tf.cast(relative_position, tf.float32) / tf.cast(max_exact, tf.float32))
/ math.log(max_distance / max_exact)
* (num_buckets - max_exact),
dtype=relative_position.dtype,
)
relative_position_if_large = tf.math.minimum(relative_position_if_large, num_buckets - 1)
relative_buckets += tf.where(is_small, relative_position, relative_position_if_large)
return relative_buckets
def compute_bias(self, query_length, key_length):
"""Compute binned relative position bias"""
context_position = tf.range(query_length)[:, None]
memory_position = tf.range(key_length)[None, :]
relative_position = memory_position - context_position # shape (query_length, key_length)
relative_position_bucket = self._relative_position_bucket(
relative_position,
bidirectional=(not self.is_decoder),
num_buckets=self.relative_attention_num_buckets,
max_distance=self.relative_attention_max_distance,
)
values = tf.gather(
self.relative_attention_bias, relative_position_bucket
) # shape (query_length, key_length, num_heads)
values = tf.expand_dims(
tf.transpose(values, [2, 0, 1]), axis=0
) # shape (1, num_heads, query_length, key_length)
return values
def call(
self,
hidden_states,
mask=None,
key_value_states=None,
position_bias=None,
past_key_value=None,
layer_head_mask=None,
query_length=None,
use_cache=False,
training=False,
output_attentions=False,
):
"""
Self-attention (if key_value_states is None) or attention over source sentence (provided by key_value_states).
"""
# Input is (batch_size, query_length, dim)
# Mask is (batch_size, key_length) (non-causal) or (batch_size, key_length, key_length)
# past_key_value[0] is (batch_size, n_heads, q_len - 1, dim_per_head)
batch_size, seq_length = shape_list(hidden_states)[:2]
real_seq_length = seq_length
if past_key_value is not None:
assert (
len(past_key_value) == 2
), f"past_key_value should have 2 past states: keys and values. Got {len(past_key_value)} past states"
real_seq_length += shape_list(past_key_value[0])[2] if query_length is None else query_length
key_length = real_seq_length if key_value_states is None else shape_list(key_value_states)[1]
def shape(hidden_states):
"""projection"""
return tf.transpose(
tf.reshape(hidden_states, (batch_size, -1, self.n_heads, self.key_value_proj_dim)), perm=(0, 2, 1, 3)
)
def unshape(hidden_states):
"""compute context"""
return tf.reshape(tf.transpose(hidden_states, perm=(0, 2, 1, 3)), (batch_size, -1, self.inner_dim))
def project(hidden_states, proj_layer, key_value_states, past_key_value):
"""projects hidden states correctly to key/query states"""
if key_value_states is None:
# self-attn
# (batch_size, n_heads, seq_length, dim_per_head)
hidden_states = shape(proj_layer(hidden_states))
elif past_key_value is None:
# cross-attn
# (batch_size, n_heads, seq_length, dim_per_head)
hidden_states = shape(proj_layer(key_value_states))
if past_key_value is not None:
if key_value_states is None:
# self-attn
# (batch_size, n_heads, key_length, dim_per_head)
hidden_states = tf.concat([past_key_value, hidden_states], axis=2)
else:
# cross-attn
hidden_states = past_key_value
return hidden_states
# get query
query_states = shape(self.q(hidden_states)) # (batch_size, n_heads, query_length, dim_per_head)
# get key/value
key_states = project(
hidden_states, self.k, key_value_states, past_key_value[0] if past_key_value is not None else None
)
value_states = project(
hidden_states, self.v, key_value_states, past_key_value[1] if past_key_value is not None else None
)
# to cope with keras serialization
if self.is_decoder and use_cache:
present_key_value_state = (key_states, value_states)
else:
present_key_value_state = None
scores = tf.einsum(
"bnqd,bnkd->bnqk", query_states, key_states
) # (batch_size, n_heads, query_length, key_length)
if position_bias is None:
if not self.has_relative_attention_bias:
position_bias = tf.zeros((1, self.n_heads, real_seq_length, key_length))
else:
position_bias = self.compute_bias(real_seq_length, key_length)
# if key and values are already calculated we want only the last query position bias
if past_key_value is not None:
if not self.has_relative_attention_bias:
position_bias = position_bias[:, :, -seq_length:, :]
else:
# we might have a padded past structure, in which case we want to fetch the position bias slice
# right after the most recently filled past index
most_recently_filled_past_index = tf.reduce_max(tf.where(past_key_value[0][0, 0, :, 0] != 0.0))
position_bias = dynamic_slice(
position_bias,
(0, 0, most_recently_filled_past_index + 1, 0),
(1, self.n_heads, seq_length, real_seq_length),
)
if mask is not None:
position_bias = tf.cast(position_bias, dtype=mask.dtype)
position_bias = position_bias + mask # (batch_size, n_heads, query_length, key_length)
scores += position_bias
weights = stable_softmax(scores, axis=-1) # (batch_size, n_heads, query_length, key_length)
weights = self.dropout(weights, training=training) # (batch_size, n_heads, query_length, key_length)
# Mask heads if we want to
if layer_head_mask is not None:
tf.debugging.assert_equal(
shape_list(layer_head_mask),
[self.n_heads],
message=(
f"Head mask for a single layer should be of size {(self.n_heads)}, but is"
f" {shape_list(layer_head_mask)}"
),
)
weights = tf.reshape(layer_head_mask, (1, -1, 1, 1)) * weights
attn_output = tf.matmul(weights, value_states) # (batch_size, n_heads, query_length, dim_per_head)
attn_output = self.o(unshape(attn_output))
outputs = (attn_output,) + (present_key_value_state,) + (position_bias,)
if output_attentions:
outputs = outputs + (weights,)
return outputs
class TFT5LayerSelfAttention(keras.layers.Layer):
def __init__(self, config, has_relative_attention_bias=False, **kwargs):
super().__init__(**kwargs)
self.SelfAttention = TFT5Attention(
config,
has_relative_attention_bias=has_relative_attention_bias,
name="SelfAttention",
)
self.layer_norm = TFT5LayerNorm(config.d_model, epsilon=config.layer_norm_epsilon, name="layer_norm")
self.dropout = keras.layers.Dropout(config.dropout_rate)
def call(
self,
hidden_states,
attention_mask=None,
position_bias=None,
layer_head_mask=None,
past_key_value=None,
use_cache=False,
output_attentions=False,
training=False,
):
normed_hidden_states = self.layer_norm(hidden_states)
attention_output = self.SelfAttention(
normed_hidden_states,
mask=attention_mask,
position_bias=position_bias,
layer_head_mask=layer_head_mask,
past_key_value=past_key_value,
use_cache=use_cache,
output_attentions=output_attentions,
training=training,
)
hidden_states = hidden_states + self.dropout(attention_output[0], training=training)
outputs = (hidden_states,) + attention_output[1:] # add attentions if we output them
return outputs
def build(self, input_shape=None):
if self.built:
return
self.built = True
if getattr(self, "SelfAttention", None) is not None:
with tf.name_scope(self.SelfAttention.name):
self.SelfAttention.build(None)
if getattr(self, "layer_norm", None) is not None:
with tf.name_scope(self.layer_norm.name):
self.layer_norm.build(None)
class TFT5LayerCrossAttention(keras.layers.Layer):
def __init__(self, config, **kwargs):
super().__init__(**kwargs)
self.EncDecAttention = TFT5Attention(
config,
has_relative_attention_bias=False,
name="EncDecAttention",
)
self.layer_norm = TFT5LayerNorm(config.d_model, epsilon=config.layer_norm_epsilon, name="layer_norm")
self.dropout = keras.layers.Dropout(config.dropout_rate)
def call(
self,
hidden_states,
key_value_states,
attention_mask=None,
position_bias=None,
layer_head_mask=None,
past_key_value=None,
query_length=None,
use_cache=False,
output_attentions=False,
training=False,
):
normed_hidden_states = self.layer_norm(hidden_states)
attention_output = self.EncDecAttention(
normed_hidden_states,
mask=attention_mask,
key_value_states=key_value_states,
position_bias=position_bias,
layer_head_mask=layer_head_mask,
past_key_value=past_key_value,
query_length=query_length,
use_cache=use_cache,
output_attentions=output_attentions,
training=training,
)
hidden_states = hidden_states + self.dropout(attention_output[0], training=training)
outputs = (hidden_states,) + attention_output[1:] # add attentions if we output them
return outputs
def build(self, input_shape=None):
if self.built:
return
self.built = True
if getattr(self, "EncDecAttention", None) is not None:
with tf.name_scope(self.EncDecAttention.name):
self.EncDecAttention.build(None)
if getattr(self, "layer_norm", None) is not None:
with tf.name_scope(self.layer_norm.name):
self.layer_norm.build(None)
class TFT5Block(keras.layers.Layer):
def __init__(self, config, has_relative_attention_bias=False, **kwargs):
super().__init__(**kwargs)
self.is_decoder = config.is_decoder
self.layer = []
self.layer.append(
TFT5LayerSelfAttention(
config,
has_relative_attention_bias=has_relative_attention_bias,
name="layer_._0",
)
)
if self.is_decoder:
self.layer.append(
TFT5LayerCrossAttention(
config,
name="layer_._1",
)
)
self.layer.append(TFT5LayerFF(config, name=f"layer_._{len(self.layer)}"))
def call(
self,
hidden_states,
attention_mask=None,
position_bias=None,
encoder_hidden_states=None,
encoder_attention_mask=None,
encoder_decoder_position_bias=None,
layer_head_mask=None,
encoder_layer_head_mask=None,
past_key_value=None,
use_cache=False,
output_attentions=False,
training=False,
):
if past_key_value is not None:
assert self.is_decoder, "Only decoder can use `past_key_values`"
expected_num_past_key_values = 2 if encoder_hidden_states is None else 4
if len(past_key_value) != expected_num_past_key_values:
raise ValueError(
f"There should be {expected_num_past_key_values} past states. "
f"{'2 (past / key) for cross attention' if expected_num_past_key_values == 4 else ''}. "
f"Got {len(past_key_value)} past key / value states"
)
self_attn_past_key_value = past_key_value[:2]
cross_attn_past_key_value = past_key_value[2:]
else:
self_attn_past_key_value, cross_attn_past_key_value = None, None
self_attention_outputs = self.layer[0](
hidden_states,
attention_mask=attention_mask,
position_bias=position_bias,
layer_head_mask=layer_head_mask,
past_key_value=self_attn_past_key_value,
use_cache=use_cache,
output_attentions=output_attentions,
training=training,
)
hidden_states, present_key_value_state = self_attention_outputs[:2]
attention_outputs = self_attention_outputs[2:] # Keep self-attention outputs and relative position weights
if self.is_decoder and encoder_hidden_states is not None:
# the actual query length is unknown for cross attention
# if using past key value states. Need to inject it here
if present_key_value_state is not None:
query_length = shape_list(present_key_value_state[0])[2]
else:
query_length = None
cross_attention_outputs = self.layer[1](
hidden_states,
key_value_states=encoder_hidden_states,
attention_mask=encoder_attention_mask,
position_bias=encoder_decoder_position_bias,
layer_head_mask=encoder_layer_head_mask,
past_key_value=cross_attn_past_key_value,
query_length=query_length,
use_cache=use_cache,
output_attentions=output_attentions,
training=training,
)
hidden_states = cross_attention_outputs[0]
# Combine self attn and cross attn key value states
if present_key_value_state is not None:
present_key_value_state = present_key_value_state + cross_attention_outputs[1]
# Keep cross-attention outputs and relative position weights
attention_outputs = attention_outputs + cross_attention_outputs[2:]
# Apply Feed Forward layer
hidden_states = self.layer[-1](hidden_states, training=training)
outputs = (hidden_states,)
# Add attentions if we output them
outputs = outputs + (present_key_value_state,) + attention_outputs
return outputs # hidden-states, present_key_value_states, (self-attention weights), (self-attention position bias), (cross-attention weights), (cross-attention position bias)
def build(self, input_shape=None):
if self.built:
return
self.built = True
for layer_module in self.layer:
if hasattr(layer_module, "name"):
with tf.name_scope(layer_module.name):
layer_module.build(None)
####################################################
# The full model without a specific pretrained or finetuning head is
# provided as a keras.layers.Layer usually called "TFT5MainLayer"
####################################################
@keras_serializable
class TFT5MainLayer(keras.layers.Layer):
config_class = T5Config
def __init__(self, config, embed_tokens=None, **kwargs):
super().__init__(**kwargs)
self.config = config
self.output_hidden_states = config.output_hidden_states
self.output_attentions = config.output_attentions
self.use_cache = config.use_cache
self.embed_tokens = embed_tokens
self.is_decoder = config.is_decoder
self.config = config
self.num_hidden_layers = config.num_layers
self.block = [
TFT5Block(config, has_relative_attention_bias=bool(i == 0), name=f"block_._{i}")
for i in range(config.num_layers)
]
self.final_layer_norm = TFT5LayerNorm(
config.d_model, epsilon=config.layer_norm_epsilon, name="final_layer_norm"
)
self.dropout = keras.layers.Dropout(config.dropout_rate)
def _prune_heads(self, heads_to_prune):
raise NotImplementedError # Not implemented yet in the library fr TF 2.0 models
@unpack_inputs
def call(
self,
input_ids=None,
attention_mask=None,
encoder_hidden_states=None,
encoder_attention_mask=None,
inputs_embeds=None,
head_mask=None,
encoder_head_mask=None,
past_key_values=None,
use_cache=None,
output_attentions=None,
output_hidden_states=None,
return_dict=None,
training=False,
) -> Tuple:
if input_ids is not None and inputs_embeds is not None:
err_msg_prefix = "decoder_" if self.is_decoder else ""
raise ValueError(
f"You cannot specify both {err_msg_prefix}input_ids and {err_msg_prefix}inputs_embeds at the same time"
)
elif input_ids is not None:
input_shape = shape_list(input_ids)
input_ids = tf.reshape(input_ids, (-1, input_shape[-1]))
elif inputs_embeds is not None:
input_shape = shape_list(inputs_embeds)[:-1]
else:
err_msg_prefix = "decoder_" if self.is_decoder else ""
raise ValueError(f"You have to specify either {err_msg_prefix}input_ids or {err_msg_prefix}inputs_embeds")
if inputs_embeds is None:
assert self.embed_tokens is not None, "You have to initialize the model with valid token embeddings"
check_embeddings_within_bounds(input_ids, self.embed_tokens.input_dim)
inputs_embeds = self.embed_tokens(input_ids)
batch_size, seq_length = input_shape
# required mask seq length can be calculated via length of past
mask_seq_length = (
shape_list(past_key_values[0][0])[2] + seq_length if past_key_values is not None else seq_length
)
if attention_mask is None:
attention_mask = tf.fill((batch_size, mask_seq_length), 1)
if self.is_decoder and encoder_attention_mask is None and encoder_hidden_states is not None:
encoder_seq_length = shape_list(encoder_hidden_states)[1]
encoder_attention_mask = tf.fill((batch_size, encoder_seq_length), 1)
# initialize past_key_values with `None` if past does not exist
if past_key_values is None:
past_key_values = [None] * len(self.block)
# We can provide a self-attention mask of dimensions [batch_size, from_seq_length, to_seq_length]
# ourselves in which case we just need to make it broadcastable to all heads.
attention_mask = tf.cast(attention_mask, dtype=inputs_embeds.dtype)
num_dims_attention_mask = len(shape_list(attention_mask))
if num_dims_attention_mask == 3:
extended_attention_mask = attention_mask[:, None, :, :]
elif num_dims_attention_mask == 2:
# Provided a padding mask of dimensions [batch_size, mask_seq_length]
# - if the model is a decoder, apply a causal mask in addition to the padding mask
# - if the model is an encoder, make the mask broadcastable to [batch_size, num_heads, mask_seq_length, mask_seq_length]
if self.is_decoder:
seq_ids = tf.range(mask_seq_length)
causal_mask = tf.less_equal(
tf.tile(seq_ids[None, None, :], (batch_size, mask_seq_length, 1)),
seq_ids[None, :, None],
)
causal_mask = tf.cast(causal_mask, dtype=attention_mask.dtype)
extended_attention_mask = causal_mask[:, None, :, :] * attention_mask[:, None, None, :]
if past_key_values[0] is not None:
extended_attention_mask = extended_attention_mask[:, :, -seq_length:, :]
else:
extended_attention_mask = attention_mask[:, None, None, :]
# Since attention_mask is 1.0 for positions we want to attend and 0.0 for
# masked positions, this operation will create a tensor which is 0.0 for
# positions we want to attend and -1e9 for masked positions.
# Since we are adding it to the raw scores before the softmax, this is
# effectively the same as removing these entirely.
# T5 has a mask that can compare sequence ids, we can simulate this here with this transposition
# Cf. https://github.com/tensorflow/mesh/blob/8d2465e9bc93129b913b5ccc6a59aa97abd96ec6/mesh_tensorflow/transformer/transformer_layers.py#L270
# extended_attention_mask = tf.math.equal(extended_attention_mask,
# tf.transpose(extended_attention_mask, perm=(-1, -2)))
extended_attention_mask = (1.0 - extended_attention_mask) * -1e9
if self.is_decoder and encoder_attention_mask is not None:
# If a 2D ou 3D attention mask is provided for the cross-attention
# we need to make broadcastable to [batch_size, num_heads, mask_seq_length, mask_seq_length]
# we need to make broadcastable to [batch_size, num_heads, seq_length, seq_length]
encoder_attention_mask = tf.cast(encoder_attention_mask, dtype=extended_attention_mask.dtype)
num_dims_encoder_attention_mask = len(shape_list(encoder_attention_mask))
if num_dims_encoder_attention_mask == 3:
encoder_extended_attention_mask = encoder_attention_mask[:, None, :, :]
if num_dims_encoder_attention_mask == 2:
encoder_extended_attention_mask = encoder_attention_mask[:, None, None, :]
# T5 has a mask that can compare sequence ids, we can simulate this here with this transposition
# Cf. https://github.com/tensorflow/mesh/blob/8d2465e9bc93129b913b5ccc6a59aa97abd96ec6/mesh_tensorflow/transformer/transformer_layers.py#L270
# encoder_extended_attention_mask = tf.math.equal(encoder_extended_attention_mask,
# tf.transpose(encoder_extended_attention_mask, perm=(-1, -2)))
encoder_extended_attention_mask = (1.0 - encoder_extended_attention_mask) * -1e9
else:
encoder_extended_attention_mask = None
present_key_value_states = () if use_cache and self.is_decoder else None
all_hidden_states = () if output_hidden_states else None
all_attentions = () if output_attentions else None
all_cross_attentions = () if (output_attentions and self.is_decoder) else None
position_bias = None
encoder_decoder_position_bias = None
hidden_states = self.dropout(inputs_embeds, training=training)
for idx, (layer_module, past_key_value) in enumerate(zip(self.block, past_key_values)):
if output_hidden_states:
all_hidden_states = all_hidden_states + (hidden_states,)
layer_outputs = layer_module(
hidden_states,
attention_mask=extended_attention_mask,
position_bias=position_bias,
encoder_hidden_states=encoder_hidden_states,
encoder_attention_mask=encoder_extended_attention_mask,
encoder_decoder_position_bias=encoder_decoder_position_bias,
layer_head_mask=head_mask[idx] if head_mask is not None else None,
encoder_layer_head_mask=encoder_head_mask[idx] if encoder_head_mask is not None else None,
past_key_value=past_key_value,
use_cache=use_cache,
output_attentions=output_attentions,
training=training,
)
# layer_outputs is a tuple with:
# hidden-states, key-value-states, (self-attention weights), (self-attention position bias), (cross-attention weights), (cross-attention position bias)
hidden_states, present_key_value_state = layer_outputs[:2]
# We share the position biases between the layers - the first layer store them
# layer_outputs = hidden-states, past_key_values, (self-attention weights),
# (self-attention position bias), (cross-attention position bias), (cross-attention weights),
position_bias = layer_outputs[2]
if self.is_decoder and encoder_hidden_states is not None:
encoder_decoder_position_bias = layer_outputs[4 if output_attentions else 3]
# append next layer key value states
if present_key_value_state is not None and use_cache and self.is_decoder:
present_key_value_states = present_key_value_states + (present_key_value_state,)
if output_attentions:
all_attentions = all_attentions + (layer_outputs[3],)
if self.is_decoder:
all_cross_attentions = all_cross_attentions + (layer_outputs[5],)
hidden_states = self.final_layer_norm(hidden_states)
hidden_states = self.dropout(hidden_states, training=training)
# Add last layer
if output_hidden_states:
all_hidden_states = all_hidden_states + (hidden_states,)
if not return_dict:
outputs = (hidden_states,)
# need to check if is decoder here as well for special cases when using keras compile
if use_cache and self.is_decoder:
outputs = outputs + (present_key_value_states,)
if output_hidden_states:
outputs = outputs + (all_hidden_states,)
if output_attentions:
outputs = outputs + (all_attentions,)
if self.is_decoder:
outputs + (all_cross_attentions,)
return outputs # last-layer hidden state, (past_key_values), (all hidden states), (all attentions), (all_cross_attentions)
if self.is_decoder:
return TFBaseModelOutputWithPastAndCrossAttentions(
last_hidden_state=hidden_states,
past_key_values=present_key_value_states,
hidden_states=all_hidden_states,
attentions=all_attentions,
cross_attentions=all_cross_attentions,
)
else:
return TFBaseModelOutput(
last_hidden_state=hidden_states,
hidden_states=all_hidden_states,
attentions=all_attentions,
)
def build(self, input_shape=None):
if self.built:
return
self.built = True
if getattr(self, "final_layer_norm", None) is not None:
with tf.name_scope(self.final_layer_norm.name):
self.final_layer_norm.build(None)
if getattr(self, "block", None) is not None:
for layer in self.block:
with tf.name_scope(layer.name):
layer.build(None)
####################################################
# TFT5PreTrainedModel is a sub-class of keras.Model
# which take care of loading and saving pretrained weights
# and various common utilities.
# Here you just need to specify a few (self-explanatory)
# pointers for your model.
####################################################
class TFT5PreTrainedModel(TFPreTrainedModel):
"""
An abstract class to handle weights initialization and a simple interface for downloading and loading pretrained
models.
"""
config_class = T5Config
base_model_prefix = "transformer"
# names with a '.' represents the authorized unexpected/missing layers when a TF model is loaded from a PT model
_keys_to_ignore_on_load_unexpected = [r"decoder\Wblock[\W_0]+layer[\W_1]+EncDecAttention\Wrelative_attention_bias"]
def get_input_embeddings(self):
return self.shared
def set_input_embeddings(self, value):
self.shared = value
self.encoder.embed_tokens = self.shared
if hasattr(self, "decoder"):
self.decoder.embed_tokens = self.shared
def _shift_right(self, input_ids):
decoder_start_token_id = self.config.decoder_start_token_id
pad_token_id = self.config.pad_token_id
assert decoder_start_token_id is not None, (
"self.model.config.decoder_start_token_id has to be defined. In TF T5 it is usually set to the"
" pad_token_id. See T5 docs for more information"
)
start_tokens = tf.fill((shape_list(input_ids)[0], 1), decoder_start_token_id)
start_tokens = tf.cast(start_tokens, input_ids.dtype) # Ensure compatible dtypes for concatenation
shifted_input_ids = tf.concat([start_tokens, input_ids[:, :-1]], -1)
assert pad_token_id is not None, "self.model.config.pad_token_id has to be defined."
# replace possible -100 values in labels by `pad_token_id`
shifted_input_ids = tf.where(
shifted_input_ids == -100,
tf.cast(tf.fill(shape_list(shifted_input_ids), pad_token_id), shifted_input_ids.dtype),
shifted_input_ids,
)
# "Verify that `labels` has only positive values and -100"
assert_gte0 = tf.debugging.assert_greater_equal(
shifted_input_ids, tf.constant(0, dtype=shifted_input_ids.dtype)
)
# Make sure the assertion op is called by wrapping the result in an identity no-op
with tf.control_dependencies([assert_gte0]):
shifted_input_ids = tf.identity(shifted_input_ids)
return shifted_input_ids
T5_START_DOCSTRING = r"""
The T5 model was proposed in [Exploring the Limits of Transfer Learning with a Unified Text-to-Text
Transformer](https://arxiv.org/abs/1910.10683) by Colin Raffel, Noam Shazeer, Adam Roberts, Katherine Lee, Sharan
Narang, Michael Matena, Yanqi Zhou, Wei Li, Peter J. Liu. It's an encoder decoder transformer pre-trained in a
text-to-text denoising generative setting.
This model inherits from [`TFPreTrainedModel`]. Check the superclass documentation for the generic methods the
library implements for all its model (such as downloading or saving, resizing the input embeddings, pruning heads
etc.)
This model is also a [keras.Model](https://www.tensorflow.org/api_docs/python/tf/keras/Model) subclass. Use it
as a regular TF 2.0 Keras Model and refer to the TF 2.0 documentation for all matter related to general usage and
behavior.
<Tip>
TensorFlow models and layers in `transformers` accept two formats as input:
- having all inputs as keyword arguments (like PyTorch models), or
- having all inputs as a list, tuple or dict in the first positional argument.
The reason the second format is supported is that Keras methods prefer this format when passing inputs to models
and layers. Because of this support, when using methods like `model.fit()` things should "just work" for you - just
pass your inputs and labels in any format that `model.fit()` supports! If, however, you want to use the second
format outside of Keras methods like `fit()` and `predict()`, such as when creating your own layers or models with
the Keras `Functional` API, there are three possibilities you can use to gather all the input Tensors in the first
positional argument:
- a single Tensor with `input_ids` only and nothing else: `model(input_ids)`
- a list of varying length with one or several input Tensors IN THE ORDER given in the docstring:
`model([input_ids, attention_mask])` or `model([input_ids, attention_mask, token_type_ids])`
- a dictionary with one or several input Tensors associated to the input names given in the docstring:
`model({"input_ids": input_ids, "token_type_ids": token_type_ids})`
Note that when creating models and layers with
[subclassing](https://keras.io/guides/making_new_layers_and_models_via_subclassing/) then you don't need to worry
about any of this, as you can just pass inputs like you would to any other Python function!
</Tip>
Parameters:
config ([`T5Config`]): Model configuration class with all the parameters of the model.
Initializing with a config file does not load the weights associated with the model, only the
configuration. Check out the [`~PreTrainedModel.from_pretrained`] method to load the model weights.
"""
T5_INPUTS_DOCSTRING = r"""
Args:
input_ids (`tf.Tensor` of shape `(batch_size, sequence_length)`):
Indices of input sequence tokens in the vocabulary. T5 is a model with relative position embeddings so you
should be able to pad the inputs on the right or the left.
Indices can be obtained using [`AutoTokenizer`]. See [`PreTrainedTokenizer.__call__`] and
[`PreTrainedTokenizer.encode`] for details.
[What are input IDs?](../glossary#input-ids)
To know more on how to prepare `inputs` for pretraining take a look at [T5 Training](./t5#training).
decoder_input_ids (`tf.Tensor` of shape `(batch_size, target_sequence_length)`, *optional*):
Provide for sequence to sequence training. T5 uses the `pad_token_id` as the starting token for
`decoder_input_ids` generation. If `past_key_values` is used, optionally only the last `decoder_input_ids`
have to be input (see `past_key_values`).
To know more on how to prepare `decoder_input_ids` for pretraining take a look at [T5
Training](./t5#training).
attention_mask (`tf.Tensor` of shape `(batch_size, sequence_length)`, *optional*):
Mask to avoid performing attention on padding token indices. Mask values selected in `[0, 1]`:
- 1 for tokens that are **not masked**,
- 0 for tokens that are **masked**.
[What are attention masks?](../glossary#attention-mask)
decoder_attention_mask (`tf.Tensor` of shape `(batch_size, target_sequence_length)`, *optional*):
Default behavior: generate a tensor that ignores pad tokens in `decoder_input_ids`. Causal mask will also
be used by default.
head_mask (`tf.Tensor` of shape `(num_heads,)` or `(num_layers, num_heads)`, *optional*):
Mask to nullify selected heads of the self-attention modules in the encoder. Mask values selected in `[0,
1]`:
- 1 indicates the head is **not masked**,
- 0 indicates the head is **masked**.
decoder_head_mask (`tf.Tensor` of shape `(num_heads,)` or `(num_layers, num_heads)`, *optional*):
Mask to nullify selected heads of the self-attention modules in the decoder. Mask values selected in `[0,
1]`:
- 1 indicates the head is **not masked**,
- 0 indicates the head is **masked**.
encoder_outputs (`tuple(tuple(tf.FloatTensor)`, *optional*):
Tuple consists of (`last_hidden_state`, `optional`: *hidden_states*, `optional`: *attentions*)
`last_hidden_state` of shape `(batch_size, sequence_length, hidden_size)` is a sequence of hidden states at
the output of the last layer of the encoder. Used in the cross-attention of the decoder.
past_key_values (`tuple(tuple(tf.Tensor))` of length `config.n_layers` with each tuple having 4 tensors of shape `(batch_size, num_heads, sequence_length - 1, embed_size_per_head)`):
contains precomputed key and value hidden states of the attention blocks. Can be used to speed up decoding.
If `past_key_values` are used, the user can optionally input only the last `decoder_input_ids` (those that
don't have their past key value states given to this model) of shape `(batch_size, 1)` instead of all
`decoder_input_ids` of shape `(batch_size, sequence_length)`.
inputs_embeds (`tf.Tensor` of shape `(batch_size, sequence_length, hidden_size)`, *optional*):
Optionally, instead of passing `input_ids` you can choose to directly pass an embedded representation. This
is useful if you want more control over how to convert `input_ids` indices into associated vectors than the
model's internal embedding lookup matrix.
decoder_inputs_embeds (`tf.Tensor` of shape `(batch_size, target_sequence_length, hidden_size)`, *optional*):
Optionally, instead of passing `decoder_input_ids` you can choose to directly pass an embedded
representation. If `past_key_values` is used, optionally only the last `decoder_inputs_embeds` have to be
input (see `past_key_values`). This is useful if you want more control over how to convert
`decoder_input_ids` indices into associated vectors than the model's internal embedding lookup matrix.
If `decoder_input_ids` and `decoder_inputs_embeds` are both unset, `decoder_inputs_embeds` takes the value
of `inputs_embeds`.
use_cache (`bool`, *optional*, defaults to `True`):
If set to `True`, `past_key_values` key value states are returned and can be used to speed up decoding (see
`past_key_values`).
output_attentions (`bool`, *optional*):
Whether or not to return the attentions tensors of all attention layers. See `attentions` under returned
tensors for more detail. This argument can be used only in eager mode, in graph mode the value in the
config will be used instead.
output_hidden_states (`bool`, *optional*):
Whether or not to return the hidden states of all layers. See `hidden_states` under returned tensors for
more detail. This argument can be used only in eager mode, in graph mode the value in the config will be
used instead.
return_dict (`bool`, *optional*):
Whether or not to return a [`~utils.ModelOutput`] instead of a plain tuple. This argument can be used in
eager mode, in graph mode the value will always be set to True.
training (`bool`, *optional*, defaults to `False`):
Whether or not to use the model in training mode (some modules like dropout modules have different
behaviors between training and evaluation).
"""
T5_ENCODER_INPUTS_DOCSTRING = r"""
Args:
inputs (`tf.Tensor` of shape `(batch_size, sequence_length)`):
Indices of input sequence tokens in the vocabulary. T5 is a model with relative position embeddings so you
should be able to pad the inputs on the right or the left.
Indices can be obtained using [`AutoTokenizer`]. See [`PreTrainedTokenizer.__call__`] and
[`PreTrainedTokenizer.encode`] for details.
To know more on how to prepare `inputs` for pre-training take a look at [T5 Training](./t5#training).
attention_mask (`tf.Tensor` of shape `(batch_size, sequence_length)`, *optional*):
Mask to avoid performing attention on padding token indices. Mask values selected in `[0, 1]`:
- 1 for tokens that are **not masked**,
- 0 for tokens that are **masked**.
[What are attention masks?](../glossary#attention-mask)
inputs_embeds (`tf.Tensor` of shape `(batch_size, sequence_length, hidden_size)`, *optional*):
Optionally, instead of passing `input_ids` you can choose to directly pass an embedded representation. This
is useful if you want more control over how to convert `input_ids` indices into associated vectors than the
model's internal embedding lookup matrix.
head_mask (`tf.Tensor` of shape `(num_heads,)` or `(num_layers, num_heads)`, *optional*):
Mask to nullify selected heads of the self-attention modules. Mask values selected in `[0, 1]`:
- 1 indicates the head is **not masked**,
- 0 indicates the head is **masked**.
output_attentions (`bool`, *optional*):
Whether or not to return the attentions tensors of all attention layers. See `attentions` under returned
tensors for more detail.
output_hidden_states (`bool`, *optional*):
Whether or not to return the hidden states of all layers. See `hidden_states` under returned tensors for
more detail.
return_dict (`bool`, *optional*):
Whether or not to return a [`~utils.ModelOutput`] instead of a plain tuple.
training (`bool`, *optional*, defaults to `False`):
Whether or not to use the model in training mode (some modules like dropout modules have different
behaviors between training and evaluation).
"""
_HEAD_MASK_WARNING_MSG = """
The input argument `head_mask` was split into two arguments `head_mask` and `decoder_head_mask`. Currently,
`decoder_head_mask` is set to copy `head_mask`, but this feature is deprecated and will be removed in future versions.
If you do not want to use any `decoder_head_mask` now, please set `decoder_head_mask = tf.ones((num_layers,
num_heads))`.
"""
@add_start_docstrings(
"The bare T5 Model transformer outputting raw hidden-stateswithout any specific head on top.",
T5_START_DOCSTRING,
)
class TFT5Model(TFT5PreTrainedModel):
def __init__(self, config, *inputs, **kwargs):
super().__init__(config, *inputs, **kwargs)
self.shared = keras.layers.Embedding(
input_dim=config.vocab_size,
output_dim=config.d_model,
embeddings_initializer=keras.initializers.TruncatedNormal(self.config.initializer_factor),
name="shared",
)
# Additional attribute to specify the expected name scope of the layer (for loading/storing weights)
self.shared.load_weight_prefix = "shared"
encoder_config = copy.deepcopy(config)
encoder_config.use_cache = False
self.encoder = TFT5MainLayer(encoder_config, self.shared, name="encoder")
decoder_config = copy.deepcopy(config)
decoder_config.is_decoder = True
decoder_config.num_layers = config.num_decoder_layers
self.decoder = TFT5MainLayer(decoder_config, self.shared, name="decoder")
def get_encoder(self):
return self.encoder
def get_decoder(self):
return self.decoder
@unpack_inputs
@add_start_docstrings_to_model_forward(T5_INPUTS_DOCSTRING)
@replace_return_docstrings(output_type=TFSeq2SeqModelOutput, config_class=_CONFIG_FOR_DOC)
def call(
self,
input_ids: TFModelInputType | None = None,
attention_mask: np.ndarray | tf.Tensor | None = None,
decoder_input_ids: np.ndarray | tf.Tensor | None = None,
decoder_attention_mask: np.ndarray | tf.Tensor | None = None,
head_mask: np.ndarray | tf.Tensor | None = None,
decoder_head_mask: np.ndarray | tf.Tensor | None = None,
encoder_outputs: np.ndarray | tf.Tensor | None = None,
past_key_values: Optional[Tuple[Tuple[Union[np.ndarray, tf.Tensor]]]] = None,
inputs_embeds: np.ndarray | tf.Tensor | None = None,
decoder_inputs_embeds: np.ndarray | tf.Tensor | None = None,
use_cache: Optional[bool] = None,
output_attentions: Optional[bool] = None,
output_hidden_states: Optional[bool] = None,
return_dict: Optional[bool] = None,
training: Optional[bool] = False,
) -> Union[Tuple, TFSeq2SeqModelOutput]:
r"""
Returns:
Examples:
```python
>>> from transformers import AutoTokenizer, TFT5Model
>>> tokenizer = AutoTokenizer.from_pretrained("t5-small")
>>> model = TFT5Model.from_pretrained("t5-small")
>>> input_ids = tokenizer(
... "Studies have been shown that owning a dog is good for you", return_tensors="tf"
... ).input_ids # Batch size 1
>>> decoder_input_ids = tokenizer("Studies show that", return_tensors="tf").input_ids # Batch size 1
>>> # preprocess: Prepend decoder_input_ids with start token which is pad token for T5Model.
>>> # This is not needed for torch's T5ForConditionalGeneration as it does this internally using labels arg.
>>> decoder_input_ids = model._shift_right(decoder_input_ids)
>>> # forward pass
>>> outputs = model(input_ids, decoder_input_ids=decoder_input_ids)
>>> last_hidden_states = outputs.last_hidden_state
```"""
# FutureWarning: head_mask was separated into two input args - head_mask, decoder_head_mask
if head_mask is not None and decoder_head_mask is None:
warnings.warn(_HEAD_MASK_WARNING_MSG, FutureWarning)
decoder_head_mask = head_mask
# Encode if needed (training, first prediction pass)
if encoder_outputs is None:
encoder_outputs = self.encoder(
input_ids,
attention_mask=attention_mask,
encoder_hidden_states=None,
encoder_attention_mask=None,
inputs_embeds=inputs_embeds,
head_mask=head_mask,
past_key_values=None,
use_cache=False,
output_attentions=output_attentions,
output_hidden_states=output_hidden_states,
return_dict=return_dict,
training=training,
)
hidden_states = encoder_outputs[0]
# Decode
decoder_outputs = self.decoder(
decoder_input_ids,
attention_mask=decoder_attention_mask,
encoder_hidden_states=hidden_states,
encoder_attention_mask=attention_mask,
inputs_embeds=decoder_inputs_embeds,
head_mask=decoder_head_mask,
encoder_head_mask=head_mask,
past_key_values=past_key_values,
use_cache=use_cache,
output_attentions=output_attentions,
output_hidden_states=output_hidden_states,
return_dict=return_dict,
training=training,
)
past = decoder_outputs[1] if use_cache else None
if not return_dict:
if past_key_values is not None:
decoder_outputs = decoder_outputs[:1] + (past,) + decoder_outputs[2:]
return decoder_outputs + encoder_outputs
return TFSeq2SeqModelOutput(
last_hidden_state=decoder_outputs.last_hidden_state,
past_key_values=past,
decoder_hidden_states=decoder_outputs.hidden_states,
decoder_attentions=decoder_outputs.attentions,
cross_attentions=decoder_outputs.cross_attentions,
encoder_last_hidden_state=encoder_outputs.last_hidden_state,
encoder_hidden_states=encoder_outputs.hidden_states,
encoder_attentions=encoder_outputs.attentions,
)
def build(self, input_shape=None):
if self.built:
return
self.built = True
# The shared/tied weights expect to be in the model base namespace
# Adding "/" to the end (not the start!) of a tf.name_scope puts it in the root namespace rather than
# the current one.
with tf.name_scope(self.shared.load_weight_prefix + "/" + self.shared.name + "/"):
self.shared.build(None)
if getattr(self, "encoder", None) is not None:
with tf.name_scope(self.encoder.name):
self.encoder.build(None)
if getattr(self, "decoder", None) is not None:
with tf.name_scope(self.decoder.name):
self.decoder.build(None)
@add_start_docstrings("""T5 Model with a `language modeling` head on top.""", T5_START_DOCSTRING)
class TFT5ForConditionalGeneration(TFT5PreTrainedModel, TFCausalLanguageModelingLoss):
def __init__(self, config, *inputs, **kwargs):
super().__init__(config, *inputs, **kwargs)
self.model_dim = config.d_model
self.shared = keras.layers.Embedding(
config.vocab_size,
config.d_model,
name="shared",
embeddings_initializer=get_initializer(self.config.initializer_factor),
)
# Additional attribute to specify the expected name scope of the layer (for loading/storing weights)
self.shared.load_weight_prefix = "shared"
encoder_config = copy.deepcopy(config)
encoder_config.use_cache = False
self.encoder = TFT5MainLayer(encoder_config, self.shared, name="encoder")
decoder_config = copy.deepcopy(config)
decoder_config.is_decoder = True
decoder_config.num_layers = config.num_decoder_layers
self.decoder = TFT5MainLayer(decoder_config, self.shared, name="decoder")
if not config.tie_word_embeddings:
lm_head_initializer = keras.initializers.RandomNormal(mean=0, stddev=config.initializer_factor)
self.lm_head = keras.layers.Dense(
config.vocab_size, use_bias=False, name="lm_head", kernel_initializer=lm_head_initializer
) # Update init weights as in flax
self.config = config
def get_output_embeddings(self):
if self.config.tie_word_embeddings:
return self.get_input_embeddings()
else:
# in a dense layer the kernel has a shape (last_dim, units), for us (dim, num_tokens)
# value has a shape (num_tokens, dim) then needs to be transposed
return tf.transpose(self.lm_head.kernel)
def set_output_embeddings(self, value):
if self.config.tie_word_embeddings:
self.set_input_embeddings(value)
else:
lm_head_initializer = keras.initializers.RandomNormal(mean=0, stddev=self.config.initializer_factor)
self.lm_head = keras.layers.Dense(
shape_list(value)[0], use_bias=False, name="lm_head", kernel_initializer=lm_head_initializer
) # Update init weights as in flax
# in a dense layer the kernel has a shape (last_dim, units), for us (dim, num_tokens)
# value has a shape (num_tokens, dim) then needs to be transposed
transposed_value = tf.transpose(value)
self.lm_head.kernel = transposed_value
def get_encoder(self):
return self.encoder
def get_decoder(self):
return self.decoder
@unpack_inputs
@add_start_docstrings_to_model_forward(T5_INPUTS_DOCSTRING)
@replace_return_docstrings(output_type=TFSeq2SeqLMOutput, config_class=_CONFIG_FOR_DOC)
def call(
self,
input_ids: TFModelInputType | None = None,
attention_mask: np.ndarray | tf.Tensor | None = None,
decoder_input_ids: np.ndarray | tf.Tensor | None = None,
decoder_attention_mask: np.ndarray | tf.Tensor | None = None,
head_mask: np.ndarray | tf.Tensor | None = None,
decoder_head_mask: np.ndarray | tf.Tensor | None = None,
encoder_outputs: np.ndarray | tf.Tensor | None = None,
past_key_values: Optional[Tuple[Tuple[Union[np.ndarray, tf.Tensor]]]] = None,
inputs_embeds: np.ndarray | tf.Tensor | None = None,
decoder_inputs_embeds: np.ndarray | tf.Tensor | None = None,
labels: np.ndarray | tf.Tensor | None = None,
use_cache: Optional[bool] = None,
output_attentions: Optional[bool] = None,
output_hidden_states: Optional[bool] = None,
return_dict: Optional[bool] = None,
training: Optional[bool] = False,
) -> Union[Tuple, TFSeq2SeqLMOutput]:
r"""
labels (`tf.Tensor` of shape `(batch_size, sequence_length)`, *optional*):
Labels for computing the cross entropy classification loss. Indices should be in `[0, ...,
config.vocab_size - 1]`.
Returns:
Examples:
```python
>>> from transformers import AutoTokenizer, TFT5ForConditionalGeneration
>>> tokenizer = AutoTokenizer.from_pretrained("t5-small")
>>> model = TFT5ForConditionalGeneration.from_pretrained("t5-small")
>>> # training
>>> inputs = tokenizer("The <extra_id_0> walks in <extra_id_1> park", return_tensors="tf").input_ids
>>> labels = tokenizer("<extra_id_0> cute dog <extra_id_1> the <extra_id_2>", return_tensors="tf").input_ids
>>> outputs = model(inputs, labels=labels)
>>> loss = outputs.loss
>>> logits = outputs.logits
>>> # inference
>>> inputs = tokenizer(
... "summarize: studies have shown that owning a dog is good for you", return_tensors="tf"
... ).input_ids # Batch size 1
>>> outputs = model.generate(inputs)
>>> print(tokenizer.decode(outputs[0], skip_special_tokens=True))
>>> # studies have shown that owning a dog is good for you
```"""
# FutureWarning: head_mask was separated into two input args - head_mask, decoder_head_mask
if head_mask is not None and decoder_head_mask is None:
warnings.warn(_HEAD_MASK_WARNING_MSG, FutureWarning)
decoder_head_mask = head_mask
# Encode if needed (training, first prediction pass)
if encoder_outputs is None:
encoder_outputs = self.encoder(
input_ids,
attention_mask=attention_mask,
inputs_embeds=inputs_embeds,
head_mask=head_mask,
output_attentions=output_attentions,
output_hidden_states=output_hidden_states,
return_dict=return_dict,
training=training,
)
hidden_states = encoder_outputs[0]
if labels is not None and decoder_input_ids is None and decoder_inputs_embeds is None:
# get decoder inputs from shifting lm labels to the right
decoder_input_ids = self._shift_right(labels)
# Decode
decoder_outputs = self.decoder(
decoder_input_ids,
attention_mask=decoder_attention_mask,
encoder_hidden_states=hidden_states,
encoder_attention_mask=attention_mask,
inputs_embeds=decoder_inputs_embeds,
head_mask=decoder_head_mask,
past_key_values=past_key_values,
use_cache=use_cache,
output_attentions=output_attentions,
output_hidden_states=output_hidden_states,
return_dict=return_dict,
training=training,
)
sequence_output = decoder_outputs[0]
# T5v1.1 does not tie output word embeddings and thus does not require downscaling
if self.config.tie_word_embeddings:
sequence_output = sequence_output * (self.model_dim**-0.5)
logits = tf.matmul(sequence_output, self.shared.weights, transpose_b=True)
else:
logits = self.lm_head(sequence_output)
logits = tf.cast(logits, tf.float32)
loss = None if labels is None else self.hf_compute_loss(labels, logits)
past = decoder_outputs[1] if use_cache else None
if not return_dict:
if past_key_values is not None:
decoder_outputs = decoder_outputs[:1] + (past,) + decoder_outputs[2:]
output = (logits,) + decoder_outputs[1:] + encoder_outputs
return ((loss,) + output) if loss is not None else output
# If the user passed a tuple for encoder_outputs, we wrap it in a TFBaseModelOutput when return_dict=True
elif isinstance(encoder_outputs, tuple):
last_hidden_state = encoder_outputs[0]
hidden_states = None
attentions = None
idx = 0
if output_hidden_states:
idx += 1
hidden_states = encoder_outputs[idx]
if output_attentions:
idx += 1
attentions = encoder_outputs[idx]
encoder_outputs = TFBaseModelOutput(
last_hidden_state=last_hidden_state,
hidden_states=hidden_states,
attentions=attentions,
)
return TFSeq2SeqLMOutput(
loss=loss,
logits=logits,
past_key_values=past,
decoder_hidden_states=decoder_outputs.hidden_states,
decoder_attentions=decoder_outputs.attentions,
cross_attentions=decoder_outputs.cross_attentions,
encoder_last_hidden_state=encoder_outputs.last_hidden_state,
encoder_hidden_states=encoder_outputs.hidden_states,
encoder_attentions=encoder_outputs.attentions,
)
def serving_output(self, output):
pkv = tf.convert_to_tensor(output.past_key_values[1:]) if self.config.use_cache else None
dec_hs = tf.convert_to_tensor(output.decoder_hidden_states) if self.config.output_hidden_states else None
dec_attns = tf.convert_to_tensor(output.decoder_attentions) if self.config.output_attentions else None
cross_attns = tf.convert_to_tensor(output.cross_attentions) if self.config.output_attentions else None
enc_hs = tf.convert_to_tensor(output.encoder_hidden_states) if self.config.output_hidden_states else None
enc_attns = tf.convert_to_tensor(output.encoder_attentions) if self.config.output_attentions else None
return TFSeq2SeqLMOutput(
logits=output.logits,
past_key_values=pkv,
decoder_hidden_states=dec_hs,
decoder_attentions=dec_attns,
cross_attentions=cross_attns,
encoder_last_hidden_state=output.encoder_last_hidden_state,
encoder_hidden_states=enc_hs,
encoder_attentions=enc_attns,
)
def prepare_inputs_for_generation(
self,
input_ids,
past_key_values=None,
attention_mask=None,
decoder_attention_mask=None,
head_mask=None,
decoder_head_mask=None,
use_cache=None,
encoder_outputs=None,
**kwargs,
):
# cut decoder_input_ids if past is used
if past_key_values is not None:
input_ids = input_ids[:, -1:]
return {
"input_ids": None, # needs to be passed to make Keras.layer.__call__ happy
"decoder_input_ids": input_ids,
"past_key_values": past_key_values,
"encoder_outputs": encoder_outputs,
"attention_mask": attention_mask,
"decoder_attention_mask": decoder_attention_mask,
"head_mask": head_mask,
"decoder_head_mask": decoder_head_mask,
"use_cache": use_cache,
}
def prepare_decoder_input_ids_from_labels(self, labels: tf.Tensor):
return self._shift_right(labels)
def build(self, input_shape=None):
if self.built:
return
self.built = True
# The shared/tied weights expect to be in the model base namespace
# Adding "/" to the end (not the start!) of a tf.name_scope puts it in the root namespace rather than
# the current one.
with tf.name_scope(self.shared.load_weight_prefix + "/" + self.shared.name + "/"):
self.shared.build(None)
if getattr(self, "encoder", None) is not None:
with tf.name_scope(self.encoder.name):
self.encoder.build(None)
if getattr(self, "decoder", None) is not None:
with tf.name_scope(self.decoder.name):
self.decoder.build(None)
if getattr(self, "lm_head", None) is not None:
with tf.name_scope(self.lm_head.name):
self.lm_head.build([None, None, self.config.d_model])
@add_start_docstrings(
"The bare T5 Model transformer outputting encoder's raw hidden-stateswithout any specific head on top.",
T5_START_DOCSTRING,
)
class TFT5EncoderModel(TFT5PreTrainedModel):
def __init__(self, config, *inputs, **kwargs):
super().__init__(config, *inputs, **kwargs)
self.shared = keras.layers.Embedding(
config.vocab_size,
config.d_model,
name="shared",
embeddings_initializer=get_initializer(self.config.initializer_factor),
)
# Additional attribute to specify the expected name scope of the layer (for loading/storing weights)
self.shared.load_weight_prefix = "shared"
encoder_config = copy.deepcopy(config)
encoder_config.use_cache = False
self.encoder = TFT5MainLayer(encoder_config, self.shared, name="encoder")
def get_encoder(self):
return self.encoder
@unpack_inputs
@add_start_docstrings_to_model_forward(T5_ENCODER_INPUTS_DOCSTRING)
@replace_return_docstrings(output_type=TFBaseModelOutput, config_class=_CONFIG_FOR_DOC)
def call(
self,
input_ids: TFModelInputType | None = None,
attention_mask: np.ndarray | tf.Tensor | None = None,
head_mask: np.ndarray | tf.Tensor | None = None,
inputs_embeds: np.ndarray | tf.Tensor | None = None,
output_attentions: Optional[bool] = None,
output_hidden_states: Optional[bool] = None,
return_dict: Optional[bool] = None,
training: Optional[bool] = False,
) -> Union[Tuple, TFBaseModelOutput]:
r"""
Returns:
Examples:
```python
>>> from transformers import AutoTokenizer, TFT5EncoderModel
>>> tokenizer = AutoTokenizer.from_pretrained("t5-small")
>>> model = TFT5EncoderModel.from_pretrained("t5-small")
>>> input_ids = tokenizer(
... "Studies have been shown that owning a dog is good for you", return_tensors="tf"
... ).input_ids # Batch size 1
>>> outputs = model(input_ids)
```"""
encoder_outputs = self.encoder(
input_ids,
attention_mask=attention_mask,
encoder_hidden_states=None,
encoder_attention_mask=None,
inputs_embeds=inputs_embeds,
head_mask=head_mask,
past_key_values=None,
use_cache=False,
output_attentions=output_attentions,
output_hidden_states=output_hidden_states,
return_dict=return_dict,
training=training,
)
if not return_dict:
return encoder_outputs
return TFBaseModelOutput(
last_hidden_state=encoder_outputs.last_hidden_state,
hidden_states=encoder_outputs.hidden_states,
attentions=encoder_outputs.attentions,
)
def build(self, input_shape=None):
if self.built:
return
self.built = True
# The shared/tied weights expect to be in the model base namespace
# Adding "/" to the end (not the start!) of a tf.name_scope puts it in the root namespace rather than
# the current one.
with tf.name_scope(self.shared.load_weight_prefix + "/" + self.shared.name + "/"):
self.shared.build(None)
if getattr(self, "encoder", None) is not None:
with tf.name_scope(self.encoder.name):
self.encoder.build(None)
| transformers/src/transformers/models/t5/modeling_tf_t5.py/0 | {
"file_path": "transformers/src/transformers/models/t5/modeling_tf_t5.py",
"repo_id": "transformers",
"token_count": 33722
} | 326 |
# coding=utf-8
# Copyright 2022 The HuggingFace Inc. team. All rights reserved.
#
# Licensed under the Apache License, Version 2.0 (the "License");
# you may not use this file except in compliance with the License.
# You may obtain a copy of the License at
#
# http://www.apache.org/licenses/LICENSE-2.0
#
# Unless required by applicable law or agreed to in writing, software
# distributed under the License is distributed on an "AS IS" BASIS,
# WITHOUT WARRANTIES OR CONDITIONS OF ANY KIND, either express or implied.
# See the License for the specific language governing permissions and
# limitations under the License.
""" VideoMAE model configuration"""
from ...configuration_utils import PretrainedConfig
from ...utils import logging
logger = logging.get_logger(__name__)
VIDEOMAE_PRETRAINED_CONFIG_ARCHIVE_MAP = {
"MCG-NJU/videomae-base": "https://huggingface.co/MCG-NJU/videomae-base/resolve/main/config.json",
}
class VideoMAEConfig(PretrainedConfig):
r"""
This is the configuration class to store the configuration of a [`VideoMAEModel`]. It is used to instantiate a
VideoMAE model according to the specified arguments, defining the model architecture. Instantiating a configuration
with the defaults will yield a similar configuration to that of the VideoMAE
[MCG-NJU/videomae-base](https://huggingface.co/MCG-NJU/videomae-base) architecture.
Configuration objects inherit from [`PretrainedConfig`] and can be used to control the model outputs. Read the
documentation from [`PretrainedConfig`] for more information.
Args:
image_size (`int`, *optional*, defaults to 224):
The size (resolution) of each image.
patch_size (`int`, *optional*, defaults to 16):
The size (resolution) of each patch.
num_channels (`int`, *optional*, defaults to 3):
The number of input channels.
num_frames (`int`, *optional*, defaults to 16):
The number of frames in each video.
tubelet_size (`int`, *optional*, defaults to 2):
The number of tubelets.
hidden_size (`int`, *optional*, defaults to 768):
Dimensionality of the encoder layers and the pooler layer.
num_hidden_layers (`int`, *optional*, defaults to 12):
Number of hidden layers in the Transformer encoder.
num_attention_heads (`int`, *optional*, defaults to 12):
Number of attention heads for each attention layer in the Transformer encoder.
intermediate_size (`int`, *optional*, defaults to 3072):
Dimensionality of the "intermediate" (i.e., feed-forward) layer in the Transformer encoder.
hidden_act (`str` or `function`, *optional*, defaults to `"gelu"`):
The non-linear activation function (function or string) in the encoder and pooler. If string, `"gelu"`,
`"relu"`, `"selu"` and `"gelu_new"` are supported.
hidden_dropout_prob (`float`, *optional*, defaults to 0.0):
The dropout probability for all fully connected layers in the embeddings, encoder, and pooler.
attention_probs_dropout_prob (`float`, *optional*, defaults to 0.0):
The dropout ratio for the attention probabilities.
initializer_range (`float`, *optional*, defaults to 0.02):
The standard deviation of the truncated_normal_initializer for initializing all weight matrices.
layer_norm_eps (`float`, *optional*, defaults to 1e-12):
The epsilon used by the layer normalization layers.
qkv_bias (`bool`, *optional*, defaults to `True`):
Whether to add a bias to the queries, keys and values.
use_mean_pooling (`bool`, *optional*, defaults to `True`):
Whether to mean pool the final hidden states instead of using the final hidden state of the [CLS] token.
decoder_num_attention_heads (`int`, *optional*, defaults to 6):
Number of attention heads for each attention layer in the decoder.
decoder_hidden_size (`int`, *optional*, defaults to 384):
Dimensionality of the decoder.
decoder_num_hidden_layers (`int`, *optional*, defaults to 4):
Number of hidden layers in the decoder.
decoder_intermediate_size (`int`, *optional*, defaults to 1536):
Dimensionality of the "intermediate" (i.e., feed-forward) layer in the decoder.
norm_pix_loss (`bool`, *optional*, defaults to `True`):
Whether to normalize the target patch pixels.
Example:
```python
>>> from transformers import VideoMAEConfig, VideoMAEModel
>>> # Initializing a VideoMAE videomae-base style configuration
>>> configuration = VideoMAEConfig()
>>> # Randomly initializing a model from the configuration
>>> model = VideoMAEModel(configuration)
>>> # Accessing the model configuration
>>> configuration = model.config
```"""
model_type = "videomae"
def __init__(
self,
image_size=224,
patch_size=16,
num_channels=3,
num_frames=16,
tubelet_size=2,
hidden_size=768,
num_hidden_layers=12,
num_attention_heads=12,
intermediate_size=3072,
hidden_act="gelu",
hidden_dropout_prob=0.0,
attention_probs_dropout_prob=0.0,
initializer_range=0.02,
layer_norm_eps=1e-12,
qkv_bias=True,
use_mean_pooling=True,
decoder_num_attention_heads=6,
decoder_hidden_size=384,
decoder_num_hidden_layers=4,
decoder_intermediate_size=1536,
norm_pix_loss=True,
**kwargs,
):
super().__init__(**kwargs)
self.image_size = image_size
self.patch_size = patch_size
self.num_channels = num_channels
self.num_frames = num_frames
self.tubelet_size = tubelet_size
self.hidden_size = hidden_size
self.num_hidden_layers = num_hidden_layers
self.num_attention_heads = num_attention_heads
self.intermediate_size = intermediate_size
self.hidden_act = hidden_act
self.hidden_dropout_prob = hidden_dropout_prob
self.attention_probs_dropout_prob = attention_probs_dropout_prob
self.initializer_range = initializer_range
self.layer_norm_eps = layer_norm_eps
self.qkv_bias = qkv_bias
self.use_mean_pooling = use_mean_pooling
self.decoder_num_attention_heads = decoder_num_attention_heads
self.decoder_hidden_size = decoder_hidden_size
self.decoder_num_hidden_layers = decoder_num_hidden_layers
self.decoder_intermediate_size = decoder_intermediate_size
self.norm_pix_loss = norm_pix_loss
| transformers/src/transformers/models/videomae/configuration_videomae.py/0 | {
"file_path": "transformers/src/transformers/models/videomae/configuration_videomae.py",
"repo_id": "transformers",
"token_count": 2593
} | 327 |
# coding=utf-8
# Copyright 2021 Google AI and The HuggingFace Inc. team. All rights reserved.
#
# Licensed under the Apache License, Version 2.0 (the "License");
# you may not use this file except in compliance with the License.
# You may obtain a copy of the License at
#
# http://www.apache.org/licenses/LICENSE-2.0
#
# Unless required by applicable law or agreed to in writing, software
# distributed under the License is distributed on an "AS IS" BASIS,
# WITHOUT WARRANTIES OR CONDITIONS OF ANY KIND, either express or implied.
# See the License for the specific language governing permissions and
# limitations under the License.
""" ViT model configuration"""
from collections import OrderedDict
from typing import Mapping
from packaging import version
from ...configuration_utils import PretrainedConfig
from ...onnx import OnnxConfig
from ...utils import logging
logger = logging.get_logger(__name__)
VIT_PRETRAINED_CONFIG_ARCHIVE_MAP = {
"google/vit-base-patch16-224": "https://huggingface.co/vit-base-patch16-224/resolve/main/config.json",
# See all ViT models at https://huggingface.co/models?filter=vit
}
class ViTConfig(PretrainedConfig):
r"""
This is the configuration class to store the configuration of a [`ViTModel`]. It is used to instantiate an ViT
model according to the specified arguments, defining the model architecture. Instantiating a configuration with the
defaults will yield a similar configuration to that of the ViT
[google/vit-base-patch16-224](https://huggingface.co/google/vit-base-patch16-224) architecture.
Configuration objects inherit from [`PretrainedConfig`] and can be used to control the model outputs. Read the
documentation from [`PretrainedConfig`] for more information.
Args:
hidden_size (`int`, *optional*, defaults to 768):
Dimensionality of the encoder layers and the pooler layer.
num_hidden_layers (`int`, *optional*, defaults to 12):
Number of hidden layers in the Transformer encoder.
num_attention_heads (`int`, *optional*, defaults to 12):
Number of attention heads for each attention layer in the Transformer encoder.
intermediate_size (`int`, *optional*, defaults to 3072):
Dimensionality of the "intermediate" (i.e., feed-forward) layer in the Transformer encoder.
hidden_act (`str` or `function`, *optional*, defaults to `"gelu"`):
The non-linear activation function (function or string) in the encoder and pooler. If string, `"gelu"`,
`"relu"`, `"selu"` and `"gelu_new"` are supported.
hidden_dropout_prob (`float`, *optional*, defaults to 0.0):
The dropout probability for all fully connected layers in the embeddings, encoder, and pooler.
attention_probs_dropout_prob (`float`, *optional*, defaults to 0.0):
The dropout ratio for the attention probabilities.
initializer_range (`float`, *optional*, defaults to 0.02):
The standard deviation of the truncated_normal_initializer for initializing all weight matrices.
layer_norm_eps (`float`, *optional*, defaults to 1e-12):
The epsilon used by the layer normalization layers.
image_size (`int`, *optional*, defaults to 224):
The size (resolution) of each image.
patch_size (`int`, *optional*, defaults to 16):
The size (resolution) of each patch.
num_channels (`int`, *optional*, defaults to 3):
The number of input channels.
qkv_bias (`bool`, *optional*, defaults to `True`):
Whether to add a bias to the queries, keys and values.
encoder_stride (`int`, *optional*, defaults to 16):
Factor to increase the spatial resolution by in the decoder head for masked image modeling.
Example:
```python
>>> from transformers import ViTConfig, ViTModel
>>> # Initializing a ViT vit-base-patch16-224 style configuration
>>> configuration = ViTConfig()
>>> # Initializing a model (with random weights) from the vit-base-patch16-224 style configuration
>>> model = ViTModel(configuration)
>>> # Accessing the model configuration
>>> configuration = model.config
```"""
model_type = "vit"
def __init__(
self,
hidden_size=768,
num_hidden_layers=12,
num_attention_heads=12,
intermediate_size=3072,
hidden_act="gelu",
hidden_dropout_prob=0.0,
attention_probs_dropout_prob=0.0,
initializer_range=0.02,
layer_norm_eps=1e-12,
image_size=224,
patch_size=16,
num_channels=3,
qkv_bias=True,
encoder_stride=16,
**kwargs,
):
super().__init__(**kwargs)
self.hidden_size = hidden_size
self.num_hidden_layers = num_hidden_layers
self.num_attention_heads = num_attention_heads
self.intermediate_size = intermediate_size
self.hidden_act = hidden_act
self.hidden_dropout_prob = hidden_dropout_prob
self.attention_probs_dropout_prob = attention_probs_dropout_prob
self.initializer_range = initializer_range
self.layer_norm_eps = layer_norm_eps
self.image_size = image_size
self.patch_size = patch_size
self.num_channels = num_channels
self.qkv_bias = qkv_bias
self.encoder_stride = encoder_stride
class ViTOnnxConfig(OnnxConfig):
torch_onnx_minimum_version = version.parse("1.11")
@property
def inputs(self) -> Mapping[str, Mapping[int, str]]:
return OrderedDict(
[
("pixel_values", {0: "batch", 1: "num_channels", 2: "height", 3: "width"}),
]
)
@property
def atol_for_validation(self) -> float:
return 1e-4
| transformers/src/transformers/models/vit/configuration_vit.py/0 | {
"file_path": "transformers/src/transformers/models/vit/configuration_vit.py",
"repo_id": "transformers",
"token_count": 2203
} | 328 |
# coding=utf-8
# Copyright 2022 Facebook AI and The HuggingFace Inc. team. All rights reserved.
#
# Licensed under the Apache License, Version 2.0 (the "License");
# you may not use this file except in compliance with the License.
# You may obtain a copy of the License at
#
# http://www.apache.org/licenses/LICENSE-2.0
#
# Unless required by applicable law or agreed to in writing, software
# distributed under the License is distributed on an "AS IS" BASIS,
# WITHOUT WARRANTIES OR CONDITIONS OF ANY KIND, either express or implied.
# See the License for the specific language governing permissions and
# limitations under the License.
""" TF 2.0 ViT MAE (masked autoencoder) model."""
from __future__ import annotations
import collections.abc
import math
from copy import deepcopy
from dataclasses import dataclass
from typing import Optional, Tuple, Union
import numpy as np
import tensorflow as tf
from ...activations_tf import get_tf_activation
from ...file_utils import (
ModelOutput,
add_start_docstrings,
add_start_docstrings_to_model_forward,
replace_return_docstrings,
)
from ...modeling_tf_outputs import TFBaseModelOutput
from ...modeling_tf_utils import (
TFModelInputType,
TFPreTrainedModel,
get_initializer,
keras,
keras_serializable,
unpack_inputs,
)
from ...tf_utils import shape_list, stable_softmax
from ...utils import logging
from .configuration_vit_mae import ViTMAEConfig
logger = logging.get_logger(__name__)
_CONFIG_FOR_DOC = "ViTMAEConfig"
_CHECKPOINT_FOR_DOC = "facebook/vit-mae-base"
@dataclass
class TFViTMAEModelOutput(ModelOutput):
"""
Class for TFViTMAEModel's outputs, with potential hidden states and attentions.
Args:
last_hidden_state (`tf.Tensor` of shape `(batch_size, sequence_length, hidden_size)`):
Sequence of hidden-states at the output of the last layer of the model.
mask (`tf.Tensor` of shape `(batch_size, sequence_length)`):
Tensor indicating which patches are masked (1) and which are not (0).
ids_restore (`tf.Tensor` of shape `(batch_size, sequence_length)`):
Tensor containing the original index of the (shuffled) masked patches.
hidden_states (`tuple(tf.Tensor)`, *optional*, returned when `output_hidden_states=True` is passed or when `config.output_hidden_states=True`):
Tuple of `tf.Tensor` (one for the output of the embeddings + one for the output of each layer) of shape
`(batch_size, sequence_length, hidden_size)`. Hidden-states of the model at the output of each layer plus
the initial embedding outputs.
attentions (`tuple(tf.Tensor)`, *optional*, returned when `output_attentions=True` is passed or when `config.output_attentions=True`):
Tuple of `tf.Tensor` (one for each layer) of shape `(batch_size, num_heads, sequence_length,
sequence_length)`. Attentions weights after the attention softmax, used to compute the weighted average in
the self-attention heads.
"""
last_hidden_state: tf.Tensor = None
mask: tf.Tensor = None
ids_restore: tf.Tensor = None
hidden_states: Tuple[tf.Tensor] | None = None
attentions: Tuple[tf.Tensor] | None = None
@dataclass
class TFViTMAEDecoderOutput(ModelOutput):
"""
Class for TFViTMAEDecoder's outputs, with potential hidden states and attentions.
Args:
logits (`tf.Tensor` of shape `(batch_size, sequence_length, patch_size ** 2 * num_channels)`):
Pixel reconstruction logits.
hidden_states (`tuple(tf.Tensor)`, *optional*, returned when `output_hidden_states=True` is passed or when `config.output_hidden_states=True`):
Tuple of `tf.Tensor` (one for the output of the embeddings + one for the output of each layer) of shape
`(batch_size, sequence_length, hidden_size)`. Hidden-states of the model at the output of each layer plus
the initial embedding outputs.
attentions (`tuple(tf.Tensor)`, *optional*, returned when `output_attentions=True` is passed or when `config.output_attentions=True`):
Tuple of `tf.Tensor` (one for each layer) of shape `(batch_size, num_heads, sequence_length,
sequence_length)`. Attentions weights after the attention softmax, used to compute the weighted average in
the self-attention heads.
"""
logits: tf.Tensor = None
hidden_states: Tuple[tf.Tensor] | None = None
attentions: Tuple[tf.Tensor] | None = None
@dataclass
class TFViTMAEForPreTrainingOutput(ModelOutput):
"""
Class for TFViTMAEForPreTraining's outputs, with potential hidden states and attentions.
Args:
loss (`tf.Tensor` of shape `(1,)`):
Pixel reconstruction loss.
logits (`tf.Tensor` of shape `(batch_size, sequence_length, patch_size ** 2 * num_channels)`):
Pixel reconstruction logits.
mask (`tf.Tensor` of shape `(batch_size, sequence_length)`):
Tensor indicating which patches are masked (1) and which are not (0).
ids_restore (`tf.Tensor` of shape `(batch_size, sequence_length)`):
Tensor containing the original index of the (shuffled) masked patches.
hidden_states (`tuple(tf.Tensor)`, *optional*, returned when `output_hidden_states=True` is passed or when `config.output_hidden_states=True`):
Tuple of `tf.Tensor` (one for the output of the embeddings + one for the output of each layer) of shape
`(batch_size, sequence_length, hidden_size)`. Hidden-states of the model at the output of each layer plus
the initial embedding outputs.
attentions (`tuple(tf.Tensor)`, *optional*, returned when `output_attentions=True` is passed or when `config.output_attentions=True`):
Tuple of `tf.Tensor` (one for each layer) of shape `(batch_size, num_heads, sequence_length,
sequence_length)`. Attentions weights after the attention softmax, used to compute the weighted average in
the self-attention heads.
"""
loss: tf.Tensor | None = None
logits: tf.Tensor = None
mask: tf.Tensor = None
ids_restore: tf.Tensor = None
hidden_states: Tuple[tf.Tensor] | None = None
attentions: Tuple[tf.Tensor] | None = None
def get_2d_sincos_pos_embed(embed_dim, grid_size, add_cls_token=False):
"""
Create 2D sin/cos positional embeddings.
Args:
embed_dim (`int`):
Embedding dimension.
grid_size (`int`):
The grid height and width.
add_cls_token (`bool`, *optional*, defaults to `False`):
Whether or not to add a classification (CLS) token.
Returns:
(`tf.Tensor` of shape (grid_size*grid_size, embed_dim) or (1+grid_size*grid_size, embed_dim): the position
embeddings (with or without classification token)
"""
grid_h = tf.range(grid_size, dtype=tf.float32)
grid_w = tf.range(grid_size, dtype=tf.float32)
grid = tf.meshgrid(grid_w, grid_h) # here w goes first
grid = tf.stack(grid, axis=0)
grid = tf.reshape(grid, [2, 1, grid_size, grid_size])
pos_embed = get_2d_sincos_pos_embed_from_grid(embed_dim, grid)
if add_cls_token:
pos_embed = tf.concat([tf.zeros((1, embed_dim)), pos_embed], axis=0)
return pos_embed
def get_2d_sincos_pos_embed_from_grid(embed_dim, grid):
if embed_dim % 2 != 0:
raise ValueError("embed_dim must be even")
# use half of dimensions to encode grid_h
emb_h = get_1d_sincos_pos_embed_from_grid(embed_dim // 2, grid[0]) # (H*W, D/2)
emb_w = get_1d_sincos_pos_embed_from_grid(embed_dim // 2, grid[1]) # (H*W, D/2)
emb = tf.concat([emb_h, emb_w], axis=1) # (H*W, D)
return emb
def get_1d_sincos_pos_embed_from_grid(embed_dim, pos):
"""
embed_dim: output dimension for each position pos: a list of positions to be encoded: size (M,) out: (M, D)
"""
if embed_dim % 2 != 0:
raise ValueError("embed_dim must be even")
omega = tf.range(embed_dim // 2, dtype="float32")
omega /= embed_dim / 2.0
omega = 1.0 / 10000**omega # (D/2,)
pos = tf.reshape(pos, [-1]) # (M,)
out = tf.einsum("m,d->md", pos, omega) # (M, D/2), outer product
# half of the positions get sinusoidal pattern and the rest gets
# cosine pattern and then they are concatenated
emb_sin = tf.sin(out) # (M, D/2)
emb_cos = tf.cos(out) # (M, D/2)
emb = tf.concat([emb_sin, emb_cos], axis=1) # (M, D)
return emb
class TFViTMAEEmbeddings(keras.layers.Layer):
"""
Construct the CLS token, position and patch embeddings.
"""
def __init__(self, config: ViTMAEConfig, **kwargs):
super().__init__(**kwargs)
self.patch_embeddings = TFViTMAEPatchEmbeddings(config, name="patch_embeddings")
self.num_patches = self.patch_embeddings.num_patches
self.config = config
def build(self, input_shape=None):
self.cls_token = self.add_weight(
shape=(1, 1, self.config.hidden_size),
initializer=tf.random_normal_initializer(stddev=self.config.initializer_range),
trainable=True,
name="cls_token",
)
self.position_embeddings = self.add_weight(
shape=(1, self.num_patches + 1, self.config.hidden_size),
initializer="zeros",
trainable=False, # fixed sin-cos embedding
name="position_embeddings",
)
pos_embed = get_2d_sincos_pos_embed(
self.position_embeddings.shape[-1],
int(self.patch_embeddings.num_patches**0.5),
add_cls_token=True,
)[None, ...]
self.position_embeddings.assign(pos_embed)
if self.built:
return
self.built = True
if getattr(self, "patch_embeddings", None) is not None:
with tf.name_scope(self.patch_embeddings.name):
self.patch_embeddings.build(None)
def random_masking(self, sequence: tf.Tensor, noise: tf.Tensor | None = None):
"""
Perform per-sample random masking by per-sample shuffling. Per-sample shuffling is done by argsort random
noise.
Args:
sequence (`tf.Tensor` of shape `(batch_size, sequence_length, dim)`)
noise (`tf.Tensor` of shape `(batch_size, sequence_length)`, *optional*) which is
mainly used for testing purposes to control randomness and maintain the reproducibility
"""
batch_size, seq_length, dim = shape_list(sequence)
len_keep = int(seq_length * (1 - self.config.mask_ratio))
if noise is None:
noise = tf.random.uniform(shape=(batch_size, seq_length), minval=0.0, maxval=1.0) # noise in [0, 1)
# sort noise for each sample
ids_shuffle = tf.argsort(noise, axis=1) # ascend: small is keep, large is remove
ids_restore = tf.argsort(ids_shuffle, axis=1)
# keep the first subset
ids_keep = ids_shuffle[:, :len_keep]
sequence_unmasked = tf.gather(
sequence,
axis=1,
batch_dims=1,
indices=ids_keep,
)
# generate the binary mask: 0 is keep, 1 is remove
# this hack is needed because TF's EagerTensors don't support
# assignment
mask_keep = tf.zeros((batch_size, len_keep))
mask_remove = tf.ones((batch_size, seq_length - len_keep))
mask = tf.concat([mask_keep, mask_remove], axis=-1)
# unshuffle to get the binary mask
mask = tf.gather(mask, axis=1, batch_dims=1, indices=ids_restore)
return sequence_unmasked, mask, ids_restore
def call(self, pixel_values: tf.Tensor, noise: tf.Tensor = None) -> tf.Tensor:
embeddings = self.patch_embeddings(pixel_values)
# add position embeddings w/o cls token
embeddings = embeddings + self.position_embeddings[:, 1:, :]
# masking: length -> length * config.mask_ratio
embeddings, mask, ids_restore = self.random_masking(embeddings, noise)
# append cls token
cls_token = self.cls_token + self.position_embeddings[:, :1, :]
cls_tokens = tf.tile(cls_token, (shape_list(embeddings)[0], 1, 1))
embeddings = tf.concat([cls_tokens, embeddings], axis=1)
return embeddings, mask, ids_restore
class TFViTMAEPatchEmbeddings(keras.layers.Layer):
"""
This class turns `pixel_values` of shape `(batch_size, num_channels, height, width)` into the initial
`hidden_states` (patch embeddings) of shape `(batch_size, seq_length, hidden_size)` to be consumed by a
Transformer.
"""
def __init__(self, config: ViTMAEConfig, **kwargs):
super().__init__(**kwargs)
image_size, patch_size = config.image_size, config.patch_size
num_channels, hidden_size = config.num_channels, config.hidden_size
image_size = image_size if isinstance(image_size, collections.abc.Iterable) else (image_size, image_size)
patch_size = patch_size if isinstance(patch_size, collections.abc.Iterable) else (patch_size, patch_size)
num_patches = (image_size[1] // patch_size[1]) * (image_size[0] // patch_size[0])
self.image_size = image_size
self.patch_size = patch_size
self.num_patches = num_patches
self.num_channels = num_channels
self.config = config
self.projection = keras.layers.Conv2D(
filters=hidden_size,
kernel_size=patch_size,
strides=patch_size,
padding="valid",
data_format="channels_last",
kernel_initializer="glorot_uniform", # following torch.nn.Linear
bias_initializer="zeros",
name="projection",
)
def call(self, pixel_values: tf.Tensor, training: bool = False) -> tf.Tensor:
batch_size, num_channels, height, width = shape_list(pixel_values)
if tf.executing_eagerly():
if num_channels != self.num_channels:
raise ValueError(
"Make sure that the channel dimension of the pixel values match with the one set in the"
" configuration."
)
if height != self.image_size[0] or width != self.image_size[1]:
raise ValueError(
f"Input image size ({height}*{width}) doesn't match model"
f" ({self.image_size[0]}*{self.image_size[1]})."
)
# When running on CPU, `keras.layers.Conv2D` doesn't support `NCHW` format.
# So change the input format from `NCHW` to `NHWC`.
# shape = (batch_size, in_height, in_width, in_channels=num_channels)
pixel_values = tf.transpose(pixel_values, perm=(0, 2, 3, 1))
projection = self.projection(pixel_values)
# Change the 2D spatial dimensions to a single temporal dimension.
# shape = (batch_size, num_patches, out_channels=embed_dim)
num_patches = (width // self.patch_size[1]) * (height // self.patch_size[0])
x = tf.reshape(tensor=projection, shape=(batch_size, num_patches, -1))
return x
def build(self, input_shape=None):
if self.built:
return
self.built = True
if getattr(self, "projection", None) is not None:
with tf.name_scope(self.projection.name):
self.projection.build([None, None, None, self.num_channels])
# Copied from transformers.models.vit.modeling_tf_vit.TFViTSelfAttention with ViT->ViTMAE
class TFViTMAESelfAttention(keras.layers.Layer):
def __init__(self, config: ViTMAEConfig, **kwargs):
super().__init__(**kwargs)
if config.hidden_size % config.num_attention_heads != 0:
raise ValueError(
f"The hidden size ({config.hidden_size}) is not a multiple of the number "
f"of attention heads ({config.num_attention_heads})"
)
self.num_attention_heads = config.num_attention_heads
self.attention_head_size = int(config.hidden_size / config.num_attention_heads)
self.all_head_size = self.num_attention_heads * self.attention_head_size
self.sqrt_att_head_size = math.sqrt(self.attention_head_size)
self.query = keras.layers.Dense(
units=self.all_head_size, kernel_initializer=get_initializer(config.initializer_range), name="query"
)
self.key = keras.layers.Dense(
units=self.all_head_size, kernel_initializer=get_initializer(config.initializer_range), name="key"
)
self.value = keras.layers.Dense(
units=self.all_head_size, kernel_initializer=get_initializer(config.initializer_range), name="value"
)
self.dropout = keras.layers.Dropout(rate=config.attention_probs_dropout_prob)
self.config = config
def transpose_for_scores(self, tensor: tf.Tensor, batch_size: int) -> tf.Tensor:
# Reshape from [batch_size, seq_length, all_head_size] to [batch_size, seq_length, num_attention_heads, attention_head_size]
tensor = tf.reshape(tensor=tensor, shape=(batch_size, -1, self.num_attention_heads, self.attention_head_size))
# Transpose the tensor from [batch_size, seq_length, num_attention_heads, attention_head_size] to [batch_size, num_attention_heads, seq_length, attention_head_size]
return tf.transpose(tensor, perm=[0, 2, 1, 3])
def call(
self,
hidden_states: tf.Tensor,
head_mask: tf.Tensor,
output_attentions: bool,
training: bool = False,
) -> Tuple[tf.Tensor]:
batch_size = shape_list(hidden_states)[0]
mixed_query_layer = self.query(inputs=hidden_states)
mixed_key_layer = self.key(inputs=hidden_states)
mixed_value_layer = self.value(inputs=hidden_states)
query_layer = self.transpose_for_scores(mixed_query_layer, batch_size)
key_layer = self.transpose_for_scores(mixed_key_layer, batch_size)
value_layer = self.transpose_for_scores(mixed_value_layer, batch_size)
# Take the dot product between "query" and "key" to get the raw attention scores.
# (batch size, num_heads, seq_len_q, seq_len_k)
attention_scores = tf.matmul(query_layer, key_layer, transpose_b=True)
dk = tf.cast(self.sqrt_att_head_size, dtype=attention_scores.dtype)
attention_scores = tf.divide(attention_scores, dk)
# Normalize the attention scores to probabilities.
attention_probs = stable_softmax(logits=attention_scores, axis=-1)
# This is actually dropping out entire tokens to attend to, which might
# seem a bit unusual, but is taken from the original Transformer paper.
attention_probs = self.dropout(inputs=attention_probs, training=training)
# Mask heads if we want to
if head_mask is not None:
attention_probs = tf.multiply(attention_probs, head_mask)
attention_output = tf.matmul(attention_probs, value_layer)
attention_output = tf.transpose(attention_output, perm=[0, 2, 1, 3])
# (batch_size, seq_len_q, all_head_size)
attention_output = tf.reshape(tensor=attention_output, shape=(batch_size, -1, self.all_head_size))
outputs = (attention_output, attention_probs) if output_attentions else (attention_output,)
return outputs
def build(self, input_shape=None):
if self.built:
return
self.built = True
if getattr(self, "query", None) is not None:
with tf.name_scope(self.query.name):
self.query.build([None, None, self.config.hidden_size])
if getattr(self, "key", None) is not None:
with tf.name_scope(self.key.name):
self.key.build([None, None, self.config.hidden_size])
if getattr(self, "value", None) is not None:
with tf.name_scope(self.value.name):
self.value.build([None, None, self.config.hidden_size])
# Copied from transformers.models.vit.modeling_tf_vit.TFViTSelfOutput with ViT->ViTMAE
class TFViTMAESelfOutput(keras.layers.Layer):
"""
The residual connection is defined in TFViTMAELayer instead of here (as is the case with other models), due to the
layernorm applied before each block.
"""
def __init__(self, config: ViTMAEConfig, **kwargs):
super().__init__(**kwargs)
self.dense = keras.layers.Dense(
units=config.hidden_size, kernel_initializer=get_initializer(config.initializer_range), name="dense"
)
self.dropout = keras.layers.Dropout(rate=config.hidden_dropout_prob)
self.config = config
def call(self, hidden_states: tf.Tensor, input_tensor: tf.Tensor, training: bool = False) -> tf.Tensor:
hidden_states = self.dense(inputs=hidden_states)
hidden_states = self.dropout(inputs=hidden_states, training=training)
return hidden_states
def build(self, input_shape=None):
if self.built:
return
self.built = True
if getattr(self, "dense", None) is not None:
with tf.name_scope(self.dense.name):
self.dense.build([None, None, self.config.hidden_size])
# Copied from transformers.models.vit.modeling_tf_vit.TFViTAttention with ViT->ViTMAE
class TFViTMAEAttention(keras.layers.Layer):
def __init__(self, config: ViTMAEConfig, **kwargs):
super().__init__(**kwargs)
self.self_attention = TFViTMAESelfAttention(config, name="attention")
self.dense_output = TFViTMAESelfOutput(config, name="output")
def prune_heads(self, heads):
raise NotImplementedError
def call(
self,
input_tensor: tf.Tensor,
head_mask: tf.Tensor,
output_attentions: bool,
training: bool = False,
) -> Tuple[tf.Tensor]:
self_outputs = self.self_attention(
hidden_states=input_tensor, head_mask=head_mask, output_attentions=output_attentions, training=training
)
attention_output = self.dense_output(
hidden_states=self_outputs[0], input_tensor=input_tensor, training=training
)
outputs = (attention_output,) + self_outputs[1:] # add attentions if we output them
return outputs
def build(self, input_shape=None):
if self.built:
return
self.built = True
if getattr(self, "self_attention", None) is not None:
with tf.name_scope(self.self_attention.name):
self.self_attention.build(None)
if getattr(self, "dense_output", None) is not None:
with tf.name_scope(self.dense_output.name):
self.dense_output.build(None)
# Copied from transformers.models.vit.modeling_tf_vit.TFViTIntermediate with ViT->ViTMAE
class TFViTMAEIntermediate(keras.layers.Layer):
def __init__(self, config: ViTMAEConfig, **kwargs):
super().__init__(**kwargs)
self.dense = keras.layers.Dense(
units=config.intermediate_size, kernel_initializer=get_initializer(config.initializer_range), name="dense"
)
if isinstance(config.hidden_act, str):
self.intermediate_act_fn = get_tf_activation(config.hidden_act)
else:
self.intermediate_act_fn = config.hidden_act
self.config = config
def call(self, hidden_states: tf.Tensor) -> tf.Tensor:
hidden_states = self.dense(inputs=hidden_states)
hidden_states = self.intermediate_act_fn(hidden_states)
return hidden_states
def build(self, input_shape=None):
if self.built:
return
self.built = True
if getattr(self, "dense", None) is not None:
with tf.name_scope(self.dense.name):
self.dense.build([None, None, self.config.hidden_size])
# Copied from transformers.models.vit.modeling_tf_vit.TFViTOutput with ViT->ViTMAE
class TFViTMAEOutput(keras.layers.Layer):
def __init__(self, config: ViTMAEConfig, **kwargs):
super().__init__(**kwargs)
self.dense = keras.layers.Dense(
units=config.hidden_size, kernel_initializer=get_initializer(config.initializer_range), name="dense"
)
self.dropout = keras.layers.Dropout(rate=config.hidden_dropout_prob)
self.config = config
def call(self, hidden_states: tf.Tensor, input_tensor: tf.Tensor, training: bool = False) -> tf.Tensor:
hidden_states = self.dense(inputs=hidden_states)
hidden_states = self.dropout(inputs=hidden_states, training=training)
hidden_states = hidden_states + input_tensor
return hidden_states
def build(self, input_shape=None):
if self.built:
return
self.built = True
if getattr(self, "dense", None) is not None:
with tf.name_scope(self.dense.name):
self.dense.build([None, None, self.config.intermediate_size])
# Copied from transformers.models.vit.modeling_tf_vit.TFViTLayer with ViT->ViTMAE
class TFViTMAELayer(keras.layers.Layer):
"""This corresponds to the Block class in the timm implementation."""
def __init__(self, config: ViTMAEConfig, **kwargs):
super().__init__(**kwargs)
self.attention = TFViTMAEAttention(config, name="attention")
self.intermediate = TFViTMAEIntermediate(config, name="intermediate")
self.vit_output = TFViTMAEOutput(config, name="output")
self.layernorm_before = keras.layers.LayerNormalization(epsilon=config.layer_norm_eps, name="layernorm_before")
self.layernorm_after = keras.layers.LayerNormalization(epsilon=config.layer_norm_eps, name="layernorm_after")
self.config = config
def call(
self,
hidden_states: tf.Tensor,
head_mask: tf.Tensor,
output_attentions: bool,
training: bool = False,
) -> Tuple[tf.Tensor]:
attention_outputs = self.attention(
# in ViTMAE, layernorm is applied before self-attention
input_tensor=self.layernorm_before(inputs=hidden_states),
head_mask=head_mask,
output_attentions=output_attentions,
training=training,
)
attention_output = attention_outputs[0]
# first residual connection
hidden_states = attention_output + hidden_states
# in ViTMAE, layernorm is also applied after self-attention
layer_output = self.layernorm_after(inputs=hidden_states)
intermediate_output = self.intermediate(hidden_states=layer_output)
# second residual connection is done here
layer_output = self.vit_output(
hidden_states=intermediate_output, input_tensor=hidden_states, training=training
)
outputs = (layer_output,) + attention_outputs[1:] # add attentions if we output them
return outputs
def build(self, input_shape=None):
if self.built:
return
self.built = True
if getattr(self, "attention", None) is not None:
with tf.name_scope(self.attention.name):
self.attention.build(None)
if getattr(self, "intermediate", None) is not None:
with tf.name_scope(self.intermediate.name):
self.intermediate.build(None)
if getattr(self, "vit_output", None) is not None:
with tf.name_scope(self.vit_output.name):
self.vit_output.build(None)
if getattr(self, "layernorm_before", None) is not None:
with tf.name_scope(self.layernorm_before.name):
self.layernorm_before.build([None, None, self.config.hidden_size])
if getattr(self, "layernorm_after", None) is not None:
with tf.name_scope(self.layernorm_after.name):
self.layernorm_after.build([None, None, self.config.hidden_size])
# Copied from transformers.models.vit.modeling_tf_vit.TFViTEncoder with ViT->ViTMAE
class TFViTMAEEncoder(keras.layers.Layer):
def __init__(self, config: ViTMAEConfig, **kwargs):
super().__init__(**kwargs)
self.layer = [TFViTMAELayer(config, name=f"layer_._{i}") for i in range(config.num_hidden_layers)]
def call(
self,
hidden_states: tf.Tensor,
head_mask: tf.Tensor,
output_attentions: bool,
output_hidden_states: bool,
return_dict: bool,
training: bool = False,
) -> Union[TFBaseModelOutput, Tuple[tf.Tensor]]:
all_hidden_states = () if output_hidden_states else None
all_attentions = () if output_attentions else None
for i, layer_module in enumerate(self.layer):
if output_hidden_states:
all_hidden_states = all_hidden_states + (hidden_states,)
layer_outputs = layer_module(
hidden_states=hidden_states,
head_mask=head_mask[i],
output_attentions=output_attentions,
training=training,
)
hidden_states = layer_outputs[0]
if output_attentions:
all_attentions = all_attentions + (layer_outputs[1],)
# Add last layer
if output_hidden_states:
all_hidden_states = all_hidden_states + (hidden_states,)
if not return_dict:
return tuple(v for v in [hidden_states, all_hidden_states, all_attentions] if v is not None)
return TFBaseModelOutput(
last_hidden_state=hidden_states, hidden_states=all_hidden_states, attentions=all_attentions
)
def build(self, input_shape=None):
if self.built:
return
self.built = True
if getattr(self, "layer", None) is not None:
for layer in self.layer:
with tf.name_scope(layer.name):
layer.build(None)
@keras_serializable
class TFViTMAEMainLayer(keras.layers.Layer):
config_class = ViTMAEConfig
def __init__(self, config: ViTMAEConfig, **kwargs):
super().__init__(**kwargs)
self.config = config
self.embeddings = TFViTMAEEmbeddings(config, name="embeddings")
self.encoder = TFViTMAEEncoder(config, name="encoder")
self.layernorm = keras.layers.LayerNormalization(epsilon=config.layer_norm_eps, name="layernorm")
def get_input_embeddings(self) -> keras.layers.Layer:
return self.embeddings.patch_embeddings
def _prune_heads(self, heads_to_prune):
"""
Prunes heads of the model. heads_to_prune: dict of {layer_num: list of heads to prune in this layer} See base
class PreTrainedModel
"""
raise NotImplementedError
@unpack_inputs
def call(
self,
pixel_values: TFModelInputType | None = None,
noise: tf.Tensor = None,
head_mask: np.ndarray | tf.Tensor | None = None,
output_attentions: Optional[bool] = None,
output_hidden_states: Optional[bool] = None,
return_dict: Optional[bool] = None,
training: bool = False,
) -> Union[TFViTMAEModelOutput, Tuple[tf.Tensor]]:
embedding_output, mask, ids_restore = self.embeddings(
pixel_values=pixel_values, training=training, noise=noise
)
# Prepare head mask if needed
# 1.0 in head_mask indicate we keep the head
# attention_probs has shape bsz x n_heads x N x N
# input head_mask has shape [num_heads] or [num_hidden_layers x num_heads]
# and head_mask is converted to shape [num_hidden_layers x batch x num_heads x seq_length x seq_length]
if head_mask is not None:
raise NotImplementedError
else:
head_mask = [None] * self.config.num_hidden_layers
encoder_outputs = self.encoder(
embedding_output,
head_mask=head_mask,
output_attentions=output_attentions,
output_hidden_states=output_hidden_states,
return_dict=return_dict,
training=training,
)
sequence_output = encoder_outputs[0]
sequence_output = self.layernorm(inputs=sequence_output)
if not return_dict:
return (sequence_output, mask, ids_restore) + encoder_outputs[1:]
return TFViTMAEModelOutput(
last_hidden_state=sequence_output,
mask=mask,
ids_restore=ids_restore,
hidden_states=encoder_outputs.hidden_states,
attentions=encoder_outputs.attentions,
)
def build(self, input_shape=None):
if self.built:
return
self.built = True
if getattr(self, "embeddings", None) is not None:
with tf.name_scope(self.embeddings.name):
self.embeddings.build(None)
if getattr(self, "encoder", None) is not None:
with tf.name_scope(self.encoder.name):
self.encoder.build(None)
if getattr(self, "layernorm", None) is not None:
with tf.name_scope(self.layernorm.name):
self.layernorm.build([None, None, self.config.hidden_size])
class TFViTMAEPreTrainedModel(TFPreTrainedModel):
"""
An abstract class to handle weights initialization and a simple interface for downloading and loading pretrained
models.
"""
config_class = ViTMAEConfig
base_model_prefix = "vit"
main_input_name = "pixel_values"
VIT_MAE_START_DOCSTRING = r"""
This model inherits from [`TFPreTrainedModel`]. Check the superclass documentation for the generic methods the
library implements for all its model (such as downloading or saving, resizing the input embeddings, pruning heads
etc.)
This model is also a [keras.Model](https://www.tensorflow.org/api_docs/python/tf/keras/Model) subclass. Use it
as a regular TF 2.0 Keras Model and refer to the TF 2.0 documentation for all matter related to general usage and
behavior.
<Tip>
TensorFlow models and layers in `transformers` accept two formats as input:
- having all inputs as keyword arguments (like PyTorch models), or
- having all inputs as a list, tuple or dict in the first positional argument.
The reason the second format is supported is that Keras methods prefer this format when passing inputs to models
and layers. Because of this support, when using methods like `model.fit()` things should "just work" for you - just
pass your inputs and labels in any format that `model.fit()` supports! If, however, you want to use the second
format outside of Keras methods like `fit()` and `predict()`, such as when creating your own layers or models with
the Keras `Functional` API, there are three possibilities you can use to gather all the input Tensors in the first
positional argument:
- a single Tensor with `pixel_values` only and nothing else: `model(pixel_values)`
- a list of varying length with one or several input Tensors IN THE ORDER given in the docstring:
`model([pixel_values, attention_mask])` or `model([pixel_values, attention_mask, token_type_ids])`
- a dictionary with one or several input Tensors associated to the input names given in the docstring:
`model({"pixel_values": pixel_values, "token_type_ids": token_type_ids})`
Note that when creating models and layers with
[subclassing](https://keras.io/guides/making_new_layers_and_models_via_subclassing/) then you don't need to worry
about any of this, as you can just pass inputs like you would to any other Python function!
</Tip>
Args:
config ([`ViTMAEConfig`]): Model configuration class with all the parameters of the model.
Initializing with a config file does not load the weights associated with the model, only the
configuration. Check out the [`~TFPreTrainedModel.from_pretrained`] method to load the model weights.
"""
VIT_MAE_INPUTS_DOCSTRING = r"""
Args:
pixel_values (`np.ndarray`, `tf.Tensor`, `List[tf.Tensor]` ``Dict[str, tf.Tensor]` or `Dict[str, np.ndarray]` and each example must have the shape `(batch_size, num_channels, height, width)`):
Pixel values. Pixel values can be obtained using [`AutoImageProcessor`]. See [`ViTImageProcessor.__call__`]
for details.
head_mask (`np.ndarray` or `tf.Tensor` of shape `(num_heads,)` or `(num_layers, num_heads)`, *optional*):
Mask to nullify selected heads of the self-attention modules. Mask values selected in `[0, 1]`:
- 1 indicates the head is **not masked**,
- 0 indicates the head is **masked**.
output_attentions (`bool`, *optional*):
Whether or not to return the attentions tensors of all attention layers. See `attentions` under returned
tensors for more detail. This argument can be used only in eager mode, in graph mode the value in the
config will be used instead.
output_hidden_states (`bool`, *optional*):
Whether or not to return the hidden states of all layers. See `hidden_states` under returned tensors for
more detail. This argument can be used only in eager mode, in graph mode the value in the config will be
used instead.
return_dict (`bool`, *optional*):
Whether or not to return a [`~file_utils.ModelOutput`] instead of a plain tuple. This argument can be used
in eager mode, in graph mode the value will always be set to True.
training (`bool`, *optional*, defaults to `False``):
Whether or not to use the model in training mode (some modules like dropout modules have different
behaviors between training and evaluation).
"""
@add_start_docstrings(
"The bare ViTMAE Model transformer outputting raw hidden-states without any specific head on top.",
VIT_MAE_START_DOCSTRING,
)
class TFViTMAEModel(TFViTMAEPreTrainedModel):
def __init__(self, config: ViTMAEConfig, *inputs, **kwargs):
super().__init__(config, *inputs, **kwargs)
self.vit = TFViTMAEMainLayer(config, name="vit")
def get_input_embeddings(self):
return self.vit.get_input_embeddings()
@unpack_inputs
@add_start_docstrings_to_model_forward(VIT_MAE_INPUTS_DOCSTRING)
@replace_return_docstrings(output_type=TFViTMAEModelOutput, config_class=_CONFIG_FOR_DOC)
def call(
self,
pixel_values: TFModelInputType | None = None,
noise: tf.Tensor = None,
head_mask: np.ndarray | tf.Tensor | None = None,
output_attentions: Optional[bool] = None,
output_hidden_states: Optional[bool] = None,
return_dict: Optional[bool] = None,
training: bool = False,
) -> Union[TFViTMAEModelOutput, Tuple[tf.Tensor]]:
r"""
Returns:
Examples:
```python
>>> from transformers import AutoImageProcessor, TFViTMAEModel
>>> from PIL import Image
>>> import requests
>>> url = "http://images.cocodataset.org/val2017/000000039769.jpg"
>>> image = Image.open(requests.get(url, stream=True).raw)
>>> image_processor = AutoImageProcessor.from_pretrained("facebook/vit-mae-base")
>>> model = TFViTMAEModel.from_pretrained("facebook/vit-mae-base")
>>> inputs = image_processor(images=image, return_tensors="tf")
>>> outputs = model(**inputs)
>>> last_hidden_states = outputs.last_hidden_state
```"""
outputs = self.vit(
pixel_values=pixel_values,
noise=noise,
head_mask=head_mask,
output_attentions=output_attentions,
output_hidden_states=output_hidden_states,
return_dict=return_dict,
training=training,
)
return outputs
def build(self, input_shape=None):
if self.built:
return
self.built = True
if getattr(self, "vit", None) is not None:
with tf.name_scope(self.vit.name):
self.vit.build(None)
class TFViTMAEDecoder(keras.layers.Layer):
def __init__(self, config, num_patches, **kwargs):
super().__init__(**kwargs)
self.decoder_embed = keras.layers.Dense(config.decoder_hidden_size, name="decoder_embed")
decoder_config = deepcopy(config)
decoder_config.hidden_size = config.decoder_hidden_size
decoder_config.num_hidden_layers = config.decoder_num_hidden_layers
decoder_config.num_attention_heads = config.decoder_num_attention_heads
decoder_config.intermediate_size = config.decoder_intermediate_size
self.decoder_layers = [
TFViTMAELayer(decoder_config, name=f"decoder_layers.{j}") for j in range(config.decoder_num_hidden_layers)
]
self.decoder_norm = keras.layers.LayerNormalization(epsilon=config.layer_norm_eps, name="decoder_norm")
self.decoder_pred = keras.layers.Dense(
config.patch_size**2 * config.num_channels,
kernel_initializer=get_initializer(config.initializer_range),
name="decoder_pred",
) # encoder to decoder
self.config = config
self.num_patches = num_patches
def build(self, input_shape=None):
self.mask_token = self.add_weight(
shape=(1, 1, self.config.decoder_hidden_size),
initializer=tf.random_normal_initializer(stddev=self.config.initializer_range),
trainable=True,
name="mask_token",
)
self.decoder_pos_embed = self.add_weight(
shape=(1, self.num_patches + 1, self.config.decoder_hidden_size),
initializer="zeros",
trainable=False,
name="decoder_pos_embed",
)
decoder_pos_embed = get_2d_sincos_pos_embed(
self.decoder_pos_embed.shape[-1],
int(self.num_patches**0.5),
add_cls_token=True,
)[None, ...]
self.decoder_pos_embed.assign(decoder_pos_embed)
if self.built:
return
self.built = True
if getattr(self, "decoder_embed", None) is not None:
with tf.name_scope(self.decoder_embed.name):
self.decoder_embed.build([None, None, self.config.hidden_size])
if getattr(self, "decoder_norm", None) is not None:
with tf.name_scope(self.decoder_norm.name):
self.decoder_norm.build([None, None, self.config.decoder_hidden_size])
if getattr(self, "decoder_pred", None) is not None:
with tf.name_scope(self.decoder_pred.name):
self.decoder_pred.build([None, None, self.config.decoder_hidden_size])
if getattr(self, "decoder_layers", None) is not None:
for layer in self.decoder_layers:
with tf.name_scope(layer.name):
layer.build(None)
def call(
self,
hidden_states,
ids_restore,
output_attentions=False,
output_hidden_states=False,
return_dict=True,
):
# embed tokens
x = self.decoder_embed(hidden_states)
# append mask tokens to sequence
mask_tokens = tf.tile(
self.mask_token,
(shape_list(x)[0], shape_list(ids_restore)[1] + 1 - shape_list(x)[1], 1),
)
x_ = tf.concat([x[:, 1:, :], mask_tokens], axis=1) # no cls token
x_ = tf.gather(x_, axis=1, batch_dims=1, indices=ids_restore) # unshuffle
x = tf.concat([x[:, :1, :], x_], axis=1) # append cls token
# add pos embed
hidden_states = x + self.decoder_pos_embed
# apply Transformer layers (blocks)
all_hidden_states = () if output_hidden_states else None
all_self_attentions = () if output_attentions else None
for i, layer_module in enumerate(self.decoder_layers):
if output_hidden_states:
all_hidden_states = all_hidden_states + (hidden_states,)
layer_outputs = layer_module(
hidden_states,
head_mask=None,
output_attentions=output_attentions,
)
hidden_states = layer_outputs[0]
if output_attentions:
all_self_attentions = all_self_attentions + (layer_outputs[1],)
if output_hidden_states:
all_hidden_states = all_hidden_states + (hidden_states,)
hidden_states = self.decoder_norm(hidden_states)
# predictor projection
logits = self.decoder_pred(hidden_states)
# remove cls token
logits = logits[:, 1:, :]
if not return_dict:
return tuple(v for v in [logits, all_hidden_states, all_self_attentions] if v is not None)
return TFViTMAEDecoderOutput(logits=logits, hidden_states=all_hidden_states, attentions=all_self_attentions)
@add_start_docstrings(
"The ViTMAE Model transformer with the decoder on top for self-supervised pre-training.",
VIT_MAE_START_DOCSTRING,
)
class TFViTMAEForPreTraining(TFViTMAEPreTrainedModel):
def __init__(self, config):
super().__init__(config)
self.config = config
self.vit = TFViTMAEMainLayer(config, name="vit")
self.decoder = TFViTMAEDecoder(
config,
num_patches=self.vit.embeddings.num_patches,
name="decoder",
)
def get_input_embeddings(self):
return self.vit.get_input_embeddings()
def _prune_heads(self, heads_to_prune):
raise NotImplementedError
def patchify(self, pixel_values):
"""
Args:
pixel_values (`tf.Tensor` of shape `(batch_size, height, width, num_channels)` or `(batch_size, num_channels, height, width)`):
Pixel values.
Returns:
`tf.Tensor` of shape `(batch_size, num_patches, patch_size**2 * num_channels)`:
Patchified pixel values.
"""
patch_size, num_channels = self.config.patch_size, self.config.num_channels
# make sure channels are last
if shape_list(pixel_values)[1] == num_channels:
pixel_values = tf.transpose(pixel_values, perm=(0, 2, 3, 1))
# sanity checks
tf.debugging.assert_equal(
shape_list(pixel_values)[1],
shape_list(pixel_values)[2],
message="Make sure the pixel values have a squared size",
)
tf.debugging.assert_equal(
shape_list(pixel_values)[1] % patch_size,
0,
message="Make sure the pixel values have a size that is divisible by the patch size",
)
tf.debugging.assert_equal(
shape_list(pixel_values)[3],
num_channels,
message=(
"Make sure the number of channels of the pixel values is equal to the one set in the configuration"
),
)
# patchify
batch_size = shape_list(pixel_values)[0]
num_patches_one_direction = shape_list(pixel_values)[2] // patch_size
patchified_pixel_values = tf.reshape(
pixel_values,
(batch_size, num_patches_one_direction, patch_size, num_patches_one_direction, patch_size, num_channels),
)
patchified_pixel_values = tf.einsum("nhpwqc->nhwpqc", patchified_pixel_values)
patchified_pixel_values = tf.reshape(
patchified_pixel_values,
(batch_size, num_patches_one_direction * num_patches_one_direction, patch_size**2 * num_channels),
)
return patchified_pixel_values
def unpatchify(self, patchified_pixel_values):
"""
Args:
patchified_pixel_values (`tf.Tensor` of shape `(batch_size, num_patches, patch_size**2 * num_channels)`:
Patchified pixel values.
Returns:
`tf.Tensor` of shape `(batch_size, height, width, num_channels)`:
Pixel values.
"""
patch_size, num_channels = self.config.patch_size, self.config.num_channels
num_patches_one_direction = int(shape_list(patchified_pixel_values)[1] ** 0.5)
# sanity check
tf.debugging.assert_equal(
num_patches_one_direction * num_patches_one_direction,
shape_list(patchified_pixel_values)[1],
message="Make sure that the number of patches can be squared",
)
# unpatchify
batch_size = shape_list(patchified_pixel_values)[0]
patchified_pixel_values = tf.reshape(
patchified_pixel_values,
(batch_size, num_patches_one_direction, num_patches_one_direction, patch_size, patch_size, num_channels),
)
patchified_pixel_values = tf.einsum("nhwpqc->nhpwqc", patchified_pixel_values)
pixel_values = tf.reshape(
patchified_pixel_values,
(batch_size, num_patches_one_direction * patch_size, num_patches_one_direction * patch_size, num_channels),
)
return pixel_values
def forward_loss(self, pixel_values, pred, mask):
"""
Args:
pixel_values (`tf.Tensor` of shape `(batch_size, height, width, num_channels)`):
Pixel values.
pred (`tf.Tensor` of shape `(batch_size, num_patches, patch_size**2 * num_channels)`:
Predicted pixel values.
mask (`tf.Tensor` of shape `(batch_size, sequence_length)`):
Tensor indicating which patches are masked (1) and which are not (0).
Returns:
`tf.Tensor`: Pixel reconstruction loss.
"""
target = self.patchify(pixel_values)
if self.config.norm_pix_loss:
mean = tf.reduce_mean(target, axis=-1, keepdims=True)
var = tf.math.reduce_variance(target, axis=-1, keepdims=True)
target = (target - mean) / (var + 1.0e-6) ** 0.5
loss = (pred - target) ** 2
loss = tf.reduce_mean(loss, axis=-1) # [batch_size, num_patches], mean loss per patch
loss = tf.reduce_sum(loss * mask) / tf.reduce_sum(mask) # mean loss on removed patches
loss = tf.reshape(loss, (1,))
return loss
@unpack_inputs
@add_start_docstrings_to_model_forward(VIT_MAE_INPUTS_DOCSTRING)
@replace_return_docstrings(output_type=TFViTMAEForPreTrainingOutput, config_class=_CONFIG_FOR_DOC)
def call(
self,
pixel_values: TFModelInputType | None = None,
noise: tf.Tensor = None,
head_mask: np.ndarray | tf.Tensor | None = None,
output_attentions: Optional[bool] = None,
output_hidden_states: Optional[bool] = None,
return_dict: Optional[bool] = None,
training: bool = False,
) -> Union[TFViTMAEForPreTrainingOutput, Tuple[tf.Tensor]]:
r"""
Returns:
Examples:
```python
>>> from transformers import AutoImageProcessor, TFViTMAEForPreTraining
>>> from PIL import Image
>>> import requests
>>> url = "http://images.cocodataset.org/val2017/000000039769.jpg"
>>> image = Image.open(requests.get(url, stream=True).raw)
>>> image_processor = AutoImageProcessor.from_pretrained("facebook/vit-mae-base")
>>> model = TFViTMAEForPreTraining.from_pretrained("facebook/vit-mae-base")
>>> inputs = image_processor(images=image, return_tensors="pt")
>>> outputs = model(**inputs)
>>> loss = outputs.loss
>>> mask = outputs.mask
>>> ids_restore = outputs.ids_restore
```"""
return_dict = return_dict if return_dict is not None else self.config.use_return_dict
outputs = self.vit(
pixel_values=pixel_values,
noise=noise,
head_mask=head_mask,
output_attentions=output_attentions,
output_hidden_states=output_hidden_states,
return_dict=return_dict,
training=training,
)
latent = outputs.last_hidden_state
ids_restore = outputs.ids_restore
mask = outputs.mask
decoder_outputs = self.decoder(latent, ids_restore) # [batch_size, num_patches, patch_size**2*3]
logits = decoder_outputs.logits
loss = self.forward_loss(pixel_values, logits, mask)
if not return_dict:
output = (logits, mask, ids_restore) + outputs[2:]
return ((loss,) + output) if loss is not None else output
return TFViTMAEForPreTrainingOutput(
loss=loss,
logits=logits,
mask=mask,
ids_restore=ids_restore,
hidden_states=outputs.hidden_states,
attentions=outputs.attentions,
)
def build(self, input_shape=None):
if self.built:
return
self.built = True
if getattr(self, "vit", None) is not None:
with tf.name_scope(self.vit.name):
self.vit.build(None)
if getattr(self, "decoder", None) is not None:
with tf.name_scope(self.decoder.name):
self.decoder.build(None)
| transformers/src/transformers/models/vit_mae/modeling_tf_vit_mae.py/0 | {
"file_path": "transformers/src/transformers/models/vit_mae/modeling_tf_vit_mae.py",
"repo_id": "transformers",
"token_count": 22784
} | 329 |
# coding=utf-8
# Copyright 2023 The HuggingFace Inc. team. All rights reserved.
#
# Licensed under the Apache License, Version 2.0 (the "License");
# you may not use this file except in compliance with the License.
# You may obtain a copy of the License at
#
# http://www.apache.org/licenses/LICENSE-2.0
#
# Unless required by applicable law or agreed to in writing, software
# distributed under the License is distributed on an "AS IS" BASIS,
# WITHOUT WARRANTIES OR CONDITIONS OF ANY KIND, either express or implied.
# See the License for the specific language governing permissions and
# limitations under the License.
"""Convert VITS checkpoint."""
import argparse
import json
import tempfile
import torch
from huggingface_hub import hf_hub_download
from transformers import VitsConfig, VitsModel, VitsTokenizer, logging
logging.set_verbosity_info()
logger = logging.get_logger("transformers.models.vits")
MAPPING_TEXT_ENCODER = {
"enc_p.emb": "text_encoder.embed_tokens",
"enc_p.encoder.attn_layers.*.conv_k": "text_encoder.encoder.layers.*.attention.k_proj",
"enc_p.encoder.attn_layers.*.conv_v": "text_encoder.encoder.layers.*.attention.v_proj",
"enc_p.encoder.attn_layers.*.conv_q": "text_encoder.encoder.layers.*.attention.q_proj",
"enc_p.encoder.attn_layers.*.conv_o": "text_encoder.encoder.layers.*.attention.out_proj",
"enc_p.encoder.attn_layers.*.emb_rel_k": "text_encoder.encoder.layers.*.attention.emb_rel_k",
"enc_p.encoder.attn_layers.*.emb_rel_v": "text_encoder.encoder.layers.*.attention.emb_rel_v",
"enc_p.encoder.norm_layers_1.*.gamma": "text_encoder.encoder.layers.*.layer_norm.weight",
"enc_p.encoder.norm_layers_1.*.beta": "text_encoder.encoder.layers.*.layer_norm.bias",
"enc_p.encoder.ffn_layers.*.conv_1": "text_encoder.encoder.layers.*.feed_forward.conv_1",
"enc_p.encoder.ffn_layers.*.conv_2": "text_encoder.encoder.layers.*.feed_forward.conv_2",
"enc_p.encoder.norm_layers_2.*.gamma": "text_encoder.encoder.layers.*.final_layer_norm.weight",
"enc_p.encoder.norm_layers_2.*.beta": "text_encoder.encoder.layers.*.final_layer_norm.bias",
"enc_p.proj": "text_encoder.project",
}
MAPPING_STOCHASTIC_DURATION_PREDICTOR = {
"dp.pre": "duration_predictor.conv_pre",
"dp.proj": "duration_predictor.conv_proj",
"dp.convs.convs_sep.*": "duration_predictor.conv_dds.convs_dilated.*",
"dp.convs.convs_1x1.*": "duration_predictor.conv_dds.convs_pointwise.*",
"dp.convs.norms_1.*.gamma": "duration_predictor.conv_dds.norms_1.*.weight",
"dp.convs.norms_1.*.beta": "duration_predictor.conv_dds.norms_1.*.bias",
"dp.convs.norms_2.*.gamma": "duration_predictor.conv_dds.norms_2.*.weight",
"dp.convs.norms_2.*.beta": "duration_predictor.conv_dds.norms_2.*.bias",
"dp.flows.0.logs": "duration_predictor.flows.0.log_scale",
"dp.flows.0.m": "duration_predictor.flows.0.translate",
"dp.flows.*.pre": "duration_predictor.flows.*.conv_pre",
"dp.flows.*.proj": "duration_predictor.flows.*.conv_proj",
"dp.flows.*.convs.convs_1x1.0": "duration_predictor.flows.*.conv_dds.convs_pointwise.0",
"dp.flows.*.convs.convs_1x1.1": "duration_predictor.flows.*.conv_dds.convs_pointwise.1",
"dp.flows.*.convs.convs_1x1.2": "duration_predictor.flows.*.conv_dds.convs_pointwise.2",
"dp.flows.*.convs.convs_sep.0": "duration_predictor.flows.*.conv_dds.convs_dilated.0",
"dp.flows.*.convs.convs_sep.1": "duration_predictor.flows.*.conv_dds.convs_dilated.1",
"dp.flows.*.convs.convs_sep.2": "duration_predictor.flows.*.conv_dds.convs_dilated.2",
"dp.flows.*.convs.norms_1.0.gamma": "duration_predictor.flows.*.conv_dds.norms_1.0.weight",
"dp.flows.*.convs.norms_1.0.beta": "duration_predictor.flows.*.conv_dds.norms_1.0.bias",
"dp.flows.*.convs.norms_1.1.gamma": "duration_predictor.flows.*.conv_dds.norms_1.1.weight",
"dp.flows.*.convs.norms_1.1.beta": "duration_predictor.flows.*.conv_dds.norms_1.1.bias",
"dp.flows.*.convs.norms_1.2.gamma": "duration_predictor.flows.*.conv_dds.norms_1.2.weight",
"dp.flows.*.convs.norms_1.2.beta": "duration_predictor.flows.*.conv_dds.norms_1.2.bias",
"dp.flows.*.convs.norms_2.0.gamma": "duration_predictor.flows.*.conv_dds.norms_2.0.weight",
"dp.flows.*.convs.norms_2.0.beta": "duration_predictor.flows.*.conv_dds.norms_2.0.bias",
"dp.flows.*.convs.norms_2.1.gamma": "duration_predictor.flows.*.conv_dds.norms_2.1.weight",
"dp.flows.*.convs.norms_2.1.beta": "duration_predictor.flows.*.conv_dds.norms_2.1.bias",
"dp.flows.*.convs.norms_2.2.gamma": "duration_predictor.flows.*.conv_dds.norms_2.2.weight",
"dp.flows.*.convs.norms_2.2.beta": "duration_predictor.flows.*.conv_dds.norms_2.2.bias",
"dp.post_pre": "duration_predictor.post_conv_pre",
"dp.post_proj": "duration_predictor.post_conv_proj",
"dp.post_convs.convs_sep.*": "duration_predictor.post_conv_dds.convs_dilated.*",
"dp.post_convs.convs_1x1.*": "duration_predictor.post_conv_dds.convs_pointwise.*",
"dp.post_convs.norms_1.*.gamma": "duration_predictor.post_conv_dds.norms_1.*.weight",
"dp.post_convs.norms_1.*.beta": "duration_predictor.post_conv_dds.norms_1.*.bias",
"dp.post_convs.norms_2.*.gamma": "duration_predictor.post_conv_dds.norms_2.*.weight",
"dp.post_convs.norms_2.*.beta": "duration_predictor.post_conv_dds.norms_2.*.bias",
"dp.post_flows.0.logs": "duration_predictor.post_flows.0.log_scale",
"dp.post_flows.0.m": "duration_predictor.post_flows.0.translate",
"dp.post_flows.*.pre": "duration_predictor.post_flows.*.conv_pre",
"dp.post_flows.*.proj": "duration_predictor.post_flows.*.conv_proj",
"dp.post_flows.*.convs.convs_1x1.0": "duration_predictor.post_flows.*.conv_dds.convs_pointwise.0",
"dp.post_flows.*.convs.convs_1x1.1": "duration_predictor.post_flows.*.conv_dds.convs_pointwise.1",
"dp.post_flows.*.convs.convs_1x1.2": "duration_predictor.post_flows.*.conv_dds.convs_pointwise.2",
"dp.post_flows.*.convs.convs_sep.0": "duration_predictor.post_flows.*.conv_dds.convs_dilated.0",
"dp.post_flows.*.convs.convs_sep.1": "duration_predictor.post_flows.*.conv_dds.convs_dilated.1",
"dp.post_flows.*.convs.convs_sep.2": "duration_predictor.post_flows.*.conv_dds.convs_dilated.2",
"dp.post_flows.*.convs.norms_1.0.gamma": "duration_predictor.post_flows.*.conv_dds.norms_1.0.weight",
"dp.post_flows.*.convs.norms_1.0.beta": "duration_predictor.post_flows.*.conv_dds.norms_1.0.bias",
"dp.post_flows.*.convs.norms_1.1.gamma": "duration_predictor.post_flows.*.conv_dds.norms_1.1.weight",
"dp.post_flows.*.convs.norms_1.1.beta": "duration_predictor.post_flows.*.conv_dds.norms_1.1.bias",
"dp.post_flows.*.convs.norms_1.2.gamma": "duration_predictor.post_flows.*.conv_dds.norms_1.2.weight",
"dp.post_flows.*.convs.norms_1.2.beta": "duration_predictor.post_flows.*.conv_dds.norms_1.2.bias",
"dp.post_flows.*.convs.norms_2.0.gamma": "duration_predictor.post_flows.*.conv_dds.norms_2.0.weight",
"dp.post_flows.*.convs.norms_2.0.beta": "duration_predictor.post_flows.*.conv_dds.norms_2.0.bias",
"dp.post_flows.*.convs.norms_2.1.gamma": "duration_predictor.post_flows.*.conv_dds.norms_2.1.weight",
"dp.post_flows.*.convs.norms_2.1.beta": "duration_predictor.post_flows.*.conv_dds.norms_2.1.bias",
"dp.post_flows.*.convs.norms_2.2.gamma": "duration_predictor.post_flows.*.conv_dds.norms_2.2.weight",
"dp.post_flows.*.convs.norms_2.2.beta": "duration_predictor.post_flows.*.conv_dds.norms_2.2.bias",
"dp.cond": "duration_predictor.cond", # num_speakers > 1
}
MAPPING_FLOW = {
"flow.flows.*.pre": "flow.flows.*.conv_pre",
"flow.flows.*.enc.in_layers.0": "flow.flows.*.wavenet.in_layers.0",
"flow.flows.*.enc.in_layers.1": "flow.flows.*.wavenet.in_layers.1",
"flow.flows.*.enc.in_layers.2": "flow.flows.*.wavenet.in_layers.2",
"flow.flows.*.enc.in_layers.3": "flow.flows.*.wavenet.in_layers.3",
"flow.flows.*.enc.res_skip_layers.0": "flow.flows.*.wavenet.res_skip_layers.0",
"flow.flows.*.enc.res_skip_layers.1": "flow.flows.*.wavenet.res_skip_layers.1",
"flow.flows.*.enc.res_skip_layers.2": "flow.flows.*.wavenet.res_skip_layers.2",
"flow.flows.*.enc.res_skip_layers.3": "flow.flows.*.wavenet.res_skip_layers.3",
"flow.flows.*.enc.cond_layer": "flow.flows.*.wavenet.cond_layer", # num_speakers > 1
"flow.flows.*.post": "flow.flows.*.conv_post",
}
MAPPING_GENERATOR = {
"dec.conv_pre": "decoder.conv_pre",
"dec.ups.0": "decoder.upsampler.0",
"dec.ups.1": "decoder.upsampler.1",
"dec.ups.2": "decoder.upsampler.2",
"dec.ups.3": "decoder.upsampler.3",
"dec.resblocks.*.convs1.0": "decoder.resblocks.*.convs1.0",
"dec.resblocks.*.convs1.1": "decoder.resblocks.*.convs1.1",
"dec.resblocks.*.convs1.2": "decoder.resblocks.*.convs1.2",
"dec.resblocks.*.convs2.0": "decoder.resblocks.*.convs2.0",
"dec.resblocks.*.convs2.1": "decoder.resblocks.*.convs2.1",
"dec.resblocks.*.convs2.2": "decoder.resblocks.*.convs2.2",
"dec.conv_post": "decoder.conv_post",
"dec.cond": "decoder.cond", # num_speakers > 1
}
MAPPING_POSTERIOR_ENCODER = {
"enc_q.pre": "posterior_encoder.conv_pre",
"enc_q.enc.in_layers.*": "posterior_encoder.wavenet.in_layers.*",
"enc_q.enc.res_skip_layers.*": "posterior_encoder.wavenet.res_skip_layers.*",
"enc_q.enc.cond_layer": "posterior_encoder.wavenet.cond_layer", # num_speakers > 1
"enc_q.proj": "posterior_encoder.conv_proj",
}
MAPPING = {
**MAPPING_TEXT_ENCODER,
**MAPPING_STOCHASTIC_DURATION_PREDICTOR,
**MAPPING_FLOW,
**MAPPING_GENERATOR,
**MAPPING_POSTERIOR_ENCODER,
"emb_g": "embed_speaker", # num_speakers > 1
}
TOP_LEVEL_KEYS = []
IGNORE_KEYS = []
def set_recursively(hf_pointer, key, value, full_name, weight_type):
for attribute in key.split("."):
hf_pointer = getattr(hf_pointer, attribute)
if weight_type is not None:
hf_shape = getattr(hf_pointer, weight_type).shape
else:
hf_shape = hf_pointer.shape
# strip off the kernel dimension at the end (original weights are Conv1d)
if key.endswith(".k_proj") or key.endswith(".v_proj") or key.endswith(".q_proj") or key.endswith(".out_proj"):
value = value.squeeze(-1)
if hf_shape != value.shape:
raise ValueError(
f"Shape of hf {key + '.' + weight_type if weight_type is not None else ''} is {hf_shape}, but should be"
f" {value.shape} for {full_name}"
)
if weight_type == "weight":
hf_pointer.weight.data = value
elif weight_type == "weight_g":
hf_pointer.weight_g.data = value
elif weight_type == "weight_v":
hf_pointer.weight_v.data = value
elif weight_type == "bias":
hf_pointer.bias.data = value
elif weight_type == "running_mean":
hf_pointer.running_mean.data = value
elif weight_type == "running_var":
hf_pointer.running_var.data = value
elif weight_type == "num_batches_tracked":
hf_pointer.num_batches_tracked.data = value
else:
hf_pointer.data = value
logger.info(f"{key + ('.' + weight_type if weight_type is not None else '')} was initialized from {full_name}.")
def should_ignore(name, ignore_keys):
for key in ignore_keys:
if key.endswith(".*"):
if name.startswith(key[:-1]):
return True
elif ".*." in key:
prefix, suffix = key.split(".*.")
if prefix in name and suffix in name:
return True
elif key in name:
return True
return False
def recursively_load_weights(fairseq_dict, hf_model):
unused_weights = []
for name, value in fairseq_dict.items():
if should_ignore(name, IGNORE_KEYS):
logger.info(f"{name} was ignored")
continue
is_used = False
for key, mapped_key in MAPPING.items():
if key.endswith(".*"):
key = key[:-1]
elif "*" in key:
prefix, suffix = key.split(".*.")
if prefix in name and suffix in name:
key = suffix
if key in name:
is_used = True
if mapped_key.endswith(".*"):
layer_index = name.split(key)[-1].split(".")[0]
mapped_key = mapped_key.replace("*", layer_index)
elif "*" in mapped_key:
layer_index = name.split(key)[0].split(".")[-2]
# remap the layer index since we removed the Flip layers
if "flow.flows" in mapped_key:
layer_index = str(int(layer_index) // 2)
if "duration_predictor.flows" in mapped_key or "duration_predictor.post_flows" in mapped_key:
layer_index = str(int(layer_index) // 2 + 1)
mapped_key = mapped_key.replace("*", layer_index)
if "weight_g" in name:
weight_type = "weight_g"
elif "weight_v" in name:
weight_type = "weight_v"
elif "bias" in name:
weight_type = "bias"
elif "weight" in name:
weight_type = "weight"
elif "running_mean" in name:
weight_type = "running_mean"
elif "running_var" in name:
weight_type = "running_var"
elif "num_batches_tracked" in name:
weight_type = "num_batches_tracked"
else:
weight_type = None
set_recursively(hf_model, mapped_key, value, name, weight_type)
continue
if not is_used:
unused_weights.append(name)
logger.warning(f"Unused weights: {unused_weights}")
@torch.no_grad()
def convert_checkpoint(
pytorch_dump_folder_path,
checkpoint_path=None,
config_path=None,
vocab_path=None,
language=None,
num_speakers=None,
sampling_rate=None,
repo_id=None,
):
"""
Copy/paste/tweak model's weights to transformers design.
"""
if config_path is not None:
config = VitsConfig.from_pretrained(config_path)
else:
config = VitsConfig()
if num_speakers:
config.num_speakers = num_speakers
config.speaker_embedding_size = 256
if sampling_rate:
config.sampling_rate = sampling_rate
if checkpoint_path is None:
logger.info(f"***Converting model: facebook/mms-tts {language}***")
vocab_path = hf_hub_download(
repo_id="facebook/mms-tts",
filename="vocab.txt",
subfolder=f"models/{language}",
)
config_file = hf_hub_download(
repo_id="facebook/mms-tts",
filename="config.json",
subfolder=f"models/{language}",
)
checkpoint_path = hf_hub_download(
repo_id="facebook/mms-tts",
filename="G_100000.pth",
subfolder=f"models/{language}",
)
with open(config_file, "r") as f:
data = f.read()
hps = json.loads(data)
is_uroman = hps["data"]["training_files"].split(".")[-1] == "uroman"
if is_uroman:
logger.warning("For this checkpoint, you should use `uroman` to convert input text before tokenizing it!")
else:
logger.info(f"***Converting model: {checkpoint_path}***")
is_uroman = False
# original VITS checkpoint
if vocab_path is None:
_pad = "_"
_punctuation = ';:,.!?¡¿—…"«»“” '
_letters = "ABCDEFGHIJKLMNOPQRSTUVWXYZabcdefghijklmnopqrstuvwxyz"
_letters_ipa = "ɑɐɒæɓʙβɔɕçɗɖðʤəɘɚɛɜɝɞɟʄɡɠɢʛɦɧħɥʜɨɪʝɭɬɫɮʟɱɯɰŋɳɲɴøɵɸθœɶʘɹɺɾɻʀʁɽʂʃʈʧʉʊʋⱱʌɣɤʍχʎʏʑʐʒʔʡʕʢǀǁǂǃˈˌːˑʼʴʰʱʲʷˠˤ˞↓↑→↗↘'̩'ᵻ"
symbols = _pad + _punctuation + _letters + _letters_ipa
symbol_to_id = {s: i for i, s in enumerate(symbols)}
phonemize = True
else:
# Save vocab as temporary json file
symbols = [line.replace("\n", "") for line in open(vocab_path, encoding="utf-8").readlines()]
symbol_to_id = {s: i for i, s in enumerate(symbols)}
# MMS-TTS does not use a <pad> token, so we set to the token used to space characters
_pad = symbols[0]
phonemize = False
with tempfile.NamedTemporaryFile() as tf:
with open(tf.name, "w", encoding="utf-8") as f:
f.write(json.dumps(symbol_to_id, indent=2, sort_keys=True, ensure_ascii=False) + "\n")
tokenizer = VitsTokenizer(tf.name, language=language, phonemize=phonemize, is_uroman=is_uroman, pad_token=_pad)
config.vocab_size = len(symbols)
model = VitsModel(config)
model.decoder.apply_weight_norm()
orig_checkpoint = torch.load(checkpoint_path, map_location=torch.device("cpu"))
recursively_load_weights(orig_checkpoint["model"], model)
model.decoder.remove_weight_norm()
model.save_pretrained(pytorch_dump_folder_path)
tokenizer.save_pretrained(pytorch_dump_folder_path)
if repo_id:
print("Pushing to the hub...")
tokenizer.push_to_hub(repo_id)
model.push_to_hub(repo_id)
if __name__ == "__main__":
parser = argparse.ArgumentParser()
parser.add_argument("--checkpoint_path", default=None, type=str, help="Local path to original checkpoint")
parser.add_argument("--vocab_path", default=None, type=str, help="Path to vocab.txt")
parser.add_argument("--config_path", default=None, type=str, help="Path to hf config.json of model to convert")
parser.add_argument("--language", default=None, type=str, help="Tokenizer language (three-letter code)")
parser.add_argument("--num_speakers", default=None, type=int, help="Number of speakers")
parser.add_argument(
"--sampling_rate", default=None, type=int, help="Sampling rate on which the model was trained."
)
parser.add_argument(
"--pytorch_dump_folder_path", required=True, default=None, type=str, help="Path to the output PyTorch model."
)
parser.add_argument(
"--push_to_hub", default=None, type=str, help="Where to upload the converted model on the 🤗 hub."
)
args = parser.parse_args()
convert_checkpoint(
args.pytorch_dump_folder_path,
args.checkpoint_path,
args.config_path,
args.vocab_path,
args.language,
args.num_speakers,
args.sampling_rate,
args.push_to_hub,
)
| transformers/src/transformers/models/vits/convert_original_checkpoint.py/0 | {
"file_path": "transformers/src/transformers/models/vits/convert_original_checkpoint.py",
"repo_id": "transformers",
"token_count": 8722
} | 330 |
# coding=utf-8
# Copyright 2021 The HuggingFace Inc. team.
#
# Licensed under the Apache License, Version 2.0 (the "License");
# you may not use this file except in compliance with the License.
# You may obtain a copy of the License at
#
# http://www.apache.org/licenses/LICENSE-2.0
#
# Unless required by applicable law or agreed to in writing, software
# distributed under the License is distributed on an "AS IS" BASIS,
# WITHOUT WARRANTIES OR CONDITIONS OF ANY KIND, either express or implied.
# See the License for the specific language governing permissions and
# limitations under the License.
"""
Speech processor class for Wav2Vec2
"""
import warnings
from contextlib import contextmanager
from ...processing_utils import ProcessorMixin
from .feature_extraction_wav2vec2 import Wav2Vec2FeatureExtractor
from .tokenization_wav2vec2 import Wav2Vec2CTCTokenizer
class Wav2Vec2Processor(ProcessorMixin):
r"""
Constructs a Wav2Vec2 processor which wraps a Wav2Vec2 feature extractor and a Wav2Vec2 CTC tokenizer into a single
processor.
[`Wav2Vec2Processor`] offers all the functionalities of [`Wav2Vec2FeatureExtractor`] and [`PreTrainedTokenizer`].
See the docstring of [`~Wav2Vec2Processor.__call__`] and [`~Wav2Vec2Processor.decode`] for more information.
Args:
feature_extractor (`Wav2Vec2FeatureExtractor`):
An instance of [`Wav2Vec2FeatureExtractor`]. The feature extractor is a required input.
tokenizer ([`PreTrainedTokenizer`]):
An instance of [`PreTrainedTokenizer`]. The tokenizer is a required input.
"""
feature_extractor_class = "Wav2Vec2FeatureExtractor"
tokenizer_class = "AutoTokenizer"
def __init__(self, feature_extractor, tokenizer):
super().__init__(feature_extractor, tokenizer)
self.current_processor = self.feature_extractor
self._in_target_context_manager = False
@classmethod
def from_pretrained(cls, pretrained_model_name_or_path, **kwargs):
try:
return super().from_pretrained(pretrained_model_name_or_path, **kwargs)
except OSError:
warnings.warn(
f"Loading a tokenizer inside {cls.__name__} from a config that does not"
" include a `tokenizer_class` attribute is deprecated and will be "
"removed in v5. Please add `'tokenizer_class': 'Wav2Vec2CTCTokenizer'`"
" attribute to either your `config.json` or `tokenizer_config.json` "
"file to suppress this warning: ",
FutureWarning,
)
feature_extractor = Wav2Vec2FeatureExtractor.from_pretrained(pretrained_model_name_or_path, **kwargs)
tokenizer = Wav2Vec2CTCTokenizer.from_pretrained(pretrained_model_name_or_path, **kwargs)
return cls(feature_extractor=feature_extractor, tokenizer=tokenizer)
def __call__(self, *args, **kwargs):
"""
When used in normal mode, this method forwards all its arguments to Wav2Vec2FeatureExtractor's
[`~Wav2Vec2FeatureExtractor.__call__`] and returns its output. If used in the context
[`~Wav2Vec2Processor.as_target_processor`] this method forwards all its arguments to PreTrainedTokenizer's
[`~PreTrainedTokenizer.__call__`]. Please refer to the docstring of the above two methods for more information.
"""
# For backward compatibility
if self._in_target_context_manager:
return self.current_processor(*args, **kwargs)
if "raw_speech" in kwargs:
warnings.warn("Using `raw_speech` as a keyword argument is deprecated. Use `audio` instead.")
audio = kwargs.pop("raw_speech")
else:
audio = kwargs.pop("audio", None)
sampling_rate = kwargs.pop("sampling_rate", None)
text = kwargs.pop("text", None)
if len(args) > 0:
audio = args[0]
args = args[1:]
if audio is None and text is None:
raise ValueError("You need to specify either an `audio` or `text` input to process.")
if audio is not None:
inputs = self.feature_extractor(audio, *args, sampling_rate=sampling_rate, **kwargs)
if text is not None:
encodings = self.tokenizer(text, **kwargs)
if text is None:
return inputs
elif audio is None:
return encodings
else:
inputs["labels"] = encodings["input_ids"]
return inputs
def pad(self, *args, **kwargs):
"""
When used in normal mode, this method forwards all its arguments to Wav2Vec2FeatureExtractor's
[`~Wav2Vec2FeatureExtractor.pad`] and returns its output. If used in the context
[`~Wav2Vec2Processor.as_target_processor`] this method forwards all its arguments to PreTrainedTokenizer's
[`~PreTrainedTokenizer.pad`]. Please refer to the docstring of the above two methods for more information.
"""
# For backward compatibility
if self._in_target_context_manager:
return self.current_processor.pad(*args, **kwargs)
input_features = kwargs.pop("input_features", None)
labels = kwargs.pop("labels", None)
if len(args) > 0:
input_features = args[0]
args = args[1:]
if input_features is not None:
input_features = self.feature_extractor.pad(input_features, *args, **kwargs)
if labels is not None:
labels = self.tokenizer.pad(labels, **kwargs)
if labels is None:
return input_features
elif input_features is None:
return labels
else:
input_features["labels"] = labels["input_ids"]
return input_features
def batch_decode(self, *args, **kwargs):
"""
This method forwards all its arguments to PreTrainedTokenizer's [`~PreTrainedTokenizer.batch_decode`]. Please
refer to the docstring of this method for more information.
"""
return self.tokenizer.batch_decode(*args, **kwargs)
def decode(self, *args, **kwargs):
"""
This method forwards all its arguments to PreTrainedTokenizer's [`~PreTrainedTokenizer.decode`]. Please refer
to the docstring of this method for more information.
"""
return self.tokenizer.decode(*args, **kwargs)
@contextmanager
def as_target_processor(self):
"""
Temporarily sets the tokenizer for processing the input. Useful for encoding the labels when fine-tuning
Wav2Vec2.
"""
warnings.warn(
"`as_target_processor` is deprecated and will be removed in v5 of Transformers. You can process your "
"labels by using the argument `text` of the regular `__call__` method (either in the same call as "
"your audio inputs, or in a separate call."
)
self._in_target_context_manager = True
self.current_processor = self.tokenizer
yield
self.current_processor = self.feature_extractor
self._in_target_context_manager = False
| transformers/src/transformers/models/wav2vec2/processing_wav2vec2.py/0 | {
"file_path": "transformers/src/transformers/models/wav2vec2/processing_wav2vec2.py",
"repo_id": "transformers",
"token_count": 2839
} | 331 |
# coding=utf-8
# Copyright 2021 The Fairseq Authors, Microsoft Research, and The HuggingFace Inc. team. All rights reserved.
#
# Licensed under the Apache License, Version 2.0 (the "License");
# you may not use this file except in compliance with the License.
# You may obtain a copy of the License at
#
# http://www.apache.org/licenses/LICENSE-2.0
#
# Unless required by applicable law or agreed to in writing, software
# distributed under the License is distributed on an "AS IS" BASIS,
# WITHOUT WARRANTIES OR CONDITIONS OF ANY KIND, either express or implied.
# See the License for the specific language governing permissions and
# limitations under the License.
""" WavLM model configuration"""
import functools
import operator
from ...configuration_utils import PretrainedConfig
from ...utils import logging
logger = logging.get_logger(__name__)
WAVLM_PRETRAINED_CONFIG_ARCHIVE_MAP = {
"microsoft/wavlm-base": "https://huggingface.co/microsoft/wavlm-base/resolve/main/config.json",
# See all WavLM models at https://huggingface.co/models?filter=wavlm
}
class WavLMConfig(PretrainedConfig):
r"""
This is the configuration class to store the configuration of a [`WavLMModel`]. It is used to instantiate an WavLM
model according to the specified arguments, defining the model architecture. Instantiating a configuration with the
defaults will yield a similar configuration to that of the WavLM
[microsoft/wavlm-base](https://huggingface.co/microsoft/wavlm-base) architecture.
Configuration objects inherit from [`PretrainedConfig`] and can be used to control the model outputs. Read the
documentation from [`PretrainedConfig`] for more information.
Args:
vocab_size (`int`, *optional*, defaults to 32):
Vocabulary size of the WavLM model. Defines the number of different tokens that can be represented by the
`inputs_ids` passed when calling [`WavLMModel`]. Vocabulary size of the model. Defines the different tokens
that can be represented by the *inputs_ids* passed to the forward method of [`WavLMModel`].
hidden_size (`int`, *optional*, defaults to 768):
Dimensionality of the encoder layers and the pooler layer.
num_hidden_layers (`int`, *optional*, defaults to 12):
Number of hidden layers in the Transformer encoder.
num_attention_heads (`int`, *optional*, defaults to 12):
Number of attention heads for each attention layer in the Transformer encoder.
intermediate_size (`int`, *optional*, defaults to 3072):
Dimensionality of the "intermediate" (i.e., feed-forward) layer in the Transformer encoder.
hidden_act (`str` or `function`, *optional*, defaults to `"gelu"`):
The non-linear activation function (function or string) in the encoder and pooler. If string, `"gelu"`,
`"relu"`, `"selu"` and `"gelu_new"` are supported.
hidden_dropout (`float`, *optional*, defaults to 0.1):
The dropout probability for all fully connected layers in the embeddings, encoder, and pooler.
activation_dropout (`float`, *optional*, defaults to 0.1):
The dropout ratio for activations inside the fully connected layer.
attention_dropout (`float`, *optional*, defaults to 0.1):
The dropout ratio for the attention probabilities.
final_dropout (`float`, *optional*, defaults to 0.1):
The dropout probability for the final projection layer of [`WavLMForCTC`].
layerdrop (`float`, *optional*, defaults to 0.1):
The LayerDrop probability. See the [LayerDrop paper](see https://arxiv.org/abs/1909.11556) for more
details.
initializer_range (`float`, *optional*, defaults to 0.02):
The standard deviation of the truncated_normal_initializer for initializing all weight matrices.
layer_norm_eps (`float`, *optional*, defaults to 1e-12):
The epsilon used by the layer normalization layers.
feat_extract_norm (`str`, *optional*, defaults to `"group"`):
The norm to be applied to 1D convolutional layers in feature encoder. One of `"group"` for group
normalization of only the first 1D convolutional layer or `"layer"` for layer normalization of all 1D
convolutional layers.
feat_proj_dropout (`float`, *optional*, defaults to 0.0):
The dropout probability for output of the feature encoder.
feat_extract_activation (`str, `optional`, defaults to `"gelu"`):
The non-linear activation function (function or string) in the 1D convolutional layers of the feature
extractor. If string, `"gelu"`, `"relu"`, `"selu"` and `"gelu_new"` are supported.
conv_dim (`Tuple[int]` or `List[int]`, *optional*, defaults to `(512, 512, 512, 512, 512, 512, 512)`):
A tuple of integers defining the number of input and output channels of each 1D convolutional layer in the
feature encoder. The length of *conv_dim* defines the number of 1D convolutional layers.
conv_stride (`Tuple[int]` or `List[int]`, *optional*, defaults to `(5, 2, 2, 2, 2, 2, 2)`):
A tuple of integers defining the stride of each 1D convolutional layer in the feature encoder. The length
of *conv_stride* defines the number of convolutional layers and has to match the length of *conv_dim*.
conv_kernel (`Tuple[int]` or `List[int]`, *optional*, defaults to `(10, 3, 3, 3, 3, 3, 3)`):
A tuple of integers defining the kernel size of each 1D convolutional layer in the feature encoder. The
length of *conv_kernel* defines the number of convolutional layers and has to match the length of
*conv_dim*.
conv_bias (`bool`, *optional*, defaults to `False`):
Whether the 1D convolutional layers have a bias.
num_conv_pos_embeddings (`int`, *optional*, defaults to 128):
Number of convolutional positional embeddings. Defines the kernel size of 1D convolutional positional
embeddings layer.
num_conv_pos_embedding_groups (`int`, *optional*, defaults to 16):
Number of groups of 1D convolutional positional embeddings layer.
do_stable_layer_norm (`bool`, *optional*, defaults to `False`):
Whether to apply *stable* layer norm architecture of the Transformer encoder. `do_stable_layer_norm is
True` corresponds to applying layer norm before the attention layer, whereas `do_stable_layer_norm is
False` corresponds to applying layer norm after the attention layer.
apply_spec_augment (`bool`, *optional*, defaults to `True`):
Whether to apply *SpecAugment* data augmentation to the outputs of the feature encoder. For reference see
[SpecAugment: A Simple Data Augmentation Method for Automatic Speech
Recognition](https://arxiv.org/abs/1904.08779).
mask_time_prob (`float`, *optional*, defaults to 0.05):
Propability of each feature vector along the time axis to be chosen as the start of the vector span to be
masked. Approximately `mask_time_prob * sequence_length // mask_time_length` feature vectors will be masked
along the time axis. This is only relevant if `apply_spec_augment is True`.
mask_time_length (`int`, *optional*, defaults to 10):
Length of vector span along the time axis.
mask_time_min_masks (`int`, *optional*, defaults to 2),:
The minimum number of masks of length `mask_feature_length` generated along the time axis, each time step,
irrespectively of `mask_feature_prob`. Only relevant if ''mask_time_prob*len(time_axis)/mask_time_length <
mask_time_min_masks''
mask_feature_prob (`float`, *optional*, defaults to 0.0):
Propability of each feature vector along the feature axis to be chosen as the start of the vector span to
be masked. Approximately `mask_time_prob * hidden_size // mask_time_length` feature vectors will be masked
along the time axis. This is only relevant if `apply_spec_augment is True`.
mask_feature_length (`int`, *optional*, defaults to 10):
Length of vector span along the feature axis.
num_codevectors_per_group (`int`, *optional*, defaults to 320):
Number of entries in each quantization codebook (group).
num_codevector_groups (`int`, *optional*, defaults to 2):
Number of codevector groups for product codevector quantization.
contrastive_logits_temperature (`float`, *optional*, defaults to 0.1):
The temperature *kappa* in the contrastive loss.
num_negatives (`int`, *optional*, defaults to 100):
Number of negative samples for the contrastive loss.
codevector_dim (`int`, *optional*, defaults to 256):
Dimensionality of the quantized feature vectors.
proj_codevector_dim (`int`, *optional*, defaults to 256):
Dimensionality of the final projection of both the quantized and the transformer features.
diversity_loss_weight (`int`, *optional*, defaults to 0.1):
The weight of the codebook diversity loss component.
ctc_loss_reduction (`str`, *optional*, defaults to `"mean"`):
Specifies the reduction to apply to the output of `torch.nn.CTCLoss`. Only relevant when training an
instance of [`WavLMForCTC`].
ctc_zero_infinity (`bool`, *optional*, defaults to `False`):
Whether to zero infinite losses and the associated gradients of `torch.nn.CTCLoss`. Infinite losses mainly
occur when the inputs are too short to be aligned to the targets. Only relevant when training an instance
of [`WavLMForCTC`].
use_weighted_layer_sum (`bool`, *optional*, defaults to `False`):
Whether to use a weighted average of layer outputs with learned weights. Only relevant when using an
instance of [`WavLMForSequenceClassification`].
classifier_proj_size (`int`, *optional*, defaults to 256):
Dimensionality of the projection before token mean-pooling for classification.
tdnn_dim (`Tuple[int]` or `List[int]`, *optional*, defaults to `(512, 512, 512, 512, 1500)`):
A tuple of integers defining the number of output channels of each 1D convolutional layer in the *TDNN*
module of the *XVector* model. The length of *tdnn_dim* defines the number of *TDNN* layers.
tdnn_kernel (`Tuple[int]` or `List[int]`, *optional*, defaults to `(5, 3, 3, 1, 1)`):
A tuple of integers defining the kernel size of each 1D convolutional layer in the *TDNN* module of the
*XVector* model. The length of *tdnn_kernel* has to match the length of *tdnn_dim*.
tdnn_dilation (`Tuple[int]` or `List[int]`, *optional*, defaults to `(1, 2, 3, 1, 1)`):
A tuple of integers defining the dilation factor of each 1D convolutional layer in *TDNN* module of the
*XVector* model. The length of *tdnn_dilation* has to match the length of *tdnn_dim*.
xvector_output_dim (`int`, *optional*, defaults to 512):
Dimensionality of the *XVector* embedding vectors.
add_adapter (`bool`, *optional*, defaults to `False`):
Whether a convolutional network should be stacked on top of the Wav2Vec2 Encoder. Can be very useful for
warm-starting Wav2Vec2 for SpeechEncoderDecoder models.
adapter_kernel_size (`int`, *optional*, defaults to 3):
Kernel size of the convolutional layers in the adapter network. Only relevant if `add_adapter is True`.
adapter_stride (`int`, *optional*, defaults to 2):
Stride of the convolutional layers in the adapter network. Only relevant if `add_adapter is True`.
num_adapter_layers (`int`, *optional*, defaults to 3):
Number of convolutional layers that should be used in the adapter network. Only relevant if `add_adapter is
True`.
output_hidden_size (`int`, *optional*):
Dimensionality of the encoder output layer. If not defined, this defaults to *hidden-size*. Only relevant
if `add_adapter is True`.
Example:
```python
```
Example:
```python
>>> from transformers import WavLMConfig, WavLMModel
>>> # Initializing a WavLM facebook/wavlm-base-960h style configuration
>>> configuration = WavLMConfig()
>>> # Initializing a model (with random weights) from the facebook/wavlm-base-960h style configuration
>>> model = WavLMModel(configuration)
>>> # Accessing the model configuration
>>> configuration = model.config
```"""
model_type = "wavlm"
def __init__(
self,
vocab_size=32,
hidden_size=768,
num_hidden_layers=12,
num_attention_heads=12,
intermediate_size=3072,
hidden_act="gelu",
hidden_dropout=0.1,
activation_dropout=0.1,
attention_dropout=0.1,
feat_proj_dropout=0.0,
final_dropout=0.1,
layerdrop=0.1,
initializer_range=0.02,
layer_norm_eps=1e-5,
feat_extract_norm="group",
feat_extract_activation="gelu",
conv_dim=(512, 512, 512, 512, 512, 512, 512),
conv_stride=(5, 2, 2, 2, 2, 2, 2),
conv_kernel=(10, 3, 3, 3, 3, 2, 2),
conv_bias=False,
num_conv_pos_embeddings=128,
num_conv_pos_embedding_groups=16,
num_buckets=320,
max_bucket_distance=800,
do_stable_layer_norm=False,
apply_spec_augment=True,
mask_time_prob=0.05,
mask_time_length=10,
mask_time_min_masks=2,
mask_feature_prob=0.0,
mask_feature_length=10,
num_codevectors_per_group=320,
num_codevector_groups=2,
contrastive_logits_temperature=0.1,
num_negatives=100,
codevector_dim=256,
proj_codevector_dim=256,
diversity_loss_weight=0.1,
ctc_loss_reduction="mean",
ctc_zero_infinity=False,
use_weighted_layer_sum=False,
classifier_proj_size=256,
tdnn_dim=(512, 512, 512, 512, 1500),
tdnn_kernel=(5, 3, 3, 1, 1),
tdnn_dilation=(1, 2, 3, 1, 1),
xvector_output_dim=512,
num_ctc_classes=80,
pad_token_id=0,
bos_token_id=1,
eos_token_id=2,
add_adapter=False,
adapter_kernel_size=3,
adapter_stride=2,
num_adapter_layers=3,
output_hidden_size=None,
**kwargs,
):
super().__init__(**kwargs, pad_token_id=pad_token_id, bos_token_id=bos_token_id, eos_token_id=eos_token_id)
self.hidden_size = hidden_size
self.feat_extract_norm = feat_extract_norm
self.feat_extract_activation = feat_extract_activation
self.conv_dim = list(conv_dim)
self.conv_stride = list(conv_stride)
self.conv_kernel = list(conv_kernel)
self.conv_bias = conv_bias
self.num_buckets = num_buckets
self.max_bucket_distance = max_bucket_distance
self.num_conv_pos_embeddings = num_conv_pos_embeddings
self.num_conv_pos_embedding_groups = num_conv_pos_embedding_groups
self.num_feat_extract_layers = len(self.conv_dim)
self.num_hidden_layers = num_hidden_layers
self.intermediate_size = intermediate_size
self.hidden_act = hidden_act
self.num_attention_heads = num_attention_heads
self.hidden_dropout = hidden_dropout
self.attention_dropout = attention_dropout
self.activation_dropout = activation_dropout
self.feat_proj_dropout = feat_proj_dropout
self.final_dropout = final_dropout
self.layerdrop = layerdrop
self.layer_norm_eps = layer_norm_eps
self.initializer_range = initializer_range
self.num_ctc_classes = num_ctc_classes
self.vocab_size = vocab_size
self.do_stable_layer_norm = do_stable_layer_norm
self.use_weighted_layer_sum = use_weighted_layer_sum
self.classifier_proj_size = classifier_proj_size
if (
(len(self.conv_stride) != self.num_feat_extract_layers)
or (len(self.conv_kernel) != self.num_feat_extract_layers)
or (len(self.conv_dim) != self.num_feat_extract_layers)
):
raise ValueError(
"Configuration for convolutional layers is incorrect. It is required that `len(config.conv_dim)` =="
" `len(config.conv_stride)` == `len(config.conv_kernel)`, but is `len(config.conv_dim) ="
f" {len(self.conv_dim)}`, `len(config.conv_stride) = {len(self.conv_stride)}`,"
f" `len(config.conv_kernel) = {len(self.conv_kernel)}`."
)
# fine-tuning config parameters for SpecAugment: https://arxiv.org/abs/1904.08779
self.apply_spec_augment = apply_spec_augment
self.mask_time_prob = mask_time_prob
self.mask_time_length = mask_time_length
self.mask_time_min_masks = mask_time_min_masks
self.mask_feature_prob = mask_feature_prob
self.mask_feature_length = mask_feature_length
# parameters for pretraining with codevector quantized representations
self.num_codevectors_per_group = num_codevectors_per_group
self.num_codevector_groups = num_codevector_groups
self.contrastive_logits_temperature = contrastive_logits_temperature
self.num_negatives = num_negatives
self.codevector_dim = codevector_dim
self.proj_codevector_dim = proj_codevector_dim
self.diversity_loss_weight = diversity_loss_weight
# ctc loss
self.ctc_loss_reduction = ctc_loss_reduction
self.ctc_zero_infinity = ctc_zero_infinity
# adapter
self.add_adapter = add_adapter
self.adapter_kernel_size = adapter_kernel_size
self.adapter_stride = adapter_stride
self.num_adapter_layers = num_adapter_layers
self.output_hidden_size = output_hidden_size or hidden_size
# SequenceClassification-specific parameter. Feel free to ignore for other classes.
self.classifier_proj_size = classifier_proj_size
# XVector-specific parameters. Feel free to ignore for other classes.
self.tdnn_dim = list(tdnn_dim)
self.tdnn_kernel = list(tdnn_kernel)
self.tdnn_dilation = list(tdnn_dilation)
self.xvector_output_dim = xvector_output_dim
@property
def inputs_to_logits_ratio(self):
return functools.reduce(operator.mul, self.conv_stride, 1)
| transformers/src/transformers/models/wavlm/configuration_wavlm.py/0 | {
"file_path": "transformers/src/transformers/models/wavlm/configuration_wavlm.py",
"repo_id": "transformers",
"token_count": 7367
} | 332 |
# coding=utf-8
# Copyright 2019-present, Facebook, Inc and the HuggingFace Inc. team.
#
# Licensed under the Apache License, Version 2.0 (the "License");
# you may not use this file except in compliance with the License.
# You may obtain a copy of the License at
#
# http://www.apache.org/licenses/LICENSE-2.0
#
# Unless required by applicable law or agreed to in writing, software
# distributed under the License is distributed on an "AS IS" BASIS,
# WITHOUT WARRANTIES OR CONDITIONS OF ANY KIND, either express or implied.
# See the License for the specific language governing permissions and
# limitations under the License.
"""
TF 2.0 XLM model.
"""
from __future__ import annotations
import itertools
import warnings
from dataclasses import dataclass
from typing import Dict, Optional, Tuple, Union
import numpy as np
import tensorflow as tf
from ...activations_tf import get_tf_activation
from ...modeling_tf_outputs import (
TFBaseModelOutput,
TFMultipleChoiceModelOutput,
TFQuestionAnsweringModelOutput,
TFSequenceClassifierOutput,
TFTokenClassifierOutput,
)
from ...modeling_tf_utils import (
TFModelInputType,
TFMultipleChoiceLoss,
TFPreTrainedModel,
TFQuestionAnsweringLoss,
TFSequenceClassificationLoss,
TFSequenceSummary,
TFSharedEmbeddings,
TFTokenClassificationLoss,
get_initializer,
keras,
keras_serializable,
unpack_inputs,
)
from ...tf_utils import check_embeddings_within_bounds, shape_list, stable_softmax
from ...utils import (
MULTIPLE_CHOICE_DUMMY_INPUTS,
ModelOutput,
add_code_sample_docstrings,
add_start_docstrings,
add_start_docstrings_to_model_forward,
logging,
)
from .configuration_xlm import XLMConfig
logger = logging.get_logger(__name__)
_CHECKPOINT_FOR_DOC = "xlm-mlm-en-2048"
_CONFIG_FOR_DOC = "XLMConfig"
TF_XLM_PRETRAINED_MODEL_ARCHIVE_LIST = [
"xlm-mlm-en-2048",
"xlm-mlm-ende-1024",
"xlm-mlm-enfr-1024",
"xlm-mlm-enro-1024",
"xlm-mlm-tlm-xnli15-1024",
"xlm-mlm-xnli15-1024",
"xlm-clm-enfr-1024",
"xlm-clm-ende-1024",
"xlm-mlm-17-1280",
"xlm-mlm-100-1280",
# See all XLM models at https://huggingface.co/models?filter=xlm
]
def create_sinusoidal_embeddings(n_pos, dim, out):
position_enc = np.array([[pos / np.power(10000, 2 * (j // 2) / dim) for j in range(dim)] for pos in range(n_pos)])
out[:, 0::2] = tf.constant(np.sin(position_enc[:, 0::2]))
out[:, 1::2] = tf.constant(np.cos(position_enc[:, 1::2]))
def get_masks(slen, lengths, causal, padding_mask=None):
"""
Generate hidden states mask, and optionally an attention mask.
"""
bs = shape_list(lengths)[0]
if padding_mask is not None:
mask = padding_mask
else:
# assert lengths.max().item() <= slen
alen = tf.range(slen, dtype=lengths.dtype)
mask = alen < tf.expand_dims(lengths, axis=1)
# attention mask is the same as mask, or triangular inferior attention (causal)
if causal:
attn_mask = tf.less_equal(
tf.tile(tf.reshape(alen, (1, 1, slen)), (bs, slen, 1)), tf.reshape(alen, (1, slen, 1))
)
else:
attn_mask = mask
# sanity check
# assert shape_list(mask) == [bs, slen]
tf.debugging.assert_equal(shape_list(mask), [bs, slen])
if causal:
tf.debugging.assert_equal(shape_list(attn_mask), [bs, slen, slen])
return mask, attn_mask
class TFXLMMultiHeadAttention(keras.layers.Layer):
NEW_ID = itertools.count()
def __init__(self, n_heads, dim, config, **kwargs):
super().__init__(**kwargs)
self.layer_id = next(TFXLMMultiHeadAttention.NEW_ID)
self.dim = dim
self.n_heads = n_heads
self.output_attentions = config.output_attentions
assert self.dim % self.n_heads == 0
self.q_lin = keras.layers.Dense(dim, kernel_initializer=get_initializer(config.init_std), name="q_lin")
self.k_lin = keras.layers.Dense(dim, kernel_initializer=get_initializer(config.init_std), name="k_lin")
self.v_lin = keras.layers.Dense(dim, kernel_initializer=get_initializer(config.init_std), name="v_lin")
self.out_lin = keras.layers.Dense(dim, kernel_initializer=get_initializer(config.init_std), name="out_lin")
self.dropout = keras.layers.Dropout(config.attention_dropout)
self.pruned_heads = set()
self.dim = dim
def prune_heads(self, heads):
raise NotImplementedError
def call(self, input, mask, kv, cache, head_mask, output_attentions, training=False):
"""
Self-attention (if kv is None) or attention over source sentence (provided by kv).
"""
# Input is (bs, qlen, dim)
# Mask is (bs, klen) (non-causal) or (bs, klen, klen)
bs, qlen, dim = shape_list(input)
if kv is None:
klen = qlen if cache is None else cache["slen"] + qlen
else:
klen = shape_list(kv)[1]
# assert dim == self.dim, f'Dimensions do not match: {dim} input vs {self.dim} configured'
dim_per_head = self.dim // self.n_heads
mask_reshape = (bs, 1, qlen, klen) if len(shape_list(mask)) == 3 else (bs, 1, 1, klen)
def shape(x):
"""projection"""
return tf.transpose(tf.reshape(x, (bs, -1, self.n_heads, dim_per_head)), perm=(0, 2, 1, 3))
def unshape(x):
"""compute context"""
return tf.reshape(tf.transpose(x, perm=(0, 2, 1, 3)), (bs, -1, self.n_heads * dim_per_head))
q = shape(self.q_lin(input)) # (bs, n_heads, qlen, dim_per_head)
if kv is None:
k = shape(self.k_lin(input)) # (bs, n_heads, qlen, dim_per_head)
v = shape(self.v_lin(input)) # (bs, n_heads, qlen, dim_per_head)
elif cache is None or self.layer_id not in cache:
k = v = kv
k = shape(self.k_lin(k)) # (bs, n_heads, qlen, dim_per_head)
v = shape(self.v_lin(v)) # (bs, n_heads, qlen, dim_per_head)
if cache is not None:
if self.layer_id in cache:
if kv is None:
k_, v_ = cache[self.layer_id]
k = tf.concat([k_, k], axis=2) # (bs, n_heads, klen, dim_per_head)
v = tf.concat([v_, v], axis=2) # (bs, n_heads, klen, dim_per_head)
else:
k, v = cache[self.layer_id]
cache[self.layer_id] = (k, v)
f_dim_per_head = tf.cast(dim_per_head, dtype=q.dtype)
q = tf.multiply(q, tf.math.rsqrt(f_dim_per_head)) # (bs, n_heads, qlen, dim_per_head)
k = tf.cast(k, dtype=q.dtype)
scores = tf.matmul(q, k, transpose_b=True) # (bs, n_heads, qlen, klen)
mask = tf.reshape(mask, mask_reshape) # (bs, n_heads, qlen, klen)
# scores.masked_fill_(mask, -float('inf')) # (bs, n_heads, qlen, klen)
mask = tf.cast(mask, dtype=scores.dtype)
scores = scores - 1e30 * (1.0 - mask)
weights = stable_softmax(scores, axis=-1) # (bs, n_heads, qlen, klen)
weights = self.dropout(weights, training=training) # (bs, n_heads, qlen, klen)
# Mask heads if we want to
if head_mask is not None:
weights = weights * head_mask
context = tf.matmul(weights, v) # (bs, n_heads, qlen, dim_per_head)
context = unshape(context) # (bs, qlen, dim)
outputs = (self.out_lin(context),)
if output_attentions:
outputs = outputs + (weights,)
return outputs
def build(self, input_shape=None):
if self.built:
return
self.built = True
if getattr(self, "q_lin", None) is not None:
with tf.name_scope(self.q_lin.name):
self.q_lin.build([None, None, self.dim])
if getattr(self, "k_lin", None) is not None:
with tf.name_scope(self.k_lin.name):
self.k_lin.build([None, None, self.dim])
if getattr(self, "v_lin", None) is not None:
with tf.name_scope(self.v_lin.name):
self.v_lin.build([None, None, self.dim])
if getattr(self, "out_lin", None) is not None:
with tf.name_scope(self.out_lin.name):
self.out_lin.build([None, None, self.dim])
class TFXLMTransformerFFN(keras.layers.Layer):
def __init__(self, in_dim, dim_hidden, out_dim, config, **kwargs):
super().__init__(**kwargs)
self.lin1 = keras.layers.Dense(dim_hidden, kernel_initializer=get_initializer(config.init_std), name="lin1")
self.lin2 = keras.layers.Dense(out_dim, kernel_initializer=get_initializer(config.init_std), name="lin2")
self.act = get_tf_activation("gelu") if config.gelu_activation else get_tf_activation("relu")
self.dropout = keras.layers.Dropout(config.dropout)
self.in_dim = in_dim
self.dim_hidden = dim_hidden
def call(self, input, training=False):
x = self.lin1(input)
x = self.act(x)
x = self.lin2(x)
x = self.dropout(x, training=training)
return x
def build(self, input_shape=None):
if self.built:
return
self.built = True
if getattr(self, "lin1", None) is not None:
with tf.name_scope(self.lin1.name):
self.lin1.build([None, None, self.in_dim])
if getattr(self, "lin2", None) is not None:
with tf.name_scope(self.lin2.name):
self.lin2.build([None, None, self.dim_hidden])
@keras_serializable
class TFXLMMainLayer(keras.layers.Layer):
config_class = XLMConfig
def __init__(self, config, **kwargs):
super().__init__(**kwargs)
self.config = config
self.output_hidden_states = config.output_hidden_states
self.output_attentions = config.output_attentions
self.return_dict = config.use_return_dict
# encoder / decoder, output layer
self.is_encoder = config.is_encoder
self.is_decoder = not config.is_encoder
if self.is_decoder:
raise NotImplementedError("Currently XLM can only be used as an encoder")
# self.with_output = with_output
self.causal = config.causal
# dictionary / languages
self.n_langs = config.n_langs
self.use_lang_emb = config.use_lang_emb
self.n_words = config.n_words
self.eos_index = config.eos_index
self.pad_index = config.pad_index
# self.dico = dico
# self.id2lang = config.id2lang
# self.lang2id = config.lang2id
# assert len(self.dico) == self.n_words
# assert len(self.id2lang) == len(self.lang2id) == self.n_langs
# model parameters
self.dim = config.emb_dim # 512 by default
self.hidden_dim = self.dim * 4 # 2048 by default
self.n_heads = config.n_heads # 8 by default
self.n_layers = config.n_layers
self.max_position_embeddings = config.max_position_embeddings
self.embed_init_std = config.embed_init_std
if self.dim % self.n_heads != 0:
raise ValueError("transformer dim must be a multiple of n_heads")
# embeddings
self.dropout = keras.layers.Dropout(config.dropout)
self.attention_dropout = keras.layers.Dropout(config.attention_dropout)
if config.sinusoidal_embeddings:
raise NotImplementedError
# create_sinusoidal_embeddings(config.max_position_embeddings, self.dim, out=self.position_embeddings.weight)
self.embeddings = TFSharedEmbeddings(
self.n_words, self.dim, initializer_range=config.embed_init_std, name="embeddings"
) # padding_idx=self.pad_index)
self.layer_norm_emb = keras.layers.LayerNormalization(epsilon=config.layer_norm_eps, name="layer_norm_emb")
# transformer layers
self.attentions = []
self.layer_norm1 = []
self.ffns = []
self.layer_norm2 = []
# if self.is_decoder:
# self.layer_norm15 = []
# self.encoder_attn = []
for i in range(self.n_layers):
self.attentions.append(
TFXLMMultiHeadAttention(self.n_heads, self.dim, config=config, name=f"attentions_._{i}")
)
self.layer_norm1.append(
keras.layers.LayerNormalization(epsilon=config.layer_norm_eps, name=f"layer_norm1_._{i}")
)
# if self.is_decoder:
# self.layer_norm15.append(nn.LayerNorm(self.dim, eps=config.layer_norm_eps))
# self.encoder_attn.append(MultiHeadAttention(self.n_heads, self.dim, dropout=self.attention_dropout))
self.ffns.append(
TFXLMTransformerFFN(self.dim, self.hidden_dim, self.dim, config=config, name=f"ffns_._{i}")
)
self.layer_norm2.append(
keras.layers.LayerNormalization(epsilon=config.layer_norm_eps, name=f"layer_norm2_._{i}")
)
if hasattr(config, "pruned_heads"):
pruned_heads = config.pruned_heads.copy().items()
config.pruned_heads = {}
for layer, heads in pruned_heads:
if self.attentions[int(layer)].n_heads == config.n_heads:
self.prune_heads({int(layer): list(map(int, heads))})
def build(self, input_shape=None):
if self.built:
return
self.built = True
with tf.name_scope("position_embeddings"):
self.position_embeddings = self.add_weight(
name="embeddings",
shape=[self.max_position_embeddings, self.dim],
initializer=get_initializer(self.embed_init_std),
)
if self.n_langs > 1 and self.use_lang_emb:
with tf.name_scope("lang_embeddings"):
self.lang_embeddings = self.add_weight(
name="embeddings",
shape=[self.n_langs, self.dim],
initializer=get_initializer(self.embed_init_std),
)
if getattr(self, "embeddings", None) is not None:
with tf.name_scope(self.embeddings.name):
self.embeddings.build(None)
if getattr(self, "layer_norm_emb", None) is not None:
with tf.name_scope(self.layer_norm_emb.name):
self.layer_norm_emb.build([None, None, self.dim])
for layer in self.attentions:
with tf.name_scope(layer.name):
layer.build(None)
for layer in self.layer_norm1:
with tf.name_scope(layer.name):
layer.build([None, None, self.dim])
for layer in self.ffns:
with tf.name_scope(layer.name):
layer.build(None)
for layer in self.layer_norm2:
with tf.name_scope(layer.name):
layer.build([None, None, self.dim])
def get_input_embeddings(self):
return self.embeddings
def set_input_embeddings(self, value):
self.embeddings.weight = value
self.embeddings.vocab_size = shape_list(value)[0]
def _prune_heads(self, heads_to_prune):
"""
Prunes heads of the model. heads_to_prune: dict of {layer_num: list of heads to prune in this layer} See base
class PreTrainedModel
"""
raise NotImplementedError
@unpack_inputs
def call(
self,
input_ids=None,
attention_mask=None,
langs=None,
token_type_ids=None,
position_ids=None,
lengths=None,
cache=None,
head_mask=None,
inputs_embeds=None,
output_attentions=None,
output_hidden_states=None,
return_dict=None,
training=False,
) -> Union[TFBaseModelOutput, Tuple[tf.Tensor]]:
# removed: src_enc=None, src_len=None
if input_ids is not None and inputs_embeds is not None:
raise ValueError("You cannot specify both input_ids and inputs_embeds at the same time")
elif input_ids is not None:
bs, slen = shape_list(input_ids)
elif inputs_embeds is not None:
bs, slen = shape_list(inputs_embeds)[:2]
else:
raise ValueError("You have to specify either input_ids or inputs_embeds")
if lengths is None:
if input_ids is not None:
lengths = tf.reduce_sum(
tf.cast(tf.not_equal(input_ids, self.pad_index), dtype=input_ids.dtype), axis=1
)
else:
lengths = tf.convert_to_tensor([slen] * bs)
# mask = input_ids != self.pad_index
# check inputs
# assert shape_list(lengths)[0] == bs
(
tf.debugging.assert_equal(shape_list(lengths)[0], bs),
f"Expected batch size {shape_list(lengths)[0]} and received batch size {bs} mismatched",
)
# assert lengths.max().item() <= slen
# input_ids = input_ids.transpose(0, 1) # batch size as dimension 0
# assert (src_enc is None) == (src_len is None)
# if src_enc is not None:
# assert self.is_decoder
# assert src_enc.size(0) == bs
# generate masks
mask, attn_mask = get_masks(slen, lengths, self.causal, padding_mask=attention_mask)
# if self.is_decoder and src_enc is not None:
# src_mask = torch.arange(src_len.max(), dtype=torch.long, device=lengths.device) < src_len[:, None]
# position_ids
if position_ids is None:
position_ids = tf.expand_dims(tf.range(slen), axis=0)
position_ids = tf.tile(position_ids, (bs, 1))
# assert shape_list(position_ids) == [bs, slen] # (slen, bs)
(
tf.debugging.assert_equal(shape_list(position_ids), [bs, slen]),
f"Position id shape {shape_list(position_ids)} and input shape {[bs, slen]} mismatched",
)
# position_ids = position_ids.transpose(0, 1)
# langs
if langs is not None:
# assert shape_list(langs) == [bs, slen] # (slen, bs)
(
tf.debugging.assert_equal(shape_list(langs), [bs, slen]),
f"Lang shape {shape_list(langs)} and input shape {[bs, slen]} mismatched",
)
# langs = langs.transpose(0, 1)
# Prepare head mask if needed
# 1.0 in head_mask indicate we keep the head
# attention_probs has shape bsz x n_heads x N x N
# input head_mask has shape [num_heads] or [num_hidden_layers x num_heads]
# and head_mask is converted to shape [num_hidden_layers x batch x num_heads x qlen x klen]
if head_mask is not None:
raise NotImplementedError
else:
head_mask = [None] * self.n_layers
# do not recompute cached elements
if cache is not None and input_ids is not None:
_slen = slen - cache["slen"]
input_ids = input_ids[:, -_slen:]
position_ids = position_ids[:, -_slen:]
if langs is not None:
langs = langs[:, -_slen:]
mask = mask[:, -_slen:]
attn_mask = attn_mask[:, -_slen:]
# embeddings
if inputs_embeds is None:
check_embeddings_within_bounds(input_ids, self.embeddings.vocab_size)
inputs_embeds = self.embeddings(input_ids)
tensor = inputs_embeds + tf.gather(self.position_embeddings, position_ids)
if langs is not None and self.use_lang_emb and self.n_langs > 1:
tensor = tensor + tf.gather(self.lang_embeddings, langs)
if token_type_ids is not None:
tensor = tensor + self.embeddings(token_type_ids)
tensor = self.layer_norm_emb(tensor)
tensor = self.dropout(tensor, training=training)
mask = tf.cast(mask, dtype=tensor.dtype)
tensor = tensor * tf.expand_dims(mask, axis=-1)
# transformer layers
hidden_states = () if output_hidden_states else None
attentions = () if output_attentions else None
for i in range(self.n_layers):
if output_hidden_states:
hidden_states = hidden_states + (tensor,)
# self attention
attn_outputs = self.attentions[i](
tensor,
attn_mask,
None,
cache,
head_mask[i],
output_attentions,
training=training,
)
attn = attn_outputs[0]
if output_attentions:
attentions = attentions + (attn_outputs[1],)
attn = self.dropout(attn, training=training)
tensor = tensor + attn
tensor = self.layer_norm1[i](tensor)
# encoder attention (for decoder only)
# if self.is_decoder and src_enc is not None:
# attn = self.encoder_attn[i](tensor, src_mask, kv=src_enc, cache=cache)
# attn = nn.functional.dropout(attn, p=self.dropout, training=self.training)
# tensor = tensor + attn
# tensor = self.layer_norm15[i](tensor)
# FFN
tensor = tensor + self.ffns[i](tensor)
tensor = self.layer_norm2[i](tensor)
tensor = tensor * tf.expand_dims(mask, axis=-1)
# Add last hidden state
if output_hidden_states:
hidden_states = hidden_states + (tensor,)
# update cache length
if cache is not None:
cache["slen"] += tensor.size(1)
# move back sequence length to dimension 0
# tensor = tensor.transpose(0, 1)
if not return_dict:
return tuple(v for v in [tensor, hidden_states, attentions] if v is not None)
return TFBaseModelOutput(last_hidden_state=tensor, hidden_states=hidden_states, attentions=attentions)
class TFXLMPreTrainedModel(TFPreTrainedModel):
"""
An abstract class to handle weights initialization and a simple interface for downloading and loading pretrained
models.
"""
config_class = XLMConfig
base_model_prefix = "transformer"
@property
def dummy_inputs(self):
# Sometimes XLM has language embeddings so don't forget to build them as well if needed
inputs_list = tf.constant([[7, 6, 0, 0, 1], [1, 2, 3, 0, 0], [0, 0, 0, 4, 5]], dtype=tf.int32)
attns_list = tf.constant([[1, 1, 0, 0, 1], [1, 1, 1, 0, 0], [1, 0, 0, 1, 1]], dtype=tf.int32)
if self.config.use_lang_emb and self.config.n_langs > 1:
return {
"input_ids": inputs_list,
"attention_mask": attns_list,
"langs": tf.constant([[1, 1, 0, 0, 1], [1, 1, 1, 0, 0], [1, 0, 0, 1, 1]], dtype=tf.int32),
}
else:
return {"input_ids": inputs_list, "attention_mask": attns_list}
# Remove when XLMWithLMHead computes loss like other LM models
@dataclass
class TFXLMWithLMHeadModelOutput(ModelOutput):
"""
Base class for [`TFXLMWithLMHeadModel`] outputs.
Args:
logits (`tf.Tensor` of shape `(batch_size, sequence_length, config.vocab_size)`):
Prediction scores of the language modeling head (scores for each vocabulary token before SoftMax).
hidden_states (`tuple(tf.Tensor)`, *optional*, returned when `output_hidden_states=True` is passed or when `config.output_hidden_states=True`):
Tuple of `tf.Tensor` (one for the output of the embeddings + one for the output of each layer) of shape
`(batch_size, sequence_length, hidden_size)`.
Hidden-states of the model at the output of each layer plus the initial embedding outputs.
attentions (`tuple(tf.Tensor)`, *optional*, returned when `output_attentions=True` is passed or when `config.output_attentions=True`):
Tuple of `tf.Tensor` (one for each layer) of shape `(batch_size, num_heads, sequence_length,
sequence_length)`.
Attentions weights after the attention softmax, used to compute the weighted average in the self-attention
heads.
"""
logits: tf.Tensor = None
hidden_states: Tuple[tf.Tensor, ...] | None = None
attentions: Tuple[tf.Tensor, ...] | None = None
XLM_START_DOCSTRING = r"""
This model inherits from [`TFPreTrainedModel`]. Check the superclass documentation for the generic methods the
library implements for all its model (such as downloading or saving, resizing the input embeddings, pruning heads
etc.)
This model is also a [keras.Model](https://www.tensorflow.org/api_docs/python/tf/keras/Model) subclass. Use it
as a regular TF 2.0 Keras Model and refer to the TF 2.0 documentation for all matter related to general usage and
behavior.
<Tip>
TensorFlow models and layers in `transformers` accept two formats as input:
- having all inputs as keyword arguments (like PyTorch models), or
- having all inputs as a list, tuple or dict in the first positional argument.
The reason the second format is supported is that Keras methods prefer this format when passing inputs to models
and layers. Because of this support, when using methods like `model.fit()` things should "just work" for you - just
pass your inputs and labels in any format that `model.fit()` supports! If, however, you want to use the second
format outside of Keras methods like `fit()` and `predict()`, such as when creating your own layers or models with
the Keras `Functional` API, there are three possibilities you can use to gather all the input Tensors in the first
positional argument:
- a single Tensor with `input_ids` only and nothing else: `model(input_ids)`
- a list of varying length with one or several input Tensors IN THE ORDER given in the docstring:
`model([input_ids, attention_mask])` or `model([input_ids, attention_mask, token_type_ids])`
- a dictionary with one or several input Tensors associated to the input names given in the docstring:
`model({"input_ids": input_ids, "token_type_ids": token_type_ids})`
Note that when creating models and layers with
[subclassing](https://keras.io/guides/making_new_layers_and_models_via_subclassing/) then you don't need to worry
about any of this, as you can just pass inputs like you would to any other Python function!
</Tip>
Parameters:
config ([`XLMConfig`]): Model configuration class with all the parameters of the model.
Initializing with a config file does not load the weights associated with the model, only the
configuration. Check out the [`~PreTrainedModel.from_pretrained`] method to load the model weights.
"""
XLM_INPUTS_DOCSTRING = r"""
Args:
input_ids (`Numpy array` or `tf.Tensor` of shape `({0})`):
Indices of input sequence tokens in the vocabulary.
Indices can be obtained using [`AutoTokenizer`]. See [`PreTrainedTokenizer.__call__`] and
[`PreTrainedTokenizer.encode`] for details.
[What are input IDs?](../glossary#input-ids)
attention_mask (`Numpy array` or `tf.Tensor` of shape `({0})`, *optional*):
Mask to avoid performing attention on padding token indices. Mask values selected in `[0, 1]`:
- 1 for tokens that are **not masked**,
- 0 for tokens that are **masked**.
[What are attention masks?](../glossary#attention-mask)
langs (`tf.Tensor` or `Numpy array` of shape `({0})`, *optional*):
A parallel sequence of tokens to be used to indicate the language of each token in the input. Indices are
languages ids which can be obtained from the language names by using two conversion mappings provided in
the configuration of the model (only provided for multilingual models). More precisely, the *language name
to language id* mapping is in `model.config.lang2id` (which is a dictionary string to int) and the
*language id to language name* mapping is in `model.config.id2lang` (dictionary int to string).
See usage examples detailed in the [multilingual documentation](../multilingual).
token_type_ids (`Numpy array` or `tf.Tensor` of shape `({0})`, *optional*):
Segment token indices to indicate first and second portions of the inputs. Indices are selected in `[0,
1]`:
- 0 corresponds to a *sentence A* token,
- 1 corresponds to a *sentence B* token.
[What are token type IDs?](../glossary#token-type-ids)
position_ids (`Numpy array` or `tf.Tensor` of shape `({0})`, *optional*):
Indices of positions of each input sequence tokens in the position embeddings. Selected in the range `[0,
config.max_position_embeddings - 1]`.
[What are position IDs?](../glossary#position-ids)
lengths (`tf.Tensor` or `Numpy array` of shape `(batch_size,)`, *optional*):
Length of each sentence that can be used to avoid performing attention on padding token indices. You can
also use *attention_mask* for the same result (see above), kept here for compatibility. Indices selected in
`[0, ..., input_ids.size(-1)]`.
cache (`Dict[str, tf.Tensor]`, *optional*):
Dictionary string to `tf.Tensor` that contains precomputed hidden states (key and values in the attention
blocks) as computed by the model (see `cache` output below). Can be used to speed up sequential decoding.
The dictionary object will be modified in-place during the forward pass to add newly computed
hidden-states.
head_mask (`Numpy array` or `tf.Tensor` of shape `(num_heads,)` or `(num_layers, num_heads)`, *optional*):
Mask to nullify selected heads of the self-attention modules. Mask values selected in `[0, 1]`:
- 1 indicates the head is **not masked**,
- 0 indicates the head is **masked**.
inputs_embeds (`tf.Tensor` of shape `({0}, hidden_size)`, *optional*):
Optionally, instead of passing `input_ids` you can choose to directly pass an embedded representation. This
is useful if you want more control over how to convert `input_ids` indices into associated vectors than the
model's internal embedding lookup matrix.
output_attentions (`bool`, *optional*):
Whether or not to return the attentions tensors of all attention layers. See `attentions` under returned
tensors for more detail. This argument can be used only in eager mode, in graph mode the value in the
config will be used instead.
output_hidden_states (`bool`, *optional*):
Whether or not to return the hidden states of all layers. See `hidden_states` under returned tensors for
more detail. This argument can be used only in eager mode, in graph mode the value in the config will be
used instead.
return_dict (`bool`, *optional*):
Whether or not to return a [`~utils.ModelOutput`] instead of a plain tuple. This argument can be used in
eager mode, in graph mode the value will always be set to True.
training (`bool`, *optional*, defaults to `False`):
Whether or not to use the model in training mode (some modules like dropout modules have different
behaviors between training and evaluation).
"""
@add_start_docstrings(
"The bare XLM Model transformer outputting raw hidden-states without any specific head on top.",
XLM_START_DOCSTRING,
)
class TFXLMModel(TFXLMPreTrainedModel):
def __init__(self, config, *inputs, **kwargs):
super().__init__(config, *inputs, **kwargs)
self.transformer = TFXLMMainLayer(config, name="transformer")
@unpack_inputs
@add_start_docstrings_to_model_forward(XLM_INPUTS_DOCSTRING.format("batch_size, sequence_length"))
@add_code_sample_docstrings(
checkpoint=_CHECKPOINT_FOR_DOC,
output_type=TFBaseModelOutput,
config_class=_CONFIG_FOR_DOC,
)
def call(
self,
input_ids: TFModelInputType | None = None,
attention_mask: tf.Tensor | None = None,
langs: tf.Tensor | None = None,
token_type_ids: tf.Tensor | None = None,
position_ids: tf.Tensor | None = None,
lengths: tf.Tensor | None = None,
cache: Dict[str, tf.Tensor] | None = None,
head_mask: tf.Tensor | None = None,
inputs_embeds: tf.Tensor | None = None,
output_attentions: bool | None = None,
output_hidden_states: bool | None = None,
return_dict: bool | None = None,
training: bool = False,
) -> TFBaseModelOutput | Tuple[tf.Tensor]:
outputs = self.transformer(
input_ids=input_ids,
attention_mask=attention_mask,
langs=langs,
token_type_ids=token_type_ids,
position_ids=position_ids,
lengths=lengths,
cache=cache,
head_mask=head_mask,
inputs_embeds=inputs_embeds,
output_attentions=output_attentions,
output_hidden_states=output_hidden_states,
return_dict=return_dict,
training=training,
)
return outputs
def build(self, input_shape=None):
if self.built:
return
self.built = True
if getattr(self, "transformer", None) is not None:
with tf.name_scope(self.transformer.name):
self.transformer.build(None)
class TFXLMPredLayer(keras.layers.Layer):
"""
Prediction layer (cross_entropy or adaptive_softmax).
"""
def __init__(self, config, input_embeddings, **kwargs):
super().__init__(**kwargs)
self.asm = config.asm
self.n_words = config.n_words
self.pad_index = config.pad_index
if config.asm is False:
self.input_embeddings = input_embeddings
else:
raise NotImplementedError
# self.proj = nn.AdaptiveLogSoftmaxWithLoss(
# in_features=dim,
# n_classes=config.n_words,
# cutoffs=config.asm_cutoffs,
# div_value=config.asm_div_value,
# head_bias=True, # default is False
# )
def build(self, input_shape):
# The output weights are the same as the input embeddings, but there is an output-only bias for each token.
self.bias = self.add_weight(shape=(self.n_words,), initializer="zeros", trainable=True, name="bias")
super().build(input_shape)
def get_output_embeddings(self):
return self.input_embeddings
def set_output_embeddings(self, value):
self.input_embeddings.weight = value
self.input_embeddings.vocab_size = shape_list(value)[0]
def get_bias(self):
return {"bias": self.bias}
def set_bias(self, value):
self.bias = value["bias"]
self.vocab_size = shape_list(value["bias"])[0]
def call(self, hidden_states):
hidden_states = self.input_embeddings(hidden_states, mode="linear")
hidden_states = hidden_states + self.bias
return hidden_states
@add_start_docstrings(
"""
The XLM Model transformer with a language modeling head on top (linear layer with weights tied to the input
embeddings).
""",
XLM_START_DOCSTRING,
)
class TFXLMWithLMHeadModel(TFXLMPreTrainedModel):
def __init__(self, config, *inputs, **kwargs):
super().__init__(config, *inputs, **kwargs)
self.transformer = TFXLMMainLayer(config, name="transformer")
self.pred_layer = TFXLMPredLayer(config, self.transformer.embeddings, name="pred_layer_._proj")
# XLM does not have past caching features
self.supports_xla_generation = False
def get_lm_head(self):
return self.pred_layer
def get_prefix_bias_name(self):
warnings.warn("The method get_prefix_bias_name is deprecated. Please use `get_bias` instead.", FutureWarning)
return self.name + "/" + self.pred_layer.name
def prepare_inputs_for_generation(self, inputs, **kwargs):
mask_token_id = self.config.mask_token_id
lang_id = self.config.lang_id
effective_batch_size = inputs.shape[0]
mask_token = tf.fill((effective_batch_size, 1), 1) * mask_token_id
inputs = tf.concat([inputs, mask_token], axis=1)
if lang_id is not None:
langs = tf.ones_like(inputs) * lang_id
else:
langs = None
return {"input_ids": inputs, "langs": langs}
@unpack_inputs
@add_start_docstrings_to_model_forward(XLM_INPUTS_DOCSTRING.format("batch_size, sequence_length"))
@add_code_sample_docstrings(
checkpoint=_CHECKPOINT_FOR_DOC,
output_type=TFXLMWithLMHeadModelOutput,
config_class=_CONFIG_FOR_DOC,
)
def call(
self,
input_ids: TFModelInputType | None = None,
attention_mask: np.ndarray | tf.Tensor | None = None,
langs: np.ndarray | tf.Tensor | None = None,
token_type_ids: np.ndarray | tf.Tensor | None = None,
position_ids: np.ndarray | tf.Tensor | None = None,
lengths: np.ndarray | tf.Tensor | None = None,
cache: Optional[Dict[str, tf.Tensor]] = None,
head_mask: np.ndarray | tf.Tensor | None = None,
inputs_embeds: np.ndarray | tf.Tensor | None = None,
output_attentions: Optional[bool] = None,
output_hidden_states: Optional[bool] = None,
return_dict: Optional[bool] = None,
training: bool = False,
) -> Union[TFXLMWithLMHeadModelOutput, Tuple[tf.Tensor]]:
transformer_outputs = self.transformer(
input_ids=input_ids,
attention_mask=attention_mask,
langs=langs,
token_type_ids=token_type_ids,
position_ids=position_ids,
lengths=lengths,
cache=cache,
head_mask=head_mask,
inputs_embeds=inputs_embeds,
output_attentions=output_attentions,
output_hidden_states=output_hidden_states,
return_dict=return_dict,
training=training,
)
output = transformer_outputs[0]
outputs = self.pred_layer(output)
if not return_dict:
return (outputs,) + transformer_outputs[1:]
return TFXLMWithLMHeadModelOutput(
logits=outputs, hidden_states=transformer_outputs.hidden_states, attentions=transformer_outputs.attentions
)
def build(self, input_shape=None):
if self.built:
return
self.built = True
if getattr(self, "transformer", None) is not None:
with tf.name_scope(self.transformer.name):
self.transformer.build(None)
if getattr(self, "pred_layer", None) is not None:
with tf.name_scope(self.pred_layer.name):
self.pred_layer.build(None)
@add_start_docstrings(
"""
XLM Model with a sequence classification/regression head on top (a linear layer on top of the pooled output) e.g.
for GLUE tasks.
""",
XLM_START_DOCSTRING,
)
class TFXLMForSequenceClassification(TFXLMPreTrainedModel, TFSequenceClassificationLoss):
def __init__(self, config, *inputs, **kwargs):
super().__init__(config, *inputs, **kwargs)
self.num_labels = config.num_labels
self.transformer = TFXLMMainLayer(config, name="transformer")
self.sequence_summary = TFSequenceSummary(config, initializer_range=config.init_std, name="sequence_summary")
@unpack_inputs
@add_start_docstrings_to_model_forward(XLM_INPUTS_DOCSTRING.format("batch_size, sequence_length"))
@add_code_sample_docstrings(
checkpoint=_CHECKPOINT_FOR_DOC,
output_type=TFSequenceClassifierOutput,
config_class=_CONFIG_FOR_DOC,
)
def call(
self,
input_ids: TFModelInputType | None = None,
attention_mask: np.ndarray | tf.Tensor | None = None,
langs: np.ndarray | tf.Tensor | None = None,
token_type_ids: np.ndarray | tf.Tensor | None = None,
position_ids: np.ndarray | tf.Tensor | None = None,
lengths: np.ndarray | tf.Tensor | None = None,
cache: Optional[Dict[str, tf.Tensor]] = None,
head_mask: np.ndarray | tf.Tensor | None = None,
inputs_embeds: np.ndarray | tf.Tensor | None = None,
output_attentions: Optional[bool] = None,
output_hidden_states: Optional[bool] = None,
return_dict: Optional[bool] = None,
labels: np.ndarray | tf.Tensor | None = None,
training: bool = False,
) -> Union[TFSequenceClassifierOutput, Tuple[tf.Tensor]]:
r"""
labels (`tf.Tensor` of shape `(batch_size,)`, *optional*):
Labels for computing the sequence classification/regression loss. Indices should be in `[0, ...,
config.num_labels - 1]`. If `config.num_labels == 1` a regression loss is computed (Mean-Square loss), If
`config.num_labels > 1` a classification loss is computed (Cross-Entropy).
"""
transformer_outputs = self.transformer(
input_ids=input_ids,
attention_mask=attention_mask,
langs=langs,
token_type_ids=token_type_ids,
position_ids=position_ids,
lengths=lengths,
cache=cache,
head_mask=head_mask,
inputs_embeds=inputs_embeds,
output_attentions=output_attentions,
output_hidden_states=output_hidden_states,
return_dict=return_dict,
training=training,
)
output = transformer_outputs[0]
logits = self.sequence_summary(output)
loss = None if labels is None else self.hf_compute_loss(labels, logits)
if not return_dict:
output = (logits,) + transformer_outputs[1:]
return ((loss,) + output) if loss is not None else output
return TFSequenceClassifierOutput(
loss=loss,
logits=logits,
hidden_states=transformer_outputs.hidden_states,
attentions=transformer_outputs.attentions,
)
def build(self, input_shape=None):
if self.built:
return
self.built = True
if getattr(self, "transformer", None) is not None:
with tf.name_scope(self.transformer.name):
self.transformer.build(None)
if getattr(self, "sequence_summary", None) is not None:
with tf.name_scope(self.sequence_summary.name):
self.sequence_summary.build(None)
@add_start_docstrings(
"""
XLM Model with a multiple choice classification head on top (a linear layer on top of the pooled output and a
softmax) e.g. for RocStories/SWAG tasks.
""",
XLM_START_DOCSTRING,
)
class TFXLMForMultipleChoice(TFXLMPreTrainedModel, TFMultipleChoiceLoss):
def __init__(self, config, *inputs, **kwargs):
super().__init__(config, *inputs, **kwargs)
self.transformer = TFXLMMainLayer(config, name="transformer")
self.sequence_summary = TFSequenceSummary(config, initializer_range=config.init_std, name="sequence_summary")
self.logits_proj = keras.layers.Dense(
1, kernel_initializer=get_initializer(config.initializer_range), name="logits_proj"
)
self.config = config
@property
def dummy_inputs(self):
"""
Dummy inputs to build the network.
Returns:
tf.Tensor with dummy inputs
"""
# Sometimes XLM has language embeddings so don't forget to build them as well if needed
if self.config.use_lang_emb and self.config.n_langs > 1:
return {
"input_ids": tf.constant(MULTIPLE_CHOICE_DUMMY_INPUTS, dtype=tf.int32),
"langs": tf.constant(MULTIPLE_CHOICE_DUMMY_INPUTS, dtype=tf.int32),
}
else:
return {
"input_ids": tf.constant(MULTIPLE_CHOICE_DUMMY_INPUTS, dtype=tf.int32),
}
@unpack_inputs
@add_start_docstrings_to_model_forward(XLM_INPUTS_DOCSTRING.format("batch_size, num_choices, sequence_length"))
@add_code_sample_docstrings(
checkpoint=_CHECKPOINT_FOR_DOC,
output_type=TFMultipleChoiceModelOutput,
config_class=_CONFIG_FOR_DOC,
)
def call(
self,
input_ids: TFModelInputType | None = None,
attention_mask: np.ndarray | tf.Tensor | None = None,
langs: np.ndarray | tf.Tensor | None = None,
token_type_ids: np.ndarray | tf.Tensor | None = None,
position_ids: np.ndarray | tf.Tensor | None = None,
lengths: np.ndarray | tf.Tensor | None = None,
cache: Optional[Dict[str, tf.Tensor]] = None,
head_mask: np.ndarray | tf.Tensor | None = None,
inputs_embeds: np.ndarray | tf.Tensor | None = None,
output_attentions: Optional[bool] = None,
output_hidden_states: Optional[bool] = None,
return_dict: Optional[bool] = None,
labels: np.ndarray | tf.Tensor | None = None,
training: bool = False,
) -> Union[TFMultipleChoiceModelOutput, Tuple[tf.Tensor]]:
if input_ids is not None:
num_choices = shape_list(input_ids)[1]
seq_length = shape_list(input_ids)[2]
else:
num_choices = shape_list(inputs_embeds)[1]
seq_length = shape_list(inputs_embeds)[2]
flat_input_ids = tf.reshape(input_ids, (-1, seq_length)) if input_ids is not None else None
flat_attention_mask = tf.reshape(attention_mask, (-1, seq_length)) if attention_mask is not None else None
flat_token_type_ids = tf.reshape(token_type_ids, (-1, seq_length)) if token_type_ids is not None else None
flat_position_ids = tf.reshape(position_ids, (-1, seq_length)) if position_ids is not None else None
flat_langs = tf.reshape(langs, (-1, seq_length)) if langs is not None else None
flat_inputs_embeds = (
tf.reshape(inputs_embeds, (-1, seq_length, shape_list(inputs_embeds)[3]))
if inputs_embeds is not None
else None
)
if lengths is not None:
logger.warning(
"The `lengths` parameter cannot be used with the XLM multiple choice models. Please use the "
"attention mask instead.",
)
lengths = None
transformer_outputs = self.transformer(
flat_input_ids,
flat_attention_mask,
flat_langs,
flat_token_type_ids,
flat_position_ids,
lengths,
cache,
head_mask,
flat_inputs_embeds,
output_attentions,
output_hidden_states,
return_dict=return_dict,
training=training,
)
output = transformer_outputs[0]
logits = self.sequence_summary(output)
logits = self.logits_proj(logits)
reshaped_logits = tf.reshape(logits, (-1, num_choices))
loss = None if labels is None else self.hf_compute_loss(labels, reshaped_logits)
if not return_dict:
output = (reshaped_logits,) + transformer_outputs[1:]
return ((loss,) + output) if loss is not None else output
return TFMultipleChoiceModelOutput(
loss=loss,
logits=reshaped_logits,
hidden_states=transformer_outputs.hidden_states,
attentions=transformer_outputs.attentions,
)
def build(self, input_shape=None):
if self.built:
return
self.built = True
if getattr(self, "transformer", None) is not None:
with tf.name_scope(self.transformer.name):
self.transformer.build(None)
if getattr(self, "sequence_summary", None) is not None:
with tf.name_scope(self.sequence_summary.name):
self.sequence_summary.build(None)
if getattr(self, "logits_proj", None) is not None:
with tf.name_scope(self.logits_proj.name):
self.logits_proj.build([None, None, self.config.num_labels])
@add_start_docstrings(
"""
XLM Model with a token classification head on top (a linear layer on top of the hidden-states output) e.g. for
Named-Entity-Recognition (NER) tasks.
""",
XLM_START_DOCSTRING,
)
class TFXLMForTokenClassification(TFXLMPreTrainedModel, TFTokenClassificationLoss):
def __init__(self, config, *inputs, **kwargs):
super().__init__(config, *inputs, **kwargs)
self.num_labels = config.num_labels
self.transformer = TFXLMMainLayer(config, name="transformer")
self.dropout = keras.layers.Dropout(config.dropout)
self.classifier = keras.layers.Dense(
config.num_labels, kernel_initializer=get_initializer(config.init_std), name="classifier"
)
self.config = config
@unpack_inputs
@add_start_docstrings_to_model_forward(XLM_INPUTS_DOCSTRING.format("batch_size, sequence_length"))
@add_code_sample_docstrings(
checkpoint=_CHECKPOINT_FOR_DOC,
output_type=TFTokenClassifierOutput,
config_class=_CONFIG_FOR_DOC,
)
def call(
self,
input_ids: TFModelInputType | None = None,
attention_mask: np.ndarray | tf.Tensor | None = None,
langs: np.ndarray | tf.Tensor | None = None,
token_type_ids: np.ndarray | tf.Tensor | None = None,
position_ids: np.ndarray | tf.Tensor | None = None,
lengths: np.ndarray | tf.Tensor | None = None,
cache: Optional[Dict[str, tf.Tensor]] = None,
head_mask: np.ndarray | tf.Tensor | None = None,
inputs_embeds: np.ndarray | tf.Tensor | None = None,
output_attentions: Optional[bool] = None,
output_hidden_states: Optional[bool] = None,
return_dict: Optional[bool] = None,
labels: np.ndarray | tf.Tensor | None = None,
training: bool = False,
) -> Union[TFTokenClassifierOutput, Tuple[tf.Tensor]]:
r"""
labels (`tf.Tensor` of shape `(batch_size, sequence_length)`, *optional*):
Labels for computing the token classification loss. Indices should be in `[0, ..., config.num_labels - 1]`.
"""
transformer_outputs = self.transformer(
input_ids=input_ids,
attention_mask=attention_mask,
langs=langs,
token_type_ids=token_type_ids,
position_ids=position_ids,
lengths=lengths,
cache=cache,
head_mask=head_mask,
inputs_embeds=inputs_embeds,
output_attentions=output_attentions,
output_hidden_states=output_hidden_states,
return_dict=return_dict,
training=training,
)
sequence_output = transformer_outputs[0]
sequence_output = self.dropout(sequence_output, training=training)
logits = self.classifier(sequence_output)
loss = None if labels is None else self.hf_compute_loss(labels, logits)
if not return_dict:
output = (logits,) + transformer_outputs[1:]
return ((loss,) + output) if loss is not None else output
return TFTokenClassifierOutput(
loss=loss,
logits=logits,
hidden_states=transformer_outputs.hidden_states,
attentions=transformer_outputs.attentions,
)
def build(self, input_shape=None):
if self.built:
return
self.built = True
if getattr(self, "transformer", None) is not None:
with tf.name_scope(self.transformer.name):
self.transformer.build(None)
if getattr(self, "classifier", None) is not None:
with tf.name_scope(self.classifier.name):
self.classifier.build([None, None, self.config.hidden_size])
@add_start_docstrings(
"""
XLM Model with a span classification head on top for extractive question-answering tasks like SQuAD (a linear layer
on top of the hidden-states output to compute `span start logits` and `span end logits`).
""",
XLM_START_DOCSTRING,
)
class TFXLMForQuestionAnsweringSimple(TFXLMPreTrainedModel, TFQuestionAnsweringLoss):
def __init__(self, config, *inputs, **kwargs):
super().__init__(config, *inputs, **kwargs)
self.transformer = TFXLMMainLayer(config, name="transformer")
self.qa_outputs = keras.layers.Dense(
config.num_labels, kernel_initializer=get_initializer(config.init_std), name="qa_outputs"
)
self.config = config
@unpack_inputs
@add_start_docstrings_to_model_forward(XLM_INPUTS_DOCSTRING.format("batch_size, sequence_length"))
@add_code_sample_docstrings(
checkpoint=_CHECKPOINT_FOR_DOC,
output_type=TFQuestionAnsweringModelOutput,
config_class=_CONFIG_FOR_DOC,
)
def call(
self,
input_ids: TFModelInputType | None = None,
attention_mask: np.ndarray | tf.Tensor | None = None,
langs: np.ndarray | tf.Tensor | None = None,
token_type_ids: np.ndarray | tf.Tensor | None = None,
position_ids: np.ndarray | tf.Tensor | None = None,
lengths: np.ndarray | tf.Tensor | None = None,
cache: Optional[Dict[str, tf.Tensor]] = None,
head_mask: np.ndarray | tf.Tensor | None = None,
inputs_embeds: np.ndarray | tf.Tensor | None = None,
output_attentions: Optional[bool] = None,
output_hidden_states: Optional[bool] = None,
return_dict: Optional[bool] = None,
start_positions: np.ndarray | tf.Tensor | None = None,
end_positions: np.ndarray | tf.Tensor | None = None,
training: bool = False,
) -> Union[TFQuestionAnsweringModelOutput, Tuple[tf.Tensor]]:
r"""
start_positions (`tf.Tensor` of shape `(batch_size,)`, *optional*):
Labels for position (index) of the start of the labelled span for computing the token classification loss.
Positions are clamped to the length of the sequence (`sequence_length`). Position outside of the sequence
are not taken into account for computing the loss.
end_positions (`tf.Tensor` of shape `(batch_size,)`, *optional*):
Labels for position (index) of the end of the labelled span for computing the token classification loss.
Positions are clamped to the length of the sequence (`sequence_length`). Position outside of the sequence
are not taken into account for computing the loss.
"""
transformer_outputs = self.transformer(
input_ids=input_ids,
attention_mask=attention_mask,
langs=langs,
token_type_ids=token_type_ids,
position_ids=position_ids,
lengths=lengths,
cache=cache,
head_mask=head_mask,
inputs_embeds=inputs_embeds,
output_attentions=output_attentions,
output_hidden_states=output_hidden_states,
return_dict=return_dict,
training=training,
)
sequence_output = transformer_outputs[0]
logits = self.qa_outputs(sequence_output)
start_logits, end_logits = tf.split(logits, 2, axis=-1)
start_logits = tf.squeeze(start_logits, axis=-1)
end_logits = tf.squeeze(end_logits, axis=-1)
loss = None
if start_positions is not None and end_positions is not None:
labels = {"start_position": start_positions}
labels["end_position"] = end_positions
loss = self.hf_compute_loss(labels, (start_logits, end_logits))
if not return_dict:
output = (start_logits, end_logits) + transformer_outputs[1:]
return ((loss,) + output) if loss is not None else output
return TFQuestionAnsweringModelOutput(
loss=loss,
start_logits=start_logits,
end_logits=end_logits,
hidden_states=transformer_outputs.hidden_states,
attentions=transformer_outputs.attentions,
)
def build(self, input_shape=None):
if self.built:
return
self.built = True
if getattr(self, "transformer", None) is not None:
with tf.name_scope(self.transformer.name):
self.transformer.build(None)
if getattr(self, "qa_outputs", None) is not None:
with tf.name_scope(self.qa_outputs.name):
self.qa_outputs.build([None, None, self.config.hidden_size])
| transformers/src/transformers/models/xlm/modeling_tf_xlm.py/0 | {
"file_path": "transformers/src/transformers/models/xlm/modeling_tf_xlm.py",
"repo_id": "transformers",
"token_count": 25192
} | 333 |
# coding=utf-8
# Copyright 2018 The HuggingFace Inc. team.
#
# Licensed under the Apache License, Version 2.0 (the "License");
# you may not use this file except in compliance with the License.
# You may obtain a copy of the License at
#
# http://www.apache.org/licenses/LICENSE-2.0
#
# Unless required by applicable law or agreed to in writing, software
# distributed under the License is distributed on an "AS IS" BASIS,
# WITHOUT WARRANTIES OR CONDITIONS OF ANY KIND, either express or implied.
# See the License for the specific language governing permissions and
# limitations under the License.
"""Convert RoBERTa checkpoint."""
import argparse
import pathlib
import fairseq
import torch
from fairseq.models.roberta import RobertaModel as FairseqRobertaModel
from fairseq.modules import TransformerSentenceEncoderLayer
from packaging import version
from transformers import XLMRobertaConfig, XLMRobertaXLForMaskedLM, XLMRobertaXLForSequenceClassification
from transformers.models.bert.modeling_bert import (
BertIntermediate,
BertLayer,
BertOutput,
BertSelfAttention,
BertSelfOutput,
)
from transformers.models.roberta.modeling_roberta import RobertaAttention
from transformers.utils import logging
if version.parse(fairseq.__version__) < version.parse("1.0.0a"):
raise Exception("requires fairseq >= 1.0.0a")
logging.set_verbosity_info()
logger = logging.get_logger(__name__)
SAMPLE_TEXT = "Hello world! cécé herlolip"
def convert_xlm_roberta_xl_checkpoint_to_pytorch(
roberta_checkpoint_path: str, pytorch_dump_folder_path: str, classification_head: bool
):
"""
Copy/paste/tweak roberta's weights to our BERT structure.
"""
roberta = FairseqRobertaModel.from_pretrained(roberta_checkpoint_path)
roberta.eval() # disable dropout
roberta_sent_encoder = roberta.model.encoder.sentence_encoder
config = XLMRobertaConfig(
vocab_size=roberta_sent_encoder.embed_tokens.num_embeddings,
hidden_size=roberta.cfg.model.encoder_embed_dim,
num_hidden_layers=roberta.cfg.model.encoder_layers,
num_attention_heads=roberta.cfg.model.encoder_attention_heads,
intermediate_size=roberta.cfg.model.encoder_ffn_embed_dim,
max_position_embeddings=514,
type_vocab_size=1,
layer_norm_eps=1e-5, # PyTorch default used in fairseq
)
if classification_head:
config.num_labels = roberta.model.classification_heads["mnli"].out_proj.weight.shape[0]
print("Our RoBERTa config:", config)
model = XLMRobertaXLForSequenceClassification(config) if classification_head else XLMRobertaXLForMaskedLM(config)
model.eval()
# Now let's copy all the weights.
# Embeddings
model.roberta.embeddings.word_embeddings.weight = roberta_sent_encoder.embed_tokens.weight
model.roberta.embeddings.position_embeddings.weight = roberta_sent_encoder.embed_positions.weight
model.roberta.embeddings.token_type_embeddings.weight.data = torch.zeros_like(
model.roberta.embeddings.token_type_embeddings.weight
) # just zero them out b/c RoBERTa doesn't use them.
model.roberta.encoder.LayerNorm.weight = roberta_sent_encoder.layer_norm.weight
model.roberta.encoder.LayerNorm.bias = roberta_sent_encoder.layer_norm.bias
for i in range(config.num_hidden_layers):
# Encoder: start of layer
layer: BertLayer = model.roberta.encoder.layer[i]
roberta_layer: TransformerSentenceEncoderLayer = roberta_sent_encoder.layers[i]
attention: RobertaAttention = layer.attention
attention.self_attn_layer_norm.weight = roberta_layer.self_attn_layer_norm.weight
attention.self_attn_layer_norm.bias = roberta_layer.self_attn_layer_norm.bias
# self attention
self_attn: BertSelfAttention = layer.attention.self
assert (
roberta_layer.self_attn.k_proj.weight.data.shape
== roberta_layer.self_attn.q_proj.weight.data.shape
== roberta_layer.self_attn.v_proj.weight.data.shape
== torch.Size((config.hidden_size, config.hidden_size))
)
self_attn.query.weight.data = roberta_layer.self_attn.q_proj.weight
self_attn.query.bias.data = roberta_layer.self_attn.q_proj.bias
self_attn.key.weight.data = roberta_layer.self_attn.k_proj.weight
self_attn.key.bias.data = roberta_layer.self_attn.k_proj.bias
self_attn.value.weight.data = roberta_layer.self_attn.v_proj.weight
self_attn.value.bias.data = roberta_layer.self_attn.v_proj.bias
# self-attention output
self_output: BertSelfOutput = layer.attention.output
assert self_output.dense.weight.shape == roberta_layer.self_attn.out_proj.weight.shape
self_output.dense.weight = roberta_layer.self_attn.out_proj.weight
self_output.dense.bias = roberta_layer.self_attn.out_proj.bias
# this one is final layer norm
layer.LayerNorm.weight = roberta_layer.final_layer_norm.weight
layer.LayerNorm.bias = roberta_layer.final_layer_norm.bias
# intermediate
intermediate: BertIntermediate = layer.intermediate
assert intermediate.dense.weight.shape == roberta_layer.fc1.weight.shape
intermediate.dense.weight = roberta_layer.fc1.weight
intermediate.dense.bias = roberta_layer.fc1.bias
# output
bert_output: BertOutput = layer.output
assert bert_output.dense.weight.shape == roberta_layer.fc2.weight.shape
bert_output.dense.weight = roberta_layer.fc2.weight
bert_output.dense.bias = roberta_layer.fc2.bias
# end of layer
if classification_head:
model.classifier.dense.weight = roberta.model.classification_heads["mnli"].dense.weight
model.classifier.dense.bias = roberta.model.classification_heads["mnli"].dense.bias
model.classifier.out_proj.weight = roberta.model.classification_heads["mnli"].out_proj.weight
model.classifier.out_proj.bias = roberta.model.classification_heads["mnli"].out_proj.bias
else:
# LM Head
model.lm_head.dense.weight = roberta.model.encoder.lm_head.dense.weight
model.lm_head.dense.bias = roberta.model.encoder.lm_head.dense.bias
model.lm_head.layer_norm.weight = roberta.model.encoder.lm_head.layer_norm.weight
model.lm_head.layer_norm.bias = roberta.model.encoder.lm_head.layer_norm.bias
model.lm_head.decoder.weight = roberta.model.encoder.lm_head.weight
model.lm_head.decoder.bias = roberta.model.encoder.lm_head.bias
# Let's check that we get the same results.
input_ids: torch.Tensor = roberta.encode(SAMPLE_TEXT).unsqueeze(0) # batch of size 1
our_output = model(input_ids)[0]
if classification_head:
their_output = roberta.model.classification_heads["mnli"](roberta.extract_features(input_ids))
else:
their_output = roberta.model(input_ids)[0]
print(our_output.shape, their_output.shape)
max_absolute_diff = torch.max(torch.abs(our_output - their_output)).item()
print(f"max_absolute_diff = {max_absolute_diff}") # ~ 1e-7
success = torch.allclose(our_output, their_output, atol=1e-3)
print("Do both models output the same tensors?", "🔥" if success else "💩")
if not success:
raise Exception("Something went wRoNg")
pathlib.Path(pytorch_dump_folder_path).mkdir(parents=True, exist_ok=True)
print(f"Saving model to {pytorch_dump_folder_path}")
model.save_pretrained(pytorch_dump_folder_path)
if __name__ == "__main__":
parser = argparse.ArgumentParser()
# Required parameters
parser.add_argument(
"--roberta_checkpoint_path", default=None, type=str, required=True, help="Path the official PyTorch dump."
)
parser.add_argument(
"--pytorch_dump_folder_path", default=None, type=str, required=True, help="Path to the output PyTorch model."
)
parser.add_argument(
"--classification_head", action="store_true", help="Whether to convert a final classification head."
)
args = parser.parse_args()
convert_xlm_roberta_xl_checkpoint_to_pytorch(
args.roberta_checkpoint_path, args.pytorch_dump_folder_path, args.classification_head
)
| transformers/src/transformers/models/xlm_roberta_xl/convert_xlm_roberta_xl_original_pytorch_checkpoint_to_pytorch.py/0 | {
"file_path": "transformers/src/transformers/models/xlm_roberta_xl/convert_xlm_roberta_xl_original_pytorch_checkpoint_to_pytorch.py",
"repo_id": "transformers",
"token_count": 3308
} | 334 |
# Copyright 2021 The HuggingFace Team. All rights reserved.
#
# Licensed under the Apache License, Version 2.0 (the "License");
# you may not use this file except in compliance with the License.
# You may obtain a copy of the License at
#
# http://www.apache.org/licenses/LICENSE-2.0
#
# Unless required by applicable law or agreed to in writing, software
# distributed under the License is distributed on an "AS IS" BASIS,
# WITHOUT WARRANTIES OR CONDITIONS OF ANY KIND, either express or implied.
# See the License for the specific language governing permissions and
# limitations under the License.
import subprocess
from typing import Union
import numpy as np
import requests
from ..utils import add_end_docstrings, is_torch_available, is_torchaudio_available, logging
from .base import Pipeline, build_pipeline_init_args
if is_torch_available():
from ..models.auto.modeling_auto import MODEL_FOR_AUDIO_CLASSIFICATION_MAPPING_NAMES
logger = logging.get_logger(__name__)
def ffmpeg_read(bpayload: bytes, sampling_rate: int) -> np.array:
"""
Helper function to read an audio file through ffmpeg.
"""
ar = f"{sampling_rate}"
ac = "1"
format_for_conversion = "f32le"
ffmpeg_command = [
"ffmpeg",
"-i",
"pipe:0",
"-ac",
ac,
"-ar",
ar,
"-f",
format_for_conversion,
"-hide_banner",
"-loglevel",
"quiet",
"pipe:1",
]
try:
ffmpeg_process = subprocess.Popen(ffmpeg_command, stdin=subprocess.PIPE, stdout=subprocess.PIPE)
except FileNotFoundError:
raise ValueError("ffmpeg was not found but is required to load audio files from filename")
output_stream = ffmpeg_process.communicate(bpayload)
out_bytes = output_stream[0]
audio = np.frombuffer(out_bytes, np.float32)
if audio.shape[0] == 0:
raise ValueError("Malformed soundfile")
return audio
@add_end_docstrings(build_pipeline_init_args(has_feature_extractor=True))
class AudioClassificationPipeline(Pipeline):
"""
Audio classification pipeline using any `AutoModelForAudioClassification`. This pipeline predicts the class of a
raw waveform or an audio file. In case of an audio file, ffmpeg should be installed to support multiple audio
formats.
Example:
```python
>>> from transformers import pipeline
>>> classifier = pipeline(model="superb/wav2vec2-base-superb-ks")
>>> classifier("https://huggingface.co/datasets/Narsil/asr_dummy/resolve/main/1.flac")
[{'score': 0.997, 'label': '_unknown_'}, {'score': 0.002, 'label': 'left'}, {'score': 0.0, 'label': 'yes'}, {'score': 0.0, 'label': 'down'}, {'score': 0.0, 'label': 'stop'}]
```
Learn more about the basics of using a pipeline in the [pipeline tutorial](../pipeline_tutorial)
This pipeline can currently be loaded from [`pipeline`] using the following task identifier:
`"audio-classification"`.
See the list of available models on
[huggingface.co/models](https://huggingface.co/models?filter=audio-classification).
"""
def __init__(self, *args, **kwargs):
# Default, might be overriden by the model.config.
kwargs["top_k"] = 5
super().__init__(*args, **kwargs)
if self.framework != "pt":
raise ValueError(f"The {self.__class__} is only available in PyTorch.")
self.check_model_type(MODEL_FOR_AUDIO_CLASSIFICATION_MAPPING_NAMES)
def __call__(
self,
inputs: Union[np.ndarray, bytes, str],
**kwargs,
):
"""
Classify the sequence(s) given as inputs. See the [`AutomaticSpeechRecognitionPipeline`] documentation for more
information.
Args:
inputs (`np.ndarray` or `bytes` or `str` or `dict`):
The inputs is either :
- `str` that is the filename of the audio file, the file will be read at the correct sampling rate
to get the waveform using *ffmpeg*. This requires *ffmpeg* to be installed on the system.
- `bytes` it is supposed to be the content of an audio file and is interpreted by *ffmpeg* in the
same way.
- (`np.ndarray` of shape (n, ) of type `np.float32` or `np.float64`)
Raw audio at the correct sampling rate (no further check will be done)
- `dict` form can be used to pass raw audio sampled at arbitrary `sampling_rate` and let this
pipeline do the resampling. The dict must be either be in the format `{"sampling_rate": int,
"raw": np.array}`, or `{"sampling_rate": int, "array": np.array}`, where the key `"raw"` or
`"array"` is used to denote the raw audio waveform.
top_k (`int`, *optional*, defaults to None):
The number of top labels that will be returned by the pipeline. If the provided number is `None` or
higher than the number of labels available in the model configuration, it will default to the number of
labels.
Return:
A list of `dict` with the following keys:
- **label** (`str`) -- The label predicted.
- **score** (`float`) -- The corresponding probability.
"""
return super().__call__(inputs, **kwargs)
def _sanitize_parameters(self, top_k=None, **kwargs):
# No parameters on this pipeline right now
postprocess_params = {}
if top_k is not None:
if top_k > self.model.config.num_labels:
top_k = self.model.config.num_labels
postprocess_params["top_k"] = top_k
return {}, {}, postprocess_params
def preprocess(self, inputs):
if isinstance(inputs, str):
if inputs.startswith("http://") or inputs.startswith("https://"):
# We need to actually check for a real protocol, otherwise it's impossible to use a local file
# like http_huggingface_co.png
inputs = requests.get(inputs).content
else:
with open(inputs, "rb") as f:
inputs = f.read()
if isinstance(inputs, bytes):
inputs = ffmpeg_read(inputs, self.feature_extractor.sampling_rate)
if isinstance(inputs, dict):
# Accepting `"array"` which is the key defined in `datasets` for
# better integration
if not ("sampling_rate" in inputs and ("raw" in inputs or "array" in inputs)):
raise ValueError(
"When passing a dictionary to AudioClassificationPipeline, the dict needs to contain a "
'"raw" key containing the numpy array representing the audio and a "sampling_rate" key, '
"containing the sampling_rate associated with that array"
)
_inputs = inputs.pop("raw", None)
if _inputs is None:
# Remove path which will not be used from `datasets`.
inputs.pop("path", None)
_inputs = inputs.pop("array", None)
in_sampling_rate = inputs.pop("sampling_rate")
inputs = _inputs
if in_sampling_rate != self.feature_extractor.sampling_rate:
import torch
if is_torchaudio_available():
from torchaudio import functional as F
else:
raise ImportError(
"torchaudio is required to resample audio samples in AudioClassificationPipeline. "
"The torchaudio package can be installed through: `pip install torchaudio`."
)
inputs = F.resample(
torch.from_numpy(inputs), in_sampling_rate, self.feature_extractor.sampling_rate
).numpy()
if not isinstance(inputs, np.ndarray):
raise ValueError("We expect a numpy ndarray as input")
if len(inputs.shape) != 1:
raise ValueError("We expect a single channel audio input for AudioClassificationPipeline")
processed = self.feature_extractor(
inputs, sampling_rate=self.feature_extractor.sampling_rate, return_tensors="pt"
)
return processed
def _forward(self, model_inputs):
model_outputs = self.model(**model_inputs)
return model_outputs
def postprocess(self, model_outputs, top_k=5):
probs = model_outputs.logits[0].softmax(-1)
scores, ids = probs.topk(top_k)
scores = scores.tolist()
ids = ids.tolist()
labels = [{"score": score, "label": self.model.config.id2label[_id]} for score, _id in zip(scores, ids)]
return labels
| transformers/src/transformers/pipelines/audio_classification.py/0 | {
"file_path": "transformers/src/transformers/pipelines/audio_classification.py",
"repo_id": "transformers",
"token_count": 3711
} | 335 |
import inspect
import types
import warnings
from collections.abc import Iterable
from typing import TYPE_CHECKING, Dict, List, Optional, Tuple, Union
import numpy as np
from ..data import SquadExample, SquadFeatures, squad_convert_examples_to_features
from ..modelcard import ModelCard
from ..tokenization_utils import PreTrainedTokenizer
from ..utils import (
PaddingStrategy,
add_end_docstrings,
is_tf_available,
is_tokenizers_available,
is_torch_available,
logging,
)
from .base import ArgumentHandler, ChunkPipeline, build_pipeline_init_args
logger = logging.get_logger(__name__)
if TYPE_CHECKING:
from ..modeling_tf_utils import TFPreTrainedModel
from ..modeling_utils import PreTrainedModel
if is_tokenizers_available():
import tokenizers
if is_tf_available():
import tensorflow as tf
from ..models.auto.modeling_tf_auto import TF_MODEL_FOR_QUESTION_ANSWERING_MAPPING_NAMES
Dataset = None
if is_torch_available():
import torch
from torch.utils.data import Dataset
from ..models.auto.modeling_auto import MODEL_FOR_QUESTION_ANSWERING_MAPPING_NAMES
def decode_spans(
start: np.ndarray, end: np.ndarray, topk: int, max_answer_len: int, undesired_tokens: np.ndarray
) -> Tuple:
"""
Take the output of any `ModelForQuestionAnswering` and will generate probabilities for each span to be the actual
answer.
In addition, it filters out some unwanted/impossible cases like answer len being greater than max_answer_len or
answer end position being before the starting position. The method supports output the k-best answer through the
topk argument.
Args:
start (`np.ndarray`): Individual start probabilities for each token.
end (`np.ndarray`): Individual end probabilities for each token.
topk (`int`): Indicates how many possible answer span(s) to extract from the model output.
max_answer_len (`int`): Maximum size of the answer to extract from the model's output.
undesired_tokens (`np.ndarray`): Mask determining tokens that can be part of the answer
"""
# Ensure we have batch axis
if start.ndim == 1:
start = start[None]
if end.ndim == 1:
end = end[None]
# Compute the score of each tuple(start, end) to be the real answer
outer = np.matmul(np.expand_dims(start, -1), np.expand_dims(end, 1))
# Remove candidate with end < start and end - start > max_answer_len
candidates = np.tril(np.triu(outer), max_answer_len - 1)
# Inspired by Chen & al. (https://github.com/facebookresearch/DrQA)
scores_flat = candidates.flatten()
if topk == 1:
idx_sort = [np.argmax(scores_flat)]
elif len(scores_flat) < topk:
idx_sort = np.argsort(-scores_flat)
else:
idx = np.argpartition(-scores_flat, topk)[0:topk]
idx_sort = idx[np.argsort(-scores_flat[idx])]
starts, ends = np.unravel_index(idx_sort, candidates.shape)[1:]
desired_spans = np.isin(starts, undesired_tokens.nonzero()) & np.isin(ends, undesired_tokens.nonzero())
starts = starts[desired_spans]
ends = ends[desired_spans]
scores = candidates[0, starts, ends]
return starts, ends, scores
def select_starts_ends(
start,
end,
p_mask,
attention_mask,
min_null_score=1000000,
top_k=1,
handle_impossible_answer=False,
max_answer_len=15,
):
"""
Takes the raw output of any `ModelForQuestionAnswering` and first normalizes its outputs and then uses
`decode_spans()` to generate probabilities for each span to be the actual answer.
Args:
start (`np.ndarray`): Individual start logits for each token.
end (`np.ndarray`): Individual end logits for each token.
p_mask (`np.ndarray`): A mask with 1 for values that cannot be in the answer
attention_mask (`np.ndarray`): The attention mask generated by the tokenizer
min_null_score(`float`): The minimum null (empty) answer score seen so far.
topk (`int`): Indicates how many possible answer span(s) to extract from the model output.
handle_impossible_answer(`bool`): Whether to allow null (empty) answers
max_answer_len (`int`): Maximum size of the answer to extract from the model's output.
"""
# Ensure padded tokens & question tokens cannot belong to the set of candidate answers.
undesired_tokens = np.abs(np.array(p_mask) - 1)
if attention_mask is not None:
undesired_tokens = undesired_tokens & attention_mask
# Generate mask
undesired_tokens_mask = undesired_tokens == 0.0
# Make sure non-context indexes in the tensor cannot contribute to the softmax
start = np.where(undesired_tokens_mask, -10000.0, start)
end = np.where(undesired_tokens_mask, -10000.0, end)
# Normalize logits and spans to retrieve the answer
start = np.exp(start - start.max(axis=-1, keepdims=True))
start = start / start.sum()
end = np.exp(end - end.max(axis=-1, keepdims=True))
end = end / end.sum()
if handle_impossible_answer:
min_null_score = min(min_null_score, (start[0, 0] * end[0, 0]).item())
# Mask CLS
start[0, 0] = end[0, 0] = 0.0
starts, ends, scores = decode_spans(start, end, top_k, max_answer_len, undesired_tokens)
return starts, ends, scores, min_null_score
class QuestionAnsweringArgumentHandler(ArgumentHandler):
"""
QuestionAnsweringPipeline requires the user to provide multiple arguments (i.e. question & context) to be mapped to
internal [`SquadExample`].
QuestionAnsweringArgumentHandler manages all the possible to create a [`SquadExample`] from the command-line
supplied arguments.
"""
def normalize(self, item):
if isinstance(item, SquadExample):
return item
elif isinstance(item, dict):
for k in ["question", "context"]:
if k not in item:
raise KeyError("You need to provide a dictionary with keys {question:..., context:...}")
elif item[k] is None:
raise ValueError(f"`{k}` cannot be None")
elif isinstance(item[k], str) and len(item[k]) == 0:
raise ValueError(f"`{k}` cannot be empty")
return QuestionAnsweringPipeline.create_sample(**item)
raise ValueError(f"{item} argument needs to be of type (SquadExample, dict)")
def __call__(self, *args, **kwargs):
# Detect where the actual inputs are
if args is not None and len(args) > 0:
if len(args) == 1:
inputs = args[0]
elif len(args) == 2 and {type(el) for el in args} == {str}:
inputs = [{"question": args[0], "context": args[1]}]
else:
inputs = list(args)
# Generic compatibility with sklearn and Keras
# Batched data
elif "X" in kwargs:
inputs = kwargs["X"]
elif "data" in kwargs:
inputs = kwargs["data"]
elif "question" in kwargs and "context" in kwargs:
if isinstance(kwargs["question"], list) and isinstance(kwargs["context"], str):
inputs = [{"question": Q, "context": kwargs["context"]} for Q in kwargs["question"]]
elif isinstance(kwargs["question"], list) and isinstance(kwargs["context"], list):
if len(kwargs["question"]) != len(kwargs["context"]):
raise ValueError("Questions and contexts don't have the same lengths")
inputs = [{"question": Q, "context": C} for Q, C in zip(kwargs["question"], kwargs["context"])]
elif isinstance(kwargs["question"], str) and isinstance(kwargs["context"], str):
inputs = [{"question": kwargs["question"], "context": kwargs["context"]}]
else:
raise ValueError("Arguments can't be understood")
else:
raise ValueError(f"Unknown arguments {kwargs}")
# When user is sending a generator we need to trust it's a valid example
generator_types = (types.GeneratorType, Dataset) if Dataset is not None else (types.GeneratorType,)
if isinstance(inputs, generator_types):
return inputs
# Normalize inputs
if isinstance(inputs, dict):
inputs = [inputs]
elif isinstance(inputs, Iterable):
# Copy to avoid overriding arguments
inputs = list(inputs)
else:
raise ValueError(f"Invalid arguments {kwargs}")
for i, item in enumerate(inputs):
inputs[i] = self.normalize(item)
return inputs
@add_end_docstrings(build_pipeline_init_args(has_tokenizer=True))
class QuestionAnsweringPipeline(ChunkPipeline):
"""
Question Answering pipeline using any `ModelForQuestionAnswering`. See the [question answering
examples](../task_summary#question-answering) for more information.
Example:
```python
>>> from transformers import pipeline
>>> oracle = pipeline(model="deepset/roberta-base-squad2")
>>> oracle(question="Where do I live?", context="My name is Wolfgang and I live in Berlin")
{'score': 0.9191, 'start': 34, 'end': 40, 'answer': 'Berlin'}
```
Learn more about the basics of using a pipeline in the [pipeline tutorial](../pipeline_tutorial)
This question answering pipeline can currently be loaded from [`pipeline`] using the following task identifier:
`"question-answering"`.
The models that this pipeline can use are models that have been fine-tuned on a question answering task. See the
up-to-date list of available models on
[huggingface.co/models](https://huggingface.co/models?filter=question-answering).
"""
default_input_names = "question,context"
handle_impossible_answer = False
def __init__(
self,
model: Union["PreTrainedModel", "TFPreTrainedModel"],
tokenizer: PreTrainedTokenizer,
modelcard: Optional[ModelCard] = None,
framework: Optional[str] = None,
task: str = "",
**kwargs,
):
super().__init__(
model=model,
tokenizer=tokenizer,
modelcard=modelcard,
framework=framework,
task=task,
**kwargs,
)
self._args_parser = QuestionAnsweringArgumentHandler()
self.check_model_type(
TF_MODEL_FOR_QUESTION_ANSWERING_MAPPING_NAMES
if self.framework == "tf"
else MODEL_FOR_QUESTION_ANSWERING_MAPPING_NAMES
)
@staticmethod
def create_sample(
question: Union[str, List[str]], context: Union[str, List[str]]
) -> Union[SquadExample, List[SquadExample]]:
"""
QuestionAnsweringPipeline leverages the [`SquadExample`] internally. This helper method encapsulate all the
logic for converting question(s) and context(s) to [`SquadExample`].
We currently support extractive question answering.
Arguments:
question (`str` or `List[str]`): The question(s) asked.
context (`str` or `List[str]`): The context(s) in which we will look for the answer.
Returns:
One or a list of [`SquadExample`]: The corresponding [`SquadExample`] grouping question and context.
"""
if isinstance(question, list):
return [SquadExample(None, q, c, None, None, None) for q, c in zip(question, context)]
else:
return SquadExample(None, question, context, None, None, None)
def _sanitize_parameters(
self,
padding=None,
topk=None,
top_k=None,
doc_stride=None,
max_answer_len=None,
max_seq_len=None,
max_question_len=None,
handle_impossible_answer=None,
align_to_words=None,
**kwargs,
):
# Set defaults values
preprocess_params = {}
if padding is not None:
preprocess_params["padding"] = padding
if doc_stride is not None:
preprocess_params["doc_stride"] = doc_stride
if max_question_len is not None:
preprocess_params["max_question_len"] = max_question_len
if max_seq_len is not None:
preprocess_params["max_seq_len"] = max_seq_len
postprocess_params = {}
if topk is not None and top_k is None:
warnings.warn("topk parameter is deprecated, use top_k instead", UserWarning)
top_k = topk
if top_k is not None:
if top_k < 1:
raise ValueError(f"top_k parameter should be >= 1 (got {top_k})")
postprocess_params["top_k"] = top_k
if max_answer_len is not None:
if max_answer_len < 1:
raise ValueError(f"max_answer_len parameter should be >= 1 (got {max_answer_len}")
if max_answer_len is not None:
postprocess_params["max_answer_len"] = max_answer_len
if handle_impossible_answer is not None:
postprocess_params["handle_impossible_answer"] = handle_impossible_answer
if align_to_words is not None:
postprocess_params["align_to_words"] = align_to_words
return preprocess_params, {}, postprocess_params
def __call__(self, *args, **kwargs):
"""
Answer the question(s) given as inputs by using the context(s).
Args:
args ([`SquadExample`] or a list of [`SquadExample`]):
One or several [`SquadExample`] containing the question and context.
X ([`SquadExample`] or a list of [`SquadExample`], *optional*):
One or several [`SquadExample`] containing the question and context (will be treated the same way as if
passed as the first positional argument).
data ([`SquadExample`] or a list of [`SquadExample`], *optional*):
One or several [`SquadExample`] containing the question and context (will be treated the same way as if
passed as the first positional argument).
question (`str` or `List[str]`):
One or several question(s) (must be used in conjunction with the `context` argument).
context (`str` or `List[str]`):
One or several context(s) associated with the question(s) (must be used in conjunction with the
`question` argument).
topk (`int`, *optional*, defaults to 1):
The number of answers to return (will be chosen by order of likelihood). Note that we return less than
topk answers if there are not enough options available within the context.
doc_stride (`int`, *optional*, defaults to 128):
If the context is too long to fit with the question for the model, it will be split in several chunks
with some overlap. This argument controls the size of that overlap.
max_answer_len (`int`, *optional*, defaults to 15):
The maximum length of predicted answers (e.g., only answers with a shorter length are considered).
max_seq_len (`int`, *optional*, defaults to 384):
The maximum length of the total sentence (context + question) in tokens of each chunk passed to the
model. The context will be split in several chunks (using `doc_stride` as overlap) if needed.
max_question_len (`int`, *optional*, defaults to 64):
The maximum length of the question after tokenization. It will be truncated if needed.
handle_impossible_answer (`bool`, *optional*, defaults to `False`):
Whether or not we accept impossible as an answer.
align_to_words (`bool`, *optional*, defaults to `True`):
Attempts to align the answer to real words. Improves quality on space separated langages. Might hurt on
non-space-separated languages (like Japanese or Chinese)
Return:
A `dict` or a list of `dict`: Each result comes as a dictionary with the following keys:
- **score** (`float`) -- The probability associated to the answer.
- **start** (`int`) -- The character start index of the answer (in the tokenized version of the input).
- **end** (`int`) -- The character end index of the answer (in the tokenized version of the input).
- **answer** (`str`) -- The answer to the question.
"""
# Convert inputs to features
examples = self._args_parser(*args, **kwargs)
if isinstance(examples, (list, tuple)) and len(examples) == 1:
return super().__call__(examples[0], **kwargs)
return super().__call__(examples, **kwargs)
def preprocess(self, example, padding="do_not_pad", doc_stride=None, max_question_len=64, max_seq_len=None):
# XXX: This is specal, args_parser will not handle anything generator or dataset like
# For those we expect user to send a simple valid example either directly as a SquadExample or simple dict.
# So we still need a little sanitation here.
if isinstance(example, dict):
example = SquadExample(None, example["question"], example["context"], None, None, None)
if max_seq_len is None:
max_seq_len = min(self.tokenizer.model_max_length, 384)
if doc_stride is None:
doc_stride = min(max_seq_len // 2, 128)
if doc_stride > max_seq_len:
raise ValueError(f"`doc_stride` ({doc_stride}) is larger than `max_seq_len` ({max_seq_len})")
if not self.tokenizer.is_fast:
features = squad_convert_examples_to_features(
examples=[example],
tokenizer=self.tokenizer,
max_seq_length=max_seq_len,
doc_stride=doc_stride,
max_query_length=max_question_len,
padding_strategy=PaddingStrategy.MAX_LENGTH,
is_training=False,
tqdm_enabled=False,
)
else:
# Define the side we want to truncate / pad and the text/pair sorting
question_first = self.tokenizer.padding_side == "right"
encoded_inputs = self.tokenizer(
text=example.question_text if question_first else example.context_text,
text_pair=example.context_text if question_first else example.question_text,
padding=padding,
truncation="only_second" if question_first else "only_first",
max_length=max_seq_len,
stride=doc_stride,
return_token_type_ids=True,
return_overflowing_tokens=True,
return_offsets_mapping=True,
return_special_tokens_mask=True,
)
# When the input is too long, it's converted in a batch of inputs with overflowing tokens
# and a stride of overlap between the inputs. If a batch of inputs is given, a special output
# "overflow_to_sample_mapping" indicate which member of the encoded batch belong to which original batch sample.
# Here we tokenize examples one-by-one so we don't need to use "overflow_to_sample_mapping".
# "num_span" is the number of output samples generated from the overflowing tokens.
num_spans = len(encoded_inputs["input_ids"])
# p_mask: mask with 1 for token than cannot be in the answer (0 for token which can be in an answer)
# We put 0 on the tokens from the context and 1 everywhere else (question and special tokens)
p_mask = [
[tok != 1 if question_first else 0 for tok in encoded_inputs.sequence_ids(span_id)]
for span_id in range(num_spans)
]
features = []
for span_idx in range(num_spans):
input_ids_span_idx = encoded_inputs["input_ids"][span_idx]
attention_mask_span_idx = (
encoded_inputs["attention_mask"][span_idx] if "attention_mask" in encoded_inputs else None
)
token_type_ids_span_idx = (
encoded_inputs["token_type_ids"][span_idx] if "token_type_ids" in encoded_inputs else None
)
# keep the cls_token unmasked (some models use it to indicate unanswerable questions)
if self.tokenizer.cls_token_id is not None:
cls_indices = np.nonzero(np.array(input_ids_span_idx) == self.tokenizer.cls_token_id)[0]
for cls_index in cls_indices:
p_mask[span_idx][cls_index] = 0
submask = p_mask[span_idx]
features.append(
SquadFeatures(
input_ids=input_ids_span_idx,
attention_mask=attention_mask_span_idx,
token_type_ids=token_type_ids_span_idx,
p_mask=submask,
encoding=encoded_inputs[span_idx],
# We don't use the rest of the values - and actually
# for Fast tokenizer we could totally avoid using SquadFeatures and SquadExample
cls_index=None,
token_to_orig_map={},
example_index=0,
unique_id=0,
paragraph_len=0,
token_is_max_context=0,
tokens=[],
start_position=0,
end_position=0,
is_impossible=False,
qas_id=None,
)
)
for i, feature in enumerate(features):
fw_args = {}
others = {}
model_input_names = self.tokenizer.model_input_names + ["p_mask", "token_type_ids"]
for k, v in feature.__dict__.items():
if k in model_input_names:
if self.framework == "tf":
tensor = tf.constant(v)
if tensor.dtype == tf.int64:
tensor = tf.cast(tensor, tf.int32)
fw_args[k] = tf.expand_dims(tensor, 0)
elif self.framework == "pt":
tensor = torch.tensor(v)
if tensor.dtype == torch.int32:
tensor = tensor.long()
fw_args[k] = tensor.unsqueeze(0)
else:
others[k] = v
is_last = i == len(features) - 1
yield {"example": example, "is_last": is_last, **fw_args, **others}
def _forward(self, inputs):
example = inputs["example"]
model_inputs = {k: inputs[k] for k in self.tokenizer.model_input_names}
# `XXXForSequenceClassification` models should not use `use_cache=True` even if it's supported
model_forward = self.model.forward if self.framework == "pt" else self.model.call
if "use_cache" in inspect.signature(model_forward).parameters.keys():
model_inputs["use_cache"] = False
output = self.model(**model_inputs)
if isinstance(output, dict):
return {"start": output["start_logits"], "end": output["end_logits"], "example": example, **inputs}
else:
start, end = output[:2]
return {"start": start, "end": end, "example": example, **inputs}
def postprocess(
self,
model_outputs,
top_k=1,
handle_impossible_answer=False,
max_answer_len=15,
align_to_words=True,
):
min_null_score = 1000000 # large and positive
answers = []
for output in model_outputs:
start_ = output["start"]
end_ = output["end"]
example = output["example"]
p_mask = output["p_mask"]
attention_mask = (
output["attention_mask"].numpy() if output.get("attention_mask", None) is not None else None
)
starts, ends, scores, min_null_score = select_starts_ends(
start_, end_, p_mask, attention_mask, min_null_score, top_k, handle_impossible_answer, max_answer_len
)
if not self.tokenizer.is_fast:
char_to_word = np.array(example.char_to_word_offset)
# Convert the answer (tokens) back to the original text
# Score: score from the model
# Start: Index of the first character of the answer in the context string
# End: Index of the character following the last character of the answer in the context string
# Answer: Plain text of the answer
for s, e, score in zip(starts, ends, scores):
token_to_orig_map = output["token_to_orig_map"]
answers.append(
{
"score": score.item(),
"start": np.where(char_to_word == token_to_orig_map[s])[0][0].item(),
"end": np.where(char_to_word == token_to_orig_map[e])[0][-1].item(),
"answer": " ".join(example.doc_tokens[token_to_orig_map[s] : token_to_orig_map[e] + 1]),
}
)
else:
# Convert the answer (tokens) back to the original text
# Score: score from the model
# Start: Index of the first character of the answer in the context string
# End: Index of the character following the last character of the answer in the context string
# Answer: Plain text of the answer
question_first = bool(self.tokenizer.padding_side == "right")
enc = output["encoding"]
# Encoding was *not* padded, input_ids *might*.
# It doesn't make a difference unless we're padding on
# the left hand side, since now we have different offsets
# everywhere.
if self.tokenizer.padding_side == "left":
offset = (output["input_ids"] == self.tokenizer.pad_token_id).numpy().sum()
else:
offset = 0
# Sometimes the max probability token is in the middle of a word so:
# - we start by finding the right word containing the token with `token_to_word`
# - then we convert this word in a character span with `word_to_chars`
sequence_index = 1 if question_first else 0
for s, e, score in zip(starts, ends, scores):
s = s - offset
e = e - offset
start_index, end_index = self.get_indices(enc, s, e, sequence_index, align_to_words)
answers.append(
{
"score": score.item(),
"start": start_index,
"end": end_index,
"answer": example.context_text[start_index:end_index],
}
)
if handle_impossible_answer:
answers.append({"score": min_null_score, "start": 0, "end": 0, "answer": ""})
answers = sorted(answers, key=lambda x: x["score"], reverse=True)[:top_k]
if len(answers) == 1:
return answers[0]
return answers
def get_indices(
self, enc: "tokenizers.Encoding", s: int, e: int, sequence_index: int, align_to_words: bool
) -> Tuple[int, int]:
if align_to_words:
try:
start_word = enc.token_to_word(s)
end_word = enc.token_to_word(e)
start_index = enc.word_to_chars(start_word, sequence_index=sequence_index)[0]
end_index = enc.word_to_chars(end_word, sequence_index=sequence_index)[1]
except Exception:
# Some tokenizers don't really handle words. Keep to offsets then.
start_index = enc.offsets[s][0]
end_index = enc.offsets[e][1]
else:
start_index = enc.offsets[s][0]
end_index = enc.offsets[e][1]
return start_index, end_index
def span_to_answer(self, text: str, start: int, end: int) -> Dict[str, Union[str, int]]:
"""
When decoding from token probabilities, this method maps token indexes to actual word in the initial context.
Args:
text (`str`): The actual context to extract the answer from.
start (`int`): The answer starting token index.
end (`int`): The answer end token index.
Returns:
Dictionary like `{'answer': str, 'start': int, 'end': int}`
"""
words = []
token_idx = char_start_idx = char_end_idx = chars_idx = 0
for i, word in enumerate(text.split(" ")):
token = self.tokenizer.tokenize(word)
# Append words if they are in the span
if start <= token_idx <= end:
if token_idx == start:
char_start_idx = chars_idx
if token_idx == end:
char_end_idx = chars_idx + len(word)
words += [word]
# Stop if we went over the end of the answer
if token_idx > end:
break
# Append the subtokenization length to the running index
token_idx += len(token)
chars_idx += len(word) + 1
# Join text with spaces
return {
"answer": " ".join(words),
"start": max(0, char_start_idx),
"end": min(len(text), char_end_idx),
}
| transformers/src/transformers/pipelines/question_answering.py/0 | {
"file_path": "transformers/src/transformers/pipelines/question_answering.py",
"repo_id": "transformers",
"token_count": 13330
} | 336 |
# Copyright 2024 The HuggingFace Inc. team. All rights reserved.
#
# Licensed under the Apache License, Version 2.0 (the "License");
# you may not use this file except in compliance with the License.
# You may obtain a copy of the License at
#
# http://www.apache.org/licenses/LICENSE-2.0
#
# Unless required by applicable law or agreed to in writing, software
# distributed under the License is distributed on an "AS IS" BASIS,
# WITHOUT WARRANTIES OR CONDITIONS OF ANY KIND, either express or implied.
# See the License for the specific language governing permissions and
# limitations under the License.
import warnings
from typing import Dict, Optional, Union
from ..models.auto.configuration_auto import AutoConfig
from ..utils.quantization_config import (
AwqConfig,
BitsAndBytesConfig,
GPTQConfig,
QuantizationConfigMixin,
QuantizationMethod,
)
from .quantizer_awq import AwqQuantizer
from .quantizer_bnb_4bit import Bnb4BitHfQuantizer
from .quantizer_bnb_8bit import Bnb8BitHfQuantizer
from .quantizer_gptq import GptqHfQuantizer
AUTO_QUANTIZER_MAPPING = {
"awq": AwqQuantizer,
"bitsandbytes_4bit": Bnb4BitHfQuantizer,
"bitsandbytes_8bit": Bnb8BitHfQuantizer,
"gptq": GptqHfQuantizer,
}
AUTO_QUANTIZATION_CONFIG_MAPPING = {
"awq": AwqConfig,
"bitsandbytes_4bit": BitsAndBytesConfig,
"bitsandbytes_8bit": BitsAndBytesConfig,
"gptq": GPTQConfig,
}
class AutoQuantizationConfig:
"""
The Auto-HF quantization config class that takes care of automatically dispatching to the correct
quantization config given a quantization config stored in a dictionary.
"""
@classmethod
def from_dict(cls, quantization_config_dict: Dict):
quant_method = quantization_config_dict.get("quant_method", None)
# We need a special care for bnb models to make sure everything is BC ..
if quantization_config_dict.get("load_in_8bit", False) or quantization_config_dict.get("load_in_4bit", False):
suffix = "_4bit" if quantization_config_dict.get("load_in_4bit", False) else "_8bit"
quant_method = QuantizationMethod.BITS_AND_BYTES + suffix
elif quant_method is None:
raise ValueError(
"The model's quantization config from the arguments has no `quant_method` attribute. Make sure that the model has been correctly quantized"
)
if quant_method not in AUTO_QUANTIZATION_CONFIG_MAPPING.keys():
raise ValueError(
f"Unknown quantization type, got {quant_method} - supported types are:"
f" {list(AUTO_QUANTIZER_MAPPING.keys())}"
)
target_cls = AUTO_QUANTIZATION_CONFIG_MAPPING[quant_method]
return target_cls.from_dict(quantization_config_dict)
@classmethod
def from_pretrained(cls, pretrained_model_name_or_path, **kwargs):
model_config = AutoConfig.from_pretrained(pretrained_model_name_or_path, **kwargs)
if getattr(model_config, "quantization_config", None) is None:
raise ValueError(
f"Did not found a `quantization_config` in {pretrained_model_name_or_path}. Make sure that the model is correctly quantized."
)
quantization_config_dict = model_config.quantization_config
quantization_config = cls.from_dict(quantization_config_dict)
# Update with potential kwargs that are passed through from_pretrained.
quantization_config.update(kwargs)
return quantization_config
class AutoHfQuantizer:
"""
The Auto-HF quantizer class that takes care of automatically instantiating to the correct
`HfQuantizer` given the `QuantizationConfig`.
"""
@classmethod
def from_config(cls, quantization_config: Union[QuantizationConfigMixin, Dict], **kwargs):
# Convert it to a QuantizationConfig if the q_config is a dict
if isinstance(quantization_config, dict):
quantization_config = AutoQuantizationConfig.from_dict(quantization_config)
quant_method = quantization_config.quant_method
# Again, we need a special care for bnb as we have a single quantization config
# class for both 4-bit and 8-bit quantization
if quant_method == QuantizationMethod.BITS_AND_BYTES:
if quantization_config.load_in_8bit:
quant_method += "_8bit"
else:
quant_method += "_4bit"
if quant_method not in AUTO_QUANTIZER_MAPPING.keys():
raise ValueError(
f"Unknown quantization type, got {quant_method} - supported types are:"
f" {list(AUTO_QUANTIZER_MAPPING.keys())}"
)
target_cls = AUTO_QUANTIZER_MAPPING[quant_method]
return target_cls(quantization_config, **kwargs)
@classmethod
def from_pretrained(cls, pretrained_model_name_or_path, **kwargs):
quantization_config = AutoQuantizationConfig.from_pretrained(pretrained_model_name_or_path, **kwargs)
return cls.from_config(quantization_config)
@classmethod
def merge_quantization_configs(
cls,
quantization_config: Union[dict, QuantizationConfigMixin],
quantization_config_from_args: Optional[QuantizationConfigMixin],
):
"""
handles situations where both quantization_config from args and quantization_config from model config are present.
"""
warning_msg = (
"You passed `quantization_config` or equivalent parameters to `from_pretrained` but the model you're loading"
" already has a `quantization_config` attribute. The `quantization_config` from the model will be prevail."
)
if isinstance(quantization_config, dict):
quantization_config = AutoQuantizationConfig.from_dict(quantization_config)
if isinstance(quantization_config, (GPTQConfig, AwqConfig)) and quantization_config_from_args is not None:
# special case for GPTQ / AWQ config collision
loading_attr_dict = quantization_config_from_args.get_loading_attributes()
for attr, val in loading_attr_dict.items():
setattr(quantization_config, attr, val)
warning_msg += f"However, loading attributes (e.g. {list(loading_attr_dict.keys())}) will be overwritten with the one you passed to `from_pretrained`. The rest will be ignored."
warnings.warn(warning_msg)
return quantization_config
| transformers/src/transformers/quantizers/auto.py/0 | {
"file_path": "transformers/src/transformers/quantizers/auto.py",
"repo_id": "transformers",
"token_count": 2443
} | 337 |
# coding=utf-8
# Copyright 2020 The HuggingFace Inc. team.
#
# Licensed under the Apache License, Version 2.0 (the "License");
# you may not use this file except in compliance with the License.
# You may obtain a copy of the License at
#
# http://www.apache.org/licenses/LICENSE-2.0
#
# Unless required by applicable law or agreed to in writing, software
# distributed under the License is distributed on an "AS IS" BASIS,
# WITHOUT WARRANTIES OR CONDITIONS OF ANY KIND, either express or implied.
# See the License for the specific language governing permissions and
# limitations under the License.
"""
Tokenization classes for fast tokenizers (provided by HuggingFace's tokenizers library). For slow (python) tokenizers
see tokenization_utils.py
"""
import copy
import json
import os
from collections import defaultdict
from typing import Any, Dict, List, Optional, Tuple, Union
import tokenizers.pre_tokenizers as pre_tokenizers_fast
from tokenizers import Encoding as EncodingFast
from tokenizers import Tokenizer as TokenizerFast
from tokenizers.decoders import Decoder as DecoderFast
from tokenizers.trainers import BpeTrainer, UnigramTrainer, WordLevelTrainer, WordPieceTrainer
from .convert_slow_tokenizer import convert_slow_tokenizer
from .tokenization_utils import PreTrainedTokenizer
from .tokenization_utils_base import (
INIT_TOKENIZER_DOCSTRING,
AddedToken,
BatchEncoding,
PreTokenizedInput,
PreTokenizedInputPair,
PreTrainedTokenizerBase,
SpecialTokensMixin,
TextInput,
TextInputPair,
TruncationStrategy,
)
from .utils import PaddingStrategy, add_end_docstrings, logging
logger = logging.get_logger(__name__)
# Fast tokenizers (provided by HuggingFace tokenizer's library) can be saved in a single file
TOKENIZER_FILE = "tokenizer.json"
SPECIAL_TOKENS_MAP_FILE = "special_tokens_map.json"
TOKENIZER_CONFIG_FILE = "tokenizer_config.json"
# Slow tokenizers have an additional added tokens files
ADDED_TOKENS_FILE = "added_tokens.json"
INIT_TOKENIZER_DOCSTRING += """
tokenizer_object ([`tokenizers.Tokenizer`]):
A [`tokenizers.Tokenizer`] object from 🤗 tokenizers to instantiate from. See [Using tokenizers from 🤗
tokenizers](../fast_tokenizers) for more information.
tokenizer_file ([`str`]):
A path to a local JSON file representing a previously serialized [`tokenizers.Tokenizer`] object from 🤗
tokenizers.
"""
MODEL_TO_TRAINER_MAPPING = {
"BPE": BpeTrainer,
"Unigram": UnigramTrainer,
"WordLevel": WordLevelTrainer,
"WordPiece": WordPieceTrainer,
}
VOCAB_FILES_NAMES = {"tokenizer_file": TOKENIZER_FILE}
@add_end_docstrings(INIT_TOKENIZER_DOCSTRING)
class PreTrainedTokenizerFast(PreTrainedTokenizerBase):
"""
Base class for all fast tokenizers (wrapping HuggingFace tokenizers library).
Inherits from [`~tokenization_utils_base.PreTrainedTokenizerBase`].
Handles all the shared methods for tokenization and special tokens, as well as methods for
downloading/caching/loading pretrained tokenizers, as well as adding tokens to the vocabulary.
This class also contains the added tokens in a unified way on top of all tokenizers so we don't have to handle the
specific vocabulary augmentation methods of the various underlying dictionary structures (BPE, sentencepiece...).
"""
vocab_files_names = VOCAB_FILES_NAMES
slow_tokenizer_class: PreTrainedTokenizer = None
def __init__(self, *args, **kwargs):
tokenizer_object = kwargs.pop("tokenizer_object", None)
slow_tokenizer = kwargs.pop("__slow_tokenizer", None)
fast_tokenizer_file = kwargs.pop("tokenizer_file", None)
from_slow = kwargs.pop("from_slow", False)
added_tokens_decoder = kwargs.pop("added_tokens_decoder", {})
if from_slow and slow_tokenizer is None and self.slow_tokenizer_class is None:
raise ValueError(
"Cannot instantiate this tokenizer from a slow version. If it's based on sentencepiece, make sure you "
"have sentencepiece installed."
)
if tokenizer_object is not None:
fast_tokenizer = copy.deepcopy(tokenizer_object)
elif fast_tokenizer_file is not None and not from_slow:
# We have a serialization from tokenizers which let us directly build the backend
fast_tokenizer = TokenizerFast.from_file(fast_tokenizer_file)
elif slow_tokenizer is not None:
# We need to convert a slow tokenizer to build the backend
fast_tokenizer = convert_slow_tokenizer(slow_tokenizer)
elif self.slow_tokenizer_class is not None:
# We need to create and convert a slow tokenizer to build the backend
slow_tokenizer = self.slow_tokenizer_class(*args, **kwargs)
fast_tokenizer = convert_slow_tokenizer(slow_tokenizer)
else:
raise ValueError(
"Couldn't instantiate the backend tokenizer from one of: \n"
"(1) a `tokenizers` library serialization file, \n"
"(2) a slow tokenizer instance to convert or \n"
"(3) an equivalent slow tokenizer class to instantiate and convert. \n"
"You need to have sentencepiece installed to convert a slow tokenizer to a fast one."
)
self._tokenizer = fast_tokenizer
if slow_tokenizer is not None:
kwargs.update(slow_tokenizer.init_kwargs)
self._decode_use_source_tokenizer = False
_truncation = self._tokenizer.truncation
if _truncation is not None:
self._tokenizer.enable_truncation(**_truncation)
kwargs.setdefault("max_length", _truncation["max_length"])
kwargs.setdefault("truncation_side", _truncation["direction"])
kwargs.setdefault("stride", _truncation["stride"])
kwargs.setdefault("truncation_strategy", _truncation["strategy"])
else:
self._tokenizer.no_truncation()
_padding = self._tokenizer.padding
if _padding is not None:
self._tokenizer.enable_padding(**_padding)
kwargs.setdefault("pad_token", _padding["pad_token"])
kwargs.setdefault("pad_token_type_id", _padding["pad_type_id"])
kwargs.setdefault("padding_side", _padding["direction"])
kwargs.setdefault("max_length", _padding["length"])
kwargs.setdefault("pad_to_multiple_of", _padding["pad_to_multiple_of"])
# We call this after having initialized the backend tokenizer because we update it.
super().__init__(**kwargs)
# The following logic will be replace with a single add_tokens once a fix is pushed to tokenizers
# allows converting a slow -> fast, non-legacy: if the `tokenizer.json` does not have all the added tokens
# uses the information stored in `added_tokens_decoder`.
# this is costly for fast tokenizers as we re-compute the regex again. But not all tokens are added tokens
tokens_to_add = [
token
for index, token in sorted(added_tokens_decoder.items(), key=lambda x: x[0])
if token not in self.added_tokens_decoder
]
encoder = list(self.added_tokens_encoder.keys()) + [str(token) for token in tokens_to_add]
# if some of the special tokens are strings, we check if we don't already have a token
tokens_to_add += [
token for token in self.all_special_tokens_extended if token not in encoder and token not in tokens_to_add
]
if len(tokens_to_add) > 0:
# super hack: if a token.special is set, tokenizer ignores it for now so FIXME @ArthurZ
# Accumulate added tokens into batches of special/non-special tokens, because calling add_tokens() for
# individual tokens would repeatedly rebuild a trie, which can be slow.
is_last_special = None
tokens = []
special_tokens = self.all_special_tokens
for token in tokens_to_add:
is_special = (
(token.special or str(token) in special_tokens)
if isinstance(token, AddedToken)
else str(token) in special_tokens
)
if is_last_special is None or is_last_special == is_special:
tokens.append(token)
else:
self._add_tokens(tokens, special_tokens=is_last_special)
tokens = [token]
is_last_special = is_special
if tokens:
self._add_tokens(tokens, special_tokens=is_last_special)
@property
def is_fast(self) -> bool:
return True
@property
def can_save_slow_tokenizer(self) -> bool:
"""
`bool`: Whether or not the slow tokenizer can be saved. Usually for sentencepiece based slow tokenizer, this
can only be `True` if the original `"sentencepiece.model"` was not deleted.
"""
return True
@property
def vocab_size(self) -> int:
"""
`int`: Size of the base vocabulary (without the added tokens).
"""
return self._tokenizer.get_vocab_size(with_added_tokens=False)
def get_vocab(self) -> Dict[str, int]:
return self._tokenizer.get_vocab(with_added_tokens=True)
@property
def vocab(self) -> Dict[str, int]:
return self.get_vocab()
@property
def added_tokens_encoder(self) -> Dict[str, int]:
"""
Returns the sorted mapping from string to index. The added tokens encoder is cached for performance
optimisation in `self._added_tokens_encoder` for the slow tokenizers.
"""
return {k.content: v for v, k in sorted(self.added_tokens_decoder.items(), key=lambda item: item[0])}
@property
def added_tokens_decoder(self) -> Dict[int, AddedToken]:
"""
Returns the added tokens in the vocabulary as a dictionary of index to AddedToken.
Returns:
`Dict[str, int]`: The added tokens.
"""
return self._tokenizer.get_added_tokens_decoder()
def get_added_vocab(self) -> Dict[str, int]:
"""
Returns the added tokens in the vocabulary as a dictionary of token to index.
Returns:
`Dict[str, int]`: The added tokens.
"""
return {k.content: v for v, k in sorted(self.added_tokens_decoder.items(), key=lambda item: item[0])}
def __len__(self) -> int:
"""
Size of the full vocabulary with the added tokens.
"""
return self._tokenizer.get_vocab_size(with_added_tokens=True)
@property
def backend_tokenizer(self) -> TokenizerFast:
"""
`tokenizers.implementations.BaseTokenizer`: The Rust tokenizer used as a backend.
"""
return self._tokenizer
@property
def decoder(self) -> DecoderFast:
"""
`tokenizers.decoders.Decoder`: The Rust decoder for this tokenizer.
"""
return self._tokenizer.decoder
def _convert_encoding(
self,
encoding: EncodingFast,
return_token_type_ids: Optional[bool] = None,
return_attention_mask: Optional[bool] = None,
return_overflowing_tokens: bool = False,
return_special_tokens_mask: bool = False,
return_offsets_mapping: bool = False,
return_length: bool = False,
verbose: bool = True,
) -> Tuple[Dict[str, Any], List[EncodingFast]]:
"""
Convert the encoding representation (from low-level HuggingFace tokenizer output) to a python Dict and a list
of encodings, take care of building a batch from overflowing tokens.
Overflowing tokens are converted to additional examples (like batches) so the output values of the dict are
lists (overflows) of lists (tokens).
Output shape: (overflows, sequence length)
"""
if return_token_type_ids is None:
return_token_type_ids = "token_type_ids" in self.model_input_names
if return_attention_mask is None:
return_attention_mask = "attention_mask" in self.model_input_names
if return_overflowing_tokens and encoding.overflowing is not None:
encodings = [encoding] + encoding.overflowing
else:
encodings = [encoding]
encoding_dict = defaultdict(list)
for e in encodings:
encoding_dict["input_ids"].append(e.ids)
if return_token_type_ids:
encoding_dict["token_type_ids"].append(e.type_ids)
if return_attention_mask:
encoding_dict["attention_mask"].append(e.attention_mask)
if return_special_tokens_mask:
encoding_dict["special_tokens_mask"].append(e.special_tokens_mask)
if return_offsets_mapping:
encoding_dict["offset_mapping"].append(e.offsets)
if return_length:
encoding_dict["length"].append(len(e.ids))
return encoding_dict, encodings
def convert_tokens_to_ids(self, tokens: Union[str, List[str]]) -> Union[int, List[int]]:
"""
Converts a token string (or a sequence of tokens) in a single integer id (or a sequence of ids), using the
vocabulary.
Args:
tokens (`str` or `List[str]`): One or several token(s) to convert to token id(s).
Returns:
`int` or `List[int]`: The token id or list of token ids.
"""
if tokens is None:
return None
if isinstance(tokens, str):
return self._convert_token_to_id_with_added_voc(tokens)
return [self._convert_token_to_id_with_added_voc(token) for token in tokens]
def _convert_token_to_id_with_added_voc(self, token: str) -> int:
index = self._tokenizer.token_to_id(token)
if index is None:
return self.unk_token_id
return index
def _convert_id_to_token(self, index: int) -> Optional[str]:
return self._tokenizer.id_to_token(int(index))
def _add_tokens(self, new_tokens: List[Union[str, AddedToken]], special_tokens=False) -> int:
if special_tokens:
return self._tokenizer.add_special_tokens(new_tokens)
return self._tokenizer.add_tokens(new_tokens)
def num_special_tokens_to_add(self, pair: bool = False) -> int:
"""
Returns the number of added tokens when encoding a sequence with special tokens.
<Tip>
This encodes a dummy input and checks the number of added tokens, and is therefore not efficient. Do not put
this inside your training loop.
</Tip>
Args:
pair (`bool`, *optional*, defaults to `False`):
Whether the number of added tokens should be computed in the case of a sequence pair or a single
sequence.
Returns:
`int`: Number of special tokens added to sequences.
"""
return self._tokenizer.num_special_tokens_to_add(pair)
def convert_ids_to_tokens(
self, ids: Union[int, List[int]], skip_special_tokens: bool = False
) -> Union[str, List[str]]:
"""
Converts a single index or a sequence of indices in a token or a sequence of tokens, using the vocabulary and
added tokens.
Args:
ids (`int` or `List[int]`):
The token id (or token ids) to convert to tokens.
skip_special_tokens (`bool`, *optional*, defaults to `False`):
Whether or not to remove special tokens in the decoding.
Returns:
`str` or `List[str]`: The decoded token(s).
"""
if isinstance(ids, int):
return self._tokenizer.id_to_token(ids)
tokens = []
for index in ids:
index = int(index)
if skip_special_tokens and index in self.all_special_ids:
continue
tokens.append(self._tokenizer.id_to_token(index))
return tokens
def tokenize(self, text: str, pair: Optional[str] = None, add_special_tokens: bool = False, **kwargs) -> List[str]:
return self.encode_plus(text=text, text_pair=pair, add_special_tokens=add_special_tokens, **kwargs).tokens()
def set_truncation_and_padding(
self,
padding_strategy: PaddingStrategy,
truncation_strategy: TruncationStrategy,
max_length: int,
stride: int,
pad_to_multiple_of: Optional[int],
):
"""
Define the truncation and the padding strategies for fast tokenizers (provided by HuggingFace tokenizers
library) and restore the tokenizer settings afterwards.
The provided tokenizer has no padding / truncation strategy before the managed section. If your tokenizer set a
padding / truncation strategy before, then it will be reset to no padding / truncation when exiting the managed
section.
Args:
padding_strategy ([`~utils.PaddingStrategy`]):
The kind of padding that will be applied to the input
truncation_strategy ([`~tokenization_utils_base.TruncationStrategy`]):
The kind of truncation that will be applied to the input
max_length (`int`):
The maximum size of a sequence.
stride (`int`):
The stride to use when handling overflow.
pad_to_multiple_of (`int`, *optional*):
If set will pad the sequence to a multiple of the provided value. This is especially useful to enable
the use of Tensor Cores on NVIDIA hardware with compute capability `>= 7.5` (Volta).
"""
_truncation = self._tokenizer.truncation
_padding = self._tokenizer.padding
# Set truncation and padding on the backend tokenizer
if truncation_strategy == TruncationStrategy.DO_NOT_TRUNCATE:
if _truncation is not None:
self._tokenizer.no_truncation()
else:
target = {
"max_length": max_length,
"stride": stride,
"strategy": truncation_strategy.value,
"direction": self.truncation_side,
}
# _truncation might contain more keys that the target `transformers`
# supports. Use only the target keys to trigger `enable_truncation`.
# This should enable this code to works on various `tokenizers`
# targets.
if _truncation is None:
current = None
else:
current = {k: _truncation.get(k, None) for k in target}
if current != target:
self._tokenizer.enable_truncation(**target)
if padding_strategy == PaddingStrategy.DO_NOT_PAD:
if _padding is not None:
self._tokenizer.no_padding()
else:
length = max_length if padding_strategy == PaddingStrategy.MAX_LENGTH else None
target = {
"length": length,
"direction": self.padding_side,
"pad_id": self.pad_token_id,
"pad_token": self.pad_token,
"pad_type_id": self.pad_token_type_id,
"pad_to_multiple_of": pad_to_multiple_of,
}
if _padding != target:
self._tokenizer.enable_padding(**target)
def _batch_encode_plus(
self,
batch_text_or_text_pairs: Union[
List[TextInput], List[TextInputPair], List[PreTokenizedInput], List[PreTokenizedInputPair]
],
add_special_tokens: bool = True,
padding_strategy: PaddingStrategy = PaddingStrategy.DO_NOT_PAD,
truncation_strategy: TruncationStrategy = TruncationStrategy.DO_NOT_TRUNCATE,
max_length: Optional[int] = None,
stride: int = 0,
is_split_into_words: bool = False,
pad_to_multiple_of: Optional[int] = None,
return_tensors: Optional[str] = None,
return_token_type_ids: Optional[bool] = None,
return_attention_mask: Optional[bool] = None,
return_overflowing_tokens: bool = False,
return_special_tokens_mask: bool = False,
return_offsets_mapping: bool = False,
return_length: bool = False,
verbose: bool = True,
) -> BatchEncoding:
if not isinstance(batch_text_or_text_pairs, (tuple, list)):
raise TypeError(
f"batch_text_or_text_pairs has to be a list or a tuple (got {type(batch_text_or_text_pairs)})"
)
# Set the truncation and padding strategy and restore the initial configuration
self.set_truncation_and_padding(
padding_strategy=padding_strategy,
truncation_strategy=truncation_strategy,
max_length=max_length,
stride=stride,
pad_to_multiple_of=pad_to_multiple_of,
)
encodings = self._tokenizer.encode_batch(
batch_text_or_text_pairs,
add_special_tokens=add_special_tokens,
is_pretokenized=is_split_into_words,
)
# Convert encoding to dict
# `Tokens` has type: Tuple[
# List[Dict[str, List[List[int]]]] or List[Dict[str, 2D-Tensor]],
# List[EncodingFast]
# ]
# with nested dimensions corresponding to batch, overflows, sequence length
tokens_and_encodings = [
self._convert_encoding(
encoding=encoding,
return_token_type_ids=return_token_type_ids,
return_attention_mask=return_attention_mask,
return_overflowing_tokens=return_overflowing_tokens,
return_special_tokens_mask=return_special_tokens_mask,
return_offsets_mapping=return_offsets_mapping,
return_length=return_length,
verbose=verbose,
)
for encoding in encodings
]
# Convert the output to have dict[list] from list[dict] and remove the additional overflows dimension
# From (variable) shape (batch, overflows, sequence length) to ~ (batch * overflows, sequence length)
# (we say ~ because the number of overflow varies with the example in the batch)
#
# To match each overflowing sample with the original sample in the batch
# we add an overflow_to_sample_mapping array (see below)
sanitized_tokens = {}
for key in tokens_and_encodings[0][0].keys():
stack = [e for item, _ in tokens_and_encodings for e in item[key]]
sanitized_tokens[key] = stack
sanitized_encodings = [e for _, item in tokens_and_encodings for e in item]
# If returning overflowing tokens, we need to return a mapping
# from the batch idx to the original sample
if return_overflowing_tokens:
overflow_to_sample_mapping = []
for i, (toks, _) in enumerate(tokens_and_encodings):
overflow_to_sample_mapping += [i] * len(toks["input_ids"])
sanitized_tokens["overflow_to_sample_mapping"] = overflow_to_sample_mapping
for input_ids in sanitized_tokens["input_ids"]:
self._eventual_warn_about_too_long_sequence(input_ids, max_length, verbose)
return BatchEncoding(sanitized_tokens, sanitized_encodings, tensor_type=return_tensors)
def _encode_plus(
self,
text: Union[TextInput, PreTokenizedInput],
text_pair: Optional[Union[TextInput, PreTokenizedInput]] = None,
add_special_tokens: bool = True,
padding_strategy: PaddingStrategy = PaddingStrategy.DO_NOT_PAD,
truncation_strategy: TruncationStrategy = TruncationStrategy.DO_NOT_TRUNCATE,
max_length: Optional[int] = None,
stride: int = 0,
is_split_into_words: bool = False,
pad_to_multiple_of: Optional[int] = None,
return_tensors: Optional[bool] = None,
return_token_type_ids: Optional[bool] = None,
return_attention_mask: Optional[bool] = None,
return_overflowing_tokens: bool = False,
return_special_tokens_mask: bool = False,
return_offsets_mapping: bool = False,
return_length: bool = False,
verbose: bool = True,
**kwargs,
) -> BatchEncoding:
batched_input = [(text, text_pair)] if text_pair else [text]
batched_output = self._batch_encode_plus(
batched_input,
is_split_into_words=is_split_into_words,
add_special_tokens=add_special_tokens,
padding_strategy=padding_strategy,
truncation_strategy=truncation_strategy,
max_length=max_length,
stride=stride,
pad_to_multiple_of=pad_to_multiple_of,
return_tensors=return_tensors,
return_token_type_ids=return_token_type_ids,
return_attention_mask=return_attention_mask,
return_overflowing_tokens=return_overflowing_tokens,
return_special_tokens_mask=return_special_tokens_mask,
return_offsets_mapping=return_offsets_mapping,
return_length=return_length,
verbose=verbose,
**kwargs,
)
# Return tensor is None, then we can remove the leading batch axis
# Overflowing tokens are returned as a batch of output so we keep them in this case
if return_tensors is None and not return_overflowing_tokens:
batched_output = BatchEncoding(
{
key: value[0] if len(value) > 0 and isinstance(value[0], list) else value
for key, value in batched_output.items()
},
batched_output.encodings,
)
self._eventual_warn_about_too_long_sequence(batched_output["input_ids"], max_length, verbose)
return batched_output
def convert_tokens_to_string(self, tokens: List[str]) -> str:
return self.backend_tokenizer.decoder.decode(tokens)
def _decode(
self,
token_ids: Union[int, List[int]],
skip_special_tokens: bool = False,
clean_up_tokenization_spaces: bool = None,
**kwargs,
) -> str:
self._decode_use_source_tokenizer = kwargs.pop("use_source_tokenizer", False)
if isinstance(token_ids, int):
token_ids = [token_ids]
text = self._tokenizer.decode(token_ids, skip_special_tokens=skip_special_tokens)
clean_up_tokenization_spaces = (
clean_up_tokenization_spaces
if clean_up_tokenization_spaces is not None
else self.clean_up_tokenization_spaces
)
if clean_up_tokenization_spaces:
clean_text = self.clean_up_tokenization(text)
return clean_text
else:
return text
def _save_pretrained(
self,
save_directory: Union[str, os.PathLike],
file_names: Tuple[str],
legacy_format: Optional[bool] = None,
filename_prefix: Optional[str] = None,
) -> Tuple[str]:
"""
Save a tokenizer using the slow-tokenizer/legacy format: vocabulary + added tokens as well as in a unique JSON
file containing {config + vocab + added-tokens}.
"""
save_directory = str(save_directory)
if self.slow_tokenizer_class is None and legacy_format is True:
raise ValueError(
"Your tokenizer does not have a legacy version defined and therefore cannot register this version. You"
" might consider leaving the legacy_format at `None` or setting it to `False`."
)
save_slow = (
(legacy_format is None or legacy_format is True)
and self.slow_tokenizer_class is not None
and self.can_save_slow_tokenizer
)
save_fast = legacy_format is None or legacy_format is False
if save_slow:
added_tokens_file = os.path.join(
save_directory, (filename_prefix + "-" if filename_prefix else "") + ADDED_TOKENS_FILE
)
# make sure to be foward compatible
added_vocab = {tok: index for tok, index in self.added_tokens_encoder.items() if index >= self.vocab_size}
if added_vocab:
with open(added_tokens_file, "w", encoding="utf-8") as f:
out_str = json.dumps(added_vocab, indent=2, sort_keys=True, ensure_ascii=False) + "\n"
f.write(out_str)
vocab_files = self.save_vocabulary(save_directory, filename_prefix=filename_prefix)
file_names = file_names + vocab_files + (added_tokens_file,)
if save_fast:
tokenizer_file = os.path.join(
save_directory, (filename_prefix + "-" if filename_prefix else "") + TOKENIZER_FILE
)
self.backend_tokenizer.save(tokenizer_file)
file_names = file_names + (tokenizer_file,)
return file_names
def train_new_from_iterator(
self,
text_iterator,
vocab_size,
length=None,
new_special_tokens=None,
special_tokens_map=None,
**kwargs,
):
"""
Trains a tokenizer on a new corpus with the same defaults (in terms of special tokens or tokenization pipeline)
as the current one.
Args:
text_iterator (generator of `List[str]`):
The training corpus. Should be a generator of batches of texts, for instance a list of lists of texts
if you have everything in memory.
vocab_size (`int`):
The size of the vocabulary you want for your tokenizer.
length (`int`, *optional*):
The total number of sequences in the iterator. This is used to provide meaningful progress tracking
new_special_tokens (list of `str` or `AddedToken`, *optional*):
A list of new special tokens to add to the tokenizer you are training.
special_tokens_map (`Dict[str, str]`, *optional*):
If you want to rename some of the special tokens this tokenizer uses, pass along a mapping old special
token name to new special token name in this argument.
kwargs (`Dict[str, Any]`, *optional*):
Additional keyword arguments passed along to the trainer from the 🤗 Tokenizers library.
Returns:
[`PreTrainedTokenizerFast`]: A new tokenizer of the same type as the original one, trained on
`text_iterator`.
"""
tokenizer_json = json.loads(self._tokenizer.to_str())
# Remove added tokens for now (uses IDs of tokens)
added_tokens = tokenizer_json.pop("added_tokens")
# Remove post processor for now (uses IDs of tokens)
post_processor = tokenizer_json.pop("post_processor")
unk_token = None
# Remove vocab
if tokenizer_json["model"]["type"] == "BPE":
tokenizer_json["model"]["vocab"] = {}
tokenizer_json["model"]["merges"] = []
elif tokenizer_json["model"]["type"] == "Unigram":
if tokenizer_json["model"]["unk_id"] is not None:
unk_id = tokenizer_json["model"]["unk_id"]
unk_token = tokenizer_json["model"]["vocab"][unk_id][0]
if special_tokens_map is not None and unk_token in special_tokens_map:
unk_token = special_tokens_map[unk_token]
tokenizer_json["model"]["unk_id"] = 0
tokenizer_json["model"]["vocab"] = [[unk_token, 0.0]]
elif tokenizer_json["model"]["type"] in ["WordLevel", "WordPiece"]:
tokenizer_json["model"]["vocab"] = {}
else:
raise ValueError(
f"This method does not support this type of tokenizer (found {tokenizer_json['model']['type']}) "
"only BPE, Unigram, WordLevel and WordPiece."
)
if (
special_tokens_map is not None
and "unk_token" in tokenizer_json["model"]
and tokenizer_json["model"]["unk_token"] in special_tokens_map
):
tokenizer_json["model"]["unk_token"] = special_tokens_map[tokenizer_json["model"]["unk_token"]]
tokenizer = TokenizerFast.from_str(json.dumps(tokenizer_json))
# Get the special tokens from the current tokenizer if none are specified.
special_tokens = []
for added_token in added_tokens:
special = added_token.pop("special", None)
_ = added_token.pop("id", None)
if tokenizer_json["model"]["type"] != "Unigram" and not special:
continue
if special_tokens_map is not None and added_token["content"] in special_tokens_map:
added_token["content"] = special_tokens_map[added_token["content"]]
special_tokens.append(AddedToken(**added_token))
if new_special_tokens is not None:
special_tokens.extend(new_special_tokens)
# Trainer needs to know the end of word / continuing subword thingies in BPE
if (
tokenizer_json["model"]["type"] == "BPE"
and "continuing_subword_prefix" not in kwargs
and tokenizer_json["model"]["continuing_subword_prefix"] is not None
):
kwargs["continuing_subword_prefix"] = tokenizer_json["model"]["continuing_subword_prefix"]
if (
tokenizer_json["model"]["type"] == "BPE"
and "end_of_word_suffix" not in kwargs
and tokenizer_json["model"]["end_of_word_suffix"] is not None
):
kwargs["end_of_word_suffix"] = tokenizer_json["model"]["end_of_word_suffix"]
if tokenizer_json["model"]["type"] == "Unigram" and unk_token is not None:
kwargs["unk_token"] = unk_token
if tokenizer_json["pre_tokenizer"] is not None and tokenizer_json["pre_tokenizer"]["type"] == "ByteLevel":
kwargs["initial_alphabet"] = pre_tokenizers_fast.ByteLevel.alphabet()
trainer_class = MODEL_TO_TRAINER_MAPPING[tokenizer_json["model"]["type"]]
trainer = trainer_class(vocab_size=vocab_size, special_tokens=special_tokens, **kwargs)
tokenizer.train_from_iterator(text_iterator, length=length, trainer=trainer)
if post_processor is not None:
trained_tokenizer_json = json.loads(tokenizer.to_str())
# Almost done, we just have to adjust the token IDs in the post processor
if "special_tokens" in post_processor:
for key in post_processor["special_tokens"]:
tokens = post_processor["special_tokens"][key]["tokens"]
if special_tokens_map is not None:
tokens = [special_tokens_map.get(token, token) for token in tokens]
post_processor["special_tokens"][key]["tokens"] = tokens
post_processor["special_tokens"][key]["ids"] = [tokenizer.token_to_id(token) for token in tokens]
for special_token in ["cls", "sep"]:
if special_token in post_processor:
token, _ = post_processor[special_token]
if special_tokens_map is not None and token in special_tokens_map:
token = special_tokens_map[token]
token_id = tokenizer.token_to_id(token)
post_processor[special_token] = [token, token_id]
trained_tokenizer_json["post_processor"] = post_processor
tokenizer = TokenizerFast.from_str(json.dumps(trained_tokenizer_json))
kwargs = self.init_kwargs.copy()
# Map pad/cls/mask token at the Transformers level
special_tokens_list = SpecialTokensMixin.SPECIAL_TOKENS_ATTRIBUTES.copy()
special_tokens_list.remove("additional_special_tokens")
for token in special_tokens_list:
# Get the private one to avoid unnecessary warnings.
if getattr(self, f"_{token}") is not None:
special_token = getattr(self, token)
if special_tokens_map is not None and special_token in special_tokens_map:
special_token = special_tokens_map[special_token]
special_token_full = getattr(self, f"_{token}")
if isinstance(special_token_full, AddedToken):
# Create an added token with the same parameters except the content
kwargs[token] = AddedToken(
special_token,
single_word=special_token_full.single_word,
lstrip=special_token_full.lstrip,
rstrip=special_token_full.rstrip,
normalized=special_token_full.normalized,
special=True,
)
else:
kwargs[token] = special_token
additional_special_tokens = self.additional_special_tokens
if new_special_tokens is not None:
additional_special_tokens.extend(new_special_tokens)
if len(additional_special_tokens) > 0:
kwargs["additional_special_tokens"] = additional_special_tokens
return self.__class__(tokenizer_object=tokenizer, **kwargs)
| transformers/src/transformers/tokenization_utils_fast.py/0 | {
"file_path": "transformers/src/transformers/tokenization_utils_fast.py",
"repo_id": "transformers",
"token_count": 16473
} | 338 |
#!/usr/bin/env python
# coding=utf-8
# Copyright 2023 The HuggingFace Inc. team. All rights reserved.
#
# Licensed under the Apache License, Version 2.0 (the "License");
# you may not use this file except in compliance with the License.
# You may obtain a copy of the License at
#
# http://www.apache.org/licenses/LICENSE-2.0
#
# Unless required by applicable law or agreed to in writing, software
# distributed under the License is distributed on an "AS IS" BASIS,
# WITHOUT WARRANTIES OR CONDITIONS OF ANY KIND, either express or implied.
# See the License for the specific language governing permissions and
# limitations under the License.
import torch
from ..models.speecht5 import SpeechT5ForTextToSpeech, SpeechT5HifiGan, SpeechT5Processor
from ..utils import is_datasets_available
from .base import PipelineTool
if is_datasets_available():
from datasets import load_dataset
class TextToSpeechTool(PipelineTool):
default_checkpoint = "microsoft/speecht5_tts"
description = (
"This is a tool that reads an English text out loud. It takes an input named `text` which should contain the "
"text to read (in English) and returns a waveform object containing the sound."
)
name = "text_reader"
pre_processor_class = SpeechT5Processor
model_class = SpeechT5ForTextToSpeech
post_processor_class = SpeechT5HifiGan
inputs = ["text"]
outputs = ["audio"]
def setup(self):
if self.post_processor is None:
self.post_processor = "microsoft/speecht5_hifigan"
super().setup()
def encode(self, text, speaker_embeddings=None):
inputs = self.pre_processor(text=text, return_tensors="pt", truncation=True)
if speaker_embeddings is None:
if not is_datasets_available():
raise ImportError("Datasets needs to be installed if not passing speaker embeddings.")
embeddings_dataset = load_dataset("Matthijs/cmu-arctic-xvectors", split="validation")
speaker_embeddings = torch.tensor(embeddings_dataset[7305]["xvector"]).unsqueeze(0)
return {"input_ids": inputs["input_ids"], "speaker_embeddings": speaker_embeddings}
def forward(self, inputs):
with torch.no_grad():
return self.model.generate_speech(**inputs)
def decode(self, outputs):
with torch.no_grad():
return self.post_processor(outputs).cpu().detach()
| transformers/src/transformers/tools/text_to_speech.py/0 | {
"file_path": "transformers/src/transformers/tools/text_to_speech.py",
"repo_id": "transformers",
"token_count": 859
} | 339 |
# This file is autogenerated by the command `make fix-copies`, do not edit.
from ..utils import DummyObject, requires_backends
class Pop2PianoFeatureExtractor(metaclass=DummyObject):
_backends = ["essentia", "librosa", "pretty_midi", "scipy", "torch"]
def __init__(self, *args, **kwargs):
requires_backends(self, ["essentia", "librosa", "pretty_midi", "scipy", "torch"])
class Pop2PianoTokenizer(metaclass=DummyObject):
_backends = ["essentia", "librosa", "pretty_midi", "scipy", "torch"]
def __init__(self, *args, **kwargs):
requires_backends(self, ["essentia", "librosa", "pretty_midi", "scipy", "torch"])
class Pop2PianoProcessor(metaclass=DummyObject):
_backends = ["essentia", "librosa", "pretty_midi", "scipy", "torch"]
def __init__(self, *args, **kwargs):
requires_backends(self, ["essentia", "librosa", "pretty_midi", "scipy", "torch"])
| transformers/src/transformers/utils/dummy_essentia_and_librosa_and_pretty_midi_and_scipy_and_torch_objects.py/0 | {
"file_path": "transformers/src/transformers/utils/dummy_essentia_and_librosa_and_pretty_midi_and_scipy_and_torch_objects.py",
"repo_id": "transformers",
"token_count": 367
} | 340 |
# Copyright 2022 The HuggingFace Team. All rights reserved.
#
# Licensed under the Apache License, Version 2.0 (the "License");
# you may not use this file except in compliance with the License.
# You may obtain a copy of the License at
#
# http://www.apache.org/licenses/LICENSE-2.0
#
# Unless required by applicable law or agreed to in writing, software
# distributed under the License is distributed on an "AS IS" BASIS,
# WITHOUT WARRANTIES OR CONDITIONS OF ANY KIND, either express or implied.
# See the License for the specific language governing permissions and
# limitations under the License.
"""
Import utilities: Utilities related to imports and our lazy inits.
"""
import importlib.metadata
import importlib.util
import json
import os
import shutil
import subprocess
import sys
import warnings
from collections import OrderedDict
from functools import lru_cache
from itertools import chain
from types import ModuleType
from typing import Any, Tuple, Union
from packaging import version
from . import logging
logger = logging.get_logger(__name__) # pylint: disable=invalid-name
# TODO: This doesn't work for all packages (`bs4`, `faiss`, etc.) Talk to Sylvain to see how to do with it better.
def _is_package_available(pkg_name: str, return_version: bool = False) -> Union[Tuple[bool, str], bool]:
# Check we're not importing a "pkg_name" directory somewhere but the actual library by trying to grab the version
package_exists = importlib.util.find_spec(pkg_name) is not None
package_version = "N/A"
if package_exists:
try:
package_version = importlib.metadata.version(pkg_name)
package_exists = True
except importlib.metadata.PackageNotFoundError:
package_exists = False
logger.debug(f"Detected {pkg_name} version {package_version}")
if return_version:
return package_exists, package_version
else:
return package_exists
ENV_VARS_TRUE_VALUES = {"1", "ON", "YES", "TRUE"}
ENV_VARS_TRUE_AND_AUTO_VALUES = ENV_VARS_TRUE_VALUES.union({"AUTO"})
USE_TF = os.environ.get("USE_TF", "AUTO").upper()
USE_TORCH = os.environ.get("USE_TORCH", "AUTO").upper()
USE_JAX = os.environ.get("USE_FLAX", "AUTO").upper()
FORCE_TF_AVAILABLE = os.environ.get("FORCE_TF_AVAILABLE", "AUTO").upper()
# `transformers` requires `torch>=1.11` but this variable is exposed publicly, and we can't simply remove it.
# This is the version of torch required to run torch.fx features and torch.onnx with dictionary inputs.
TORCH_FX_REQUIRED_VERSION = version.parse("1.10")
ACCELERATE_MIN_VERSION = "0.21.0"
FSDP_MIN_VERSION = "1.12.0"
_accelerate_available, _accelerate_version = _is_package_available("accelerate", return_version=True)
_apex_available = _is_package_available("apex")
_bitsandbytes_available = _is_package_available("bitsandbytes")
# `importlib.metadata.version` doesn't work with `bs4` but `beautifulsoup4`. For `importlib.util.find_spec`, reversed.
_bs4_available = importlib.util.find_spec("bs4") is not None
_coloredlogs_available = _is_package_available("coloredlogs")
# `importlib.metadata.util` doesn't work with `opencv-python-headless`.
_cv2_available = importlib.util.find_spec("cv2") is not None
_datasets_available = _is_package_available("datasets")
_decord_available = importlib.util.find_spec("decord") is not None
_detectron2_available = _is_package_available("detectron2")
# We need to check both `faiss` and `faiss-cpu`.
_faiss_available = importlib.util.find_spec("faiss") is not None
try:
_faiss_version = importlib.metadata.version("faiss")
logger.debug(f"Successfully imported faiss version {_faiss_version}")
except importlib.metadata.PackageNotFoundError:
try:
_faiss_version = importlib.metadata.version("faiss-cpu")
logger.debug(f"Successfully imported faiss version {_faiss_version}")
except importlib.metadata.PackageNotFoundError:
_faiss_available = False
_ftfy_available = _is_package_available("ftfy")
_g2p_en_available = _is_package_available("g2p_en")
_ipex_available, _ipex_version = _is_package_available("intel_extension_for_pytorch", return_version=True)
_jieba_available = _is_package_available("jieba")
_jinja_available = _is_package_available("jinja2")
_kenlm_available = _is_package_available("kenlm")
_keras_nlp_available = _is_package_available("keras_nlp")
_levenshtein_available = _is_package_available("Levenshtein")
_librosa_available = _is_package_available("librosa")
_natten_available = _is_package_available("natten")
_nltk_available = _is_package_available("nltk")
_onnx_available = _is_package_available("onnx")
_openai_available = _is_package_available("openai")
_optimum_available = _is_package_available("optimum")
_auto_gptq_available = _is_package_available("auto_gptq")
# `importlib.metadata.version` doesn't work with `awq`
_auto_awq_available = importlib.util.find_spec("awq") is not None
_pandas_available = _is_package_available("pandas")
_peft_available = _is_package_available("peft")
_phonemizer_available = _is_package_available("phonemizer")
_psutil_available = _is_package_available("psutil")
_py3nvml_available = _is_package_available("py3nvml")
_pyctcdecode_available = _is_package_available("pyctcdecode")
_pytesseract_available = _is_package_available("pytesseract")
_pytest_available = _is_package_available("pytest")
_pytorch_quantization_available = _is_package_available("pytorch_quantization")
_rjieba_available = _is_package_available("rjieba")
_sacremoses_available = _is_package_available("sacremoses")
_safetensors_available = _is_package_available("safetensors")
_scipy_available = _is_package_available("scipy")
_sentencepiece_available = _is_package_available("sentencepiece")
_is_seqio_available = _is_package_available("seqio")
_sklearn_available = importlib.util.find_spec("sklearn") is not None
if _sklearn_available:
try:
importlib.metadata.version("scikit-learn")
except importlib.metadata.PackageNotFoundError:
_sklearn_available = False
_smdistributed_available = importlib.util.find_spec("smdistributed") is not None
_soundfile_available = _is_package_available("soundfile")
_spacy_available = _is_package_available("spacy")
_sudachipy_available = _is_package_available("sudachipy")
_tensorflow_probability_available = _is_package_available("tensorflow_probability")
_tensorflow_text_available = _is_package_available("tensorflow_text")
_tf2onnx_available = _is_package_available("tf2onnx")
_timm_available = _is_package_available("timm")
_tokenizers_available = _is_package_available("tokenizers")
_torchaudio_available = _is_package_available("torchaudio")
_torchdistx_available = _is_package_available("torchdistx")
_torchvision_available = _is_package_available("torchvision")
_torch_version = "N/A"
_torch_available = False
if USE_TORCH in ENV_VARS_TRUE_AND_AUTO_VALUES and USE_TF not in ENV_VARS_TRUE_VALUES:
_torch_available, _torch_version = _is_package_available("torch", return_version=True)
else:
logger.info("Disabling PyTorch because USE_TF is set")
_torch_available = False
_tf_version = "N/A"
_tf_available = False
if FORCE_TF_AVAILABLE in ENV_VARS_TRUE_VALUES:
_tf_available = True
else:
if USE_TF in ENV_VARS_TRUE_AND_AUTO_VALUES and USE_TORCH not in ENV_VARS_TRUE_VALUES:
# Note: _is_package_available("tensorflow") fails for tensorflow-cpu. Please test any changes to the line below
# with tensorflow-cpu to make sure it still works!
_tf_available = importlib.util.find_spec("tensorflow") is not None
if _tf_available:
candidates = (
"tensorflow",
"tensorflow-cpu",
"tensorflow-gpu",
"tf-nightly",
"tf-nightly-cpu",
"tf-nightly-gpu",
"tf-nightly-rocm",
"intel-tensorflow",
"intel-tensorflow-avx512",
"tensorflow-rocm",
"tensorflow-macos",
"tensorflow-aarch64",
)
_tf_version = None
# For the metadata, we have to look for both tensorflow and tensorflow-cpu
for pkg in candidates:
try:
_tf_version = importlib.metadata.version(pkg)
break
except importlib.metadata.PackageNotFoundError:
pass
_tf_available = _tf_version is not None
if _tf_available:
if version.parse(_tf_version) < version.parse("2"):
logger.info(
f"TensorFlow found but with version {_tf_version}. Transformers requires version 2 minimum."
)
_tf_available = False
else:
logger.info("Disabling Tensorflow because USE_TORCH is set")
_essentia_available = importlib.util.find_spec("essentia") is not None
try:
_essentia_version = importlib.metadata.version("essentia")
logger.debug(f"Successfully imported essentia version {_essentia_version}")
except importlib.metadata.PackageNotFoundError:
_essentia_version = False
_pretty_midi_available = importlib.util.find_spec("pretty_midi") is not None
try:
_pretty_midi_version = importlib.metadata.version("pretty_midi")
logger.debug(f"Successfully imported pretty_midi version {_pretty_midi_version}")
except importlib.metadata.PackageNotFoundError:
_pretty_midi_available = False
ccl_version = "N/A"
_is_ccl_available = (
importlib.util.find_spec("torch_ccl") is not None
or importlib.util.find_spec("oneccl_bindings_for_pytorch") is not None
)
try:
ccl_version = importlib.metadata.version("oneccl_bind_pt")
logger.debug(f"Detected oneccl_bind_pt version {ccl_version}")
except importlib.metadata.PackageNotFoundError:
_is_ccl_available = False
_flax_available = False
if USE_JAX in ENV_VARS_TRUE_AND_AUTO_VALUES:
_flax_available, _flax_version = _is_package_available("flax", return_version=True)
if _flax_available:
_jax_available, _jax_version = _is_package_available("jax", return_version=True)
if _jax_available:
logger.info(f"JAX version {_jax_version}, Flax version {_flax_version} available.")
else:
_flax_available = _jax_available = False
_jax_version = _flax_version = "N/A"
_torch_fx_available = False
if _torch_available:
torch_version = version.parse(_torch_version)
_torch_fx_available = (torch_version.major, torch_version.minor) >= (
TORCH_FX_REQUIRED_VERSION.major,
TORCH_FX_REQUIRED_VERSION.minor,
)
def is_kenlm_available():
return _kenlm_available
def is_cv2_available():
return _cv2_available
def is_torch_available():
return _torch_available
def get_torch_version():
return _torch_version
def is_torch_sdpa_available():
if not is_torch_available():
return False
elif _torch_version == "N/A":
return False
# NOTE: We require torch>=2.1 (and not torch>=2.0) to use SDPA in Transformers for two reasons:
# - Allow the global use of the `scale` argument introduced in https://github.com/pytorch/pytorch/pull/95259
# - Memory-efficient attention supports arbitrary attention_mask: https://github.com/pytorch/pytorch/pull/104310
# NOTE: We require torch>=2.1.1 to avoid a numerical issue in SDPA with non-contiguous inputs: https://github.com/pytorch/pytorch/issues/112577
return version.parse(_torch_version) >= version.parse("2.1.1")
def is_torchvision_available():
return _torchvision_available
def is_pyctcdecode_available():
return _pyctcdecode_available
def is_librosa_available():
return _librosa_available
def is_essentia_available():
return _essentia_available
def is_pretty_midi_available():
return _pretty_midi_available
def is_torch_cuda_available():
if is_torch_available():
import torch
return torch.cuda.is_available()
else:
return False
def is_torch_mps_available():
if is_torch_available():
import torch
if hasattr(torch.backends, "mps"):
return torch.backends.mps.is_available()
return False
def is_torch_bf16_gpu_available():
if not is_torch_available():
return False
import torch
return torch.cuda.is_available() and torch.cuda.is_bf16_supported()
def is_torch_bf16_cpu_available():
if not is_torch_available():
return False
import torch
try:
# multiple levels of AttributeError depending on the pytorch version so do them all in one check
_ = torch.cpu.amp.autocast
except AttributeError:
return False
return True
def is_torch_bf16_available():
# the original bf16 check was for gpu only, but later a cpu/bf16 combo has emerged so this util
# has become ambiguous and therefore deprecated
warnings.warn(
"The util is_torch_bf16_available is deprecated, please use is_torch_bf16_gpu_available "
"or is_torch_bf16_cpu_available instead according to whether it's used with cpu or gpu",
FutureWarning,
)
return is_torch_bf16_gpu_available()
@lru_cache()
def is_torch_fp16_available_on_device(device):
if not is_torch_available():
return False
import torch
try:
x = torch.zeros(2, 2, dtype=torch.float16).to(device)
_ = x @ x
# At this moment, let's be strict of the check: check if `LayerNorm` is also supported on device, because many
# models use this layer.
batch, sentence_length, embedding_dim = 3, 4, 5
embedding = torch.randn(batch, sentence_length, embedding_dim, dtype=torch.float16, device=device)
layer_norm = torch.nn.LayerNorm(embedding_dim, dtype=torch.float16, device=device)
_ = layer_norm(embedding)
except: # noqa: E722
# TODO: more precise exception matching, if possible.
# most backends should return `RuntimeError` however this is not guaranteed.
return False
return True
@lru_cache()
def is_torch_bf16_available_on_device(device):
if not is_torch_available():
return False
import torch
if device == "cuda":
return is_torch_bf16_gpu_available()
try:
x = torch.zeros(2, 2, dtype=torch.bfloat16).to(device)
_ = x @ x
except: # noqa: E722
# TODO: more precise exception matching, if possible.
# most backends should return `RuntimeError` however this is not guaranteed.
return False
return True
def is_torch_tf32_available():
if not is_torch_available():
return False
import torch
if not torch.cuda.is_available() or torch.version.cuda is None:
return False
if torch.cuda.get_device_properties(torch.cuda.current_device()).major < 8:
return False
if int(torch.version.cuda.split(".")[0]) < 11:
return False
if version.parse(version.parse(torch.__version__).base_version) < version.parse("1.7"):
return False
return True
def is_torch_fx_available():
return _torch_fx_available
def is_peft_available():
return _peft_available
def is_bs4_available():
return _bs4_available
def is_tf_available():
return _tf_available
def is_coloredlogs_available():
return _coloredlogs_available
def is_tf2onnx_available():
return _tf2onnx_available
def is_onnx_available():
return _onnx_available
def is_openai_available():
return _openai_available
def is_flax_available():
return _flax_available
def is_ftfy_available():
return _ftfy_available
def is_g2p_en_available():
return _g2p_en_available
@lru_cache()
def is_torch_tpu_available(check_device=True):
"Checks if `torch_xla` is installed and potentially if a TPU is in the environment"
if not _torch_available:
return False
if importlib.util.find_spec("torch_xla") is not None:
if check_device:
# We need to check if `xla_device` can be found, will raise a RuntimeError if not
try:
import torch_xla.core.xla_model as xm
_ = xm.xla_device()
return True
except RuntimeError:
return False
return True
return False
@lru_cache()
def is_torch_neuroncore_available(check_device=True):
if importlib.util.find_spec("torch_neuronx") is not None:
return is_torch_tpu_available(check_device)
return False
@lru_cache()
def is_torch_npu_available(check_device=False):
"Checks if `torch_npu` is installed and potentially if a NPU is in the environment"
if not _torch_available or importlib.util.find_spec("torch_npu") is None:
return False
import torch
import torch_npu # noqa: F401
if check_device:
try:
# Will raise a RuntimeError if no NPU is found
_ = torch.npu.device_count()
return torch.npu.is_available()
except RuntimeError:
return False
return hasattr(torch, "npu") and torch.npu.is_available()
def is_torchdynamo_available():
if not is_torch_available():
return False
try:
import torch._dynamo as dynamo # noqa: F401
return True
except Exception:
return False
def is_torch_compile_available():
if not is_torch_available():
return False
import torch
# We don't do any version check here to support nighlies marked as 1.14. Ultimately needs to check version against
# 2.0 but let's do it later.
return hasattr(torch, "compile")
def is_torchdynamo_compiling():
if not is_torch_available():
return False
try:
import torch._dynamo as dynamo # noqa: F401
return dynamo.is_compiling()
except Exception:
return False
def is_torch_tensorrt_fx_available():
if importlib.util.find_spec("torch_tensorrt") is None:
return False
return importlib.util.find_spec("torch_tensorrt.fx") is not None
def is_datasets_available():
return _datasets_available
def is_detectron2_available():
return _detectron2_available
def is_rjieba_available():
return _rjieba_available
def is_psutil_available():
return _psutil_available
def is_py3nvml_available():
return _py3nvml_available
def is_sacremoses_available():
return _sacremoses_available
def is_apex_available():
return _apex_available
def is_ninja_available():
r"""
Code comes from *torch.utils.cpp_extension.is_ninja_available()*. Returns `True` if the
[ninja](https://ninja-build.org/) build system is available on the system, `False` otherwise.
"""
try:
subprocess.check_output("ninja --version".split())
except Exception:
return False
else:
return True
def is_ipex_available():
def get_major_and_minor_from_version(full_version):
return str(version.parse(full_version).major) + "." + str(version.parse(full_version).minor)
if not is_torch_available() or not _ipex_available:
return False
torch_major_and_minor = get_major_and_minor_from_version(_torch_version)
ipex_major_and_minor = get_major_and_minor_from_version(_ipex_version)
if torch_major_and_minor != ipex_major_and_minor:
logger.warning(
f"Intel Extension for PyTorch {ipex_major_and_minor} needs to work with PyTorch {ipex_major_and_minor}.*,"
f" but PyTorch {_torch_version} is found. Please switch to the matching version and run again."
)
return False
return True
@lru_cache
def is_torch_xpu_available(check_device=False):
"Checks if `intel_extension_for_pytorch` is installed and potentially if a XPU is in the environment"
if not is_ipex_available():
return False
import intel_extension_for_pytorch # noqa: F401
import torch
if check_device:
try:
# Will raise a RuntimeError if no XPU is found
_ = torch.xpu.device_count()
return torch.xpu.is_available()
except RuntimeError:
return False
return hasattr(torch, "xpu") and torch.xpu.is_available()
def is_bitsandbytes_available():
if not is_torch_available():
return False
# bitsandbytes throws an error if cuda is not available
# let's avoid that by adding a simple check
import torch
return _bitsandbytes_available and torch.cuda.is_available()
def is_flash_attn_2_available():
if not is_torch_available():
return False
if not _is_package_available("flash_attn"):
return False
# Let's add an extra check to see if cuda is available
import torch
if not torch.cuda.is_available():
return False
if torch.version.cuda:
return version.parse(importlib.metadata.version("flash_attn")) >= version.parse("2.1.0")
elif torch.version.hip:
# TODO: Bump the requirement to 2.1.0 once released in https://github.com/ROCmSoftwarePlatform/flash-attention
return version.parse(importlib.metadata.version("flash_attn")) >= version.parse("2.0.4")
else:
return False
def is_flash_attn_greater_or_equal_2_10():
if not _is_package_available("flash_attn"):
return False
return version.parse(importlib.metadata.version("flash_attn")) >= version.parse("2.1.0")
def is_flash_attn_available():
logger.warning(
"Using `is_flash_attn_available` is deprecated and will be removed in v4.38. "
"Please use `is_flash_attn_2_available` instead."
)
return is_flash_attn_2_available()
def is_torchdistx_available():
return _torchdistx_available
def is_faiss_available():
return _faiss_available
def is_scipy_available():
return _scipy_available
def is_sklearn_available():
return _sklearn_available
def is_sentencepiece_available():
return _sentencepiece_available
def is_seqio_available():
return _is_seqio_available
def is_protobuf_available():
if importlib.util.find_spec("google") is None:
return False
return importlib.util.find_spec("google.protobuf") is not None
def is_accelerate_available(min_version: str = ACCELERATE_MIN_VERSION):
if min_version is not None:
return _accelerate_available and version.parse(_accelerate_version) >= version.parse(min_version)
return _accelerate_available
def is_fsdp_available(min_version: str = FSDP_MIN_VERSION):
return is_torch_available() and version.parse(_torch_version) >= version.parse(min_version)
def is_optimum_available():
return _optimum_available
def is_auto_awq_available():
return _auto_awq_available
def is_auto_gptq_available():
return _auto_gptq_available
def is_levenshtein_available():
return _levenshtein_available
def is_optimum_neuron_available():
return _optimum_available and _is_package_available("optimum.neuron")
def is_safetensors_available():
return _safetensors_available
def is_tokenizers_available():
return _tokenizers_available
def is_vision_available():
_pil_available = importlib.util.find_spec("PIL") is not None
if _pil_available:
try:
package_version = importlib.metadata.version("Pillow")
except importlib.metadata.PackageNotFoundError:
try:
package_version = importlib.metadata.version("Pillow-SIMD")
except importlib.metadata.PackageNotFoundError:
return False
logger.debug(f"Detected PIL version {package_version}")
return _pil_available
def is_pytesseract_available():
return _pytesseract_available
def is_pytest_available():
return _pytest_available
def is_spacy_available():
return _spacy_available
def is_tensorflow_text_available():
return is_tf_available() and _tensorflow_text_available
def is_keras_nlp_available():
return is_tensorflow_text_available() and _keras_nlp_available
def is_in_notebook():
try:
# Test adapted from tqdm.autonotebook: https://github.com/tqdm/tqdm/blob/master/tqdm/autonotebook.py
get_ipython = sys.modules["IPython"].get_ipython
if "IPKernelApp" not in get_ipython().config:
raise ImportError("console")
if "VSCODE_PID" in os.environ:
raise ImportError("vscode")
if "DATABRICKS_RUNTIME_VERSION" in os.environ and os.environ["DATABRICKS_RUNTIME_VERSION"] < "11.0":
# Databricks Runtime 11.0 and above uses IPython kernel by default so it should be compatible with Jupyter notebook
# https://docs.microsoft.com/en-us/azure/databricks/notebooks/ipython-kernel
raise ImportError("databricks")
return importlib.util.find_spec("IPython") is not None
except (AttributeError, ImportError, KeyError):
return False
def is_pytorch_quantization_available():
return _pytorch_quantization_available
def is_tensorflow_probability_available():
return _tensorflow_probability_available
def is_pandas_available():
return _pandas_available
def is_sagemaker_dp_enabled():
# Get the sagemaker specific env variable.
sagemaker_params = os.getenv("SM_FRAMEWORK_PARAMS", "{}")
try:
# Parse it and check the field "sagemaker_distributed_dataparallel_enabled".
sagemaker_params = json.loads(sagemaker_params)
if not sagemaker_params.get("sagemaker_distributed_dataparallel_enabled", False):
return False
except json.JSONDecodeError:
return False
# Lastly, check if the `smdistributed` module is present.
return _smdistributed_available
def is_sagemaker_mp_enabled():
# Get the sagemaker specific mp parameters from smp_options variable.
smp_options = os.getenv("SM_HP_MP_PARAMETERS", "{}")
try:
# Parse it and check the field "partitions" is included, it is required for model parallel.
smp_options = json.loads(smp_options)
if "partitions" not in smp_options:
return False
except json.JSONDecodeError:
return False
# Get the sagemaker specific framework parameters from mpi_options variable.
mpi_options = os.getenv("SM_FRAMEWORK_PARAMS", "{}")
try:
# Parse it and check the field "sagemaker_distributed_dataparallel_enabled".
mpi_options = json.loads(mpi_options)
if not mpi_options.get("sagemaker_mpi_enabled", False):
return False
except json.JSONDecodeError:
return False
# Lastly, check if the `smdistributed` module is present.
return _smdistributed_available
def is_training_run_on_sagemaker():
return "SAGEMAKER_JOB_NAME" in os.environ
def is_soundfile_availble():
return _soundfile_available
def is_timm_available():
return _timm_available
def is_natten_available():
return _natten_available
def is_nltk_available():
return _nltk_available
def is_torchaudio_available():
return _torchaudio_available
def is_speech_available():
# For now this depends on torchaudio but the exact dependency might evolve in the future.
return _torchaudio_available
def is_phonemizer_available():
return _phonemizer_available
def torch_only_method(fn):
def wrapper(*args, **kwargs):
if not _torch_available:
raise ImportError(
"You need to install pytorch to use this method or class, "
"or activate it with environment variables USE_TORCH=1 and USE_TF=0."
)
else:
return fn(*args, **kwargs)
return wrapper
def is_ccl_available():
return _is_ccl_available
def is_decord_available():
return _decord_available
def is_sudachi_available():
return _sudachipy_available
def is_jumanpp_available():
return (importlib.util.find_spec("rhoknp") is not None) and (shutil.which("jumanpp") is not None)
def is_cython_available():
return importlib.util.find_spec("pyximport") is not None
def is_jieba_available():
return _jieba_available
def is_jinja_available():
return _jinja_available
# docstyle-ignore
CV2_IMPORT_ERROR = """
{0} requires the OpenCV library but it was not found in your environment. You can install it with:
```
pip install opencv-python
```
Please note that you may need to restart your runtime after installation.
"""
# docstyle-ignore
DATASETS_IMPORT_ERROR = """
{0} requires the 🤗 Datasets library but it was not found in your environment. You can install it with:
```
pip install datasets
```
In a notebook or a colab, you can install it by executing a cell with
```
!pip install datasets
```
then restarting your kernel.
Note that if you have a local folder named `datasets` or a local python file named `datasets.py` in your current
working directory, python may try to import this instead of the 🤗 Datasets library. You should rename this folder or
that python file if that's the case. Please note that you may need to restart your runtime after installation.
"""
# docstyle-ignore
TOKENIZERS_IMPORT_ERROR = """
{0} requires the 🤗 Tokenizers library but it was not found in your environment. You can install it with:
```
pip install tokenizers
```
In a notebook or a colab, you can install it by executing a cell with
```
!pip install tokenizers
```
Please note that you may need to restart your runtime after installation.
"""
# docstyle-ignore
SENTENCEPIECE_IMPORT_ERROR = """
{0} requires the SentencePiece library but it was not found in your environment. Checkout the instructions on the
installation page of its repo: https://github.com/google/sentencepiece#installation and follow the ones
that match your environment. Please note that you may need to restart your runtime after installation.
"""
# docstyle-ignore
PROTOBUF_IMPORT_ERROR = """
{0} requires the protobuf library but it was not found in your environment. Checkout the instructions on the
installation page of its repo: https://github.com/protocolbuffers/protobuf/tree/master/python#installation and follow the ones
that match your environment. Please note that you may need to restart your runtime after installation.
"""
# docstyle-ignore
FAISS_IMPORT_ERROR = """
{0} requires the faiss library but it was not found in your environment. Checkout the instructions on the
installation page of its repo: https://github.com/facebookresearch/faiss/blob/master/INSTALL.md and follow the ones
that match your environment. Please note that you may need to restart your runtime after installation.
"""
# docstyle-ignore
PYTORCH_IMPORT_ERROR = """
{0} requires the PyTorch library but it was not found in your environment. Checkout the instructions on the
installation page: https://pytorch.org/get-started/locally/ and follow the ones that match your environment.
Please note that you may need to restart your runtime after installation.
"""
# docstyle-ignore
TORCHVISION_IMPORT_ERROR = """
{0} requires the Torchvision library but it was not found in your environment. Checkout the instructions on the
installation page: https://pytorch.org/get-started/locally/ and follow the ones that match your environment.
Please note that you may need to restart your runtime after installation.
"""
# docstyle-ignore
PYTORCH_IMPORT_ERROR_WITH_TF = """
{0} requires the PyTorch library but it was not found in your environment.
However, we were able to find a TensorFlow installation. TensorFlow classes begin
with "TF", but are otherwise identically named to our PyTorch classes. This
means that the TF equivalent of the class you tried to import would be "TF{0}".
If you want to use TensorFlow, please use TF classes instead!
If you really do want to use PyTorch please go to
https://pytorch.org/get-started/locally/ and follow the instructions that
match your environment.
"""
# docstyle-ignore
TF_IMPORT_ERROR_WITH_PYTORCH = """
{0} requires the TensorFlow library but it was not found in your environment.
However, we were able to find a PyTorch installation. PyTorch classes do not begin
with "TF", but are otherwise identically named to our TF classes.
If you want to use PyTorch, please use those classes instead!
If you really do want to use TensorFlow, please follow the instructions on the
installation page https://www.tensorflow.org/install that match your environment.
"""
# docstyle-ignore
BS4_IMPORT_ERROR = """
{0} requires the Beautiful Soup library but it was not found in your environment. You can install it with pip:
`pip install beautifulsoup4`. Please note that you may need to restart your runtime after installation.
"""
# docstyle-ignore
SKLEARN_IMPORT_ERROR = """
{0} requires the scikit-learn library but it was not found in your environment. You can install it with:
```
pip install -U scikit-learn
```
In a notebook or a colab, you can install it by executing a cell with
```
!pip install -U scikit-learn
```
Please note that you may need to restart your runtime after installation.
"""
# docstyle-ignore
TENSORFLOW_IMPORT_ERROR = """
{0} requires the TensorFlow library but it was not found in your environment. Checkout the instructions on the
installation page: https://www.tensorflow.org/install and follow the ones that match your environment.
Please note that you may need to restart your runtime after installation.
"""
# docstyle-ignore
DETECTRON2_IMPORT_ERROR = """
{0} requires the detectron2 library but it was not found in your environment. Checkout the instructions on the
installation page: https://github.com/facebookresearch/detectron2/blob/master/INSTALL.md and follow the ones
that match your environment. Please note that you may need to restart your runtime after installation.
"""
# docstyle-ignore
FLAX_IMPORT_ERROR = """
{0} requires the FLAX library but it was not found in your environment. Checkout the instructions on the
installation page: https://github.com/google/flax and follow the ones that match your environment.
Please note that you may need to restart your runtime after installation.
"""
# docstyle-ignore
FTFY_IMPORT_ERROR = """
{0} requires the ftfy library but it was not found in your environment. Checkout the instructions on the
installation section: https://github.com/rspeer/python-ftfy/tree/master#installing and follow the ones
that match your environment. Please note that you may need to restart your runtime after installation.
"""
LEVENSHTEIN_IMPORT_ERROR = """
{0} requires the python-Levenshtein library but it was not found in your environment. You can install it with pip: `pip
install python-Levenshtein`. Please note that you may need to restart your runtime after installation.
"""
# docstyle-ignore
G2P_EN_IMPORT_ERROR = """
{0} requires the g2p-en library but it was not found in your environment. You can install it with pip:
`pip install g2p-en`. Please note that you may need to restart your runtime after installation.
"""
# docstyle-ignore
PYTORCH_QUANTIZATION_IMPORT_ERROR = """
{0} requires the pytorch-quantization library but it was not found in your environment. You can install it with pip:
`pip install pytorch-quantization --extra-index-url https://pypi.ngc.nvidia.com`
Please note that you may need to restart your runtime after installation.
"""
# docstyle-ignore
TENSORFLOW_PROBABILITY_IMPORT_ERROR = """
{0} requires the tensorflow_probability library but it was not found in your environment. You can install it with pip as
explained here: https://github.com/tensorflow/probability. Please note that you may need to restart your runtime after installation.
"""
# docstyle-ignore
TENSORFLOW_TEXT_IMPORT_ERROR = """
{0} requires the tensorflow_text library but it was not found in your environment. You can install it with pip as
explained here: https://www.tensorflow.org/text/guide/tf_text_intro.
Please note that you may need to restart your runtime after installation.
"""
# docstyle-ignore
PANDAS_IMPORT_ERROR = """
{0} requires the pandas library but it was not found in your environment. You can install it with pip as
explained here: https://pandas.pydata.org/pandas-docs/stable/getting_started/install.html.
Please note that you may need to restart your runtime after installation.
"""
# docstyle-ignore
PHONEMIZER_IMPORT_ERROR = """
{0} requires the phonemizer library but it was not found in your environment. You can install it with pip:
`pip install phonemizer`. Please note that you may need to restart your runtime after installation.
"""
# docstyle-ignore
SACREMOSES_IMPORT_ERROR = """
{0} requires the sacremoses library but it was not found in your environment. You can install it with pip:
`pip install sacremoses`. Please note that you may need to restart your runtime after installation.
"""
# docstyle-ignore
SCIPY_IMPORT_ERROR = """
{0} requires the scipy library but it was not found in your environment. You can install it with pip:
`pip install scipy`. Please note that you may need to restart your runtime after installation.
"""
# docstyle-ignore
SPEECH_IMPORT_ERROR = """
{0} requires the torchaudio library but it was not found in your environment. You can install it with pip:
`pip install torchaudio`. Please note that you may need to restart your runtime after installation.
"""
# docstyle-ignore
TIMM_IMPORT_ERROR = """
{0} requires the timm library but it was not found in your environment. You can install it with pip:
`pip install timm`. Please note that you may need to restart your runtime after installation.
"""
# docstyle-ignore
NATTEN_IMPORT_ERROR = """
{0} requires the natten library but it was not found in your environment. You can install it by referring to:
shi-labs.com/natten . You can also install it with pip (may take longer to build):
`pip install natten`. Please note that you may need to restart your runtime after installation.
"""
# docstyle-ignore
NLTK_IMPORT_ERROR = """
{0} requires the NLTK library but it was not found in your environment. You can install it by referring to:
https://www.nltk.org/install.html. Please note that you may need to restart your runtime after installation.
"""
# docstyle-ignore
VISION_IMPORT_ERROR = """
{0} requires the PIL library but it was not found in your environment. You can install it with pip:
`pip install pillow`. Please note that you may need to restart your runtime after installation.
"""
# docstyle-ignore
PYTESSERACT_IMPORT_ERROR = """
{0} requires the PyTesseract library but it was not found in your environment. You can install it with pip:
`pip install pytesseract`. Please note that you may need to restart your runtime after installation.
"""
# docstyle-ignore
PYCTCDECODE_IMPORT_ERROR = """
{0} requires the pyctcdecode library but it was not found in your environment. You can install it with pip:
`pip install pyctcdecode`. Please note that you may need to restart your runtime after installation.
"""
# docstyle-ignore
ACCELERATE_IMPORT_ERROR = """
{0} requires the accelerate library >= {ACCELERATE_MIN_VERSION} it was not found in your environment.
You can install or update it with pip: `pip install --upgrade accelerate`. Please note that you may need to restart your
runtime after installation.
"""
# docstyle-ignore
CCL_IMPORT_ERROR = """
{0} requires the torch ccl library but it was not found in your environment. You can install it with pip:
`pip install oneccl_bind_pt -f https://developer.intel.com/ipex-whl-stable`
Please note that you may need to restart your runtime after installation.
"""
# docstyle-ignore
ESSENTIA_IMPORT_ERROR = """
{0} requires essentia library. But that was not found in your environment. You can install them with pip:
`pip install essentia==2.1b6.dev1034`
Please note that you may need to restart your runtime after installation.
"""
# docstyle-ignore
LIBROSA_IMPORT_ERROR = """
{0} requires thes librosa library. But that was not found in your environment. You can install them with pip:
`pip install librosa`
Please note that you may need to restart your runtime after installation.
"""
# docstyle-ignore
PRETTY_MIDI_IMPORT_ERROR = """
{0} requires thes pretty_midi library. But that was not found in your environment. You can install them with pip:
`pip install pretty_midi`
Please note that you may need to restart your runtime after installation.
"""
DECORD_IMPORT_ERROR = """
{0} requires the decord library but it was not found in your environment. You can install it with pip: `pip install
decord`. Please note that you may need to restart your runtime after installation.
"""
CYTHON_IMPORT_ERROR = """
{0} requires the Cython library but it was not found in your environment. You can install it with pip: `pip install
Cython`. Please note that you may need to restart your runtime after installation.
"""
JIEBA_IMPORT_ERROR = """
{0} requires the jieba library but it was not found in your environment. You can install it with pip: `pip install
jieba`. Please note that you may need to restart your runtime after installation.
"""
PEFT_IMPORT_ERROR = """
{0} requires the peft library but it was not found in your environment. You can install it with pip: `pip install
peft`. Please note that you may need to restart your runtime after installation.
"""
JINJA_IMPORT_ERROR = """
{0} requires the jinja library but it was not found in your environment. You can install it with pip: `pip install
jinja2`. Please note that you may need to restart your runtime after installation.
"""
BACKENDS_MAPPING = OrderedDict(
[
("bs4", (is_bs4_available, BS4_IMPORT_ERROR)),
("cv2", (is_cv2_available, CV2_IMPORT_ERROR)),
("datasets", (is_datasets_available, DATASETS_IMPORT_ERROR)),
("detectron2", (is_detectron2_available, DETECTRON2_IMPORT_ERROR)),
("essentia", (is_essentia_available, ESSENTIA_IMPORT_ERROR)),
("faiss", (is_faiss_available, FAISS_IMPORT_ERROR)),
("flax", (is_flax_available, FLAX_IMPORT_ERROR)),
("ftfy", (is_ftfy_available, FTFY_IMPORT_ERROR)),
("g2p_en", (is_g2p_en_available, G2P_EN_IMPORT_ERROR)),
("pandas", (is_pandas_available, PANDAS_IMPORT_ERROR)),
("phonemizer", (is_phonemizer_available, PHONEMIZER_IMPORT_ERROR)),
("pretty_midi", (is_pretty_midi_available, PRETTY_MIDI_IMPORT_ERROR)),
("levenshtein", (is_levenshtein_available, LEVENSHTEIN_IMPORT_ERROR)),
("librosa", (is_librosa_available, LIBROSA_IMPORT_ERROR)),
("protobuf", (is_protobuf_available, PROTOBUF_IMPORT_ERROR)),
("pyctcdecode", (is_pyctcdecode_available, PYCTCDECODE_IMPORT_ERROR)),
("pytesseract", (is_pytesseract_available, PYTESSERACT_IMPORT_ERROR)),
("sacremoses", (is_sacremoses_available, SACREMOSES_IMPORT_ERROR)),
("pytorch_quantization", (is_pytorch_quantization_available, PYTORCH_QUANTIZATION_IMPORT_ERROR)),
("sentencepiece", (is_sentencepiece_available, SENTENCEPIECE_IMPORT_ERROR)),
("sklearn", (is_sklearn_available, SKLEARN_IMPORT_ERROR)),
("speech", (is_speech_available, SPEECH_IMPORT_ERROR)),
("tensorflow_probability", (is_tensorflow_probability_available, TENSORFLOW_PROBABILITY_IMPORT_ERROR)),
("tf", (is_tf_available, TENSORFLOW_IMPORT_ERROR)),
("tensorflow_text", (is_tensorflow_text_available, TENSORFLOW_TEXT_IMPORT_ERROR)),
("timm", (is_timm_available, TIMM_IMPORT_ERROR)),
("natten", (is_natten_available, NATTEN_IMPORT_ERROR)),
("nltk", (is_nltk_available, NLTK_IMPORT_ERROR)),
("tokenizers", (is_tokenizers_available, TOKENIZERS_IMPORT_ERROR)),
("torch", (is_torch_available, PYTORCH_IMPORT_ERROR)),
("torchvision", (is_torchvision_available, TORCHVISION_IMPORT_ERROR)),
("vision", (is_vision_available, VISION_IMPORT_ERROR)),
("scipy", (is_scipy_available, SCIPY_IMPORT_ERROR)),
("accelerate", (is_accelerate_available, ACCELERATE_IMPORT_ERROR)),
("oneccl_bind_pt", (is_ccl_available, CCL_IMPORT_ERROR)),
("decord", (is_decord_available, DECORD_IMPORT_ERROR)),
("cython", (is_cython_available, CYTHON_IMPORT_ERROR)),
("jieba", (is_jieba_available, JIEBA_IMPORT_ERROR)),
("peft", (is_peft_available, PEFT_IMPORT_ERROR)),
("jinja", (is_jinja_available, JINJA_IMPORT_ERROR)),
]
)
def requires_backends(obj, backends):
if not isinstance(backends, (list, tuple)):
backends = [backends]
name = obj.__name__ if hasattr(obj, "__name__") else obj.__class__.__name__
# Raise an error for users who might not realize that classes without "TF" are torch-only
if "torch" in backends and "tf" not in backends and not is_torch_available() and is_tf_available():
raise ImportError(PYTORCH_IMPORT_ERROR_WITH_TF.format(name))
# Raise the inverse error for PyTorch users trying to load TF classes
if "tf" in backends and "torch" not in backends and is_torch_available() and not is_tf_available():
raise ImportError(TF_IMPORT_ERROR_WITH_PYTORCH.format(name))
checks = (BACKENDS_MAPPING[backend] for backend in backends)
failed = [msg.format(name) for available, msg in checks if not available()]
if failed:
raise ImportError("".join(failed))
class DummyObject(type):
"""
Metaclass for the dummy objects. Any class inheriting from it will return the ImportError generated by
`requires_backend` each time a user tries to access any method of that class.
"""
def __getattribute__(cls, key):
if key.startswith("_") and key != "_from_config":
return super().__getattribute__(key)
requires_backends(cls, cls._backends)
def is_torch_fx_proxy(x):
if is_torch_fx_available():
import torch.fx
return isinstance(x, torch.fx.Proxy)
return False
class _LazyModule(ModuleType):
"""
Module class that surfaces all objects but only performs associated imports when the objects are requested.
"""
# Very heavily inspired by optuna.integration._IntegrationModule
# https://github.com/optuna/optuna/blob/master/optuna/integration/__init__.py
def __init__(self, name, module_file, import_structure, module_spec=None, extra_objects=None):
super().__init__(name)
self._modules = set(import_structure.keys())
self._class_to_module = {}
for key, values in import_structure.items():
for value in values:
self._class_to_module[value] = key
# Needed for autocompletion in an IDE
self.__all__ = list(import_structure.keys()) + list(chain(*import_structure.values()))
self.__file__ = module_file
self.__spec__ = module_spec
self.__path__ = [os.path.dirname(module_file)]
self._objects = {} if extra_objects is None else extra_objects
self._name = name
self._import_structure = import_structure
# Needed for autocompletion in an IDE
def __dir__(self):
result = super().__dir__()
# The elements of self.__all__ that are submodules may or may not be in the dir already, depending on whether
# they have been accessed or not. So we only add the elements of self.__all__ that are not already in the dir.
for attr in self.__all__:
if attr not in result:
result.append(attr)
return result
def __getattr__(self, name: str) -> Any:
if name in self._objects:
return self._objects[name]
if name in self._modules:
value = self._get_module(name)
elif name in self._class_to_module.keys():
module = self._get_module(self._class_to_module[name])
value = getattr(module, name)
else:
raise AttributeError(f"module {self.__name__} has no attribute {name}")
setattr(self, name, value)
return value
def _get_module(self, module_name: str):
try:
return importlib.import_module("." + module_name, self.__name__)
except Exception as e:
raise RuntimeError(
f"Failed to import {self.__name__}.{module_name} because of the following error (look up to see its"
f" traceback):\n{e}"
) from e
def __reduce__(self):
return (self.__class__, (self._name, self.__file__, self._import_structure))
class OptionalDependencyNotAvailable(BaseException):
"""Internally used error class for signalling an optional dependency was not found."""
def direct_transformers_import(path: str, file="__init__.py") -> ModuleType:
"""Imports transformers directly
Args:
path (`str`): The path to the source file
file (`str`, optional): The file to join with the path. Defaults to "__init__.py".
Returns:
`ModuleType`: The resulting imported module
"""
name = "transformers"
location = os.path.join(path, file)
spec = importlib.util.spec_from_file_location(name, location, submodule_search_locations=[path])
module = importlib.util.module_from_spec(spec)
spec.loader.exec_module(module)
module = sys.modules[name]
return module
| transformers/src/transformers/utils/import_utils.py/0 | {
"file_path": "transformers/src/transformers/utils/import_utils.py",
"repo_id": "transformers",
"token_count": 17659
} | 341 |
<!---
Copyright 2020 The HuggingFace Team. All rights reserved.
Licensed under the Apache License, Version 2.0 (the "License");
you may not use this file except in compliance with the License.
You may obtain a copy of the License at
http://www.apache.org/licenses/LICENSE-2.0
Unless required by applicable law or agreed to in writing, software
distributed under the License is distributed on an "AS IS" BASIS,
WITHOUT WARRANTIES OR CONDITIONS OF ANY KIND, either express or implied.
See the License for the specific language governing permissions and
limitations under the License.
-->
# Adding a new model
This folder contains templates to generate new models that fit the current API and pass all tests. It generates
models in both PyTorch, TensorFlow, and Flax and completes the `__init__.py` and auto-modeling files, and creates the
documentation. Their use is described in the [next section](#cookiecutter-templates).
There is also a CLI tool to generate a new model like an existing one called `transformers-cli add-new-model-like`.
Jump to the [Add new model like section](#add-new-model-like-command) to learn how to use it.
## Cookiecutter Templates
Using the `cookiecutter` utility requires to have all the `dev` dependencies installed. Let's first clone the
repository and install it in our environment:
```shell script
git clone https://github.com/huggingface/transformers
cd transformers
pip install -e ".[dev]"
```
Depending on your OS, and since the number of optional dependencies of Transformers is growing, you might get a
failure with this command. If that's the case make sure to install the Deep Learning framework you are working with
(PyTorch, TensorFlow and/or Flax) then do:
```bash
pip install -e ".[quality]"
```
Once the installation is done, you can use the CLI command `add-new-model` to generate your models:
```shell script
transformers-cli add-new-model
```
This should launch the `cookiecutter` package which should prompt you to fill in the configuration.
The `modelname` should be cased according to the plain text casing, i.e., BERT, RoBERTa, DeBERTa.
```
modelname [<ModelNAME>]:
uppercase_modelname [<MODEL_NAME>]:
lowercase_modelname [<model_name>]:
camelcase_modelname [<ModelName>]:
```
Fill in the `authors` with your team members:
```
authors [The HuggingFace Team]:
```
The checkpoint identifier is the checkpoint that will be used in the examples across the files. Put the name you wish,
as it will appear on the modelhub. Do not forget to include the organisation.
```
checkpoint_identifier [organisation/<model_name>-base-cased]:
```
The tokenizer should either be based on BERT if it behaves exactly like the BERT tokenizer, or a standalone otherwise.
```
Select tokenizer_type:
1 - Based on BERT
2 - Standalone
Choose from 1, 2 [1]:
```
<!---
Choose if your model is an encoder-decoder, or an encoder-only architecture.
If your model is an encoder-only architecture, the generated architecture will be based on the BERT model.
If your model is an encoder-decoder architecture, the generated architecture will be based on the BART model. You can,
of course, edit the files once the generation is complete.
```
Select is_encoder_decoder_model:
1 - True
2 - False
Choose from 1, 2 [1]:
```
-->
Once the command has finished, you should have a total of 7 new files spread across the repository:
```
docs/source/model_doc/<model_name>.md
src/transformers/models/<model_name>/configuration_<model_name>.py
src/transformers/models/<model_name>/modeling_<model_name>.py
src/transformers/models/<model_name>/modeling_tf_<model_name>.py
src/transformers/models/<model_name>/tokenization_<model_name>.py
tests/test_modeling_<model_name>.py
tests/test_modeling_tf_<model_name>.py
```
You can run the tests to ensure that they all pass:
```
python -m pytest ./tests/test_*<model_name>*.py
```
Feel free to modify each file to mimic the behavior of your model.
⚠ You should be careful about the classes preceded by the following line:️
```python
# Copied from transformers.[...]
```
This line ensures that the copy does not diverge from the source. If it *should* diverge, because the implementation
is different, this line needs to be deleted. If you don't delete this line and run `make fix-copies`,
your changes will be overwritten.
Once you have edited the files to fit your architecture, simply re-run the tests (and edit them if a change
is needed!) afterwards to make sure everything works as expected.
Once the files are generated and you are happy with your changes, here's a checklist to ensure that your contribution
will be merged quickly:
- You should run the `make fixup` utility to fix the style of the files and to ensure the code quality meets the
library's standards.
- You should complete the documentation file (`docs/source/model_doc/<model_name>.rst`) so that your model may be
usable.
## Add new model like command
Using the `transformers-cli add-new-model-like` command requires to have all the `dev` dependencies installed. Let's
first clone the repository and install it in our environment:
```shell script
git clone https://github.com/huggingface/transformers
cd transformers
pip install -e ".[dev]"
```
Depending on your OS, and since the number of optional dependencies of Transformers is growing, you might get a
failure with this command. If that's the case make sure to install the Deep Learning framework you are working with
(PyTorch, TensorFlow and/or Flax) then do:
```bash
pip install -e ".[quality]"
```
Once the installation is done, you can use the CLI command `add-new-model-like` to generate your models:
```shell script
transformers-cli add-new-model-like
```
This will start a small questionnaire you have to fill.
```
What identifier would you like to use for the model type of this model?
```
You will have to input the model type of the model you want to clone. The model type can be found in several places:
- inside the configuration of any checkpoint of that model
- the name of the documentation page of that model
For instance the doc page of `BigBirdPegasus` is `https://huggingface.co/docs/transformers/model_doc/bigbird_pegasus`
so its model type is `"bigbird_pegasus"`.
If you make a typo, the command will suggest you the closest model types it can find.
Once this is done, the questionnaire will ask you for the new model name and its various casings:
```
What is the name for your new model?
What identifier would you like to use for the model type of this model?
What name would you like to use for the module of this model?
What prefix (camel-cased) would you like to use for the model classes of this model?
What prefix (upper-cased) would you like to use for the constants relative to this model?
```
From your answer to the first question, defaults will be determined for all others. The first name should be written
as you want your model be named in the doc, with no special casing (like RoBERTa) and from there, you can either stick
with the defaults or change the cased versions.
Next will be the name of the config class to use for this model:
```
What will be the name of the config class for this model?
```
Then, you will be asked for a checkpoint identifier:
```
Please give a checkpoint identifier (on the model Hub) for this new model.
```
This is the checkpoint that will be used in the examples across the files and the integration tests. Put the name you
wish, as it will appear on the Model Hub. Do not forget to include the organisation.
Then you will have to say whether your model re-uses the same processing classes as the model you're cloning:
```
Will your new model use the same processing class as Xxx (XxxTokenizer/XxxFeatureExtractor/XxxImageProcessor)
```
Answer yes if you have no intentions to make any change to the class used for preprocessing. It can use different
files (for instance you can reuse the `BertTokenizer` with a new vocab file).
If you answer no, you will have to give the name of the classes
for the new tokenizer/image processor/feature extractor/processor (depending on the model you're cloning).
Next the questionnaire will ask
```
Should we add # Copied from statements when creating the new modeling file?
```
This is the internal mechanism used in the library to make sure code copied from various modeling files stay consistent.
If you plan to completely rewrite the modeling file, you should answer no, whereas if you just want to tweak one part
of the model, you should answer yes.
Lastly, the questionnaire will inquire about frameworks:
```
Should we add a version of your new model in all the frameworks implemented by Old Model (xxx)?
```
If you answer yes, the new model will have files for all the frameworks implemented by the model you're cloning.
Otherwise, you will get a new question to select the frameworks you want.
Once the command has finished, you will see a new subfolder in the `src/transformers/models/` folder, with the
necessary files (configuration and modeling files for all frameworks requested, and maybe the processing files,
depending on your choices).
You will also see a doc file and tests for your new models. First you should run
```
make style
make fix-copies
```
and then you can start tweaking your model. You should:
- fill the doc file at `docs/source/model_doc/model_name.md`
- tweak the configuration and modeling files to your need
Once you're done, you can run the tests to ensure that they all pass:
```
python -m pytest ./tests/test_*<model_name>*.py
```
⚠ You should be careful about the classes preceded by the following line:️
```python
# Copied from transformers.[...]
```
This line ensures that the copy does not diverge from the source. If it *should* diverge, because the implementation
is different, this line needs to be deleted. If you don't delete this line and run `make fix-copies`,
your changes will be overwritten.
Once you have edited the files to fit your architecture, simply re-run the tests (and edit them if a change
is needed!) afterwards to make sure everything works as expected.
Once the files are generated and you are happy with your changes, here's a checklist to ensure that your contribution
will be merged quickly:
- You should run the `make fixup` utility to fix the style of the files and to ensure the code quality meets the
library's standards.
- You should add your model to the main README then run `make fix-copies`.
| transformers/templates/adding_a_new_model/README.md/0 | {
"file_path": "transformers/templates/adding_a_new_model/README.md",
"repo_id": "transformers",
"token_count": 2797
} | 342 |
Currently the following model proposals are available:
- <s>[BigBird (Google)](./ADD_BIG_BIRD.md)</s>
| transformers/templates/adding_a_new_model/open_model_proposals/README.md/0 | {
"file_path": "transformers/templates/adding_a_new_model/open_model_proposals/README.md",
"repo_id": "transformers",
"token_count": 34
} | 343 |
# coding=utf-8
# Copyright 2020 The HuggingFace Team Inc.
#
# Licensed under the Apache License, Version 2.0 (the "License");
# you may not use this file except in compliance with the License.
# You may obtain a clone of the License at
#
# http://www.apache.org/licenses/LICENSE-2.0
#
# Unless required by applicable law or agreed to in writing, software
# distributed under the License is distributed on an "AS IS" BASIS,
# WITHOUT WARRANTIES OR CONDITIONS OF ANY KIND, either express or implied.
# See the License for the specific language governing permissions and
# limitations under the License.
import unittest
from transformers import is_torch_available
from transformers.testing_utils import require_torch
if is_torch_available():
import torch
from transformers.generation import DisjunctiveConstraint
@require_torch
class ConstraintTest(unittest.TestCase):
def test_input_types(self):
# For consistency across different places the DisjunctiveConstraint is called,
# dc.token_ids is a list of integers. It is also initialized only by integers.
cset = [[1, 2, 4], [1, 2, 3, 4]]
dc = DisjunctiveConstraint(cset)
self.assertTrue(isinstance(dc.token_ids, list))
with self.assertRaises(ValueError):
DisjunctiveConstraint(torch.LongTensor([[1, 2, 4], [1, 2, 3]]))
with self.assertRaises(ValueError):
DisjunctiveConstraint([torch.LongTensor([1, 2, 4]), torch.LongTensor([1, 2, 3, 4, 5])])
def test_check_illegal_input(self):
# We can't have constraints that are complete subsets of another. This leads to a preverse
# interpretation of "constraint fulfillment": does generating [1,2,3] fulfill the constraint?
# It would mean that it generated [1,2] which fulfills it, but it's in the middle of potentially
# fulfilling [1,2,3,4]. If we believe that [1,2,3] does fulfill the constraint, then the algorithm
# will necessarily never reach [1,2,3,4], giving users a false sense of control (better to just not allow it).
cset = [[1, 2], [1, 2, 3, 4]]
with self.assertRaises(ValueError):
DisjunctiveConstraint(cset) # fails here
def test_example_progression(self):
cset = [[1, 2, 3], [1, 2, 4]]
dc = DisjunctiveConstraint(cset)
stepped, completed, reset = dc.update(1)
desired = stepped is True and completed is False and reset is False
self.assertTrue(desired)
self.assertTrue(not dc.completed)
self.assertTrue(dc.current_seq == [1])
stepped, completed, reset = dc.update(2)
desired = stepped is True and completed is False and reset is False
self.assertTrue(desired)
self.assertTrue(not dc.completed)
self.assertTrue(dc.current_seq == [1, 2])
stepped, completed, reset = dc.update(3)
desired = stepped is True and completed is True and reset is False
self.assertTrue(desired)
self.assertTrue(dc.completed) # Completed!
self.assertTrue(dc.current_seq == [1, 2, 3])
def test_example_progression_unequal_three_mid_and_reset(self):
cset = [[1, 2, 3], [1, 2, 4, 5], [1, 2, 5]]
dc = DisjunctiveConstraint(cset)
stepped, completed, reset = dc.update(1)
self.assertTrue(not dc.completed)
self.assertTrue(dc.current_seq == [1])
stepped, completed, reset = dc.update(2)
self.assertTrue(not dc.completed)
self.assertTrue(dc.current_seq == [1, 2])
stepped, completed, reset = dc.update(4)
self.assertTrue(not dc.completed)
self.assertTrue(dc.current_seq == [1, 2, 4])
stepped, completed, reset = dc.update(5)
self.assertTrue(dc.completed) # Completed!
self.assertTrue(dc.current_seq == [1, 2, 4, 5])
dc.reset()
stepped, completed, reset = dc.update(1)
self.assertTrue(not dc.completed)
self.assertTrue(dc.remaining() == 3)
self.assertTrue(dc.current_seq == [1])
stepped, completed, reset = dc.update(2)
self.assertTrue(not dc.completed)
self.assertTrue(dc.remaining() == 2)
self.assertTrue(dc.current_seq == [1, 2])
stepped, completed, reset = dc.update(5)
self.assertTrue(dc.completed) # Completed!
self.assertTrue(dc.remaining() == 0)
self.assertTrue(dc.current_seq == [1, 2, 5])
| transformers/tests/generation/test_beam_constraints.py/0 | {
"file_path": "transformers/tests/generation/test_beam_constraints.py",
"repo_id": "transformers",
"token_count": 1723
} | 344 |
# coding=utf-8
# Copyright 2020 The HuggingFace Team. All rights reserved.
#
# Licensed under the Apache License, Version 2.0 (the "License");
# you may not use this file except in compliance with the License.
# You may obtain a copy of the License at
#
# http://www.apache.org/licenses/LICENSE-2.0
#
# Unless required by applicable law or agreed to in writing, software
# distributed under the License is distributed on an "AS IS" BASIS,
# WITHOUT WARRANTIES OR CONDITIONS OF ANY KIND, either express or implied.
# See the License for the specific language governing permissions and
# limitations under the License.
from __future__ import annotations
import unittest
from transformers import AlbertConfig, is_tf_available
from transformers.models.auto import get_values
from transformers.testing_utils import require_tf, slow
from ...test_configuration_common import ConfigTester
from ...test_modeling_tf_common import TFModelTesterMixin, ids_tensor, random_attention_mask
from ...test_pipeline_mixin import PipelineTesterMixin
if is_tf_available():
import tensorflow as tf
from transformers import TF_MODEL_FOR_PRETRAINING_MAPPING
from transformers.models.albert.modeling_tf_albert import (
TF_ALBERT_PRETRAINED_MODEL_ARCHIVE_LIST,
TFAlbertForMaskedLM,
TFAlbertForMultipleChoice,
TFAlbertForPreTraining,
TFAlbertForQuestionAnswering,
TFAlbertForSequenceClassification,
TFAlbertForTokenClassification,
TFAlbertModel,
)
class TFAlbertModelTester:
def __init__(
self,
parent,
batch_size=13,
seq_length=7,
is_training=True,
use_input_mask=True,
use_token_type_ids=True,
use_labels=True,
vocab_size=99,
embedding_size=16,
hidden_size=32,
num_hidden_layers=2,
num_attention_heads=4,
intermediate_size=37,
hidden_act="gelu",
hidden_dropout_prob=0.1,
attention_probs_dropout_prob=0.1,
max_position_embeddings=512,
type_vocab_size=16,
type_sequence_label_size=2,
initializer_range=0.02,
num_labels=3,
num_choices=4,
scope=None,
):
self.parent = parent
self.batch_size = 13
self.seq_length = 7
self.is_training = True
self.use_input_mask = True
self.use_token_type_ids = True
self.use_labels = True
self.vocab_size = 99
self.embedding_size = 16
self.hidden_size = 32
self.num_hidden_layers = 2
self.num_attention_heads = 4
self.intermediate_size = 37
self.hidden_act = "gelu"
self.hidden_dropout_prob = 0.1
self.attention_probs_dropout_prob = 0.1
self.max_position_embeddings = 512
self.type_vocab_size = 16
self.type_sequence_label_size = 2
self.initializer_range = 0.02
self.num_labels = 3
self.num_choices = 4
self.scope = None
def prepare_config_and_inputs(self):
input_ids = ids_tensor([self.batch_size, self.seq_length], self.vocab_size)
input_mask = None
if self.use_input_mask:
input_mask = random_attention_mask([self.batch_size, self.seq_length])
token_type_ids = None
if self.use_token_type_ids:
token_type_ids = ids_tensor([self.batch_size, self.seq_length], self.type_vocab_size)
sequence_labels = None
token_labels = None
choice_labels = None
if self.use_labels:
sequence_labels = ids_tensor([self.batch_size], self.type_sequence_label_size)
token_labels = ids_tensor([self.batch_size, self.seq_length], self.num_labels)
choice_labels = ids_tensor([self.batch_size], self.num_choices)
config = AlbertConfig(
vocab_size=self.vocab_size,
hidden_size=self.hidden_size,
embedding_size=self.embedding_size,
num_hidden_layers=self.num_hidden_layers,
num_attention_heads=self.num_attention_heads,
intermediate_size=self.intermediate_size,
hidden_act=self.hidden_act,
hidden_dropout_prob=self.hidden_dropout_prob,
attention_probs_dropout_prob=self.attention_probs_dropout_prob,
max_position_embeddings=self.max_position_embeddings,
type_vocab_size=self.type_vocab_size,
initializer_range=self.initializer_range,
)
return config, input_ids, token_type_ids, input_mask, sequence_labels, token_labels, choice_labels
def create_and_check_albert_model(
self, config, input_ids, token_type_ids, input_mask, sequence_labels, token_labels, choice_labels
):
model = TFAlbertModel(config=config)
# inputs = {'input_ids': input_ids,
# 'attention_mask': input_mask,
# 'token_type_ids': token_type_ids}
# sequence_output, pooled_output = model(**inputs)
inputs = {"input_ids": input_ids, "attention_mask": input_mask, "token_type_ids": token_type_ids}
result = model(inputs)
inputs = [input_ids, input_mask]
result = model(inputs)
result = model(input_ids)
self.parent.assertEqual(result.last_hidden_state.shape, (self.batch_size, self.seq_length, self.hidden_size))
self.parent.assertEqual(result.pooler_output.shape, (self.batch_size, self.hidden_size))
def create_and_check_albert_for_pretraining(
self, config, input_ids, token_type_ids, input_mask, sequence_labels, token_labels, choice_labels
):
config.num_labels = self.num_labels
model = TFAlbertForPreTraining(config=config)
inputs = {"input_ids": input_ids, "attention_mask": input_mask, "token_type_ids": token_type_ids}
result = model(inputs)
self.parent.assertEqual(result.prediction_logits.shape, (self.batch_size, self.seq_length, self.vocab_size))
self.parent.assertEqual(result.sop_logits.shape, (self.batch_size, self.num_labels))
def create_and_check_albert_for_masked_lm(
self, config, input_ids, token_type_ids, input_mask, sequence_labels, token_labels, choice_labels
):
model = TFAlbertForMaskedLM(config=config)
inputs = {"input_ids": input_ids, "attention_mask": input_mask, "token_type_ids": token_type_ids}
result = model(inputs)
self.parent.assertEqual(result.logits.shape, (self.batch_size, self.seq_length, self.vocab_size))
def create_and_check_albert_for_sequence_classification(
self, config, input_ids, token_type_ids, input_mask, sequence_labels, token_labels, choice_labels
):
config.num_labels = self.num_labels
model = TFAlbertForSequenceClassification(config=config)
inputs = {"input_ids": input_ids, "attention_mask": input_mask, "token_type_ids": token_type_ids}
result = model(inputs)
self.parent.assertEqual(result.logits.shape, (self.batch_size, self.num_labels))
def create_and_check_albert_for_question_answering(
self, config, input_ids, token_type_ids, input_mask, sequence_labels, token_labels, choice_labels
):
model = TFAlbertForQuestionAnswering(config=config)
inputs = {"input_ids": input_ids, "attention_mask": input_mask, "token_type_ids": token_type_ids}
result = model(inputs)
self.parent.assertEqual(result.start_logits.shape, (self.batch_size, self.seq_length))
self.parent.assertEqual(result.end_logits.shape, (self.batch_size, self.seq_length))
def create_and_check_albert_for_multiple_choice(
self, config, input_ids, token_type_ids, input_mask, sequence_labels, token_labels, choice_labels
):
config.num_choices = self.num_choices
model = TFAlbertForMultipleChoice(config=config)
multiple_choice_inputs_ids = tf.tile(tf.expand_dims(input_ids, 1), (1, self.num_choices, 1))
multiple_choice_input_mask = tf.tile(tf.expand_dims(input_mask, 1), (1, self.num_choices, 1))
multiple_choice_token_type_ids = tf.tile(tf.expand_dims(token_type_ids, 1), (1, self.num_choices, 1))
inputs = {
"input_ids": multiple_choice_inputs_ids,
"attention_mask": multiple_choice_input_mask,
"token_type_ids": multiple_choice_token_type_ids,
}
result = model(inputs)
self.parent.assertListEqual(list(result["logits"].shape), [self.batch_size, self.num_choices])
def create_and_check_albert_for_token_classification(
self, config, input_ids, token_type_ids, input_mask, sequence_labels, token_labels, choice_labels
):
config.num_labels = self.num_labels
model = TFAlbertForTokenClassification(config=config)
inputs = {
"input_ids": input_ids,
"attention_mask": input_mask,
"token_type_ids": token_type_ids,
}
result = model(inputs)
self.parent.assertListEqual(list(result["logits"].shape), [self.batch_size, self.seq_length, self.num_labels])
def prepare_config_and_inputs_for_common(self):
config_and_inputs = self.prepare_config_and_inputs()
(
config,
input_ids,
token_type_ids,
input_mask,
sequence_labels,
token_labels,
choice_labels,
) = config_and_inputs
inputs_dict = {"input_ids": input_ids, "token_type_ids": token_type_ids, "attention_mask": input_mask}
return config, inputs_dict
@require_tf
class TFAlbertModelTest(TFModelTesterMixin, PipelineTesterMixin, unittest.TestCase):
all_model_classes = (
(
TFAlbertModel,
TFAlbertForPreTraining,
TFAlbertForMaskedLM,
TFAlbertForSequenceClassification,
TFAlbertForQuestionAnswering,
TFAlbertForTokenClassification,
TFAlbertForMultipleChoice,
)
if is_tf_available()
else ()
)
pipeline_model_mapping = (
{
"feature-extraction": TFAlbertModel,
"fill-mask": TFAlbertForMaskedLM,
"question-answering": TFAlbertForQuestionAnswering,
"text-classification": TFAlbertForSequenceClassification,
"token-classification": TFAlbertForTokenClassification,
"zero-shot": TFAlbertForSequenceClassification,
}
if is_tf_available()
else {}
)
test_head_masking = False
test_onnx = False
# special case for ForPreTraining model
def _prepare_for_class(self, inputs_dict, model_class, return_labels=False):
inputs_dict = super()._prepare_for_class(inputs_dict, model_class, return_labels=return_labels)
if return_labels:
if model_class in get_values(TF_MODEL_FOR_PRETRAINING_MAPPING):
inputs_dict["sentence_order_label"] = tf.zeros(self.model_tester.batch_size, dtype=tf.int32)
return inputs_dict
def setUp(self):
self.model_tester = TFAlbertModelTester(self)
self.config_tester = ConfigTester(self, config_class=AlbertConfig, hidden_size=37)
def test_config(self):
self.config_tester.run_common_tests()
def test_albert_model(self):
config_and_inputs = self.model_tester.prepare_config_and_inputs()
self.model_tester.create_and_check_albert_model(*config_and_inputs)
def test_for_pretraining(self):
config_and_inputs = self.model_tester.prepare_config_and_inputs()
self.model_tester.create_and_check_albert_for_pretraining(*config_and_inputs)
def test_for_masked_lm(self):
config_and_inputs = self.model_tester.prepare_config_and_inputs()
self.model_tester.create_and_check_albert_for_masked_lm(*config_and_inputs)
def test_for_multiple_choice(self):
config_and_inputs = self.model_tester.prepare_config_and_inputs()
self.model_tester.create_and_check_albert_for_multiple_choice(*config_and_inputs)
def test_for_sequence_classification(self):
config_and_inputs = self.model_tester.prepare_config_and_inputs()
self.model_tester.create_and_check_albert_for_sequence_classification(*config_and_inputs)
def test_for_question_answering(self):
config_and_inputs = self.model_tester.prepare_config_and_inputs()
self.model_tester.create_and_check_albert_for_question_answering(*config_and_inputs)
@slow
def test_model_from_pretrained(self):
for model_name in TF_ALBERT_PRETRAINED_MODEL_ARCHIVE_LIST[:1]:
model = TFAlbertModel.from_pretrained(model_name)
self.assertIsNotNone(model)
@require_tf
class TFAlbertModelIntegrationTest(unittest.TestCase):
@slow
def test_inference_masked_lm(self):
model = TFAlbertForPreTraining.from_pretrained("albert-base-v2")
input_ids = tf.constant([[0, 1, 2, 3, 4, 5]])
output = model(input_ids)[0]
expected_shape = [1, 6, 30000]
self.assertEqual(output.shape, expected_shape)
expected_slice = tf.constant(
[
[
[4.595668, 0.74462754, -1.818147],
[4.5954347, 0.7454184, -1.8188258],
[4.5954905, 0.7448235, -1.8182316],
]
]
)
tf.debugging.assert_near(output[:, :3, :3], expected_slice, atol=1e-4)
| transformers/tests/models/albert/test_modeling_tf_albert.py/0 | {
"file_path": "transformers/tests/models/albert/test_modeling_tf_albert.py",
"repo_id": "transformers",
"token_count": 5940
} | 345 |
# coding=utf-8
# Copyright 2020 The HuggingFace Team. All rights reserved.
#
# Licensed under the Apache License, Version 2.0 (the "License");
# you may not use this file except in compliance with the License.
# You may obtain a copy of the License at
#
# http://www.apache.org/licenses/LICENSE-2.0
#
# Unless required by applicable law or agreed to in writing, software
# distributed under the License is distributed on an "AS IS" BASIS,
# WITHOUT WARRANTIES OR CONDITIONS OF ANY KIND, either express or implied.
# See the License for the specific language governing permissions and
# limitations under the License.
from __future__ import annotations
import copy
import tempfile
import unittest
from transformers import CONFIG_MAPPING, AutoConfig, BertConfig, GPT2Config, T5Config, TapasConfig, is_tf_available
from transformers.testing_utils import (
DUMMY_UNKNOWN_IDENTIFIER,
SMALL_MODEL_IDENTIFIER,
RequestCounter,
require_tensorflow_probability,
require_tf,
slow,
)
from ..bert.test_modeling_bert import BertModelTester
if is_tf_available():
from transformers import (
TFAutoModel,
TFAutoModelForCausalLM,
TFAutoModelForMaskedLM,
TFAutoModelForPreTraining,
TFAutoModelForQuestionAnswering,
TFAutoModelForSeq2SeqLM,
TFAutoModelForSequenceClassification,
TFAutoModelForTableQuestionAnswering,
TFAutoModelForTokenClassification,
TFAutoModelWithLMHead,
TFBertForMaskedLM,
TFBertForPreTraining,
TFBertForQuestionAnswering,
TFBertForSequenceClassification,
TFBertModel,
TFFunnelBaseModel,
TFFunnelModel,
TFGPT2LMHeadModel,
TFRobertaForMaskedLM,
TFT5ForConditionalGeneration,
TFTapasForQuestionAnswering,
)
from transformers.models.auto.modeling_tf_auto import (
TF_MODEL_FOR_CAUSAL_LM_MAPPING,
TF_MODEL_FOR_MASKED_LM_MAPPING,
TF_MODEL_FOR_PRETRAINING_MAPPING,
TF_MODEL_FOR_QUESTION_ANSWERING_MAPPING,
TF_MODEL_FOR_SEQUENCE_CLASSIFICATION_MAPPING,
TF_MODEL_FOR_TOKEN_CLASSIFICATION_MAPPING,
TF_MODEL_MAPPING,
)
from transformers.models.bert.modeling_tf_bert import TF_BERT_PRETRAINED_MODEL_ARCHIVE_LIST
from transformers.models.gpt2.modeling_tf_gpt2 import TF_GPT2_PRETRAINED_MODEL_ARCHIVE_LIST
from transformers.models.t5.modeling_tf_t5 import TF_T5_PRETRAINED_MODEL_ARCHIVE_LIST
from transformers.models.tapas.modeling_tf_tapas import TF_TAPAS_PRETRAINED_MODEL_ARCHIVE_LIST
class NewModelConfig(BertConfig):
model_type = "new-model"
if is_tf_available():
class TFNewModel(TFBertModel):
config_class = NewModelConfig
@require_tf
class TFAutoModelTest(unittest.TestCase):
@slow
def test_model_from_pretrained(self):
model_name = "bert-base-cased"
config = AutoConfig.from_pretrained(model_name)
self.assertIsNotNone(config)
self.assertIsInstance(config, BertConfig)
model = TFAutoModel.from_pretrained(model_name)
self.assertIsNotNone(model)
self.assertIsInstance(model, TFBertModel)
@slow
def test_model_for_pretraining_from_pretrained(self):
model_name = "bert-base-cased"
config = AutoConfig.from_pretrained(model_name)
self.assertIsNotNone(config)
self.assertIsInstance(config, BertConfig)
model = TFAutoModelForPreTraining.from_pretrained(model_name)
self.assertIsNotNone(model)
self.assertIsInstance(model, TFBertForPreTraining)
@slow
def test_model_for_causal_lm(self):
for model_name in TF_GPT2_PRETRAINED_MODEL_ARCHIVE_LIST[:1]:
config = AutoConfig.from_pretrained(model_name)
self.assertIsNotNone(config)
self.assertIsInstance(config, GPT2Config)
model = TFAutoModelForCausalLM.from_pretrained(model_name)
model, loading_info = TFAutoModelForCausalLM.from_pretrained(model_name, output_loading_info=True)
self.assertIsNotNone(model)
self.assertIsInstance(model, TFGPT2LMHeadModel)
@slow
def test_lmhead_model_from_pretrained(self):
for model_name in TF_BERT_PRETRAINED_MODEL_ARCHIVE_LIST[:1]:
config = AutoConfig.from_pretrained(model_name)
self.assertIsNotNone(config)
self.assertIsInstance(config, BertConfig)
model = TFAutoModelWithLMHead.from_pretrained(model_name)
self.assertIsNotNone(model)
self.assertIsInstance(model, TFBertForMaskedLM)
@slow
def test_model_for_masked_lm(self):
for model_name in TF_BERT_PRETRAINED_MODEL_ARCHIVE_LIST[:1]:
config = AutoConfig.from_pretrained(model_name)
self.assertIsNotNone(config)
self.assertIsInstance(config, BertConfig)
model = TFAutoModelForMaskedLM.from_pretrained(model_name)
model, loading_info = TFAutoModelForMaskedLM.from_pretrained(model_name, output_loading_info=True)
self.assertIsNotNone(model)
self.assertIsInstance(model, TFBertForMaskedLM)
@slow
def test_model_for_encoder_decoder_lm(self):
for model_name in TF_T5_PRETRAINED_MODEL_ARCHIVE_LIST[:1]:
config = AutoConfig.from_pretrained(model_name)
self.assertIsNotNone(config)
self.assertIsInstance(config, T5Config)
model = TFAutoModelForSeq2SeqLM.from_pretrained(model_name)
model, loading_info = TFAutoModelForSeq2SeqLM.from_pretrained(model_name, output_loading_info=True)
self.assertIsNotNone(model)
self.assertIsInstance(model, TFT5ForConditionalGeneration)
@slow
def test_sequence_classification_model_from_pretrained(self):
# for model_name in TF_BERT_PRETRAINED_MODEL_ARCHIVE_LIST[:1]:
for model_name in ["bert-base-uncased"]:
config = AutoConfig.from_pretrained(model_name)
self.assertIsNotNone(config)
self.assertIsInstance(config, BertConfig)
model = TFAutoModelForSequenceClassification.from_pretrained(model_name)
self.assertIsNotNone(model)
self.assertIsInstance(model, TFBertForSequenceClassification)
@slow
def test_question_answering_model_from_pretrained(self):
# for model_name in TF_BERT_PRETRAINED_MODEL_ARCHIVE_LIST[:1]:
for model_name in ["bert-base-uncased"]:
config = AutoConfig.from_pretrained(model_name)
self.assertIsNotNone(config)
self.assertIsInstance(config, BertConfig)
model = TFAutoModelForQuestionAnswering.from_pretrained(model_name)
self.assertIsNotNone(model)
self.assertIsInstance(model, TFBertForQuestionAnswering)
@slow
@require_tensorflow_probability
def test_table_question_answering_model_from_pretrained(self):
for model_name in TF_TAPAS_PRETRAINED_MODEL_ARCHIVE_LIST[5:6]:
config = AutoConfig.from_pretrained(model_name)
self.assertIsNotNone(config)
self.assertIsInstance(config, TapasConfig)
model = TFAutoModelForTableQuestionAnswering.from_pretrained(model_name)
model, loading_info = TFAutoModelForTableQuestionAnswering.from_pretrained(
model_name, output_loading_info=True
)
self.assertIsNotNone(model)
self.assertIsInstance(model, TFTapasForQuestionAnswering)
def test_from_pretrained_identifier(self):
model = TFAutoModelWithLMHead.from_pretrained(SMALL_MODEL_IDENTIFIER)
self.assertIsInstance(model, TFBertForMaskedLM)
self.assertEqual(model.num_parameters(), 14410)
self.assertEqual(model.num_parameters(only_trainable=True), 14410)
def test_from_identifier_from_model_type(self):
model = TFAutoModelWithLMHead.from_pretrained(DUMMY_UNKNOWN_IDENTIFIER)
self.assertIsInstance(model, TFRobertaForMaskedLM)
self.assertEqual(model.num_parameters(), 14410)
self.assertEqual(model.num_parameters(only_trainable=True), 14410)
def test_from_pretrained_with_tuple_values(self):
# For the auto model mapping, FunnelConfig has two models: FunnelModel and FunnelBaseModel
model = TFAutoModel.from_pretrained("sgugger/funnel-random-tiny")
self.assertIsInstance(model, TFFunnelModel)
config = copy.deepcopy(model.config)
config.architectures = ["FunnelBaseModel"]
model = TFAutoModel.from_config(config)
model.build_in_name_scope()
self.assertIsInstance(model, TFFunnelBaseModel)
with tempfile.TemporaryDirectory() as tmp_dir:
model.save_pretrained(tmp_dir)
model = TFAutoModel.from_pretrained(tmp_dir)
self.assertIsInstance(model, TFFunnelBaseModel)
def test_new_model_registration(self):
try:
AutoConfig.register("new-model", NewModelConfig)
auto_classes = [
TFAutoModel,
TFAutoModelForCausalLM,
TFAutoModelForMaskedLM,
TFAutoModelForPreTraining,
TFAutoModelForQuestionAnswering,
TFAutoModelForSequenceClassification,
TFAutoModelForTokenClassification,
]
for auto_class in auto_classes:
with self.subTest(auto_class.__name__):
# Wrong config class will raise an error
with self.assertRaises(ValueError):
auto_class.register(BertConfig, TFNewModel)
auto_class.register(NewModelConfig, TFNewModel)
# Trying to register something existing in the Transformers library will raise an error
with self.assertRaises(ValueError):
auto_class.register(BertConfig, TFBertModel)
# Now that the config is registered, it can be used as any other config with the auto-API
tiny_config = BertModelTester(self).get_config()
config = NewModelConfig(**tiny_config.to_dict())
model = auto_class.from_config(config)
model.build_in_name_scope()
self.assertIsInstance(model, TFNewModel)
with tempfile.TemporaryDirectory() as tmp_dir:
model.save_pretrained(tmp_dir)
new_model = auto_class.from_pretrained(tmp_dir)
self.assertIsInstance(new_model, TFNewModel)
finally:
if "new-model" in CONFIG_MAPPING._extra_content:
del CONFIG_MAPPING._extra_content["new-model"]
for mapping in (
TF_MODEL_MAPPING,
TF_MODEL_FOR_PRETRAINING_MAPPING,
TF_MODEL_FOR_QUESTION_ANSWERING_MAPPING,
TF_MODEL_FOR_SEQUENCE_CLASSIFICATION_MAPPING,
TF_MODEL_FOR_TOKEN_CLASSIFICATION_MAPPING,
TF_MODEL_FOR_CAUSAL_LM_MAPPING,
TF_MODEL_FOR_MASKED_LM_MAPPING,
):
if NewModelConfig in mapping._extra_content:
del mapping._extra_content[NewModelConfig]
def test_repo_not_found(self):
with self.assertRaisesRegex(
EnvironmentError, "bert-base is not a local folder and is not a valid model identifier"
):
_ = TFAutoModel.from_pretrained("bert-base")
def test_revision_not_found(self):
with self.assertRaisesRegex(
EnvironmentError, r"aaaaaa is not a valid git identifier \(branch name, tag name or commit id\)"
):
_ = TFAutoModel.from_pretrained(DUMMY_UNKNOWN_IDENTIFIER, revision="aaaaaa")
def test_model_file_not_found(self):
with self.assertRaisesRegex(
EnvironmentError,
"hf-internal-testing/config-no-model does not appear to have a file named pytorch_model.bin",
):
_ = TFAutoModel.from_pretrained("hf-internal-testing/config-no-model")
def test_model_from_pt_suggestion(self):
with self.assertRaisesRegex(EnvironmentError, "Use `from_pt=True` to load this model"):
_ = TFAutoModel.from_pretrained("hf-internal-testing/tiny-bert-pt-only")
def test_cached_model_has_minimum_calls_to_head(self):
# Make sure we have cached the model.
_ = TFAutoModel.from_pretrained("hf-internal-testing/tiny-random-bert")
with RequestCounter() as counter:
_ = TFAutoModel.from_pretrained("hf-internal-testing/tiny-random-bert")
self.assertEqual(counter["GET"], 0)
self.assertEqual(counter["HEAD"], 1)
self.assertEqual(counter.total_calls, 1)
# With a sharded checkpoint
_ = TFAutoModel.from_pretrained("ArthurZ/tiny-random-bert-sharded")
with RequestCounter() as counter:
_ = TFAutoModel.from_pretrained("ArthurZ/tiny-random-bert-sharded")
self.assertEqual(counter["GET"], 0)
self.assertEqual(counter["HEAD"], 1)
self.assertEqual(counter.total_calls, 1)
| transformers/tests/models/auto/test_modeling_tf_auto.py/0 | {
"file_path": "transformers/tests/models/auto/test_modeling_tf_auto.py",
"repo_id": "transformers",
"token_count": 5870
} | 346 |
# coding=utf-8
# Copyright 2020 The HuggingFace Team. All rights reserved.
#
# Licensed under the Apache License, Version 2.0 (the "License");
# you may not use this file except in compliance with the License.
# You may obtain a copy of the License at
#
# http://www.apache.org/licenses/LICENSE-2.0
#
# Unless required by applicable law or agreed to in writing, software
# distributed under the License is distributed on an "AS IS" BASIS,
# WITHOUT WARRANTIES OR CONDITIONS OF ANY KIND, either express or implied.
# See the License for the specific language governing permissions and
# limitations under the License.
import os
import pickle
import unittest
from transformers import AutoTokenizer
from transformers.models.bert.tokenization_bert import BertTokenizer
from transformers.models.bert_japanese.tokenization_bert_japanese import (
VOCAB_FILES_NAMES,
BertJapaneseTokenizer,
CharacterTokenizer,
JumanppTokenizer,
MecabTokenizer,
SudachiTokenizer,
WordpieceTokenizer,
)
from transformers.testing_utils import custom_tokenizers, require_jumanpp, require_sudachi
from ...test_tokenization_common import TokenizerTesterMixin
@custom_tokenizers
class BertJapaneseTokenizationTest(TokenizerTesterMixin, unittest.TestCase):
tokenizer_class = BertJapaneseTokenizer
test_rust_tokenizer = False
space_between_special_tokens = True
def setUp(self):
super().setUp()
vocab_tokens = [
"[UNK]",
"[CLS]",
"[SEP]",
"こんにちは",
"こん",
"にちは",
"ばんは",
"##こん",
"##にちは",
"##ばんは",
"世界",
"##世界",
"、",
"##、",
"。",
"##。",
]
self.vocab_file = os.path.join(self.tmpdirname, VOCAB_FILES_NAMES["vocab_file"])
with open(self.vocab_file, "w", encoding="utf-8") as vocab_writer:
vocab_writer.write("".join([x + "\n" for x in vocab_tokens]))
def get_input_output_texts(self, tokenizer):
input_text = "こんにちは、世界。 \nこんばんは、世界。"
output_text = "こんにちは 、 世界 。 こんばんは 、 世界 。"
return input_text, output_text
def get_clean_sequence(self, tokenizer):
input_text, output_text = self.get_input_output_texts(tokenizer)
ids = tokenizer.encode(output_text, add_special_tokens=False)
text = tokenizer.decode(ids, clean_up_tokenization_spaces=False)
return text, ids
def test_pretokenized_inputs(self):
pass # TODO add if relevant
def test_maximum_encoding_length_pair_input(self):
pass # TODO add if relevant
def test_maximum_encoding_length_single_input(self):
pass # TODO add if relevant
def test_full_tokenizer(self):
tokenizer = self.tokenizer_class(self.vocab_file)
tokens = tokenizer.tokenize("こんにちは、世界。\nこんばんは、世界。")
self.assertListEqual(tokens, ["こんにちは", "、", "世界", "。", "こん", "##ばんは", "、", "世界", "。"])
self.assertListEqual(tokenizer.convert_tokens_to_ids(tokens), [3, 12, 10, 14, 4, 9, 12, 10, 14])
def test_pickle_mecab_tokenizer(self):
tokenizer = self.tokenizer_class(self.vocab_file, word_tokenizer_type="mecab")
self.assertIsNotNone(tokenizer)
text = "こんにちは、世界。\nこんばんは、世界。"
tokens = tokenizer.tokenize(text)
self.assertListEqual(tokens, ["こんにちは", "、", "世界", "。", "こん", "##ばんは", "、", "世界", "。"])
self.assertListEqual(tokenizer.convert_tokens_to_ids(tokens), [3, 12, 10, 14, 4, 9, 12, 10, 14])
filename = os.path.join(self.tmpdirname, "tokenizer.bin")
with open(filename, "wb") as handle:
pickle.dump(tokenizer, handle)
with open(filename, "rb") as handle:
tokenizer_new = pickle.load(handle)
tokens_loaded = tokenizer_new.tokenize(text)
self.assertListEqual(tokens, tokens_loaded)
def test_mecab_tokenizer_ipadic(self):
tokenizer = MecabTokenizer(mecab_dic="ipadic")
self.assertListEqual(
tokenizer.tokenize(" \tアップルストアでiPhone8 が \n 発売された 。 "),
["アップルストア", "で", "iPhone", "8", "が", "発売", "さ", "れ", "た", "。"],
)
def test_mecab_tokenizer_unidic_lite(self):
try:
tokenizer = MecabTokenizer(mecab_dic="unidic_lite")
except ModuleNotFoundError:
return
self.assertListEqual(
tokenizer.tokenize(" \tアップルストアでiPhone8 が \n 発売された 。 "),
["アップル", "ストア", "で", "iPhone", "8", "が", "発売", "さ", "れ", "た", "。"],
)
def test_mecab_tokenizer_unidic(self):
try:
tokenizer = MecabTokenizer(mecab_dic="unidic")
except ModuleNotFoundError:
return
self.assertListEqual(
tokenizer.tokenize(" \tアップルストアでiPhone8 が \n 発売された 。 "),
["アップル", "ストア", "で", "iPhone", "8", "が", "発売", "さ", "れ", "た", "。"],
)
def test_mecab_tokenizer_lower(self):
tokenizer = MecabTokenizer(do_lower_case=True, mecab_dic="ipadic")
self.assertListEqual(
tokenizer.tokenize(" \tアップルストアでiPhone8 が \n 発売された 。 "),
["アップルストア", "で", "iphone", "8", "が", "発売", "さ", "れ", "た", "。"],
)
def test_mecab_tokenizer_with_option(self):
try:
tokenizer = MecabTokenizer(
do_lower_case=True, normalize_text=False, mecab_option="-d /usr/local/lib/mecab/dic/jumandic"
)
except RuntimeError:
# if dict doesn't exist in the system, previous code raises this error.
return
self.assertListEqual(
tokenizer.tokenize(" \tアップルストアでiPhone8 が \n 発売された 。 "),
["アップルストア", "で", "iPhone", "8", "が", "発売", "さ", "れた", "\u3000", "。"],
)
def test_mecab_tokenizer_no_normalize(self):
tokenizer = MecabTokenizer(normalize_text=False, mecab_dic="ipadic")
self.assertListEqual(
tokenizer.tokenize(" \tアップルストアでiPhone8 が \n 発売された 。 "),
["アップルストア", "で", "iPhone", "8", "が", "発売", "さ", "れ", "た", " ", "。"],
)
@require_sudachi
def test_pickle_sudachi_tokenizer(self):
tokenizer = self.tokenizer_class(self.vocab_file, word_tokenizer_type="sudachi")
self.assertIsNotNone(tokenizer)
text = "こんにちは、世界。\nこんばんは、世界。"
tokens = tokenizer.tokenize(text)
self.assertListEqual(tokens, ["こんにちは", "、", "世界", "。", "こん", "##ばんは", "、", "世界", "。"])
self.assertListEqual(tokenizer.convert_tokens_to_ids(tokens), [3, 12, 10, 14, 4, 9, 12, 10, 14])
filename = os.path.join(self.tmpdirname, "tokenizer.bin")
with open(filename, "wb") as handle:
pickle.dump(tokenizer, handle)
with open(filename, "rb") as handle:
tokenizer_new = pickle.load(handle)
tokens_loaded = tokenizer_new.tokenize(text)
self.assertListEqual(tokens, tokens_loaded)
@require_sudachi
def test_sudachi_tokenizer_core(self):
tokenizer = SudachiTokenizer(sudachi_dict_type="core")
# fmt: off
self.assertListEqual(
tokenizer.tokenize(" \tアップルストアでiPhone8 が \n 発売された 。 "),
[" ", "\t", "アップル", "ストア", "で", "iPhone", "8", " ", "が", " ", " ", "\n ", "発売", "さ", "れ", "た", " ", "。", " ", " "],
)
# fmt: on
@require_sudachi
def test_sudachi_tokenizer_split_mode_A(self):
tokenizer = SudachiTokenizer(sudachi_dict_type="core", sudachi_split_mode="A")
self.assertListEqual(tokenizer.tokenize("外国人参政権"), ["外国", "人", "参政", "権"])
@require_sudachi
def test_sudachi_tokenizer_split_mode_B(self):
tokenizer = SudachiTokenizer(sudachi_dict_type="core", sudachi_split_mode="B")
self.assertListEqual(tokenizer.tokenize("外国人参政権"), ["外国人", "参政権"])
@require_sudachi
def test_sudachi_tokenizer_split_mode_C(self):
tokenizer = SudachiTokenizer(sudachi_dict_type="core", sudachi_split_mode="C")
self.assertListEqual(tokenizer.tokenize("外国人参政権"), ["外国人参政権"])
@require_sudachi
def test_sudachi_tokenizer_lower(self):
tokenizer = SudachiTokenizer(do_lower_case=True, sudachi_dict_type="core")
self.assertListEqual(tokenizer.tokenize(" \tアップルストアでiPhone8 が \n 発売された 。 "),[" ", "\t", "アップル", "ストア", "で", "iphone", "8", " ", "が", " ", " ", "\n ", "発売", "さ", "れ", "た", " ", "。", " ", " "]) # fmt: skip
@require_sudachi
def test_sudachi_tokenizer_no_normalize(self):
tokenizer = SudachiTokenizer(normalize_text=False, sudachi_dict_type="core")
self.assertListEqual(tokenizer.tokenize(" \tアップルストアでiPhone8 が \n 発売された 。 "),[" ", "\t", "アップル", "ストア", "で", "iPhone", "8", " ", "が", " ", " ", "\n ", "発売", "さ", "れ", "た", "\u3000", "。", " ", " "]) # fmt: skip
@require_sudachi
def test_sudachi_tokenizer_trim_whitespace(self):
tokenizer = SudachiTokenizer(trim_whitespace=True, sudachi_dict_type="core")
self.assertListEqual(
tokenizer.tokenize(" \tアップルストアでiPhone8 が \n 発売された 。 "),
["アップル", "ストア", "で", "iPhone", "8", "が", "発売", "さ", "れ", "た", "。"],
)
@require_jumanpp
def test_pickle_jumanpp_tokenizer(self):
tokenizer = self.tokenizer_class(self.vocab_file, word_tokenizer_type="jumanpp")
self.assertIsNotNone(tokenizer)
text = "こんにちは、世界。\nこんばんは、世界。"
tokens = tokenizer.tokenize(text)
self.assertListEqual(tokens, ["こんにちは", "、", "世界", "。", "こん", "##ばんは", "、", "世界", "。"])
self.assertListEqual(tokenizer.convert_tokens_to_ids(tokens), [3, 12, 10, 14, 4, 9, 12, 10, 14])
filename = os.path.join(self.tmpdirname, "tokenizer.bin")
with open(filename, "wb") as handle:
pickle.dump(tokenizer, handle)
with open(filename, "rb") as handle:
tokenizer_new = pickle.load(handle)
tokens_loaded = tokenizer_new.tokenize(text)
self.assertListEqual(tokens, tokens_loaded)
@require_jumanpp
def test_jumanpp_tokenizer(self):
tokenizer = JumanppTokenizer()
self.assertListEqual(
tokenizer.tokenize(" \tアップルストアでiPhone8 が \n 発売された 。 "),["アップル", "ストア", "で", "iPhone", "8", "\u3000", "が", "\u3000", "\u3000", "\u3000", "発売", "さ", "れた", "\u3000", "。"]) # fmt: skip
@require_jumanpp
def test_jumanpp_tokenizer_lower(self):
tokenizer = JumanppTokenizer(do_lower_case=True)
self.assertListEqual(tokenizer.tokenize(" \tアップルストアでiPhone8 が \n 発売された 。 "),["アップル", "ストア", "で", "iphone", "8", "\u3000", "が", "\u3000", "\u3000", "\u3000", "発売", "さ", "れた", "\u3000", "。"],) # fmt: skip
@require_jumanpp
def test_jumanpp_tokenizer_no_normalize(self):
tokenizer = JumanppTokenizer(normalize_text=False)
self.assertListEqual(tokenizer.tokenize(" \tアップルストアでiPhone8 が \n 発売された 。 "),["ア", "ッ", "フ", "゚", "ル", "ストア", "で", "iPhone", "8", "\u3000", "が", "\u3000", "\u3000", "\u3000", "発売", "さ", "れた", "\u3000", "。"],) # fmt: skip
@require_jumanpp
def test_jumanpp_tokenizer_trim_whitespace(self):
tokenizer = JumanppTokenizer(trim_whitespace=True)
self.assertListEqual(
tokenizer.tokenize(" \tアップルストアでiPhone8 が \n 発売された 。 "),
["アップル", "ストア", "で", "iPhone", "8", "が", "発売", "さ", "れた", "。"],
)
@require_jumanpp
def test_jumanpp_tokenizer_ext(self):
tokenizer = JumanppTokenizer()
self.assertListEqual(
tokenizer.tokenize("ありがとうございますm(_ _)m見つけるのが大変です。"),
["ありがとう", "ございます", "m(_ _)m", "見つける", "の", "が", "大変です", "。"],
)
def test_wordpiece_tokenizer(self):
vocab_tokens = ["[UNK]", "[CLS]", "[SEP]", "こんにちは", "こん", "にちは", "ばんは", "##こん", "##にちは", "##ばんは"] # fmt: skip
vocab = {}
for i, token in enumerate(vocab_tokens):
vocab[token] = i
tokenizer = WordpieceTokenizer(vocab=vocab, unk_token="[UNK]")
self.assertListEqual(tokenizer.tokenize(""), [])
self.assertListEqual(tokenizer.tokenize("こんにちは"), ["こんにちは"])
self.assertListEqual(tokenizer.tokenize("こんばんは"), ["こん", "##ばんは"])
self.assertListEqual(tokenizer.tokenize("こんばんは こんばんにちは こんにちは"), ["こん", "##ばんは", "[UNK]", "こんにちは"]) # fmt: skip
def test_sentencepiece_tokenizer(self):
tokenizer = BertJapaneseTokenizer.from_pretrained("nlp-waseda/roberta-base-japanese-with-auto-jumanpp")
subword_tokenizer = tokenizer.subword_tokenizer
tokens = subword_tokenizer.tokenize("国境 の 長い トンネル を 抜ける と 雪国 であった 。")
self.assertListEqual(tokens, ["▁国境", "▁の", "▁長い", "▁トンネル", "▁を", "▁抜ける", "▁と", "▁雪", "国", "▁であった", "▁。"]) # fmt: skip
tokens = subword_tokenizer.tokenize("こんばんは こんばん にち は こんにちは")
self.assertListEqual(tokens, ["▁こん", "ばん", "は", "▁こん", "ばん", "▁に", "ち", "▁は", "▁こんにちは"])
def test_sequence_builders(self):
tokenizer = self.tokenizer_class.from_pretrained("cl-tohoku/bert-base-japanese")
text = tokenizer.encode("ありがとう。", add_special_tokens=False)
text_2 = tokenizer.encode("どういたしまして。", add_special_tokens=False)
encoded_sentence = tokenizer.build_inputs_with_special_tokens(text)
encoded_pair = tokenizer.build_inputs_with_special_tokens(text, text_2)
# 2 is for "[CLS]", 3 is for "[SEP]"
assert encoded_sentence == [2] + text + [3]
assert encoded_pair == [2] + text + [3] + text_2 + [3]
@custom_tokenizers
class BertJapaneseCharacterTokenizationTest(TokenizerTesterMixin, unittest.TestCase):
tokenizer_class = BertJapaneseTokenizer
test_rust_tokenizer = False
def setUp(self):
super().setUp()
vocab_tokens = ["[UNK]", "[CLS]", "[SEP]", "こ", "ん", "に", "ち", "は", "ば", "世", "界", "、", "。"]
self.vocab_file = os.path.join(self.tmpdirname, VOCAB_FILES_NAMES["vocab_file"])
with open(self.vocab_file, "w", encoding="utf-8") as vocab_writer:
vocab_writer.write("".join([x + "\n" for x in vocab_tokens]))
def get_tokenizer(self, **kwargs):
return BertJapaneseTokenizer.from_pretrained(self.tmpdirname, subword_tokenizer_type="character", **kwargs)
def get_input_output_texts(self, tokenizer):
input_text = "こんにちは、世界。 \nこんばんは、世界。"
output_text = "こ ん に ち は 、 世 界 。 こ ん ば ん は 、 世 界 。"
return input_text, output_text
def test_pretokenized_inputs(self):
pass # TODO add if relevant
def test_maximum_encoding_length_pair_input(self):
pass # TODO add if relevant
def test_maximum_encoding_length_single_input(self):
pass # TODO add if relevant
def test_full_tokenizer(self):
tokenizer = self.tokenizer_class(self.vocab_file, subword_tokenizer_type="character")
tokens = tokenizer.tokenize("こんにちは、世界。 \nこんばんは、世界。")
self.assertListEqual(tokens, ["こ", "ん", "に", "ち", "は", "、", "世", "界", "。", "こ", "ん", "ば", "ん", "は", "、", "世", "界", "。"]) # fmt: skip
self.assertListEqual(
tokenizer.convert_tokens_to_ids(tokens), [3, 4, 5, 6, 7, 11, 9, 10, 12, 3, 4, 8, 4, 7, 11, 9, 10, 12]
)
def test_character_tokenizer(self):
vocab_tokens = ["[UNK]", "[CLS]", "[SEP]", "こ", "ん", "に", "ち", "は", "ば", "世", "界", "、", "。"]
vocab = {}
for i, token in enumerate(vocab_tokens):
vocab[token] = i
tokenizer = CharacterTokenizer(vocab=vocab, unk_token="[UNK]")
self.assertListEqual(tokenizer.tokenize(""), [])
self.assertListEqual(tokenizer.tokenize("こんにちは"), ["こ", "ん", "に", "ち", "は"])
self.assertListEqual(tokenizer.tokenize("こんにちほ"), ["こ", "ん", "に", "ち", "[UNK]"])
def test_sequence_builders(self):
tokenizer = self.tokenizer_class.from_pretrained("cl-tohoku/bert-base-japanese-char")
text = tokenizer.encode("ありがとう。", add_special_tokens=False)
text_2 = tokenizer.encode("どういたしまして。", add_special_tokens=False)
encoded_sentence = tokenizer.build_inputs_with_special_tokens(text)
encoded_pair = tokenizer.build_inputs_with_special_tokens(text, text_2)
# 2 is for "[CLS]", 3 is for "[SEP]"
assert encoded_sentence == [2] + text + [3]
assert encoded_pair == [2] + text + [3] + text_2 + [3]
@custom_tokenizers
class AutoTokenizerCustomTest(unittest.TestCase):
def test_tokenizer_bert_japanese(self):
EXAMPLE_BERT_JAPANESE_ID = "cl-tohoku/bert-base-japanese"
tokenizer = AutoTokenizer.from_pretrained(EXAMPLE_BERT_JAPANESE_ID)
self.assertIsInstance(tokenizer, BertJapaneseTokenizer)
class BertTokenizerMismatchTest(unittest.TestCase):
def test_tokenizer_mismatch_warning(self):
EXAMPLE_BERT_JAPANESE_ID = "cl-tohoku/bert-base-japanese"
with self.assertLogs("transformers", level="WARNING") as cm:
BertTokenizer.from_pretrained(EXAMPLE_BERT_JAPANESE_ID)
self.assertTrue(
cm.records[0].message.startswith(
"The tokenizer class you load from this checkpoint is not the same type as the class this function"
" is called from."
)
)
EXAMPLE_BERT_ID = "bert-base-cased"
with self.assertLogs("transformers", level="WARNING") as cm:
BertJapaneseTokenizer.from_pretrained(EXAMPLE_BERT_ID)
self.assertTrue(
cm.records[0].message.startswith(
"The tokenizer class you load from this checkpoint is not the same type as the class this function"
" is called from."
)
)
| transformers/tests/models/bert_japanese/test_tokenization_bert_japanese.py/0 | {
"file_path": "transformers/tests/models/bert_japanese/test_tokenization_bert_japanese.py",
"repo_id": "transformers",
"token_count": 9118
} | 347 |
# coding=utf-8
# Copyright 2023 The HuggingFace Inc. team. All rights reserved.
#
# Licensed under the Apache License, Version 2.0 (the "License");
# you may not use this file except in compliance with the License.
# You may obtain a copy of the License at
#
# http://www.apache.org/licenses/LICENSE-2.0
#
# Unless required by applicable law or agreed to in writing, software
# distributed under the License is distributed on an "AS IS" BASIS,
# WITHOUT WARRANTIES OR CONDITIONS OF ANY KIND, either express or implied.
# See the License for the specific language governing permissions and
# limitations under the License.
""" Testing suite for the PyTorch BLIP-2 model. """
import inspect
import tempfile
import unittest
import numpy as np
import requests
from transformers import CONFIG_MAPPING, Blip2Config, Blip2QFormerConfig, Blip2VisionConfig
from transformers.testing_utils import (
require_torch,
require_torch_multi_accelerator,
require_vision,
slow,
torch_device,
)
from transformers.utils import is_torch_available, is_vision_available
from ...test_configuration_common import ConfigTester
from ...test_modeling_common import (
ModelTesterMixin,
_config_zero_init,
floats_tensor,
ids_tensor,
random_attention_mask,
)
from ...test_pipeline_mixin import PipelineTesterMixin
if is_torch_available():
import torch
from torch import nn
from transformers import Blip2ForConditionalGeneration, Blip2Model, Blip2VisionModel
from transformers.models.blip_2.modeling_blip_2 import BLIP_2_PRETRAINED_MODEL_ARCHIVE_LIST
if is_vision_available():
from PIL import Image
from transformers import Blip2Processor
class Blip2VisionModelTester:
def __init__(
self,
parent,
batch_size=12,
image_size=30,
patch_size=2,
num_channels=3,
is_training=True,
hidden_size=32,
projection_dim=32,
num_hidden_layers=2,
num_attention_heads=4,
intermediate_size=37,
dropout=0.1,
attention_dropout=0.1,
initializer_range=1e-10,
scope=None,
):
self.parent = parent
self.batch_size = batch_size
self.image_size = image_size
self.patch_size = patch_size
self.num_channels = num_channels
self.is_training = is_training
self.hidden_size = hidden_size
self.projection_dim = projection_dim
self.num_hidden_layers = num_hidden_layers
self.num_attention_heads = num_attention_heads
self.intermediate_size = intermediate_size
self.dropout = dropout
self.attention_dropout = attention_dropout
self.initializer_range = initializer_range
self.scope = scope
# in ViT, the seq length equals the number of patches + 1 (we add 1 for the [CLS] token)
num_patches = (image_size // patch_size) ** 2
self.seq_length = num_patches + 1
def prepare_config_and_inputs(self):
pixel_values = floats_tensor([self.batch_size, self.num_channels, self.image_size, self.image_size])
config = self.get_config()
return config, pixel_values
def get_config(self):
return Blip2VisionConfig(
image_size=self.image_size,
patch_size=self.patch_size,
num_channels=self.num_channels,
hidden_size=self.hidden_size,
projection_dim=self.projection_dim,
num_hidden_layers=self.num_hidden_layers,
num_attention_heads=self.num_attention_heads,
intermediate_size=self.intermediate_size,
dropout=self.dropout,
attention_dropout=self.attention_dropout,
initializer_range=self.initializer_range,
)
def create_and_check_model(self, config, pixel_values):
model = Blip2VisionModel(config=config)
model.to(torch_device)
model.eval()
with torch.no_grad():
result = model(pixel_values)
# expected sequence length = num_patches + 1 (we add 1 for the [CLS] token)
image_size = (self.image_size, self.image_size)
patch_size = (self.patch_size, self.patch_size)
num_patches = (image_size[1] // patch_size[1]) * (image_size[0] // patch_size[0])
self.parent.assertEqual(result.last_hidden_state.shape, (self.batch_size, num_patches + 1, self.hidden_size))
self.parent.assertEqual(result.pooler_output.shape, (self.batch_size, self.hidden_size))
def prepare_config_and_inputs_for_common(self):
config_and_inputs = self.prepare_config_and_inputs()
config, pixel_values = config_and_inputs
inputs_dict = {"pixel_values": pixel_values}
return config, inputs_dict
@require_torch
class Blip2VisionModelTest(ModelTesterMixin, unittest.TestCase):
"""
Here we also overwrite some of the tests of test_modeling_common.py, as BLIP-2's vision encoder does not use input_ids, inputs_embeds,
attention_mask and seq_length.
"""
all_model_classes = (Blip2VisionModel,) if is_torch_available() else ()
fx_compatible = False
test_pruning = False
test_resize_embeddings = False
test_head_masking = False
def setUp(self):
self.model_tester = Blip2VisionModelTester(self)
self.config_tester = ConfigTester(
self, config_class=Blip2VisionConfig, has_text_modality=False, hidden_size=37
)
def test_config(self):
self.config_tester.run_common_tests()
@unittest.skip(reason="BLIP-2's vision encoder does not use inputs_embeds")
def test_inputs_embeds(self):
pass
def test_model_common_attributes(self):
config, _ = self.model_tester.prepare_config_and_inputs_for_common()
for model_class in self.all_model_classes:
model = model_class(config)
self.assertIsInstance(model.get_input_embeddings(), (nn.Module))
x = model.get_output_embeddings()
self.assertTrue(x is None or isinstance(x, nn.Linear))
def test_forward_signature(self):
config, _ = self.model_tester.prepare_config_and_inputs_for_common()
for model_class in self.all_model_classes:
model = model_class(config)
signature = inspect.signature(model.forward)
# signature.parameters is an OrderedDict => so arg_names order is deterministic
arg_names = [*signature.parameters.keys()]
expected_arg_names = ["pixel_values"]
self.assertListEqual(arg_names[:1], expected_arg_names)
def test_model(self):
config_and_inputs = self.model_tester.prepare_config_and_inputs()
self.model_tester.create_and_check_model(*config_and_inputs)
def test_training(self):
pass
def test_training_gradient_checkpointing(self):
pass
@unittest.skip(
reason="This architecure seem to not compute gradients properly when using GC, check: https://github.com/huggingface/transformers/pull/27124"
)
def test_training_gradient_checkpointing_use_reentrant(self):
pass
@unittest.skip(
reason="This architecure seem to not compute gradients properly when using GC, check: https://github.com/huggingface/transformers/pull/27124"
)
def test_training_gradient_checkpointing_use_reentrant_false(self):
pass
@unittest.skip(reason="Blip2VisionModel has no base class and is not available in MODEL_MAPPING")
def test_save_load_fast_init_from_base(self):
pass
@unittest.skip(reason="Blip2VisionModel has no base class and is not available in MODEL_MAPPING")
def test_save_load_fast_init_to_base(self):
pass
@slow
def test_model_from_pretrained(self):
for model_name in BLIP_2_PRETRAINED_MODEL_ARCHIVE_LIST[:1]:
model = Blip2VisionModel.from_pretrained(model_name)
self.assertIsNotNone(model)
class Blip2QFormerModelTester:
def __init__(
self,
parent,
batch_size=12,
seq_length=7,
is_training=True,
use_input_mask=True,
use_labels=True,
vocab_size=99,
hidden_size=32,
projection_dim=32,
num_hidden_layers=2,
num_attention_heads=4,
intermediate_size=37,
dropout=0.1,
attention_dropout=0.1,
max_position_embeddings=512,
initializer_range=0.02,
bos_token_id=0,
scope=None,
):
self.parent = parent
self.batch_size = batch_size
self.seq_length = seq_length
self.is_training = is_training
self.use_input_mask = use_input_mask
self.use_labels = use_labels
self.vocab_size = vocab_size
self.hidden_size = hidden_size
self.projection_dim = projection_dim
self.num_hidden_layers = num_hidden_layers
self.num_attention_heads = num_attention_heads
self.intermediate_size = intermediate_size
self.dropout = dropout
self.attention_dropout = attention_dropout
self.max_position_embeddings = max_position_embeddings
self.initializer_range = initializer_range
self.scope = scope
self.bos_token_id = bos_token_id
def prepare_config_and_inputs(self):
input_ids = ids_tensor([self.batch_size, self.seq_length], self.vocab_size)
input_mask = None
if self.use_input_mask:
input_mask = random_attention_mask([self.batch_size, self.seq_length])
if input_mask is not None:
batch_size, seq_length = input_mask.shape
rnd_start_indices = np.random.randint(1, seq_length - 1, size=(batch_size,))
for batch_idx, start_index in enumerate(rnd_start_indices):
input_mask[batch_idx, :start_index] = 1
input_mask[batch_idx, start_index:] = 0
config = self.get_config()
return config, input_ids, input_mask
def get_config(self):
return Blip2QFormerConfig(
vocab_size=self.vocab_size,
hidden_size=self.hidden_size,
projection_dim=self.projection_dim,
num_hidden_layers=self.num_hidden_layers,
num_attention_heads=self.num_attention_heads,
intermediate_size=self.intermediate_size,
dropout=self.dropout,
attention_dropout=self.attention_dropout,
max_position_embeddings=self.max_position_embeddings,
initializer_range=self.initializer_range,
bos_token_id=self.bos_token_id,
)
# this class is based on `OPTModelTester` found in tests/models/opt/test_modeling_opt.py
class Blip2TextModelDecoderOnlyTester:
def __init__(
self,
parent,
batch_size=12,
seq_length=7,
is_training=True,
use_labels=False,
vocab_size=99,
hidden_size=16,
num_hidden_layers=2,
num_attention_heads=4,
intermediate_size=4,
hidden_act="gelu",
hidden_dropout_prob=0.1,
attention_probs_dropout_prob=0.1,
max_position_embeddings=20,
eos_token_id=2,
pad_token_id=1,
bos_token_id=0,
embed_dim=16,
num_labels=3,
word_embed_proj_dim=16,
type_sequence_label_size=2,
):
self.parent = parent
self.batch_size = batch_size
self.seq_length = seq_length
self.is_training = is_training
self.use_labels = use_labels
self.vocab_size = vocab_size
self.hidden_size = hidden_size
self.num_hidden_layers = num_hidden_layers
self.num_attention_heads = num_attention_heads
self.intermediate_size = intermediate_size
self.hidden_act = hidden_act
self.hidden_dropout_prob = hidden_dropout_prob
self.attention_probs_dropout_prob = attention_probs_dropout_prob
self.max_position_embeddings = max_position_embeddings
self.eos_token_id = eos_token_id
self.pad_token_id = pad_token_id
self.bos_token_id = bos_token_id
self.embed_dim = embed_dim
self.num_labels = num_labels
self.type_sequence_label_size = type_sequence_label_size
self.word_embed_proj_dim = word_embed_proj_dim
self.is_encoder_decoder = False
def prepare_config_and_inputs(self):
config = self.get_config()
input_ids = ids_tensor([self.batch_size, self.seq_length], self.vocab_size).clamp(3)
input_ids[:, -1] = self.eos_token_id # Eos Token
attention_mask = input_ids.ne(self.pad_token_id)
return config, input_ids, attention_mask
def get_config(self):
return CONFIG_MAPPING["opt"](
vocab_size=self.vocab_size,
hidden_size=self.hidden_size,
num_hidden_layers=self.num_hidden_layers,
num_attention_heads=self.num_attention_heads,
ffn_dim=self.intermediate_size,
dropout=self.hidden_dropout_prob,
attention_dropout=self.attention_probs_dropout_prob,
max_position_embeddings=self.max_position_embeddings,
eos_token_id=self.eos_token_id,
bos_token_id=self.bos_token_id,
pad_token_id=self.pad_token_id,
embed_dim=self.embed_dim,
is_encoder_decoder=False,
word_embed_proj_dim=self.word_embed_proj_dim,
)
# this model tester uses a decoder-only language model (OPT)
class Blip2ForConditionalGenerationDecoderOnlyModelTester:
def __init__(
self, parent, vision_kwargs=None, qformer_kwargs=None, text_kwargs=None, is_training=True, num_query_tokens=10
):
if vision_kwargs is None:
vision_kwargs = {}
if qformer_kwargs is None:
qformer_kwargs = {}
if text_kwargs is None:
text_kwargs = {}
self.parent = parent
self.vision_model_tester = Blip2VisionModelTester(parent, **vision_kwargs)
self.qformer_model_tester = Blip2QFormerModelTester(parent, **qformer_kwargs)
self.text_model_tester = Blip2TextModelDecoderOnlyTester(parent, **text_kwargs)
self.is_training = is_training
self.num_query_tokens = num_query_tokens
def prepare_config_and_inputs(self):
_, pixel_values = self.vision_model_tester.prepare_config_and_inputs()
_, input_ids, attention_mask = self.text_model_tester.prepare_config_and_inputs()
config = self.get_config()
return config, input_ids, attention_mask, pixel_values
def get_config(self):
return Blip2Config.from_vision_qformer_text_configs(
vision_config=self.vision_model_tester.get_config(),
qformer_config=self.qformer_model_tester.get_config(),
text_config=self.text_model_tester.get_config(),
num_query_tokens=self.num_query_tokens,
)
def create_and_check_for_conditional_generation(self, config, input_ids, attention_mask, pixel_values):
model = Blip2ForConditionalGeneration(config).to(torch_device).eval()
with torch.no_grad():
result = model(pixel_values, input_ids, attention_mask)
expected_seq_length = self.num_query_tokens + self.text_model_tester.seq_length
self.parent.assertEqual(
result.logits.shape,
(self.vision_model_tester.batch_size, expected_seq_length, self.text_model_tester.vocab_size),
)
def prepare_config_and_inputs_for_common(self):
config_and_inputs = self.prepare_config_and_inputs()
config, input_ids, attention_mask, pixel_values = config_and_inputs
inputs_dict = {
"pixel_values": pixel_values,
"input_ids": input_ids,
"attention_mask": attention_mask,
"labels": input_ids,
}
return config, inputs_dict
@require_torch
class Blip2ForConditionalGenerationDecoderOnlyTest(ModelTesterMixin, unittest.TestCase):
all_model_classes = (Blip2ForConditionalGeneration,) if is_torch_available() else ()
fx_compatible = False
test_head_masking = False
test_pruning = False
test_resize_embeddings = False
test_attention_outputs = False
test_torchscript = False
def setUp(self):
self.model_tester = Blip2ForConditionalGenerationDecoderOnlyModelTester(self)
def test_for_conditional_generation(self):
config_and_inputs = self.model_tester.prepare_config_and_inputs()
self.model_tester.create_and_check_for_conditional_generation(*config_and_inputs)
@unittest.skip(reason="Hidden_states is tested in individual model tests")
def test_hidden_states_output(self):
pass
@unittest.skip(reason="Inputs_embeds is tested in individual model tests")
def test_inputs_embeds(self):
pass
@unittest.skip(reason="Retain_grad is tested in individual model tests")
def test_retain_grad_hidden_states_attentions(self):
pass
@unittest.skip(reason="Blip2Model does not have input/output embeddings")
def test_model_common_attributes(self):
pass
@unittest.skip(reason="There's no base Blip2Model")
def test_save_load_fast_init_from_base(self):
pass
@unittest.skip(reason="There's no base Blip2Model")
def test_save_load_fast_init_to_base(self):
pass
def test_forward_signature(self):
config, _ = self.model_tester.prepare_config_and_inputs_for_common()
for model_class in self.all_model_classes:
model = model_class(config)
signature = inspect.signature(model.forward)
# signature.parameters is an OrderedDict => so arg_names order is deterministic
arg_names = [*signature.parameters.keys()]
expected_arg_names = ["pixel_values"]
self.assertListEqual(arg_names[:1], expected_arg_names)
def test_load_vision_qformer_text_config(self):
config, inputs_dict = self.model_tester.prepare_config_and_inputs_for_common()
# Save Blip2Config and check if we can load Blip2VisionConfig from it
with tempfile.TemporaryDirectory() as tmp_dir_name:
config.save_pretrained(tmp_dir_name)
vision_config = Blip2VisionConfig.from_pretrained(tmp_dir_name)
self.assertDictEqual(config.vision_config.to_dict(), vision_config.to_dict())
# Save Blip2Config and check if we can load Blip2QFormerConfig from it
with tempfile.TemporaryDirectory() as tmp_dir_name:
config.save_pretrained(tmp_dir_name)
qformer_config = Blip2QFormerConfig.from_pretrained(tmp_dir_name)
self.assertDictEqual(config.qformer_config.to_dict(), qformer_config.to_dict())
@slow
def test_model_from_pretrained(self):
for model_name in BLIP_2_PRETRAINED_MODEL_ARCHIVE_LIST:
model = Blip2ForConditionalGeneration.from_pretrained(model_name)
self.assertIsNotNone(model)
# this class is based on `T5ModelTester` found in tests/models/t5/test_modeling_t5.py
class Blip2TextModelTester:
def __init__(
self,
parent,
vocab_size=99,
batch_size=12,
encoder_seq_length=7,
decoder_seq_length=9,
# For common tests
is_training=True,
use_attention_mask=True,
use_labels=True,
hidden_size=32,
num_hidden_layers=2,
num_attention_heads=4,
d_ff=37,
relative_attention_num_buckets=8,
dropout_rate=0.1,
initializer_factor=0.002,
eos_token_id=1,
pad_token_id=0,
decoder_start_token_id=0,
scope=None,
decoder_layers=None,
):
self.parent = parent
self.batch_size = batch_size
self.encoder_seq_length = encoder_seq_length
self.decoder_seq_length = decoder_seq_length
# For common tests
self.seq_length = self.decoder_seq_length
self.is_training = is_training
self.use_attention_mask = use_attention_mask
self.use_labels = use_labels
self.vocab_size = vocab_size
self.hidden_size = hidden_size
self.num_hidden_layers = num_hidden_layers
self.num_attention_heads = num_attention_heads
self.d_ff = d_ff
self.relative_attention_num_buckets = relative_attention_num_buckets
self.dropout_rate = dropout_rate
self.initializer_factor = initializer_factor
self.eos_token_id = eos_token_id
self.pad_token_id = pad_token_id
self.decoder_start_token_id = decoder_start_token_id
self.scope = None
self.decoder_layers = decoder_layers
def prepare_config_and_inputs(self):
input_ids = ids_tensor([self.batch_size, self.encoder_seq_length], self.vocab_size)
decoder_input_ids = ids_tensor([self.batch_size, self.decoder_seq_length], self.vocab_size)
attention_mask = None
decoder_attention_mask = None
if self.use_attention_mask:
attention_mask = ids_tensor([self.batch_size, self.encoder_seq_length], vocab_size=2)
decoder_attention_mask = ids_tensor([self.batch_size, self.decoder_seq_length], vocab_size=2)
lm_labels = None
if self.use_labels:
lm_labels = ids_tensor([self.batch_size, self.decoder_seq_length], self.vocab_size)
config = self.get_config()
return (
config,
input_ids,
decoder_input_ids,
attention_mask,
decoder_attention_mask,
lm_labels,
)
def get_config(self):
return CONFIG_MAPPING["t5"](
vocab_size=self.vocab_size,
d_model=self.hidden_size,
d_ff=self.d_ff,
d_kv=self.hidden_size // self.num_attention_heads,
num_layers=self.num_hidden_layers,
num_decoder_layers=self.decoder_layers,
num_heads=self.num_attention_heads,
relative_attention_num_buckets=self.relative_attention_num_buckets,
dropout_rate=self.dropout_rate,
initializer_factor=self.initializer_factor,
eos_token_id=self.eos_token_id,
bos_token_id=self.pad_token_id,
pad_token_id=self.pad_token_id,
decoder_start_token_id=self.decoder_start_token_id,
)
# this model tester uses an encoder-decoder language model (T5)
class Blip2ModelTester:
def __init__(
self, parent, vision_kwargs=None, qformer_kwargs=None, text_kwargs=None, is_training=True, num_query_tokens=10
):
if vision_kwargs is None:
vision_kwargs = {}
if qformer_kwargs is None:
qformer_kwargs = {}
if text_kwargs is None:
text_kwargs = {}
self.parent = parent
self.vision_model_tester = Blip2VisionModelTester(parent, **vision_kwargs)
self.qformer_model_tester = Blip2QFormerModelTester(parent, **qformer_kwargs)
self.text_model_tester = Blip2TextModelTester(parent, **text_kwargs)
self.is_training = is_training
self.num_query_tokens = num_query_tokens
def prepare_config_and_inputs(self):
_, pixel_values = self.vision_model_tester.prepare_config_and_inputs()
(
_,
input_ids,
decoder_input_ids,
attention_mask,
decoder_attention_mask,
lm_labels,
) = self.text_model_tester.prepare_config_and_inputs()
config = self.get_config()
return config, input_ids, attention_mask, pixel_values, decoder_input_ids, decoder_attention_mask, lm_labels
def get_config(self):
return Blip2Config.from_vision_qformer_text_configs(
vision_config=self.vision_model_tester.get_config(),
qformer_config=self.qformer_model_tester.get_config(),
text_config=self.text_model_tester.get_config(),
num_query_tokens=self.num_query_tokens,
)
def create_and_check_for_conditional_generation(
self, config, input_ids, attention_mask, pixel_values, decoder_input_ids, decoder_attention_mask, labels
):
model = Blip2ForConditionalGeneration(config).to(torch_device).eval()
with torch.no_grad():
result = model(pixel_values, input_ids, attention_mask, decoder_input_ids, decoder_attention_mask)
self.parent.assertEqual(
result.logits.shape,
(
self.vision_model_tester.batch_size,
self.text_model_tester.seq_length,
self.text_model_tester.vocab_size,
),
)
def prepare_config_and_inputs_for_common(self):
config_and_inputs = self.prepare_config_and_inputs()
(
config,
input_ids,
attention_mask,
pixel_values,
decoder_input_ids,
decoder_attention_mask,
labels,
) = config_and_inputs
inputs_dict = {
"pixel_values": pixel_values,
"input_ids": input_ids,
"attention_mask": attention_mask,
"decoder_input_ids": decoder_input_ids,
"decoder_attention_mask": decoder_attention_mask,
"labels": labels,
}
return config, inputs_dict
@require_torch
class Blip2ModelTest(ModelTesterMixin, PipelineTesterMixin, unittest.TestCase):
all_model_classes = (Blip2ForConditionalGeneration, Blip2Model) if is_torch_available() else ()
pipeline_model_mapping = (
{
"feature-extraction": Blip2Model,
"image-to-text": Blip2ForConditionalGeneration,
"visual-question-answering": Blip2ForConditionalGeneration,
}
if is_torch_available()
else {}
)
fx_compatible = False
test_head_masking = False
test_pruning = False
test_resize_embeddings = False
test_attention_outputs = False
test_torchscript = False
def setUp(self):
self.model_tester = Blip2ModelTester(self)
def test_for_conditional_generation(self):
config_and_inputs = self.model_tester.prepare_config_and_inputs()
self.model_tester.create_and_check_for_conditional_generation(*config_and_inputs)
@unittest.skip(reason="Hidden_states is tested in individual model tests")
def test_hidden_states_output(self):
pass
@unittest.skip(reason="Inputs_embeds is tested in individual model tests")
def test_inputs_embeds(self):
pass
@unittest.skip(reason="Retain_grad is tested in individual model tests")
def test_retain_grad_hidden_states_attentions(self):
pass
@unittest.skip(reason="Blip2Model does not have input/output embeddings")
def test_model_common_attributes(self):
pass
@unittest.skip(reason="There's no base Blip2Model")
def test_save_load_fast_init_from_base(self):
pass
@unittest.skip(reason="There's no base Blip2Model")
def test_save_load_fast_init_to_base(self):
pass
@unittest.skip(reason="Does not work on the tiny model as we keep hitting edge cases.")
def test_cpu_offload(self):
pass
def test_forward_signature(self):
config, _ = self.model_tester.prepare_config_and_inputs_for_common()
for model_class in self.all_model_classes:
model = model_class(config)
signature = inspect.signature(model.forward)
# signature.parameters is an OrderedDict => so arg_names order is deterministic
arg_names = [*signature.parameters.keys()]
expected_arg_names = ["pixel_values"]
self.assertListEqual(arg_names[:1], expected_arg_names)
def test_load_vision_qformer_text_config(self):
config, inputs_dict = self.model_tester.prepare_config_and_inputs_for_common()
# Save Blip2Config and check if we can load Blip2VisionConfig from it
with tempfile.TemporaryDirectory() as tmp_dir_name:
config.save_pretrained(tmp_dir_name)
vision_config = Blip2VisionConfig.from_pretrained(tmp_dir_name)
self.assertDictEqual(config.vision_config.to_dict(), vision_config.to_dict())
# Save Blip2Config and check if we can load Blip2QFormerConfig from it
with tempfile.TemporaryDirectory() as tmp_dir_name:
config.save_pretrained(tmp_dir_name)
qformer_config = Blip2QFormerConfig.from_pretrained(tmp_dir_name)
self.assertDictEqual(config.qformer_config.to_dict(), qformer_config.to_dict())
@slow
def test_model_from_pretrained(self):
for model_name in BLIP_2_PRETRAINED_MODEL_ARCHIVE_LIST:
model = Blip2ForConditionalGeneration.from_pretrained(model_name)
self.assertIsNotNone(model)
def test_get_text_features(self):
config, _ = self.model_tester.prepare_config_and_inputs_for_common()
inputs_dict = {
"input_ids": torch.LongTensor([[1, 2, 3, 4, 5, 6, 7, 8, 9, 10]]).to(torch_device),
"attention_mask": torch.LongTensor([[1, 1, 1, 1, 1, 1, 1, 1, 1, 1]]).to(torch_device),
"decoder_input_ids": torch.LongTensor([[1, 2, 3, 4, 5, 6, 7, 8, 9, 10]]).to(torch_device),
}
model = Blip2Model(config).to(torch_device)
model.eval()
text_features = model.get_text_features(**inputs_dict)
self.assertEqual(text_features[0].shape, (1, 10, config.text_config.vocab_size))
def test_get_image_features(self):
config, inputs_dict = self.model_tester.prepare_config_and_inputs_for_common()
keys_to_pop = ["input_ids", "attention_mask", "decoder_input_ids", "decoder_attention_mask", "labels"]
for key in keys_to_pop:
inputs_dict.pop(key)
model = Blip2Model(config).to(torch_device)
model.eval()
image_features = model.get_image_features(**inputs_dict)
self.assertEqual(
image_features[0].shape,
(
self.model_tester.vision_model_tester.batch_size,
self.model_tester.vision_model_tester.seq_length,
config.vision_config.hidden_size,
),
)
def test_get_qformer_features(self):
config, inputs_dict = self.model_tester.prepare_config_and_inputs_for_common()
keys_to_pop = ["input_ids", "attention_mask", "decoder_input_ids", "decoder_attention_mask", "labels"]
for key in keys_to_pop:
inputs_dict.pop(key)
model = Blip2Model(config).to(torch_device)
model.eval()
qformer_features = model.get_qformer_features(**inputs_dict)
self.assertEqual(
qformer_features[0].shape,
(self.model_tester.vision_model_tester.batch_size, 10, config.vision_config.hidden_size),
)
# override from common to deal with nested configurations (`vision_config`, `text_config` and `qformer_config`)
def test_initialization(self):
config, inputs_dict = self.model_tester.prepare_config_and_inputs_for_common()
configs_no_init = _config_zero_init(config)
for key in ["vision_config", "qformer_config", "text_config"]:
setattr(configs_no_init, key, _config_zero_init(getattr(configs_no_init, key)))
for model_class in self.all_model_classes:
model = model_class(config=configs_no_init)
for name, param in model.named_parameters():
if param.requires_grad:
self.assertIn(
((param.data.mean() * 1e9).round() / 1e9).item(),
[0.0, 1.0],
msg=f"Parameter {name} of model {model_class} seems not properly initialized",
)
# We will verify our results on an image of cute cats
def prepare_img():
url = "https://huggingface.co/hf-internal-testing/blip-test-image/resolve/main/demo.jpg"
image = Image.open(requests.get(url, stream=True).raw)
return image
@require_vision
@require_torch
@slow
class Blip2ModelIntegrationTest(unittest.TestCase):
def test_inference_opt(self):
processor = Blip2Processor.from_pretrained("Salesforce/blip2-opt-2.7b")
model = Blip2ForConditionalGeneration.from_pretrained(
"Salesforce/blip2-opt-2.7b", torch_dtype=torch.float16
).to(torch_device)
# prepare image
image = prepare_img()
inputs = processor(images=image, return_tensors="pt").to(torch_device, dtype=torch.float16)
predictions = model.generate(**inputs)
generated_text = processor.batch_decode(predictions, skip_special_tokens=True)[0].strip()
# Test output
self.assertEqual(predictions[0].tolist(), [2, 102, 693, 2828, 15, 5, 4105, 19, 10, 2335, 50118])
self.assertEqual("a woman sitting on the beach with a dog", generated_text)
# image and context
prompt = "Question: which city is this? Answer:"
inputs = processor(images=image, text=prompt, return_tensors="pt").to(torch_device, dtype=torch.float16)
predictions = model.generate(**inputs)
generated_text = processor.batch_decode(predictions, skip_special_tokens=True)[0].strip()
# Test output
self.assertEqual(
predictions[0].tolist(),
[2, 24, 18, 45, 10, 343, 6, 24, 18, 10, 4105, 50118],
)
self.assertEqual(generated_text, "it's not a city, it's a beach")
def test_inference_opt_batched_beam_search(self):
processor = Blip2Processor.from_pretrained("Salesforce/blip2-opt-2.7b")
model = Blip2ForConditionalGeneration.from_pretrained(
"Salesforce/blip2-opt-2.7b", torch_dtype=torch.float16
).to(torch_device)
# prepare image
image = prepare_img()
inputs = processor(images=[image, image], return_tensors="pt").to(torch_device, dtype=torch.float16)
predictions = model.generate(**inputs, num_beams=2)
# Test output (in this case, slightly different from greedy search)
self.assertEqual(predictions[0].tolist(), [2, 102, 693, 2828, 15, 5, 4105, 19, 69, 2335, 50118])
self.assertEqual(predictions[1].tolist(), [2, 102, 693, 2828, 15, 5, 4105, 19, 69, 2335, 50118])
def test_inference_t5(self):
processor = Blip2Processor.from_pretrained("Salesforce/blip2-flan-t5-xl")
model = Blip2ForConditionalGeneration.from_pretrained(
"Salesforce/blip2-flan-t5-xl", torch_dtype=torch.float16
).to(torch_device)
# prepare image
image = prepare_img()
inputs = processor(images=image, return_tensors="pt").to(torch_device, dtype=torch.float16)
predictions = model.generate(**inputs)
generated_text = processor.batch_decode(predictions, skip_special_tokens=True)[0].strip()
# Test output
self.assertEqual(predictions[0].tolist(), [0, 2335, 1556, 28, 1782, 30, 8, 2608, 1])
self.assertEqual("woman playing with dog on the beach", generated_text)
# image and context
prompt = "Question: which city is this? Answer:"
inputs = processor(images=image, text=prompt, return_tensors="pt").to(torch_device, dtype=torch.float16)
predictions = model.generate(**inputs)
generated_text = processor.batch_decode(predictions, skip_special_tokens=True)[0].strip()
# Test output
self.assertEqual(
predictions[0].tolist(),
[0, 3, 7, 152, 67, 839, 1],
)
self.assertEqual(generated_text, "san diego")
def test_inference_t5_batched_beam_search(self):
processor = Blip2Processor.from_pretrained("Salesforce/blip2-flan-t5-xl")
model = Blip2ForConditionalGeneration.from_pretrained(
"Salesforce/blip2-flan-t5-xl", torch_dtype=torch.float16
).to(torch_device)
# prepare image
image = prepare_img()
inputs = processor(images=[image, image], return_tensors="pt").to(torch_device, dtype=torch.float16)
predictions = model.generate(**inputs, num_beams=2)
# Test output (in this case, slightly different from greedy search)
self.assertEqual(predictions[0].tolist(), [0, 2335, 1556, 28, 1782, 30, 8, 2608, 1])
self.assertEqual(predictions[1].tolist(), [0, 2335, 1556, 28, 1782, 30, 8, 2608, 1])
@require_torch_multi_accelerator
def test_inference_opt_multi_accelerator(self):
processor = Blip2Processor.from_pretrained("Salesforce/blip2-opt-2.7b")
model = Blip2ForConditionalGeneration.from_pretrained(
"Salesforce/blip2-opt-2.7b", torch_dtype=torch.float16, device_map="balanced"
)
# prepare image
image = prepare_img()
inputs = processor(images=image, return_tensors="pt").to(0, dtype=torch.float16)
predictions = model.generate(**inputs)
generated_text = processor.batch_decode(predictions, skip_special_tokens=True)[0].strip()
# Test output
self.assertEqual(predictions[0].tolist(), [2, 102, 693, 2828, 15, 5, 4105, 19, 10, 2335, 50118])
self.assertEqual("a woman sitting on the beach with a dog", generated_text)
# image and context
prompt = "Question: which city is this? Answer:"
inputs = processor(images=image, text=prompt, return_tensors="pt").to(0, dtype=torch.float16)
predictions = model.generate(**inputs)
generated_text = processor.batch_decode(predictions, skip_special_tokens=True)[0].strip()
# Test output
self.assertEqual(
predictions[0].tolist(),
[2, 24, 18, 45, 10, 343, 6, 24, 18, 10, 4105, 50118],
)
self.assertEqual(generated_text, "it's not a city, it's a beach")
@require_torch_multi_accelerator
def test_inference_t5_multi_accelerator(self):
processor = Blip2Processor.from_pretrained("Salesforce/blip2-flan-t5-xl")
device_map = device_map = {
"query_tokens": 0,
"vision_model": 0,
"language_model": 1,
"language_projection": 0,
"qformer": 0,
}
model = Blip2ForConditionalGeneration.from_pretrained(
"Salesforce/blip2-flan-t5-xl", torch_dtype=torch.float16, device_map=device_map
)
# prepare image
image = prepare_img()
inputs = processor(images=image, return_tensors="pt").to(0, dtype=torch.float16)
predictions = model.generate(**inputs)
generated_text = processor.batch_decode(predictions, skip_special_tokens=True)[0].strip()
# Test output
self.assertEqual(predictions[0].tolist(), [0, 2335, 1556, 28, 1782, 30, 8, 2608, 1])
self.assertEqual("woman playing with dog on the beach", generated_text)
# image and context
prompt = "Question: which city is this? Answer:"
inputs = processor(images=image, text=prompt, return_tensors="pt").to(0, dtype=torch.float16)
predictions = model.generate(**inputs)
generated_text = processor.batch_decode(predictions, skip_special_tokens=True)[0].strip()
# Test output
self.assertEqual(
predictions[0].tolist(),
[0, 3, 7, 152, 67, 839, 1],
)
self.assertEqual(generated_text, "san diego")
| transformers/tests/models/blip_2/test_modeling_blip_2.py/0 | {
"file_path": "transformers/tests/models/blip_2/test_modeling_blip_2.py",
"repo_id": "transformers",
"token_count": 17529
} | 348 |
# coding=utf-8
# Copyright 2018 HuggingFace Inc. team.
#
# Licensed under the Apache License, Version 2.0 (the "License");
# you may not use this file except in compliance with the License.
# You may obtain a copy of the License at
#
# http://www.apache.org/licenses/LICENSE-2.0
#
# Unless required by applicable law or agreed to in writing, software
# distributed under the License is distributed on an "AS IS" BASIS,
# WITHOUT WARRANTIES OR CONDITIONS OF ANY KIND, either express or implied.
# See the License for the specific language governing permissions and
# limitations under the License.
import tempfile
import unittest
from transformers import AddedToken, CamembertTokenizer, CamembertTokenizerFast
from transformers.testing_utils import get_tests_dir, require_sentencepiece, require_tokenizers, slow
from transformers.utils import is_torch_available
from ...test_tokenization_common import TokenizerTesterMixin
SAMPLE_VOCAB = get_tests_dir("fixtures/test_sentencepiece.model")
SAMPLE_BPE_VOCAB = get_tests_dir("fixtures/test_sentencepiece_bpe.model")
FRAMEWORK = "pt" if is_torch_available() else "tf"
@require_sentencepiece
@require_tokenizers
class CamembertTokenizationTest(TokenizerTesterMixin, unittest.TestCase):
tokenizer_class = CamembertTokenizer
rust_tokenizer_class = CamembertTokenizerFast
test_rust_tokenizer = True
test_sentencepiece = True
def setUp(self):
super().setUp()
# We have a SentencePiece fixture for testing
tokenizer = CamembertTokenizer(SAMPLE_VOCAB)
tokenizer.save_pretrained(self.tmpdirname)
@unittest.skip(
"Token maps are not equal because someone set the probability of ('<unk>NOTUSED', -100), so it's never encoded for fast"
)
def test_special_tokens_map_equal(self):
return
def test_convert_token_and_id(self):
"""Test ``_convert_token_to_id`` and ``_convert_id_to_token``."""
token = "<pad>"
token_id = 1 # 1 is the offset id, but in the spm vocab it's 3
self.assertEqual(self.get_tokenizer().convert_tokens_to_ids(token), token_id)
self.assertEqual(self.get_tokenizer().convert_ids_to_tokens(token_id), token)
def test_get_vocab(self):
vocab_keys = list(self.get_tokenizer().get_vocab().keys())
self.assertEqual(vocab_keys[0], "<s>NOTUSED")
self.assertEqual(vocab_keys[1], "<pad>")
self.assertEqual(vocab_keys[-1], "<mask>")
self.assertEqual(len(vocab_keys), 1_005)
def test_vocab_size(self):
self.assertEqual(self.get_tokenizer().vocab_size, 1_000)
def test_rust_and_python_bpe_tokenizers(self):
tokenizer = CamembertTokenizer(SAMPLE_BPE_VOCAB)
tokenizer.save_pretrained(self.tmpdirname)
rust_tokenizer = CamembertTokenizerFast.from_pretrained(self.tmpdirname)
sequence = "I was born in 92000, and this is falsé."
ids = tokenizer.encode(sequence)
rust_ids = rust_tokenizer.encode(sequence)
self.assertListEqual(ids, rust_ids)
ids = tokenizer.encode(sequence, add_special_tokens=False)
rust_ids = rust_tokenizer.encode(sequence, add_special_tokens=False)
self.assertListEqual(ids, rust_ids)
# <unk> tokens are not the same for `rust` than for `slow`.
# Because spm gives back raw token instead of `unk` in EncodeAsPieces
# tokens = tokenizer.tokenize(sequence)
tokens = tokenizer.convert_ids_to_tokens(ids)
rust_tokens = rust_tokenizer.tokenize(sequence)
self.assertListEqual(tokens, rust_tokens)
def test_rust_and_python_full_tokenizers(self):
if not self.test_rust_tokenizer:
return
tokenizer = self.get_tokenizer()
rust_tokenizer = self.get_rust_tokenizer()
sequence = "I was born in 92000, and this is falsé."
tokens = tokenizer.tokenize(sequence)
rust_tokens = rust_tokenizer.tokenize(sequence)
self.assertListEqual(tokens, rust_tokens)
ids = tokenizer.encode(sequence, add_special_tokens=False)
rust_ids = rust_tokenizer.encode(sequence, add_special_tokens=False)
self.assertListEqual(ids, rust_ids)
rust_tokenizer = self.get_rust_tokenizer()
ids = tokenizer.encode(sequence)
rust_ids = rust_tokenizer.encode(sequence)
self.assertListEqual(ids, rust_ids)
@slow
def test_tokenizer_integration(self):
expected_encoding = {'input_ids': [[5, 54, 7196, 297, 30, 23, 776, 18, 11, 3215, 3705, 8252, 22, 3164, 1181, 2116, 29, 16, 813, 25, 791, 3314, 20, 3446, 38, 27575, 120, 6, 1, 1, 1, 1, 1, 1, 1, 1, 1, 1, 1, 1, 1, 1, 1, 1, 1, 1, 1, 1, 1, 1], [5, 468, 17, 11, 9088, 20, 1517, 8, 22804, 18818, 10, 38, 629, 607, 607, 142, 19, 7196, 867, 56, 10326, 24, 2267, 20, 416, 5072, 15612, 233, 734, 7, 2399, 27, 16, 3015, 1649, 7, 24, 20, 4338, 2399, 27, 13, 3400, 14, 13, 6189, 8, 930, 9, 6]], 'attention_mask': [[1, 1, 1, 1, 1, 1, 1, 1, 1, 1, 1, 1, 1, 1, 1, 1, 1, 1, 1, 1, 1, 1, 1, 1, 1, 1, 1, 1, 0, 0, 0, 0, 0, 0, 0, 0, 0, 0, 0, 0, 0, 0, 0, 0, 0, 0, 0, 0, 0, 0], [1, 1, 1, 1, 1, 1, 1, 1, 1, 1, 1, 1, 1, 1, 1, 1, 1, 1, 1, 1, 1, 1, 1, 1, 1, 1, 1, 1, 1, 1, 1, 1, 1, 1, 1, 1, 1, 1, 1, 1, 1, 1, 1, 1, 1, 1, 1, 1, 1, 1]]} # fmt: skip
# camembert is a french model. So we also use french texts.
sequences = [
"Le transformeur est un modèle d'apprentissage profond introduit en 2017, "
"utilisé principalement dans le domaine du traitement automatique des langues (TAL).",
"À l'instar des réseaux de neurones récurrents (RNN), les transformeurs sont conçus "
"pour gérer des données séquentielles, telles que le langage naturel, pour des tâches "
"telles que la traduction et la synthèse de texte.",
]
self.tokenizer_integration_test_util(
expected_encoding=expected_encoding,
model_name="camembert-base",
revision="3a0641d9a1aeb7e848a74299e7e4c4bca216b4cf",
sequences=sequences,
)
# Overwritten because we have to use from slow (online pretrained is wrong, the tokenizer.json has a whole)
def test_added_tokens_serialization(self):
self.maxDiff = None
# Utility to test the added vocab
def _test_added_vocab_and_eos(expected, tokenizer_class, expected_eos, temp_dir):
tokenizer = tokenizer_class.from_pretrained(temp_dir)
self.assertTrue(str(expected_eos) not in tokenizer.additional_special_tokens)
self.assertIn(new_eos, tokenizer.added_tokens_decoder.values())
self.assertEqual(tokenizer.added_tokens_decoder[tokenizer.eos_token_id], new_eos)
self.assertDictEqual(expected, tokenizer.added_tokens_decoder)
return tokenizer
new_eos = AddedToken("[NEW_EOS]", rstrip=False, lstrip=True, normalized=False)
for tokenizer, pretrained_name, kwargs in self.tokenizers_list:
with self.subTest(f"{tokenizer.__class__.__name__} ({pretrained_name})"):
# Load a slow tokenizer from the hub, init with the new token for fast to also include it
tokenizer = self.tokenizer_class.from_pretrained(pretrained_name, eos_token=new_eos)
EXPECTED_ADDED_TOKENS_DECODER = tokenizer.added_tokens_decoder
with self.subTest("Hub -> Slow: Test loading a slow tokenizer from the hub)"):
self.assertEqual(tokenizer._eos_token, new_eos)
self.assertIn(new_eos, list(tokenizer.added_tokens_decoder.values()))
with tempfile.TemporaryDirectory() as tmp_dir_2:
tokenizer.save_pretrained(tmp_dir_2)
with self.subTest(
"Hub -> Slow -> Slow: Test saving this slow tokenizer and reloading it in the fast class"
):
_test_added_vocab_and_eos(
EXPECTED_ADDED_TOKENS_DECODER, self.tokenizer_class, new_eos, tmp_dir_2
)
if self.rust_tokenizer_class is not None:
with self.subTest(
"Hub -> Slow -> Fast: Test saving this slow tokenizer and reloading it in the fast class"
):
tokenizer_fast = _test_added_vocab_and_eos(
EXPECTED_ADDED_TOKENS_DECODER, self.rust_tokenizer_class, new_eos, tmp_dir_2
)
with tempfile.TemporaryDirectory() as tmp_dir_3:
tokenizer_fast.save_pretrained(tmp_dir_3)
with self.subTest(
"Hub -> Slow -> Fast -> Fast: Test saving this fast tokenizer and reloading it in the fast class"
):
_test_added_vocab_and_eos(
EXPECTED_ADDED_TOKENS_DECODER, self.rust_tokenizer_class, new_eos, tmp_dir_3
)
with self.subTest(
"Hub -> Slow -> Fast -> Slow: Test saving this slow tokenizer and reloading it in the slow class"
):
_test_added_vocab_and_eos(
EXPECTED_ADDED_TOKENS_DECODER, self.rust_tokenizer_class, new_eos, tmp_dir_3
)
with self.subTest("Hub -> Fast: Test loading a fast tokenizer from the hub)"):
if self.rust_tokenizer_class is not None:
tokenizer_fast = self.rust_tokenizer_class.from_pretrained(
pretrained_name, eos_token=new_eos, from_slow=True
)
self.assertEqual(tokenizer_fast._eos_token, new_eos)
self.assertIn(new_eos, list(tokenizer_fast.added_tokens_decoder.values()))
# We can't test the following because for BC we kept the default rstrip lstrip in slow not fast. Will comment once normalization is alright
with self.subTest("Hub -> Fast == Hub -> Slow: make sure slow and fast tokenizer match"):
self.assertDictEqual(EXPECTED_ADDED_TOKENS_DECODER, tokenizer_fast.added_tokens_decoder)
EXPECTED_ADDED_TOKENS_DECODER = tokenizer_fast.added_tokens_decoder
with tempfile.TemporaryDirectory() as tmp_dir_4:
tokenizer_fast.save_pretrained(tmp_dir_4)
with self.subTest("Hub -> Fast -> Fast: saving Fast1 locally and loading"):
_test_added_vocab_and_eos(
EXPECTED_ADDED_TOKENS_DECODER, self.rust_tokenizer_class, new_eos, tmp_dir_4
)
with self.subTest("Hub -> Fast -> Slow: saving Fast1 locally and loading"):
_test_added_vocab_and_eos(
EXPECTED_ADDED_TOKENS_DECODER, self.tokenizer_class, new_eos, tmp_dir_4
)
| transformers/tests/models/camembert/test_tokenization_camembert.py/0 | {
"file_path": "transformers/tests/models/camembert/test_tokenization_camembert.py",
"repo_id": "transformers",
"token_count": 5451
} | 349 |
# coding=utf-8
# Copyright 2022 The OpenBMB Team and The HuggingFace Inc. team. All rights reserved.
#
# Licensed under the Apache License, Version 2.0 (the "License");
# you may not use this file except in compliance with the License.
# You may obtain a copy of the License at
#
# http://www.apache.org/licenses/LICENSE-2.0
#
# Unless required by applicable law or agreed to in writing, software
# distributed under the License is distributed on an "AS IS" BASIS,
# WITHOUT WARRANTIES OR CONDITIONS OF ANY KIND, either express or implied.
# See the License for the specific language governing permissions and
# limitations under the License.
""" Testing suite for the PyTorch CPMAnt model. """
import unittest
from transformers.testing_utils import is_torch_available, require_torch, tooslow
from ...generation.test_utils import torch_device
from ...test_configuration_common import ConfigTester
from ...test_modeling_common import ModelTesterMixin, ids_tensor
from ...test_pipeline_mixin import PipelineTesterMixin
if is_torch_available():
import torch
from transformers import (
CpmAntConfig,
CpmAntForCausalLM,
CpmAntModel,
CpmAntTokenizer,
)
@require_torch
class CpmAntModelTester:
def __init__(
self,
parent,
batch_size=2,
seq_length=8,
is_training=True,
use_token_type_ids=False,
use_input_mask=False,
use_labels=False,
use_mc_token_ids=False,
vocab_size=99,
hidden_size=32,
num_hidden_layers=2,
num_attention_heads=4,
intermediate_size=37,
num_buckets=32,
max_distance=128,
prompt_length=8,
prompt_types=8,
segment_types=8,
init_std=1.0,
return_dict=True,
):
self.parent = parent
self.batch_size = batch_size
self.seq_length = seq_length
self.is_training = is_training
self.use_token_type_ids = use_token_type_ids
self.use_input_mask = use_input_mask
self.use_labels = use_labels
self.use_mc_token_ids = use_mc_token_ids
self.vocab_size = vocab_size
self.hidden_size = hidden_size
self.num_hidden_layers = num_hidden_layers
self.num_attention_heads = num_attention_heads
self.intermediate_size = intermediate_size
self.num_buckets = num_buckets
self.max_distance = max_distance
self.prompt_length = prompt_length
self.prompt_types = prompt_types
self.segment_types = segment_types
self.init_std = init_std
self.return_dict = return_dict
def prepare_config_and_inputs(self):
input_ids = {}
input_ids["input_ids"] = ids_tensor([self.batch_size, self.seq_length], self.vocab_size).type(torch.int32)
input_ids["use_cache"] = False
config = self.get_config()
return (config, input_ids)
def get_config(self):
return CpmAntConfig(
vocab_size=self.vocab_size,
hidden_size=self.hidden_size,
num_hidden_layers=self.num_hidden_layers,
num_attention_heads=self.num_attention_heads,
dim_ff=self.intermediate_size,
position_bias_num_buckets=self.num_buckets,
position_bias_max_distance=self.max_distance,
prompt_types=self.prompt_types,
prompt_length=self.prompt_length,
segment_types=self.segment_types,
use_cache=True,
init_std=self.init_std,
return_dict=self.return_dict,
)
def create_and_check_cpmant_model(self, config, input_ids, *args):
model = CpmAntModel(config=config)
model.to(torch_device)
model.eval()
hidden_states = model(**input_ids).last_hidden_state
self.parent.assertEqual(hidden_states.shape, (self.batch_size, self.seq_length, config.hidden_size))
def create_and_check_lm_head_model(self, config, input_ids, *args):
model = CpmAntForCausalLM(config)
model.to(torch_device)
input_ids["input_ids"] = input_ids["input_ids"].to(torch_device)
model.eval()
model_output = model(**input_ids)
self.parent.assertEqual(
model_output.logits.shape,
(self.batch_size, self.seq_length, config.vocab_size + config.prompt_types * config.prompt_length),
)
def prepare_config_and_inputs_for_common(self):
config, inputs_dict = self.prepare_config_and_inputs()
return config, inputs_dict
@require_torch
class CpmAntModelTest(ModelTesterMixin, PipelineTesterMixin, unittest.TestCase):
all_model_classes = (CpmAntModel, CpmAntForCausalLM) if is_torch_available() else ()
pipeline_model_mapping = (
{"feature-extraction": CpmAntModel, "text-generation": CpmAntForCausalLM} if is_torch_available() else {}
)
test_pruning = False
test_missing_keys = False
test_mismatched_shapes = False
test_head_masking = False
test_resize_embeddings = False
def setUp(self):
self.model_tester = CpmAntModelTester(self)
self.config_tester = ConfigTester(self, config_class=CpmAntConfig)
def test_config(self):
self.config_tester.create_and_test_config_common_properties()
self.config_tester.create_and_test_config_to_json_string()
self.config_tester.create_and_test_config_to_json_file()
self.config_tester.create_and_test_config_from_and_save_pretrained()
self.config_tester.check_config_can_be_init_without_params()
self.config_tester.check_config_arguments_init()
def test_inputs_embeds(self):
unittest.skip("CPMAnt doesn't support input_embeds.")(self.test_inputs_embeds)
def test_retain_grad_hidden_states_attentions(self):
unittest.skip(
"CPMAnt doesn't support retain grad in hidden_states or attentions, because prompt management will peel off the output.hidden_states from graph.\
So is attentions. We strongly recommand you use loss to tune model."
)(self.test_retain_grad_hidden_states_attentions)
def test_cpmant_model(self):
config, inputs = self.model_tester.prepare_config_and_inputs()
self.model_tester.create_and_check_cpmant_model(config, inputs)
def test_cpmant_lm_head_model(self):
config, inputs = self.model_tester.prepare_config_and_inputs()
self.model_tester.create_and_check_lm_head_model(config, inputs)
@require_torch
class CpmAntModelIntegrationTest(unittest.TestCase):
@tooslow
def test_inference_masked_lm(self):
texts = "今天天气真好!"
model_path = "openbmb/cpm-ant-10b"
model = CpmAntModel.from_pretrained(model_path)
tokenizer = CpmAntTokenizer.from_pretrained(model_path)
inputs = tokenizer(texts, return_tensors="pt")
hidden_states = model(**inputs).last_hidden_state
expected_slice = torch.tensor(
[[[6.1708, 5.9244, 1.0835], [6.5207, 6.2893, -11.3324], [-1.0107, -0.0576, -5.9577]]],
)
self.assertTrue(torch.allclose(hidden_states[:, :3, :3], expected_slice, atol=1e-2))
@require_torch
class CpmAntForCausalLMlIntegrationTest(unittest.TestCase):
@tooslow
def test_inference_casual(self):
texts = "今天天气真好!"
model_path = "openbmb/cpm-ant-10b"
model = CpmAntForCausalLM.from_pretrained(model_path)
tokenizer = CpmAntTokenizer.from_pretrained(model_path)
inputs = tokenizer(texts, return_tensors="pt")
hidden_states = model(**inputs).logits
expected_slice = torch.tensor(
[[[-6.4267, -6.4083, -6.3958], [-5.8802, -5.9447, -5.7811], [-5.3896, -5.4820, -5.4295]]],
)
self.assertTrue(torch.allclose(hidden_states[:, :3, :3], expected_slice, atol=1e-2))
@tooslow
def test_simple_generation(self):
model_path = "openbmb/cpm-ant-10b"
model = CpmAntForCausalLM.from_pretrained(model_path)
tokenizer = CpmAntTokenizer.from_pretrained(model_path)
texts = "今天天气不错,"
expected_output = "今天天气不错,阳光明媚,我和妈妈一起去超市买东西。\n在超市里,我看到了一个很好玩的玩具,它的名字叫“机器人”。它有一个圆圆的脑袋,两只圆圆的眼睛,还有一个圆圆的"
model_inputs = tokenizer(texts, return_tensors="pt")
token_ids = model.generate(**model_inputs)
output_texts = tokenizer.batch_decode(token_ids)
self.assertEqual(expected_output, output_texts)
@tooslow
def test_batch_generation(self):
model_path = "openbmb/cpm-ant-10b"
model = CpmAntForCausalLM.from_pretrained(model_path)
tokenizer = CpmAntTokenizer.from_pretrained(model_path)
texts = ["今天天气不错,", "新年快乐,万事如意!"]
expected_output = [
"今天天气不错,阳光明媚,我和妈妈一起去超市买东西。\n在超市里,我看到了一个很好玩的玩具,它的名字叫“机器人”。它有一个圆圆的脑袋,两只圆圆的眼睛,还有一个圆圆的",
"新年快乐,万事如意!在这辞旧迎新的美好时刻,我谨代表《农村新技术》杂志社全体同仁,向一直以来关心、支持《农村新技术》杂志发展的各级领导、各界朋友和广大读者致以最诚挚的",
]
model_inputs = tokenizer(texts, return_tensors="pt", padding=True)
token_ids = model.generate(**model_inputs)
output_texts = tokenizer.batch_decode(token_ids)
self.assertEqual(expected_output, output_texts)
| transformers/tests/models/cpmant/test_modeling_cpmant.py/0 | {
"file_path": "transformers/tests/models/cpmant/test_modeling_cpmant.py",
"repo_id": "transformers",
"token_count": 4461
} | 350 |
# coding=utf-8
# Copyright 2021 The HuggingFace Inc. team. All rights reserved.
#
# Licensed under the Apache License, Version 2.0 (the "License");
# you may not use this file except in compliance with the License.
# You may obtain a copy of the License at
#
# http://www.apache.org/licenses/LICENSE-2.0
#
# Unless required by applicable law or agreed to in writing, software
# distributed under the License is distributed on an "AS IS" BASIS,
# WITHOUT WARRANTIES OR CONDITIONS OF ANY KIND, either express or implied.
# See the License for the specific language governing permissions and
# limitations under the License.
from __future__ import annotations
import unittest
from transformers import DebertaConfig, is_tf_available
from transformers.testing_utils import require_tf, slow
from ...test_configuration_common import ConfigTester
from ...test_modeling_tf_common import TFModelTesterMixin, ids_tensor, random_attention_mask
from ...test_pipeline_mixin import PipelineTesterMixin
if is_tf_available():
import tensorflow as tf
from transformers import (
TFDebertaForMaskedLM,
TFDebertaForQuestionAnswering,
TFDebertaForSequenceClassification,
TFDebertaForTokenClassification,
TFDebertaModel,
)
class TFDebertaModelTester:
def __init__(
self,
parent,
batch_size=13,
seq_length=7,
is_training=True,
use_input_mask=True,
use_token_type_ids=True,
use_labels=True,
vocab_size=99,
hidden_size=32,
num_hidden_layers=2,
num_attention_heads=4,
intermediate_size=37,
hidden_act="gelu",
hidden_dropout_prob=0.1,
attention_probs_dropout_prob=0.1,
max_position_embeddings=512,
type_vocab_size=16,
type_sequence_label_size=2,
initializer_range=0.02,
num_labels=3,
num_choices=4,
scope=None,
):
self.parent = parent
self.batch_size = 13
self.seq_length = 7
self.is_training = True
self.use_input_mask = True
self.use_token_type_ids = True
self.use_labels = True
self.vocab_size = 99
self.hidden_size = 32
self.num_hidden_layers = 2
self.num_attention_heads = 4
self.intermediate_size = 37
self.hidden_act = "gelu"
self.hidden_dropout_prob = 0.1
self.attention_probs_dropout_prob = 0.1
self.max_position_embeddings = 512
self.type_vocab_size = 16
self.relative_attention = False
self.max_relative_positions = -1
self.position_biased_input = True
self.type_sequence_label_size = 2
self.initializer_range = 0.02
self.num_labels = 3
self.num_choices = 4
self.scope = None
def prepare_config_and_inputs(self):
input_ids = ids_tensor([self.batch_size, self.seq_length], self.vocab_size)
input_mask = None
if self.use_input_mask:
input_mask = random_attention_mask([self.batch_size, self.seq_length])
token_type_ids = None
if self.use_token_type_ids:
token_type_ids = ids_tensor([self.batch_size, self.seq_length], self.type_vocab_size)
sequence_labels = None
token_labels = None
choice_labels = None
if self.use_labels:
sequence_labels = ids_tensor([self.batch_size], self.type_sequence_label_size)
token_labels = ids_tensor([self.batch_size, self.seq_length], self.num_labels)
config = DebertaConfig(
vocab_size=self.vocab_size,
hidden_size=self.hidden_size,
num_hidden_layers=self.num_hidden_layers,
num_attention_heads=self.num_attention_heads,
intermediate_size=self.intermediate_size,
hidden_act=self.hidden_act,
hidden_dropout_prob=self.hidden_dropout_prob,
attention_probs_dropout_prob=self.attention_probs_dropout_prob,
max_position_embeddings=self.max_position_embeddings,
type_vocab_size=self.type_vocab_size,
relative_attention=self.relative_attention,
max_relative_positions=self.max_relative_positions,
position_biased_input=self.position_biased_input,
initializer_range=self.initializer_range,
return_dict=True,
)
return config, input_ids, token_type_ids, input_mask, sequence_labels, token_labels, choice_labels
def create_and_check_model(
self, config, input_ids, token_type_ids, input_mask, sequence_labels, token_labels, choice_labels
):
model = TFDebertaModel(config=config)
inputs = {"input_ids": input_ids, "attention_mask": input_mask, "token_type_ids": token_type_ids}
inputs = [input_ids, input_mask]
result = model(inputs)
result = model(input_ids)
self.parent.assertEqual(result.last_hidden_state.shape, (self.batch_size, self.seq_length, self.hidden_size))
def create_and_check_for_masked_lm(
self, config, input_ids, token_type_ids, input_mask, sequence_labels, token_labels, choice_labels
):
model = TFDebertaForMaskedLM(config=config)
inputs = {
"input_ids": input_ids,
"attention_mask": input_mask,
"token_type_ids": token_type_ids,
}
result = model(inputs)
self.parent.assertEqual(result.logits.shape, (self.batch_size, self.seq_length, self.vocab_size))
def create_and_check_for_sequence_classification(
self, config, input_ids, token_type_ids, input_mask, sequence_labels, token_labels, choice_labels
):
config.num_labels = self.num_labels
model = TFDebertaForSequenceClassification(config=config)
inputs = {
"input_ids": input_ids,
"attention_mask": input_mask,
"token_type_ids": token_type_ids,
}
result = model(inputs)
self.parent.assertEqual(result.logits.shape, (self.batch_size, self.num_labels))
def create_and_check_for_token_classification(
self, config, input_ids, token_type_ids, input_mask, sequence_labels, token_labels, choice_labels
):
config.num_labels = self.num_labels
model = TFDebertaForTokenClassification(config=config)
inputs = {
"input_ids": input_ids,
"attention_mask": input_mask,
"token_type_ids": token_type_ids,
}
result = model(inputs)
self.parent.assertEqual(result.logits.shape, (self.batch_size, self.seq_length, self.num_labels))
def create_and_check_for_question_answering(
self, config, input_ids, token_type_ids, input_mask, sequence_labels, token_labels, choice_labels
):
model = TFDebertaForQuestionAnswering(config=config)
inputs = {
"input_ids": input_ids,
"attention_mask": input_mask,
"token_type_ids": token_type_ids,
}
result = model(inputs)
self.parent.assertEqual(result.start_logits.shape, (self.batch_size, self.seq_length))
self.parent.assertEqual(result.end_logits.shape, (self.batch_size, self.seq_length))
def prepare_config_and_inputs_for_common(self):
config_and_inputs = self.prepare_config_and_inputs()
(
config,
input_ids,
token_type_ids,
input_mask,
sequence_labels,
token_labels,
choice_labels,
) = config_and_inputs
inputs_dict = {"input_ids": input_ids, "token_type_ids": token_type_ids, "attention_mask": input_mask}
return config, inputs_dict
@require_tf
class TFDebertaModelTest(TFModelTesterMixin, PipelineTesterMixin, unittest.TestCase):
all_model_classes = (
(
TFDebertaModel,
TFDebertaForMaskedLM,
TFDebertaForQuestionAnswering,
TFDebertaForSequenceClassification,
TFDebertaForTokenClassification,
)
if is_tf_available()
else ()
)
pipeline_model_mapping = (
{
"feature-extraction": TFDebertaModel,
"fill-mask": TFDebertaForMaskedLM,
"question-answering": TFDebertaForQuestionAnswering,
"text-classification": TFDebertaForSequenceClassification,
"token-classification": TFDebertaForTokenClassification,
"zero-shot": TFDebertaForSequenceClassification,
}
if is_tf_available()
else {}
)
test_head_masking = False
test_onnx = False
def setUp(self):
self.model_tester = TFDebertaModelTester(self)
self.config_tester = ConfigTester(self, config_class=DebertaConfig, hidden_size=37)
def test_config(self):
self.config_tester.run_common_tests()
def test_model(self):
config_and_inputs = self.model_tester.prepare_config_and_inputs()
self.model_tester.create_and_check_model(*config_and_inputs)
def test_for_masked_lm(self):
config_and_inputs = self.model_tester.prepare_config_and_inputs()
self.model_tester.create_and_check_for_masked_lm(*config_and_inputs)
def test_for_question_answering(self):
config_and_inputs = self.model_tester.prepare_config_and_inputs()
self.model_tester.create_and_check_for_question_answering(*config_and_inputs)
def test_for_sequence_classification(self):
config_and_inputs = self.model_tester.prepare_config_and_inputs()
self.model_tester.create_and_check_for_sequence_classification(*config_and_inputs)
def test_for_token_classification(self):
config_and_inputs = self.model_tester.prepare_config_and_inputs()
self.model_tester.create_and_check_for_token_classification(*config_and_inputs)
@slow
def test_model_from_pretrained(self):
model = TFDebertaModel.from_pretrained("kamalkraj/deberta-base")
self.assertIsNotNone(model)
@require_tf
class TFDeBERTaModelIntegrationTest(unittest.TestCase):
@unittest.skip(reason="Model not available yet")
def test_inference_masked_lm(self):
pass
@slow
def test_inference_no_head(self):
model = TFDebertaModel.from_pretrained("kamalkraj/deberta-base")
input_ids = tf.constant([[0, 31414, 232, 328, 740, 1140, 12695, 69, 46078, 1588, 2]])
attention_mask = tf.constant([[0, 1, 1, 1, 1, 1, 1, 1, 1, 1, 1]])
output = model(input_ids, attention_mask=attention_mask)[0]
expected_slice = tf.constant(
[
[
[-0.59855896, -0.80552566, -0.8462135],
[1.4484025, -0.93483794, -0.80593085],
[0.3122741, 0.00316059, -1.4131377],
]
]
)
tf.debugging.assert_near(output[:, 1:4, 1:4], expected_slice, atol=1e-4)
| transformers/tests/models/deberta/test_modeling_tf_deberta.py/0 | {
"file_path": "transformers/tests/models/deberta/test_modeling_tf_deberta.py",
"repo_id": "transformers",
"token_count": 4967
} | 351 |
# coding=utf-8
# Copyright 2024 The HuggingFace Inc. team. All rights reserved.
#
# Licensed under the Apache License, Version 2.0 (the "License");
# you may not use this file except in compliance with the License.
# You may obtain a copy of the License at
#
# http://www.apache.org/licenses/LICENSE-2.0
#
# Unless required by applicable law or agreed to in writing, software
# distributed under the License is distributed on an "AS IS" BASIS,
# WITHOUT WARRANTIES OR CONDITIONS OF ANY KIND, either express or implied.
# See the License for the specific language governing permissions and
# limitations under the License.
""" Testing suite for the PyTorch Depth Anything model. """
import unittest
from transformers import DepthAnythingConfig, Dinov2Config
from transformers.file_utils import is_torch_available, is_vision_available
from transformers.testing_utils import require_torch, require_vision, slow, torch_device
from ...test_configuration_common import ConfigTester
from ...test_modeling_common import ModelTesterMixin, floats_tensor, ids_tensor
from ...test_pipeline_mixin import PipelineTesterMixin
if is_torch_available():
import torch
from transformers import DepthAnythingForDepthEstimation
from transformers.models.depth_anything.modeling_depth_anything import DEPTH_ANYTHING_PRETRAINED_MODEL_ARCHIVE_LIST
if is_vision_available():
from PIL import Image
from transformers import DPTImageProcessor
class DepthAnythingModelTester:
# Copied from tests.models.dpt.test_modeling_dpt_auto_backbone.DPTModelTester.__init__
def __init__(
self,
parent,
batch_size=2,
num_channels=3,
image_size=32,
patch_size=16,
use_labels=True,
num_labels=3,
is_training=True,
hidden_size=4,
num_hidden_layers=2,
num_attention_heads=2,
intermediate_size=8,
out_features=["stage1", "stage2"],
apply_layernorm=False,
reshape_hidden_states=False,
neck_hidden_sizes=[2, 2],
fusion_hidden_size=6,
):
self.parent = parent
self.batch_size = batch_size
self.num_channels = num_channels
self.image_size = image_size
self.patch_size = patch_size
self.hidden_size = hidden_size
self.num_hidden_layers = num_hidden_layers
self.num_attention_heads = num_attention_heads
self.intermediate_size = intermediate_size
self.out_features = out_features
self.apply_layernorm = apply_layernorm
self.reshape_hidden_states = reshape_hidden_states
self.use_labels = use_labels
self.num_labels = num_labels
self.is_training = is_training
self.neck_hidden_sizes = neck_hidden_sizes
self.fusion_hidden_size = fusion_hidden_size
# DPT's sequence length
self.seq_length = (self.image_size // self.patch_size) ** 2 + 1
# Copied from tests.models.dpt.test_modeling_dpt_auto_backbone.DPTModelTester.prepare_config_and_inputs
def prepare_config_and_inputs(self):
pixel_values = floats_tensor([self.batch_size, self.num_channels, self.image_size, self.image_size])
labels = None
if self.use_labels:
labels = ids_tensor([self.batch_size, self.image_size, self.image_size], self.num_labels)
config = self.get_config()
return config, pixel_values, labels
def get_config(self):
return DepthAnythingConfig(
backbone_config=self.get_backbone_config(),
reassemble_hidden_size=self.hidden_size,
patch_size=self.patch_size,
neck_hidden_sizes=self.neck_hidden_sizes,
fusion_hidden_size=self.fusion_hidden_size,
)
# Copied from tests.models.dpt.test_modeling_dpt_auto_backbone.DPTModelTester.get_backbone_config
def get_backbone_config(self):
return Dinov2Config(
image_size=self.image_size,
patch_size=self.patch_size,
num_channels=self.num_channels,
hidden_size=self.hidden_size,
num_hidden_layers=self.num_hidden_layers,
num_attention_heads=self.num_attention_heads,
intermediate_size=self.intermediate_size,
is_training=self.is_training,
out_features=self.out_features,
reshape_hidden_states=self.reshape_hidden_states,
)
# Copied from tests.models.dpt.test_modeling_dpt_auto_backbone.DPTModelTester.create_and_check_for_depth_estimation with DPT->DepthAnything
def create_and_check_for_depth_estimation(self, config, pixel_values, labels):
config.num_labels = self.num_labels
model = DepthAnythingForDepthEstimation(config)
model.to(torch_device)
model.eval()
result = model(pixel_values)
self.parent.assertEqual(result.predicted_depth.shape, (self.batch_size, self.image_size, self.image_size))
# Copied from tests.models.dpt.test_modeling_dpt_auto_backbone.DPTModelTester.prepare_config_and_inputs_for_common
def prepare_config_and_inputs_for_common(self):
config_and_inputs = self.prepare_config_and_inputs()
config, pixel_values, labels = config_and_inputs
inputs_dict = {"pixel_values": pixel_values}
return config, inputs_dict
@require_torch
class DepthAnythingModelTest(ModelTesterMixin, PipelineTesterMixin, unittest.TestCase):
"""
Here we also overwrite some of the tests of test_modeling_common.py, as Depth Anything does not use input_ids, inputs_embeds,
attention_mask and seq_length.
"""
all_model_classes = (DepthAnythingForDepthEstimation,) if is_torch_available() else ()
pipeline_model_mapping = {"depth-estimation": DepthAnythingForDepthEstimation} if is_torch_available() else {}
test_pruning = False
test_resize_embeddings = False
test_head_masking = False
def setUp(self):
self.model_tester = DepthAnythingModelTester(self)
self.config_tester = ConfigTester(
self, config_class=DepthAnythingConfig, has_text_modality=False, hidden_size=37
)
def test_config(self):
self.config_tester.create_and_test_config_to_json_string()
self.config_tester.create_and_test_config_to_json_file()
self.config_tester.create_and_test_config_from_and_save_pretrained()
self.config_tester.create_and_test_config_from_and_save_pretrained_subfolder()
self.config_tester.create_and_test_config_with_num_labels()
self.config_tester.check_config_can_be_init_without_params()
self.config_tester.check_config_arguments_init()
@unittest.skip(reason="Depth Anything with AutoBackbone does not have a base model and hence no input_embeddings")
def test_inputs_embeds(self):
pass
def test_for_depth_estimation(self):
config_and_inputs = self.model_tester.prepare_config_and_inputs()
self.model_tester.create_and_check_for_depth_estimation(*config_and_inputs)
@unittest.skip(reason="Depth Anything does not support training yet")
def test_training(self):
pass
@unittest.skip(reason="Depth Anything does not support training yet")
def test_training_gradient_checkpointing(self):
pass
@unittest.skip(reason="Depth Anything with AutoBackbone does not have a base model and hence no input_embeddings")
def test_model_common_attributes(self):
pass
@unittest.skip(reason="Depth Anything with AutoBackbone does not have a base model")
def test_save_load_fast_init_from_base(self):
pass
@unittest.skip(reason="Depth Anything with AutoBackbone does not have a base model")
def test_save_load_fast_init_to_base(self):
pass
@unittest.skip(
reason="This architecture seems to not compute gradients properly when using GC, check: https://github.com/huggingface/transformers/pull/27124"
)
def test_training_gradient_checkpointing_use_reentrant(self):
pass
@unittest.skip(
reason="This architecture seems to not compute gradients properly when using GC, check: https://github.com/huggingface/transformers/pull/27124"
)
def test_training_gradient_checkpointing_use_reentrant_false(self):
pass
@slow
def test_model_from_pretrained(self):
for model_name in DEPTH_ANYTHING_PRETRAINED_MODEL_ARCHIVE_LIST[:1]:
model = DepthAnythingForDepthEstimation.from_pretrained(model_name)
self.assertIsNotNone(model)
# We will verify our results on an image of cute cats
def prepare_img():
image = Image.open("./tests/fixtures/tests_samples/COCO/000000039769.png")
return image
@require_torch
@require_vision
@slow
class DepthAnythingModelIntegrationTest(unittest.TestCase):
def test_inference(self):
image_processor = DPTImageProcessor.from_pretrained("LiheYoung/depth-anything-small-hf")
model = DepthAnythingForDepthEstimation.from_pretrained("LiheYoung/depth-anything-small-hf").to(torch_device)
image = prepare_img()
inputs = image_processor(images=image, return_tensors="pt").to(torch_device)
# forward pass
with torch.no_grad():
outputs = model(**inputs)
predicted_depth = outputs.predicted_depth
# verify the predicted depth
expected_shape = torch.Size([1, 518, 686])
self.assertEqual(predicted_depth.shape, expected_shape)
expected_slice = torch.tensor(
[[8.8204, 8.6468, 8.6195], [8.3313, 8.6027, 8.7526], [8.6526, 8.6866, 8.7453]],
).to(torch_device)
self.assertTrue(torch.allclose(outputs.predicted_depth[0, :3, :3], expected_slice, atol=1e-6))
| transformers/tests/models/depth_anything/test_modeling_depth_anything.py/0 | {
"file_path": "transformers/tests/models/depth_anything/test_modeling_depth_anything.py",
"repo_id": "transformers",
"token_count": 3846
} | 352 |
# coding=utf-8
# Copyright 2021 HuggingFace Inc.
#
# Licensed under the Apache License, Version 2.0 (the "License");
# you may not use this file except in compliance with the License.
# You may obtain a copy of the License at
#
# http://www.apache.org/licenses/LICENSE-2.0
#
# Unless required by applicable law or agreed to in writing, software
# distributed under the License is distributed on an "AS IS" BASIS,
# WITHOUT WARRANTIES OR CONDITIONS OF ANY KIND, either express or implied.
# See the License for the specific language governing permissions and
# limitations under the License.
import unittest
from transformers.testing_utils import require_torch, require_vision
from transformers.utils import is_vision_available
from ...test_image_processing_common import ImageProcessingTestMixin, prepare_image_inputs
if is_vision_available():
from transformers import ViTImageProcessor
class EfficientFormerImageProcessorTester(unittest.TestCase):
def __init__(
self,
parent,
batch_size=13,
num_channels=3,
image_size=224,
min_resolution=30,
max_resolution=400,
do_resize=True,
size=None,
do_normalize=True,
image_mean=[0.5, 0.5, 0.5],
image_std=[0.5, 0.5, 0.5],
):
size = size if size is not None else {"height": 18, "width": 18}
self.parent = parent
self.batch_size = batch_size
self.num_channels = num_channels
self.image_size = image_size
self.min_resolution = min_resolution
self.max_resolution = max_resolution
self.do_resize = do_resize
self.size = size
self.do_normalize = do_normalize
self.image_mean = image_mean
self.image_std = image_std
def prepare_image_processor_dict(self):
return {
"image_mean": self.image_mean,
"image_std": self.image_std,
"do_normalize": self.do_normalize,
"do_resize": self.do_resize,
"size": self.size,
}
def expected_output_image_shape(self, images):
return self.num_channels, self.size["height"], self.size["width"]
def prepare_image_inputs(self, equal_resolution=False, numpify=False, torchify=False):
return prepare_image_inputs(
batch_size=self.batch_size,
num_channels=self.num_channels,
min_resolution=self.min_resolution,
max_resolution=self.max_resolution,
equal_resolution=equal_resolution,
numpify=numpify,
torchify=torchify,
)
@require_torch
@require_vision
class EfficientFormerImageProcessorTest(ImageProcessingTestMixin, unittest.TestCase):
image_processing_class = ViTImageProcessor if is_vision_available() else None
def setUp(self):
self.image_processor_tester = EfficientFormerImageProcessorTester(self)
@property
def image_processor_dict(self):
return self.image_processor_tester.prepare_image_processor_dict()
def test_image_proc_properties(self):
image_processor = self.image_processing_class(**self.image_processor_dict)
self.assertTrue(hasattr(image_processor, "image_mean"))
self.assertTrue(hasattr(image_processor, "image_std"))
self.assertTrue(hasattr(image_processor, "do_normalize"))
self.assertTrue(hasattr(image_processor, "do_resize"))
self.assertTrue(hasattr(image_processor, "size"))
| transformers/tests/models/efficientformer/test_image_processing_efficientformer.py/0 | {
"file_path": "transformers/tests/models/efficientformer/test_image_processing_efficientformer.py",
"repo_id": "transformers",
"token_count": 1379
} | 353 |
# coding=utf-8
# Copyright 2020 The HuggingFace Team. All rights reserved.
#
# Licensed under the Apache License, Version 2.0 (the "License");
# you may not use this file except in compliance with the License.
# You may obtain a copy of the License at
#
# http://www.apache.org/licenses/LICENSE-2.0
#
# Unless required by applicable law or agreed to in writing, software
# distributed under the License is distributed on an "AS IS" BASIS,
# WITHOUT WARRANTIES OR CONDITIONS OF ANY KIND, either express or implied.
# See the License for the specific language governing permissions and
# limitations under the License.
import datetime
import gc
import math
import unittest
from transformers import GPT2Config, is_torch_available
from transformers.testing_utils import backend_empty_cache, require_torch, slow, torch_device
from ...generation.test_utils import GenerationTesterMixin
from ...test_configuration_common import ConfigTester
from ...test_modeling_common import ModelTesterMixin, floats_tensor, ids_tensor, random_attention_mask
from ...test_pipeline_mixin import PipelineTesterMixin
if is_torch_available():
import torch
from transformers import (
GPT2_PRETRAINED_MODEL_ARCHIVE_LIST,
GPT2DoubleHeadsModel,
GPT2ForQuestionAnswering,
GPT2ForSequenceClassification,
GPT2ForTokenClassification,
GPT2LMHeadModel,
GPT2Model,
GPT2Tokenizer,
)
class GPT2ModelTester:
def __init__(
self,
parent,
batch_size=14,
seq_length=7,
is_training=True,
use_token_type_ids=True,
use_input_mask=True,
use_labels=True,
use_mc_token_ids=True,
vocab_size=99,
hidden_size=32,
num_hidden_layers=2,
num_attention_heads=4,
intermediate_size=37,
hidden_act="gelu",
hidden_dropout_prob=0.1,
attention_probs_dropout_prob=0.1,
max_position_embeddings=512,
type_vocab_size=16,
type_sequence_label_size=2,
initializer_range=0.02,
num_labels=3,
num_choices=4,
scope=None,
):
self.parent = parent
self.batch_size = batch_size
self.seq_length = seq_length
self.is_training = is_training
self.use_token_type_ids = use_token_type_ids
self.use_input_mask = use_input_mask
self.use_labels = use_labels
self.use_mc_token_ids = use_mc_token_ids
self.vocab_size = vocab_size
self.hidden_size = hidden_size
self.num_hidden_layers = num_hidden_layers
self.num_attention_heads = num_attention_heads
self.intermediate_size = intermediate_size
self.hidden_act = hidden_act
self.hidden_dropout_prob = hidden_dropout_prob
self.attention_probs_dropout_prob = attention_probs_dropout_prob
self.max_position_embeddings = max_position_embeddings
self.type_vocab_size = type_vocab_size
self.type_sequence_label_size = type_sequence_label_size
self.initializer_range = initializer_range
self.num_labels = num_labels
self.num_choices = num_choices
self.scope = None
self.bos_token_id = vocab_size - 1
self.eos_token_id = vocab_size - 1
self.pad_token_id = vocab_size - 1
def get_large_model_config(self):
return GPT2Config.from_pretrained("gpt2")
def prepare_config_and_inputs(
self, gradient_checkpointing=False, scale_attn_by_inverse_layer_idx=False, reorder_and_upcast_attn=False
):
input_ids = ids_tensor([self.batch_size, self.seq_length], self.vocab_size)
input_mask = None
if self.use_input_mask:
input_mask = random_attention_mask([self.batch_size, self.seq_length])
token_type_ids = None
if self.use_token_type_ids:
token_type_ids = ids_tensor([self.batch_size, self.seq_length], self.type_vocab_size)
mc_token_ids = None
if self.use_mc_token_ids:
mc_token_ids = ids_tensor([self.batch_size, self.num_choices], self.seq_length)
sequence_labels = None
token_labels = None
choice_labels = None
if self.use_labels:
sequence_labels = ids_tensor([self.batch_size], self.type_sequence_label_size)
token_labels = ids_tensor([self.batch_size, self.seq_length], self.num_labels)
choice_labels = ids_tensor([self.batch_size], self.num_choices)
config = self.get_config(
gradient_checkpointing=gradient_checkpointing,
scale_attn_by_inverse_layer_idx=scale_attn_by_inverse_layer_idx,
reorder_and_upcast_attn=reorder_and_upcast_attn,
)
head_mask = ids_tensor([self.num_hidden_layers, self.num_attention_heads], 2)
return (
config,
input_ids,
input_mask,
head_mask,
token_type_ids,
mc_token_ids,
sequence_labels,
token_labels,
choice_labels,
)
def get_config(
self, gradient_checkpointing=False, scale_attn_by_inverse_layer_idx=False, reorder_and_upcast_attn=False
):
return GPT2Config(
vocab_size=self.vocab_size,
n_embd=self.hidden_size,
n_layer=self.num_hidden_layers,
n_head=self.num_attention_heads,
n_inner=self.intermediate_size,
activation_function=self.hidden_act,
resid_pdrop=self.hidden_dropout_prob,
attn_pdrop=self.attention_probs_dropout_prob,
n_positions=self.max_position_embeddings,
type_vocab_size=self.type_vocab_size,
initializer_range=self.initializer_range,
use_cache=True,
bos_token_id=self.bos_token_id,
eos_token_id=self.eos_token_id,
pad_token_id=self.pad_token_id,
gradient_checkpointing=gradient_checkpointing,
scale_attn_by_inverse_layer_idx=scale_attn_by_inverse_layer_idx,
reorder_and_upcast_attn=reorder_and_upcast_attn,
)
def get_pipeline_config(self):
config = self.get_config()
config.vocab_size = 300
return config
def prepare_config_and_inputs_for_decoder(self):
(
config,
input_ids,
input_mask,
head_mask,
token_type_ids,
mc_token_ids,
sequence_labels,
token_labels,
choice_labels,
) = self.prepare_config_and_inputs()
encoder_hidden_states = floats_tensor([self.batch_size, self.seq_length, self.hidden_size])
encoder_attention_mask = ids_tensor([self.batch_size, self.seq_length], vocab_size=2)
return (
config,
input_ids,
input_mask,
head_mask,
token_type_ids,
sequence_labels,
token_labels,
choice_labels,
encoder_hidden_states,
encoder_attention_mask,
)
def create_and_check_gpt2_model(self, config, input_ids, input_mask, head_mask, token_type_ids, *args):
model = GPT2Model(config=config)
model.to(torch_device)
model.eval()
result = model(input_ids, token_type_ids=token_type_ids, head_mask=head_mask)
result = model(input_ids, token_type_ids=token_type_ids)
result = model(input_ids)
self.parent.assertEqual(result.last_hidden_state.shape, (self.batch_size, self.seq_length, self.hidden_size))
self.parent.assertEqual(len(result.past_key_values), config.n_layer)
def create_and_check_gpt2_model_past(self, config, input_ids, input_mask, head_mask, token_type_ids, *args):
model = GPT2Model(config=config)
model.to(torch_device)
model.eval()
# first forward pass
outputs = model(input_ids, token_type_ids=token_type_ids, use_cache=True)
outputs_use_cache_conf = model(input_ids, token_type_ids=token_type_ids)
outputs_no_past = model(input_ids, token_type_ids=token_type_ids, use_cache=False)
self.parent.assertTrue(len(outputs) == len(outputs_use_cache_conf))
self.parent.assertTrue(len(outputs) == len(outputs_no_past) + 1)
output, past = outputs.to_tuple()
# create hypothetical next token and extent to next_input_ids
next_tokens = ids_tensor((self.batch_size, 1), config.vocab_size)
next_token_types = ids_tensor([self.batch_size, 1], self.type_vocab_size)
# append to next input_ids and token_type_ids
next_input_ids = torch.cat([input_ids, next_tokens], dim=-1)
next_token_type_ids = torch.cat([token_type_ids, next_token_types], dim=-1)
output_from_no_past = model(next_input_ids, token_type_ids=next_token_type_ids)["last_hidden_state"]
output_from_past = model(next_tokens, token_type_ids=next_token_types, past_key_values=past)[
"last_hidden_state"
]
# select random slice
random_slice_idx = ids_tensor((1,), output_from_past.shape[-1]).item()
output_from_no_past_slice = output_from_no_past[:, -1, random_slice_idx].detach()
output_from_past_slice = output_from_past[:, 0, random_slice_idx].detach()
# test that outputs are equal for slice
self.parent.assertTrue(torch.allclose(output_from_past_slice, output_from_no_past_slice, atol=1e-3))
def create_and_check_gpt2_model_attention_mask_past(
self, config, input_ids, input_mask, head_mask, token_type_ids, *args
):
model = GPT2Model(config=config)
model.to(torch_device)
model.eval()
# create attention mask
attn_mask = torch.ones(input_ids.shape, dtype=torch.long, device=torch_device)
half_seq_length = self.seq_length // 2
attn_mask[:, half_seq_length:] = 0
# first forward pass
output, past = model(input_ids, attention_mask=attn_mask).to_tuple()
# create hypothetical next token and extent to next_input_ids
next_tokens = ids_tensor((self.batch_size, 1), config.vocab_size)
# change a random masked slice from input_ids
random_seq_idx_to_change = ids_tensor((1,), half_seq_length).item() + 1
random_other_next_tokens = ids_tensor((self.batch_size, 1), config.vocab_size).squeeze(-1)
input_ids[:, -random_seq_idx_to_change] = random_other_next_tokens
# append to next input_ids and attn_mask
next_input_ids = torch.cat([input_ids, next_tokens], dim=-1)
attn_mask = torch.cat(
[attn_mask, torch.ones((attn_mask.shape[0], 1), dtype=torch.long, device=torch_device)],
dim=1,
)
# get two different outputs
output_from_no_past = model(next_input_ids, attention_mask=attn_mask)["last_hidden_state"]
output_from_past = model(next_tokens, past_key_values=past, attention_mask=attn_mask)["last_hidden_state"]
# select random slice
random_slice_idx = ids_tensor((1,), output_from_past.shape[-1]).item()
output_from_no_past_slice = output_from_no_past[:, -1, random_slice_idx].detach()
output_from_past_slice = output_from_past[:, 0, random_slice_idx].detach()
# test that outputs are equal for slice
self.parent.assertTrue(torch.allclose(output_from_past_slice, output_from_no_past_slice, atol=1e-3))
def create_and_check_gpt2_model_past_large_inputs(
self, config, input_ids, input_mask, head_mask, token_type_ids, *args
):
model = GPT2Model(config=config)
model.to(torch_device)
model.eval()
# first forward pass
outputs = model(input_ids, token_type_ids=token_type_ids, attention_mask=input_mask, use_cache=True)
output, past = outputs.to_tuple()
# create hypothetical next token and extent to next_input_ids
next_tokens = ids_tensor((self.batch_size, 3), config.vocab_size)
next_token_types = ids_tensor([self.batch_size, 3], self.type_vocab_size)
next_mask = ids_tensor((self.batch_size, 3), vocab_size=2)
# append to next input_ids and token_type_ids
next_input_ids = torch.cat([input_ids, next_tokens], dim=-1)
next_token_type_ids = torch.cat([token_type_ids, next_token_types], dim=-1)
next_attention_mask = torch.cat([input_mask, next_mask], dim=-1)
output_from_no_past = model(
next_input_ids, token_type_ids=next_token_type_ids, attention_mask=next_attention_mask
)["last_hidden_state"]
output_from_past = model(
next_tokens, token_type_ids=next_token_types, attention_mask=next_attention_mask, past_key_values=past
)["last_hidden_state"]
self.parent.assertTrue(output_from_past.shape[1] == next_tokens.shape[1])
# select random slice
random_slice_idx = ids_tensor((1,), output_from_past.shape[-1]).item()
output_from_no_past_slice = output_from_no_past[:, -3:, random_slice_idx].detach()
output_from_past_slice = output_from_past[:, :, random_slice_idx].detach()
# test that outputs are equal for slice
self.parent.assertTrue(torch.allclose(output_from_past_slice, output_from_no_past_slice, atol=1e-3))
def create_and_check_lm_head_model(self, config, input_ids, input_mask, head_mask, token_type_ids, *args):
model = GPT2LMHeadModel(config)
model.to(torch_device)
model.eval()
result = model(input_ids, token_type_ids=token_type_ids, labels=input_ids)
self.parent.assertEqual(result.loss.shape, ())
self.parent.assertEqual(result.logits.shape, (self.batch_size, self.seq_length, self.vocab_size))
def create_and_check_forward_and_backwards(
self, config, input_ids, input_mask, head_mask, token_type_ids, *args, gradient_checkpointing=False
):
model = GPT2LMHeadModel(config)
model.to(torch_device)
if gradient_checkpointing:
model.gradient_checkpointing_enable()
result = model(input_ids, token_type_ids=token_type_ids, labels=input_ids)
self.parent.assertEqual(result.loss.shape, ())
self.parent.assertEqual(result.logits.shape, (self.batch_size, self.seq_length, self.vocab_size))
result.loss.backward()
def create_and_check_double_lm_head_model(
self, config, input_ids, input_mask, head_mask, token_type_ids, mc_token_ids, *args
):
model = GPT2DoubleHeadsModel(config)
model.to(torch_device)
model.eval()
multiple_choice_inputs_ids = input_ids.unsqueeze(1).expand(-1, self.num_choices, -1).contiguous()
multiple_choice_input_mask = input_mask.unsqueeze(1).expand(-1, self.num_choices, -1).contiguous()
multiple_choice_token_type_ids = token_type_ids.unsqueeze(1).expand(-1, self.num_choices, -1).contiguous()
inputs = {
"input_ids": multiple_choice_inputs_ids,
"mc_token_ids": mc_token_ids,
"attention_mask": multiple_choice_input_mask,
"token_type_ids": multiple_choice_token_type_ids,
"labels": multiple_choice_inputs_ids,
}
result = model(**inputs)
self.parent.assertEqual(result.loss.shape, ())
self.parent.assertEqual(
result.logits.shape, (self.batch_size, self.num_choices, self.seq_length, self.vocab_size)
)
self.parent.assertEqual(result.mc_logits.shape, (self.batch_size, self.num_choices))
def create_and_check_gpt2_for_question_answering(
self, config, input_ids, input_mask, head_mask, token_type_ids, mc_token_ids, sequence_labels, *args
):
config.num_labels = self.num_labels
model = GPT2ForQuestionAnswering(config)
model.to(torch_device)
model.eval()
result = model(input_ids, attention_mask=input_mask, token_type_ids=token_type_ids)
self.parent.assertEqual(result.start_logits.shape, (self.batch_size, self.seq_length))
self.parent.assertEqual(result.end_logits.shape, (self.batch_size, self.seq_length))
def create_and_check_gpt2_for_sequence_classification(
self, config, input_ids, input_mask, head_mask, token_type_ids, mc_token_ids, sequence_labels, *args
):
config.num_labels = self.num_labels
model = GPT2ForSequenceClassification(config)
model.to(torch_device)
model.eval()
result = model(input_ids, attention_mask=input_mask, token_type_ids=token_type_ids, labels=sequence_labels)
self.parent.assertEqual(result.logits.shape, (self.batch_size, self.num_labels))
def create_and_check_gpt2_for_token_classification(
self, config, input_ids, input_mask, head_mask, token_type_ids, mc_token_ids, sequence_labels, *args
):
config.num_labels = self.num_labels
model = GPT2ForTokenClassification(config)
model.to(torch_device)
model.eval()
result = model(input_ids, attention_mask=input_mask, token_type_ids=token_type_ids)
self.parent.assertEqual(result.logits.shape, (self.batch_size, self.seq_length, self.num_labels))
def create_and_check_gpt2_weight_initialization(self, config, *args):
model = GPT2Model(config)
model_std = model.config.initializer_range / math.sqrt(2 * model.config.n_layer)
for key in model.state_dict().keys():
if "c_proj" in key and "weight" in key:
self.parent.assertLessEqual(abs(torch.std(model.state_dict()[key]) - model_std), 0.001)
self.parent.assertLessEqual(abs(torch.mean(model.state_dict()[key]) - 0.0), 0.01)
def prepare_config_and_inputs_for_common(self):
config_and_inputs = self.prepare_config_and_inputs()
(
config,
input_ids,
input_mask,
head_mask,
token_type_ids,
mc_token_ids,
sequence_labels,
token_labels,
choice_labels,
) = config_and_inputs
inputs_dict = {
"input_ids": input_ids,
"token_type_ids": token_type_ids,
"head_mask": head_mask,
}
return config, inputs_dict
@require_torch
class GPT2ModelTest(ModelTesterMixin, GenerationTesterMixin, PipelineTesterMixin, unittest.TestCase):
all_model_classes = (
(
GPT2Model,
GPT2LMHeadModel,
GPT2DoubleHeadsModel,
GPT2ForQuestionAnswering,
GPT2ForSequenceClassification,
GPT2ForTokenClassification,
)
if is_torch_available()
else ()
)
all_generative_model_classes = (GPT2LMHeadModel, GPT2DoubleHeadsModel) if is_torch_available() else ()
pipeline_model_mapping = (
{
"feature-extraction": GPT2Model,
"question-answering": GPT2ForQuestionAnswering,
"text-classification": GPT2ForSequenceClassification,
"text-generation": GPT2LMHeadModel,
"token-classification": GPT2ForTokenClassification,
"zero-shot": GPT2ForSequenceClassification,
}
if is_torch_available()
else {}
)
all_parallelizable_model_classes = (GPT2LMHeadModel, GPT2DoubleHeadsModel) if is_torch_available() else ()
fx_compatible = True
test_missing_keys = False
test_model_parallel = True
# special case for DoubleHeads model
def _prepare_for_class(self, inputs_dict, model_class, return_labels=False):
inputs_dict = super()._prepare_for_class(inputs_dict, model_class, return_labels=return_labels)
if return_labels:
if model_class.__name__ == "GPT2DoubleHeadsModel":
inputs_dict["labels"] = torch.zeros(
(self.model_tester.batch_size, self.model_tester.num_choices, self.model_tester.seq_length),
dtype=torch.long,
device=torch_device,
)
inputs_dict["input_ids"] = inputs_dict["labels"]
inputs_dict["token_type_ids"] = inputs_dict["labels"]
inputs_dict["mc_token_ids"] = torch.zeros(
(self.model_tester.batch_size, self.model_tester.num_choices),
dtype=torch.long,
device=torch_device,
)
inputs_dict["mc_labels"] = torch.zeros(
self.model_tester.batch_size, dtype=torch.long, device=torch_device
)
return inputs_dict
def setUp(self):
self.model_tester = GPT2ModelTester(self)
self.config_tester = ConfigTester(self, config_class=GPT2Config, n_embd=37)
def tearDown(self):
super().tearDown()
# clean-up as much as possible GPU memory occupied by PyTorch
gc.collect()
backend_empty_cache(torch_device)
def test_config(self):
self.config_tester.run_common_tests()
def test_gpt2_model(self):
config_and_inputs = self.model_tester.prepare_config_and_inputs()
self.model_tester.create_and_check_gpt2_model(*config_and_inputs)
def test_gpt2_model_past(self):
config_and_inputs = self.model_tester.prepare_config_and_inputs()
self.model_tester.create_and_check_gpt2_model_past(*config_and_inputs)
def test_gpt2_model_att_mask_past(self):
config_and_inputs = self.model_tester.prepare_config_and_inputs()
self.model_tester.create_and_check_gpt2_model_attention_mask_past(*config_and_inputs)
def test_gpt2_model_past_large_inputs(self):
config_and_inputs = self.model_tester.prepare_config_and_inputs()
self.model_tester.create_and_check_gpt2_model_past_large_inputs(*config_and_inputs)
def test_gpt2_lm_head_model(self):
config_and_inputs = self.model_tester.prepare_config_and_inputs()
self.model_tester.create_and_check_lm_head_model(*config_and_inputs)
def test_gpt2_double_lm_head_model(self):
config_and_inputs = self.model_tester.prepare_config_and_inputs()
self.model_tester.create_and_check_double_lm_head_model(*config_and_inputs)
def test_gpt2_question_answering_model(self):
config_and_inputs = self.model_tester.prepare_config_and_inputs()
self.model_tester.create_and_check_gpt2_for_question_answering(*config_and_inputs)
def test_gpt2_sequence_classification_model(self):
config_and_inputs = self.model_tester.prepare_config_and_inputs()
self.model_tester.create_and_check_gpt2_for_sequence_classification(*config_and_inputs)
def test_gpt2_token_classification_model(self):
config_and_inputs = self.model_tester.prepare_config_and_inputs()
self.model_tester.create_and_check_gpt2_for_token_classification(*config_and_inputs)
def test_gpt2_gradient_checkpointing(self):
config_and_inputs = self.model_tester.prepare_config_and_inputs()
self.model_tester.create_and_check_forward_and_backwards(*config_and_inputs, gradient_checkpointing=True)
def test_gpt2_scale_attn_by_inverse_layer_idx(self):
config_and_inputs = self.model_tester.prepare_config_and_inputs(scale_attn_by_inverse_layer_idx=True)
self.model_tester.create_and_check_forward_and_backwards(*config_and_inputs)
def test_gpt2_reorder_and_upcast_attn(self):
config_and_inputs = self.model_tester.prepare_config_and_inputs(reorder_and_upcast_attn=True)
self.model_tester.create_and_check_forward_and_backwards(*config_and_inputs)
def test_gpt2_weight_initialization(self):
config_and_inputs = self.model_tester.prepare_config_and_inputs()
self.model_tester.create_and_check_gpt2_weight_initialization(*config_and_inputs)
@unittest.skip(
reason="This architecure seem to not compute gradients properly when using GC, check: https://github.com/huggingface/transformers/pull/27124"
)
def test_training_gradient_checkpointing(self):
pass
@unittest.skip(
reason="This architecure seem to not compute gradients properly when using GC, check: https://github.com/huggingface/transformers/pull/27124"
)
def test_training_gradient_checkpointing_use_reentrant(self):
pass
@unittest.skip(
reason="This architecure seem to not compute gradients properly when using GC, check: https://github.com/huggingface/transformers/pull/27124"
)
def test_training_gradient_checkpointing_use_reentrant_false(self):
pass
@slow
def test_batch_generation(self):
model = GPT2LMHeadModel.from_pretrained("gpt2")
model.to(torch_device)
tokenizer = GPT2Tokenizer.from_pretrained("gpt2")
tokenizer.padding_side = "left"
# Define PAD Token = EOS Token = 50256
tokenizer.pad_token = tokenizer.eos_token
model.config.pad_token_id = model.config.eos_token_id
# use different length sentences to test batching
sentences = [
"Hello, my dog is a little",
"Today, I",
]
inputs = tokenizer(sentences, return_tensors="pt", padding=True)
input_ids = inputs["input_ids"].to(torch_device)
token_type_ids = torch.cat(
[
input_ids.new_full((input_ids.shape[0], input_ids.shape[1] - 1), 0),
input_ids.new_full((input_ids.shape[0], 1), 500),
],
dim=-1,
)
outputs = model.generate(
input_ids=input_ids,
attention_mask=inputs["attention_mask"].to(torch_device),
)
outputs_tt = model.generate(
input_ids=input_ids,
attention_mask=inputs["attention_mask"].to(torch_device),
token_type_ids=token_type_ids,
)
inputs_non_padded = tokenizer(sentences[0], return_tensors="pt").input_ids.to(torch_device)
output_non_padded = model.generate(input_ids=inputs_non_padded)
num_paddings = inputs_non_padded.shape[-1] - inputs["attention_mask"][-1].long().sum().cpu().item()
inputs_padded = tokenizer(sentences[1], return_tensors="pt").input_ids.to(torch_device)
output_padded = model.generate(input_ids=inputs_padded, max_length=model.config.max_length - num_paddings)
batch_out_sentence = tokenizer.batch_decode(outputs, skip_special_tokens=True)
batch_out_sentence_tt = tokenizer.batch_decode(outputs_tt, skip_special_tokens=True)
non_padded_sentence = tokenizer.decode(output_non_padded[0], skip_special_tokens=True)
padded_sentence = tokenizer.decode(output_padded[0], skip_special_tokens=True)
expected_output_sentence = [
"Hello, my dog is a little bit of a mess. I'm not sure if he's going",
"Today, I'm going to be doing a lot of research on this. I",
]
self.assertListEqual(expected_output_sentence, batch_out_sentence)
self.assertTrue(batch_out_sentence_tt != batch_out_sentence) # token_type_ids should change output
self.assertListEqual(expected_output_sentence, [non_padded_sentence, padded_sentence])
@slow
def test_batch_generation_2heads(self):
model = GPT2DoubleHeadsModel.from_pretrained("gpt2")
model.to(torch_device)
tokenizer = GPT2Tokenizer.from_pretrained("gpt2")
tokenizer.padding_side = "left"
# This tokenizer has no pad token, so we have to set it in some way
# Define PAD Token = EOS Token = 50256
tokenizer.pad_token = tokenizer.eos_token
model.config.pad_token_id = model.config.eos_token_id
# use different length sentences to test batching
sentences = [
"Hello, my dog is a little",
"Today, I",
]
inputs = tokenizer(sentences, return_tensors="pt", padding=True)
input_ids = inputs["input_ids"].to(torch_device)
token_type_ids = torch.cat(
[
input_ids.new_full((input_ids.shape[0], input_ids.shape[1] - 1), 0),
input_ids.new_full((input_ids.shape[0], 1), 500),
],
dim=-1,
)
outputs = model.generate(
input_ids=input_ids,
attention_mask=inputs["attention_mask"].to(torch_device),
)
outputs_tt = model.generate(
input_ids=input_ids,
attention_mask=inputs["attention_mask"].to(torch_device),
token_type_ids=token_type_ids,
)
inputs_non_padded = tokenizer(sentences[0], return_tensors="pt").input_ids.to(torch_device)
output_non_padded = model.generate(input_ids=inputs_non_padded)
num_paddings = inputs_non_padded.shape[-1] - inputs["attention_mask"][-1].long().sum().cpu().item()
inputs_padded = tokenizer(sentences[1], return_tensors="pt").input_ids.to(torch_device)
output_padded = model.generate(input_ids=inputs_padded, max_length=model.config.max_length - num_paddings)
batch_out_sentence = tokenizer.batch_decode(outputs, skip_special_tokens=True)
batch_out_sentence_tt = tokenizer.batch_decode(outputs_tt, skip_special_tokens=True)
non_padded_sentence = tokenizer.decode(output_non_padded[0], skip_special_tokens=True)
padded_sentence = tokenizer.decode(output_padded[0], skip_special_tokens=True)
expected_output_sentence = [
"Hello, my dog is a little bit of a mess. I'm not sure if he's going",
"Today, I'm going to be doing a lot of research on this. I",
]
self.assertListEqual(expected_output_sentence, batch_out_sentence)
self.assertTrue(batch_out_sentence_tt != batch_out_sentence) # token_type_ids should change output
self.assertListEqual(expected_output_sentence, [non_padded_sentence, padded_sentence])
@slow
def test_model_from_pretrained(self):
for model_name in GPT2_PRETRAINED_MODEL_ARCHIVE_LIST[:1]:
model = GPT2Model.from_pretrained(model_name)
self.assertIsNotNone(model)
@require_torch
class GPT2ModelLanguageGenerationTest(unittest.TestCase):
def tearDown(self):
super().tearDown()
# clean-up as much as possible GPU memory occupied by PyTorch
gc.collect()
backend_empty_cache(torch_device)
def _test_lm_generate_gpt2_helper(
self,
gradient_checkpointing=False,
reorder_and_upcast_attn=False,
scale_attn_by_inverse_layer_idx=False,
verify_outputs=True,
):
model = GPT2LMHeadModel.from_pretrained(
"gpt2",
reorder_and_upcast_attn=reorder_and_upcast_attn,
scale_attn_by_inverse_layer_idx=scale_attn_by_inverse_layer_idx,
)
if gradient_checkpointing:
model.gradient_checkpointing_enable()
else:
model.gradient_checkpointing_disable()
model.to(torch_device)
# The dog
input_ids = torch.tensor([[464, 3290]], dtype=torch.long, device=torch_device)
# The dog was found in a field near the intersection of West and West Streets.\n\nThe dog
expected_output_ids = [464, 3290, 373, 1043, 287, 257, 2214, 1474, 262, 16246, 286, 2688, 290, 2688, 27262, 13, 198, 198, 464, 3290,] # fmt: skip
output_ids = model.generate(input_ids, do_sample=False)
if verify_outputs:
self.assertListEqual(output_ids[0].tolist(), expected_output_ids)
@slow
def test_lm_generate_gpt2(self):
self._test_lm_generate_gpt2_helper()
@slow
def test_lm_generate_gpt2_with_gradient_checkpointing(self):
self._test_lm_generate_gpt2_helper(gradient_checkpointing=True)
@slow
def test_lm_generate_gpt2_with_reorder_and_upcast_attn(self):
self._test_lm_generate_gpt2_helper(reorder_and_upcast_attn=True)
@slow
def test_lm_generate_gpt2_with_scale_attn_by_inverse_layer_idx(self):
self._test_lm_generate_gpt2_helper(scale_attn_by_inverse_layer_idx=True, verify_outputs=False)
@slow
def test_gpt2_sample(self):
tokenizer = GPT2Tokenizer.from_pretrained("gpt2")
model = GPT2LMHeadModel.from_pretrained("gpt2")
model.to(torch_device)
torch.manual_seed(0)
tokenized = tokenizer("Today is a nice day and", return_tensors="pt", return_token_type_ids=True)
input_ids = tokenized.input_ids.to(torch_device)
output_ids = model.generate(input_ids, do_sample=True)
output_str = tokenizer.decode(output_ids[0], skip_special_tokens=True)
token_type_ids = tokenized.token_type_ids.to(torch_device)
output_seq = model.generate(input_ids=input_ids, do_sample=True, num_return_sequences=5)
output_seq_tt = model.generate(
input_ids=input_ids, token_type_ids=token_type_ids, do_sample=True, num_return_sequences=5
)
output_seq_strs = tokenizer.batch_decode(output_seq, skip_special_tokens=True)
output_seq_tt_strs = tokenizer.batch_decode(output_seq_tt, skip_special_tokens=True)
EXPECTED_OUTPUT_STR = (
"Today is a nice day and if you don't know anything about the state of play during your holiday"
)
self.assertEqual(output_str, EXPECTED_OUTPUT_STR)
self.assertTrue(
all(output_seq_strs[idx] != output_seq_tt_strs[idx] for idx in range(len(output_seq_tt_strs)))
) # token_type_ids should change output
@slow
def test_gpt2_sample_max_time(self):
tokenizer = GPT2Tokenizer.from_pretrained("gpt2")
model = GPT2LMHeadModel.from_pretrained("gpt2")
model.to(torch_device)
torch.manual_seed(0)
tokenized = tokenizer("Today is a nice day and", return_tensors="pt", return_token_type_ids=True)
input_ids = tokenized.input_ids.to(torch_device)
MAX_TIME = 0.5
start = datetime.datetime.now()
model.generate(input_ids, do_sample=True, max_time=MAX_TIME, max_length=256)
duration = datetime.datetime.now() - start
self.assertGreater(duration, datetime.timedelta(seconds=MAX_TIME))
self.assertLess(duration, datetime.timedelta(seconds=1.5 * MAX_TIME))
start = datetime.datetime.now()
model.generate(input_ids, do_sample=False, max_time=MAX_TIME, max_length=256)
duration = datetime.datetime.now() - start
self.assertGreater(duration, datetime.timedelta(seconds=MAX_TIME))
self.assertLess(duration, datetime.timedelta(seconds=1.5 * MAX_TIME))
start = datetime.datetime.now()
model.generate(input_ids, do_sample=False, num_beams=2, max_time=MAX_TIME, max_length=256)
duration = datetime.datetime.now() - start
self.assertGreater(duration, datetime.timedelta(seconds=MAX_TIME))
self.assertLess(duration, datetime.timedelta(seconds=1.5 * MAX_TIME))
start = datetime.datetime.now()
model.generate(input_ids, do_sample=True, num_beams=2, max_time=MAX_TIME, max_length=256)
duration = datetime.datetime.now() - start
self.assertGreater(duration, datetime.timedelta(seconds=MAX_TIME))
self.assertLess(duration, datetime.timedelta(seconds=1.5 * MAX_TIME))
start = datetime.datetime.now()
model.generate(input_ids, do_sample=False, max_time=None, max_length=256)
duration = datetime.datetime.now() - start
self.assertGreater(duration, datetime.timedelta(seconds=1.5 * MAX_TIME))
@slow
def test_contrastive_search_gpt2(self):
article = (
"DeepMind Technologies is a British artificial intelligence subsidiary of Alphabet Inc. and research "
"laboratory founded in 2010. DeepMind was acquired by Google in 2014. The company is based"
)
gpt2_tokenizer = GPT2Tokenizer.from_pretrained("gpt2-large")
gpt2_model = GPT2LMHeadModel.from_pretrained("gpt2-large").to(torch_device)
input_ids = gpt2_tokenizer(article, return_tensors="pt").input_ids.to(torch_device)
outputs = gpt2_model.generate(input_ids, penalty_alpha=0.6, top_k=4, max_length=256)
generated_text = gpt2_tokenizer.batch_decode(outputs, skip_special_tokens=True)
self.assertListEqual(
generated_text,
[
"DeepMind Technologies is a British artificial intelligence subsidiary of Alphabet Inc. and research "
"laboratory founded in 2010. DeepMind was acquired by Google in 2014. The company is based in London, "
"United Kingdom\n\nGoogle has a lot of data on its users and uses it to improve its products, such as "
"Google Now, which helps users find the information they're looking for on the web. But the company "
"is not the only one to collect data on its users. Facebook, for example, has its own facial "
"recognition technology, as well as a database of millions of photos that it uses to personalize its "
"News Feed.\n\nFacebook's use of data is a hot topic in the tech industry, with privacy advocates "
"concerned about the company's ability to keep users' information private. In a blog post last "
'year, Facebook CEO Mark Zuckerberg said his company would "do our best to be transparent about our '
'data use and how we use it."\n\n"We have made it clear that we do not sell or share your data with '
'third parties," Zuckerberg wrote. "If you have questions or concerns, please reach out to us at '
'[email protected]."\n\nGoogle declined to comment on the privacy implications of its use of data, '
"but said in a statement to The Associated Press that"
],
)
| transformers/tests/models/gpt2/test_modeling_gpt2.py/0 | {
"file_path": "transformers/tests/models/gpt2/test_modeling_gpt2.py",
"repo_id": "transformers",
"token_count": 16910
} | 354 |
# coding=utf-8
# Copyright 2021 The HuggingFace Inc. team. All rights reserved.
#
# Licensed under the Apache License, Version 2.0 (the "License");
# you may not use this file except in compliance with the License.
# You may obtain a copy of the License at
#
# http://www.apache.org/licenses/LICENSE-2.0
#
# Unless required by applicable law or agreed to in writing, software
# distributed under the License is distributed on an "AS IS" BASIS,
# WITHOUT WARRANTIES OR CONDITIONS OF ANY KIND, either express or implied.
# See the License for the specific language governing permissions and
# limitations under the License.
from __future__ import annotations
import copy
import inspect
import math
import os
import tempfile
import unittest
import numpy as np
import pytest
from transformers import is_tf_available
from transformers.testing_utils import is_pt_tf_cross_test, require_soundfile, require_tf, slow
from ...test_configuration_common import ConfigTester
from ...test_modeling_tf_common import TFModelTesterMixin, ids_tensor
from ...test_pipeline_mixin import PipelineTesterMixin
if is_tf_available():
import tensorflow as tf
from transformers import HubertConfig, TFHubertForCTC, TFHubertModel, Wav2Vec2Processor
from transformers.models.hubert.modeling_tf_hubert import _compute_mask_indices
@require_tf
class TFHubertModelTester:
def __init__(
self,
parent,
batch_size=13,
seq_length=1024,
is_training=False,
hidden_size=16,
feat_extract_norm="group",
feat_extract_dropout=0.0,
feat_extract_activation="gelu",
conv_dim=(32, 32, 32),
conv_stride=(4, 4, 4),
conv_kernel=(8, 8, 8),
conv_bias=False,
num_conv_pos_embeddings=16,
num_conv_pos_embedding_groups=2,
num_hidden_layers=2,
num_attention_heads=2,
hidden_dropout_prob=0.1, # this is most likely not correctly set yet
intermediate_size=20,
layer_norm_eps=1e-5,
hidden_act="gelu",
initializer_range=0.02,
vocab_size=32,
do_stable_layer_norm=False,
scope=None,
):
self.parent = parent
self.batch_size = batch_size
self.seq_length = seq_length
self.is_training = is_training
self.hidden_size = hidden_size
self.feat_extract_norm = feat_extract_norm
self.feat_extract_dropout = feat_extract_dropout
self.feat_extract_activation = feat_extract_activation
self.conv_dim = conv_dim
self.conv_stride = conv_stride
self.conv_kernel = conv_kernel
self.conv_bias = conv_bias
self.num_conv_pos_embeddings = num_conv_pos_embeddings
self.num_conv_pos_embedding_groups = num_conv_pos_embedding_groups
self.num_hidden_layers = num_hidden_layers
self.num_attention_heads = num_attention_heads
self.hidden_dropout_prob = hidden_dropout_prob
self.intermediate_size = intermediate_size
self.layer_norm_eps = layer_norm_eps
self.hidden_act = hidden_act
self.initializer_range = initializer_range
self.vocab_size = vocab_size
self.do_stable_layer_norm = do_stable_layer_norm
self.scope = scope
output_seq_length = self.seq_length
for kernel, stride in zip(self.conv_kernel, self.conv_stride):
output_seq_length = (output_seq_length - (kernel - 1)) / stride
self.output_seq_length = int(math.ceil(output_seq_length))
self.encoder_seq_length = self.output_seq_length
def prepare_config_and_inputs(self):
input_values = tf.cast(ids_tensor([self.batch_size, self.seq_length], 32768), tf.float32) / 32768.0
attention_mask = tf.ones_like(input_values)
config = HubertConfig(
hidden_size=self.hidden_size,
feat_extract_norm=self.feat_extract_norm,
feat_extract_dropout=self.feat_extract_dropout,
feat_extract_activation=self.feat_extract_activation,
conv_dim=self.conv_dim,
conv_stride=self.conv_stride,
conv_kernel=self.conv_kernel,
conv_bias=self.conv_bias,
num_conv_pos_embeddings=self.num_conv_pos_embeddings,
num_conv_pos_embedding_groups=self.num_conv_pos_embedding_groups,
num_hidden_layers=self.num_hidden_layers,
num_attention_heads=self.num_attention_heads,
hidden_dropout_prob=self.hidden_dropout_prob,
intermediate_size=self.intermediate_size,
layer_norm_eps=self.layer_norm_eps,
hidden_act=self.hidden_act,
initializer_range=self.initializer_range,
vocab_size=self.vocab_size,
do_stable_layer_norm=self.do_stable_layer_norm,
)
return config, input_values, attention_mask
def create_and_check_model(self, config, input_values, attention_mask):
model = TFHubertModel(config)
result = model(input_values, attention_mask=attention_mask)
self.parent.assertEqual(
result.last_hidden_state.shape, (self.batch_size, self.output_seq_length, self.hidden_size)
)
def create_and_check_batch_inference(self, config, input_values, *args):
# test does not pass for models making use of `group_norm`
# check: https://github.com/pytorch/fairseq/issues/3227
config.layerdrop = 0.0
model = TFHubertModel(config)
input_values = input_values[:3]
attention_mask = tf.ones_like(input_values)
input_lengths = tf.constant([input_values.shape[-1] // i for i in [4, 2, 1]])
length_mask = tf.sequence_mask(input_lengths, dtype=tf.float32)
# convert values that are over input_lengths to padding
input_values = input_values * length_mask
attention_mask = attention_mask * length_mask
batch_outputs = model(input_values, attention_mask=attention_mask, training=False).last_hidden_state
for i in range(input_values.shape[0]):
input_slice = input_values[i : i + 1, : input_lengths[i]]
output = model(input_slice, training=False).last_hidden_state
batch_output = batch_outputs[i : i + 1, : output.shape[1]]
self.parent.assertTrue(np.allclose(output, batch_output, atol=1e-3))
def check_ctc_loss(self, config, input_values, *args):
model = TFHubertForCTC(config)
input_values = input_values[:3]
attention_mask = tf.ones_like(input_values)
input_lengths = tf.constant([input_values.shape[-1] // i for i in [4, 2, 1]])
max_length_labels = model.hubert._get_feat_extract_output_lengths(input_lengths)
labels = ids_tensor((input_values.shape[0], min(max_length_labels) - 1), model.config.vocab_size)
length_mask = tf.sequence_mask(input_lengths, dtype=tf.float32)
# convert values that are over input_lengths to padding
input_values = input_values * length_mask
attention_mask = attention_mask * length_mask
model.config.ctc_loss_reduction = "sum"
sum_loss = model(input_values, attention_mask=attention_mask, labels=labels).loss
model.config.ctc_loss_reduction = "mean"
mean_loss = model(input_values, attention_mask=attention_mask, labels=labels).loss
self.parent.assertTrue(abs(labels.shape[0] * mean_loss - sum_loss) < 1e-2)
def check_training(self, config, input_values, *args):
model = TFHubertForCTC(config)
# freeze feature encoder
model.freeze_feature_encoder()
input_values = input_values[:3]
input_lengths = tf.constant([input_values.shape[-1] // i for i in [4, 2, 1]])
max_length_labels = model.hubert._get_feat_extract_output_lengths(input_lengths)
labels = ids_tensor((input_values.shape[0], max(max_length_labels) - 2), model.config.vocab_size)
length_mask = tf.sequence_mask(input_lengths, dtype=tf.float32)
input_values = input_values * length_mask
pad_size = max(max_length_labels) - labels.shape[1]
labels = tf.pad(labels, ((0, 0), (0, pad_size)), constant_values=-100)
loss = model(input_values, labels=labels, training=True).loss
self.parent.assertFalse(tf.math.is_inf(loss))
def check_labels_out_of_vocab(self, config, input_values, *args):
model = TFHubertForCTC(config)
input_lengths = tf.constant([input_values.shape[-1] // i for i in [4, 2, 1]])
max_length_labels = model.hubert._get_feat_extract_output_lengths(input_lengths)
labels = ids_tensor((input_values.shape[0], min(max_length_labels) - 1), model.config.vocab_size + 100)
with pytest.raises(ValueError):
model(input_values, labels=labels)
def prepare_config_and_inputs_for_common(self):
config, input_values, attention_mask = self.prepare_config_and_inputs()
inputs_dict = {"input_values": input_values, "attention_mask": attention_mask}
return config, inputs_dict
@require_tf
class TFHubertModelTest(TFModelTesterMixin, PipelineTesterMixin, unittest.TestCase):
all_model_classes = (TFHubertModel, TFHubertForCTC) if is_tf_available() else ()
pipeline_model_mapping = {"feature-extraction": TFHubertModel} if is_tf_available() else {}
test_resize_embeddings = False
test_head_masking = False
test_onnx = False
def setUp(self):
self.model_tester = TFHubertModelTester(self)
self.config_tester = ConfigTester(self, config_class=HubertConfig, hidden_size=37)
def test_config(self):
self.config_tester.run_common_tests()
# overwrite because input_values != input_ids
def test_forward_signature(self):
config, _ = self.model_tester.prepare_config_and_inputs_for_common()
for model_class in self.all_model_classes:
model = model_class(config)
signature = inspect.signature(model.call)
# signature.parameters is an OrderedDict => so arg_names order is deterministic
arg_names = [*signature.parameters.keys()]
expected_arg_names = ["input_values"]
self.assertListEqual(arg_names[:1], expected_arg_names)
# overwrite because input_values != input_ids
def test_keyword_and_dict_args(self):
config, inputs_dict = self.model_tester.prepare_config_and_inputs_for_common()
for model_class in self.all_model_classes:
model = model_class(config)
inputs = self._prepare_for_class(inputs_dict, model_class)
outputs_dict = model(inputs)
inputs_keywords = copy.deepcopy(self._prepare_for_class(inputs_dict, model_class))
input_values = inputs_keywords.pop("input_values", None)
outputs_keywords = model(input_values, **inputs_keywords)
output_dict = outputs_dict[0].numpy()
output_keywords = outputs_keywords[0].numpy()
self.assertLess(np.sum(np.abs(output_dict - output_keywords)), 1e-6)
def test_model(self):
config_and_inputs = self.model_tester.prepare_config_and_inputs()
self.model_tester.create_and_check_model(*config_and_inputs)
def test_hidden_states_output(self):
config, inputs_dict = self.model_tester.prepare_config_and_inputs_for_common()
def check_hidden_states_output(config, inputs_dict, model_class):
model = model_class(config)
outputs = model(self._prepare_for_class(inputs_dict, model_class))
expected_num_layers = getattr(
self.model_tester, "expected_num_hidden_layers", self.model_tester.num_hidden_layers + 1
)
hidden_states = outputs.hidden_states
self.assertEqual(config.output_attentions, False)
self.assertEqual(len(hidden_states), expected_num_layers)
self.assertListEqual(
list(hidden_states[0].shape[-2:]),
[self.model_tester.output_seq_length, self.model_tester.hidden_size],
)
for model_class in self.all_model_classes:
inputs_dict["output_hidden_states"] = True
check_hidden_states_output(config, inputs_dict, model_class)
del inputs_dict["output_hidden_states"]
config.output_hidden_states = True
check_hidden_states_output(config, inputs_dict, model_class)
def test_ctc_loss_inference(self):
config_and_inputs = self.model_tester.prepare_config_and_inputs()
self.model_tester.check_ctc_loss(*config_and_inputs)
def test_train(self):
config_and_inputs = self.model_tester.prepare_config_and_inputs()
self.model_tester.check_training(*config_and_inputs)
def test_labels_out_of_vocab(self):
config_and_inputs = self.model_tester.prepare_config_and_inputs()
self.model_tester.check_labels_out_of_vocab(*config_and_inputs)
@unittest.skip(reason="Hubert has no input embeddings")
def test_inputs_embeds(self):
pass
@unittest.skip(reason="Hubert has no tokens embeddings")
def test_resize_tokens_embeddings(self):
pass
@unittest.skip(reason="Hubert has no input embeddings")
def test_model_common_attributes(self):
pass
@slow
def test_model_from_pretrained(self):
model = TFHubertModel.from_pretrained("facebook/hubert-base-ls960")
self.assertIsNotNone(model)
@unittest.skip(reason="Fix me! Hubert hits OOM errors when loss is computed on full batch")
def test_dataset_conversion(self):
# TODO: (Amy) - check whether skipping CTC model resolves this issue and possible resolutions for CTC
pass
@unittest.skip(reason="Fix me! Hubert hits OOM errors when loss is computed on full batch")
def test_keras_fit(self):
# TODO: (Amy) - check whether skipping CTC model resolves this issue and possible resolutions for CTC
pass
@is_pt_tf_cross_test
def test_pt_tf_model_equivalence(self, allow_missing_keys=False):
# We override the base test here to skip loss calculation for Hubert models because the loss is massive with
# the default labels and frequently overflows to inf or exceeds numerical tolerances between TF/PT
import torch
import transformers
for model_class in self.all_model_classes:
config, inputs_dict = self.model_tester.prepare_config_and_inputs_for_common()
# Output all for aggressive testing
config.output_hidden_states = True
config.output_attentions = self.has_attentions
# Make sure no sequence has all zeros as attention mask, otherwise some tests fail due to the inconsistency
# of the usage `1e-4`, `1e-9`, `1e-30`, `-inf`.
# TODO: Use a uniform value for all models, make sure all tests pass without this processing, and remove it.
self._make_attention_mask_non_null(inputs_dict)
pt_model_class_name = model_class.__name__[2:] # Skip the "TF" at the beginning
pt_model_class = getattr(transformers, pt_model_class_name)
tf_model = model_class(config)
pt_model = pt_model_class(config)
tf_inputs_dict = self._prepare_for_class(inputs_dict, model_class)
# Check we can load pt model in tf and vice-versa with model => model functions
tf_model = transformers.load_pytorch_model_in_tf2_model(
tf_model, pt_model, tf_inputs=tf_inputs_dict, allow_missing_keys=allow_missing_keys
)
pt_model = transformers.load_tf2_model_in_pytorch_model(
pt_model, tf_model, allow_missing_keys=allow_missing_keys
)
# Original test: check without `labels`
self.check_pt_tf_models(tf_model, pt_model, tf_inputs_dict)
# Check we can load pt model in tf and vice-versa with checkpoint => model functions
with tempfile.TemporaryDirectory() as tmpdirname:
pt_checkpoint_path = os.path.join(tmpdirname, "pt_model.bin")
torch.save(pt_model.state_dict(), pt_checkpoint_path)
tf_model = transformers.load_pytorch_checkpoint_in_tf2_model(
tf_model, pt_checkpoint_path, allow_missing_keys=allow_missing_keys
)
tf_checkpoint_path = os.path.join(tmpdirname, "tf_model.h5")
tf_model.save_weights(tf_checkpoint_path)
pt_model = transformers.load_tf2_checkpoint_in_pytorch_model(
pt_model, tf_checkpoint_path, allow_missing_keys=allow_missing_keys
)
# Original test: check without `labels`
self.check_pt_tf_models(tf_model, pt_model, tf_inputs_dict)
@require_tf
class TFHubertRobustModelTest(TFModelTesterMixin, unittest.TestCase):
all_model_classes = (TFHubertModel, TFHubertForCTC) if is_tf_available() else ()
test_resize_embeddings = False
test_head_masking = False
test_onnx = False
def setUp(self):
self.model_tester = TFHubertModelTester(
self,
conv_stride=(3, 3, 3),
feat_extract_norm="layer",
do_stable_layer_norm=True,
scope="robust",
)
self.config_tester = ConfigTester(self, config_class=HubertConfig, hidden_size=37)
# overwrite because input_values != input_ids
def test_forward_signature(self):
config, _ = self.model_tester.prepare_config_and_inputs_for_common()
for model_class in self.all_model_classes:
model = model_class(config)
signature = inspect.signature(model.call)
# signature.parameters is an OrderedDict => so arg_names order is deterministic
arg_names = [*signature.parameters.keys()]
expected_arg_names = ["input_values"]
self.assertListEqual(arg_names[:1], expected_arg_names)
# overwrite because input_values != input_ids
def test_keyword_and_dict_args(self):
config, inputs_dict = self.model_tester.prepare_config_and_inputs_for_common()
for model_class in self.all_model_classes:
model = model_class(config)
inputs = self._prepare_for_class(inputs_dict, model_class)
outputs_dict = model(inputs)
inputs_keywords = copy.deepcopy(self._prepare_for_class(inputs_dict, model_class))
input_values = inputs_keywords.pop("input_values", None)
outputs_keywords = model(input_values, **inputs_keywords)
output_dict = outputs_dict[0].numpy()
output_keywords = outputs_keywords[0].numpy()
self.assertLess(np.sum(np.abs(output_dict - output_keywords)), 1e-6)
def test_config(self):
self.config_tester.run_common_tests()
def test_model(self):
config_and_inputs = self.model_tester.prepare_config_and_inputs()
self.model_tester.create_and_check_model(*config_and_inputs)
def test_hidden_states_output(self):
config, inputs_dict = self.model_tester.prepare_config_and_inputs_for_common()
def check_hidden_states_output(config, inputs_dict, model_class):
model = model_class(config)
outputs = model(self._prepare_for_class(inputs_dict, model_class))
expected_num_layers = getattr(
self.model_tester, "expected_num_hidden_layers", self.model_tester.num_hidden_layers + 1
)
hidden_states = outputs.hidden_states
self.assertEqual(config.output_attentions, False)
self.assertEqual(len(hidden_states), expected_num_layers)
self.assertListEqual(
list(hidden_states[0].shape[-2:]),
[self.model_tester.output_seq_length, self.model_tester.hidden_size],
)
for model_class in self.all_model_classes:
inputs_dict["output_hidden_states"] = True
check_hidden_states_output(config, inputs_dict, model_class)
del inputs_dict["output_hidden_states"]
config.output_hidden_states = True
check_hidden_states_output(config, inputs_dict, model_class)
def test_batched_inference(self):
config_and_inputs = self.model_tester.prepare_config_and_inputs()
self.model_tester.create_and_check_batch_inference(*config_and_inputs)
def test_ctc_loss_inference(self):
config_and_inputs = self.model_tester.prepare_config_and_inputs()
self.model_tester.check_ctc_loss(*config_and_inputs)
def test_train(self):
config_and_inputs = self.model_tester.prepare_config_and_inputs()
self.model_tester.check_training(*config_and_inputs)
def test_labels_out_of_vocab(self):
config_and_inputs = self.model_tester.prepare_config_and_inputs()
self.model_tester.check_labels_out_of_vocab(*config_and_inputs)
@unittest.skip(reason="Hubert has no input embeddings")
def test_inputs_embeds(self):
pass
@unittest.skip(reason="Hubert has no tokens embeddings")
def test_resize_tokens_embeddings(self):
pass
@unittest.skip(reason="Hubert has no input embeddings or get_input_embeddings method")
def test_model_common_attributes(self):
pass
@slow
def test_model_from_pretrained(self):
model = TFHubertModel.from_pretrained("facebook/hubert-large-ls960-ft")
self.assertIsNotNone(model)
@unittest.skip(reason="Fix me! Hubert hits OOM errors when loss is computed on full batch")
def test_dataset_conversion(self):
# TODO: (Amy) - check whether skipping CTC model resolves this issue and possible resolutions for CTC
pass
@unittest.skip(reason="Fix me! Hubert hits OOM errors when loss is computed on full batch")
def test_keras_fit(self):
# TODO: (Amy) - check whether skipping CTC model resolves this issue and possible resolutions for CTC
pass
@is_pt_tf_cross_test
def test_pt_tf_model_equivalence(self, allow_missing_keys=False):
# We override the base test here to skip loss calculation for Hubert models because the loss is massive with
# the default labels and frequently overflows to inf or exceeds numerical tolerances between TF/PT
import torch
import transformers
for model_class in self.all_model_classes:
config, inputs_dict = self.model_tester.prepare_config_and_inputs_for_common()
# Output all for aggressive testing
config.output_hidden_states = True
config.output_attentions = self.has_attentions
# Make sure no sequence has all zeros as attention mask, otherwise some tests fail due to the inconsistency
# of the usage `1e-4`, `1e-9`, `1e-30`, `-inf`.
# TODO: Use a uniform value for all models, make sure all tests pass without this processing, and remove it.
self._make_attention_mask_non_null(inputs_dict)
pt_model_class_name = model_class.__name__[2:] # Skip the "TF" at the beginning
pt_model_class = getattr(transformers, pt_model_class_name)
tf_model = model_class(config)
pt_model = pt_model_class(config)
tf_inputs_dict = self._prepare_for_class(inputs_dict, model_class)
# Check we can load pt model in tf and vice-versa with model => model functions
tf_model = transformers.load_pytorch_model_in_tf2_model(
tf_model, pt_model, tf_inputs=tf_inputs_dict, allow_missing_keys=allow_missing_keys
)
pt_model = transformers.load_tf2_model_in_pytorch_model(
pt_model, tf_model, allow_missing_keys=allow_missing_keys
)
# Original test: check without `labels`
self.check_pt_tf_models(tf_model, pt_model, tf_inputs_dict)
# Check we can load pt model in tf and vice-versa with checkpoint => model functions
with tempfile.TemporaryDirectory() as tmpdirname:
pt_checkpoint_path = os.path.join(tmpdirname, "pt_model.bin")
torch.save(pt_model.state_dict(), pt_checkpoint_path)
tf_model = transformers.load_pytorch_checkpoint_in_tf2_model(
tf_model, pt_checkpoint_path, allow_missing_keys=allow_missing_keys
)
tf_checkpoint_path = os.path.join(tmpdirname, "tf_model.h5")
tf_model.save_weights(tf_checkpoint_path)
pt_model = transformers.load_tf2_checkpoint_in_pytorch_model(
pt_model, tf_checkpoint_path, allow_missing_keys=allow_missing_keys
)
# Original test: check without `labels`
self.check_pt_tf_models(tf_model, pt_model, tf_inputs_dict)
@require_tf
class TFHubertUtilsTest(unittest.TestCase):
def test_compute_mask_indices(self):
batch_size = 4
sequence_length = 60
mask_prob = 0.5
mask_length = 1
mask = _compute_mask_indices((batch_size, sequence_length), mask_prob, mask_length)
self.assertListEqual(
tf.reduce_sum(mask, -1).numpy().tolist(), [mask_prob * sequence_length for _ in range(batch_size)]
)
def test_compute_mask_indices_overlap(self):
batch_size = 4
sequence_length = 80
mask_prob = 0.5
mask_length = 4
mask = _compute_mask_indices((batch_size, sequence_length), mask_prob, mask_length)
# because of overlap mask don't have to add up exactly to `mask_prob * sequence_length`, but have to be smaller or equal
for batch_sum in tf.reduce_sum(mask, -1):
self.assertTrue(int(batch_sum) <= mask_prob * sequence_length)
@require_tf
@slow
@require_soundfile
class TFHubertModelIntegrationTest(unittest.TestCase):
def _load_datasamples(self, num_samples):
from datasets import load_dataset
ds = load_dataset("hf-internal-testing/librispeech_asr_dummy", "clean", split="validation")
# automatic decoding with librispeech
speech_samples = ds.sort("id").filter(
lambda x: x["id"] in [f"1272-141231-000{i}" for i in range(num_samples)]
)[:num_samples]["audio"]
return [x["array"] for x in speech_samples]
def test_inference_ctc_normal(self):
model = TFHubertForCTC.from_pretrained("facebook/hubert-large-ls960-ft")
processor = Wav2Vec2Processor.from_pretrained("facebook/hubert-large-ls960-ft", do_lower_case=True)
input_speech = self._load_datasamples(1)
input_values = processor(input_speech, return_tensors="tf", sampling_rate=16000).input_values
logits = model(input_values).logits
predicted_ids = tf.argmax(logits, axis=-1)
predicted_trans = processor.batch_decode(predicted_ids)
EXPECTED_TRANSCRIPTIONS = ["a man said to the universe sir i exist"]
self.assertListEqual(predicted_trans, EXPECTED_TRANSCRIPTIONS)
def test_inference_ctc_normal_batched(self):
model = TFHubertForCTC.from_pretrained("facebook/hubert-large-ls960-ft")
processor = Wav2Vec2Processor.from_pretrained("facebook/hubert-large-ls960-ft", do_lower_case=True)
input_speech = self._load_datasamples(2)
input_values = processor(input_speech, return_tensors="tf", padding=True, sampling_rate=16000).input_values
logits = model(input_values).logits
predicted_ids = tf.argmax(logits, axis=-1)
predicted_trans = processor.batch_decode(predicted_ids)
EXPECTED_TRANSCRIPTIONS = [
"a man said to the universe sir i exist",
"sweat covered brion's body trickling into the tight loin cloth that was the only garment he wore",
]
self.assertListEqual(predicted_trans, EXPECTED_TRANSCRIPTIONS)
def test_inference_ctc_robust_batched(self):
model = TFHubertForCTC.from_pretrained("facebook/hubert-large-ls960-ft")
processor = Wav2Vec2Processor.from_pretrained("facebook/hubert-large-ls960-ft", do_lower_case=True)
input_speech = self._load_datasamples(4)
inputs = processor(input_speech, return_tensors="tf", padding=True, sampling_rate=16000)
input_values = inputs.input_values
attention_mask = inputs.attention_mask
logits = model(input_values, attention_mask=attention_mask).logits
predicted_ids = tf.argmax(logits, axis=-1)
predicted_trans = processor.batch_decode(predicted_ids)
EXPECTED_TRANSCRIPTIONS = [
"a man said to the universe sir i exist",
"sweat covered brion's body trickling into the tight loin cloth that was the only garment he wore",
"the cut on his chest still dripping blood the ache of his overstrained eyes even the soaring arena around"
" him with the thousands of spectators were trivialities not worth thinking about",
"his instant of panic was followed by a small sharp blow high on his chest",
]
self.assertListEqual(predicted_trans, EXPECTED_TRANSCRIPTIONS)
| transformers/tests/models/hubert/test_modeling_tf_hubert.py/0 | {
"file_path": "transformers/tests/models/hubert/test_modeling_tf_hubert.py",
"repo_id": "transformers",
"token_count": 12352
} | 355 |
# coding=utf-8
# Copyright 2022 The HuggingFace Team. All rights reserved.
#
# Licensed under the Apache License, Version 2.0 (the "License");
# you may not use this file except in compliance with the License.
# You may obtain a copy of the License at
#
# http://www.apache.org/licenses/LICENSE-2.0
#
# Unless required by applicable law or agreed to in writing, software
# distributed under the License is distributed on an "AS IS" BASIS,
# WITHOUT WARRANTIES OR CONDITIONS OF ANY KIND, either express or implied.
# See the License for the specific language governing permissions and
# limitations under the License.
import unittest
from unittest import skip
from transformers import is_torch_available
from transformers.testing_utils import (
require_torch,
require_torch_accelerator,
require_torch_fp16,
slow,
torch_device,
)
from transformers.trainer_utils import set_seed
if is_torch_available():
import torch
from transformers import JukeboxModel, JukeboxPrior, JukeboxTokenizer
@require_torch
class Jukebox1bModelTester(unittest.TestCase):
all_model_classes = (JukeboxModel,) if is_torch_available() else ()
model_id = "openai/jukebox-1b-lyrics"
metas = {
"artist": "Zac Brown Band",
"genres": "Country",
"lyrics": """I met a traveller from an antique land,
Who said "Two vast and trunkless legs of stone
Stand in the desert. . . . Near them, on the sand,
Half sunk a shattered visage lies, whose frown,
And wrinkled lip, and sneer of cold command,
Tell that its sculptor well those passions read
Which yet survive, stamped on these lifeless things,
The hand that mocked them, and the heart that fed;
And on the pedestal, these words appear:
My name is Ozymandias, King of Kings;
Look on my Works, ye Mighty, and despair!
Nothing beside remains. Round the decay
Of that colossal Wreck, boundless and bare
The lone and level sands stretch far away
""",
}
# fmt: off
EXPECTED_OUTPUT_2 = [
1864, 1536, 1213, 1870, 1357, 1536, 519, 880, 1323, 789, 1082, 534,
1000, 1445, 1105, 1130, 967, 515, 1434, 1620, 534, 1495, 283, 1445,
333, 1307, 539, 1631, 1528, 375, 1434, 673, 627, 710, 778, 1883,
1405, 1276, 1455, 1228
]
EXPECTED_OUTPUT_2_PT_2 = [
1489, 653, 653, 653, 653, 653, 653, 653, 653, 653, 653, 653,
653, 653, 653, 653, 653, 653, 653, 653, 653, 653, 653, 653,
653, 653, 653, 653, 653, 653, 653, 653, 653, 653, 653, 653,
653, 653, 653, 653
]
EXPECTED_OUTPUT_1 = [
1125, 1751, 697, 1776, 1141, 1476, 391, 697, 1125, 684, 867, 416,
844, 1372, 1274, 717, 1274, 844, 1299, 1419, 697, 1370, 317, 1125,
191, 1440, 1370, 1440, 1370, 282, 1621, 1370, 368, 349, 867, 1872,
1262, 869, 1728, 747
]
EXPECTED_OUTPUT_1_PT_2 = [
416, 416, 1125, 1125, 416, 416, 416, 416, 416, 416, 416, 416,
416, 416, 416, 416, 416, 416, 416, 416, 416, 416, 416, 416,
416, 416, 416, 416, 416, 416, 416, 416, 416, 416, 416, 416,
416, 416, 416, 416
]
EXPECTED_OUTPUT_0 = [
1755, 842, 307, 1843, 1022, 1395, 234, 1554, 806, 739, 1022, 442,
616, 556, 268, 1499, 933, 457, 1440, 1837, 755, 985, 308, 902,
293, 1443, 1671, 1141, 1533, 555, 1562, 1061, 287, 417, 1022, 2008,
1186, 1015, 1777, 268
]
EXPECTED_OUTPUT_0_PT_2 = [
854, 842, 1353, 114, 1353, 842, 185, 842, 185, 114, 591, 842,
185, 417, 185, 842, 307, 842, 591, 842, 185, 842, 307, 842,
591, 842, 1353, 842, 185, 842, 591, 842, 591, 114, 591, 842,
185, 842, 591, 89
]
EXPECTED_Y_COND = [1058304, 0, 786432, 7169, 507, 76, 27, 40, 30, 76]
EXPECTED_PRIMED_0 = [
390, 1160, 1002, 1907, 1788, 1788, 1788, 1907, 1002, 1002, 1854, 1002,
1002, 1002, 1002, 1002, 1002, 1160, 1160, 1606, 596, 596, 1160, 1002,
1516, 596, 1002, 1002, 1002, 1907, 1788, 1788, 1788, 1854, 1788, 1907,
1907, 1788, 596, 1626
]
EXPECTED_PRIMED_1 = [
1236, 1668, 1484, 1920, 1848, 1409, 139, 864, 1828, 1272, 1599, 824,
1672, 139, 555, 1484, 824, 1920, 555, 596, 1579, 1599, 1231, 1599,
1637, 1407, 212, 824, 1599, 116, 1433, 824, 258, 1599, 1433, 1895,
1063, 1433, 1433, 1599
]
EXPECTED_PRIMED_2 = [
1684, 1873, 1119, 1189, 395, 611, 1901, 972, 890, 1337, 1392, 1927,
96, 972, 672, 780, 1119, 890, 158, 771, 1073, 1927, 353, 1331,
1269, 1459, 1333, 1645, 812, 1577, 1337, 606, 353, 981, 1466, 619,
197, 391, 302, 1930
]
EXPECTED_VQVAE_ENCODE = [
390, 1160, 1002, 1907, 1788, 1788, 1788, 1907, 1002, 1002, 1854, 1002,
1002, 1002, 1002, 1002, 1002, 1160, 1160, 1606, 596, 596, 1160, 1002,
1516, 596, 1002, 1002, 1002, 1907, 1788, 1788, 1788, 1854, 1788, 1907,
1907, 1788, 596, 1626
]
EXPECTED_VQVAE_DECODE = [
-0.0492, -0.0524, -0.0565, -0.0640, -0.0686, -0.0684, -0.0677, -0.0664,
-0.0605, -0.0490, -0.0330, -0.0168, -0.0083, -0.0075, -0.0051, 0.0025,
0.0136, 0.0261, 0.0386, 0.0497, 0.0580, 0.0599, 0.0583, 0.0614,
0.0740, 0.0889, 0.1023, 0.1162, 0.1211, 0.1212, 0.1251, 0.1336,
0.1502, 0.1686, 0.1883, 0.2148, 0.2363, 0.2458, 0.2507, 0.2531
]
EXPECTED_AUDIO_COND = [
0.0256, -0.0544, 0.1600, -0.0032, 0.1066, 0.0825, -0.0013, 0.3440,
0.0210, 0.0412, -0.1777, -0.0892, -0.0164, 0.0285, -0.0613, -0.0617,
-0.0137, -0.0201, -0.0175, 0.0215, -0.0627, 0.0520, -0.0730, 0.0970,
-0.0100, 0.0442, -0.0586, 0.0207, -0.0015, -0.0082
]
EXPECTED_META_COND = [
0.0415, 0.0877, 0.0022, -0.0055, 0.0751, 0.0334, 0.0324, -0.0068,
0.0011, 0.0017, -0.0676, 0.0655, -0.0143, 0.0399, 0.0303, 0.0743,
-0.0168, -0.0394, -0.1113, 0.0124, 0.0442, 0.0267, -0.0003, -0.1536,
-0.0116, -0.1837, -0.0180, -0.1026, -0.0777, -0.0456
]
EXPECTED_LYRIC_COND = [
76, 27, 40, 30, 76, 46, 44, 47, 40, 37, 38, 31, 45, 45, 76, 38, 31, 33,
45, 76, 41, 32, 76, 45, 46, 41, 40, 31, 78, 76
]
# fmt: on
def prepare_inputs(self):
tokenizer = JukeboxTokenizer.from_pretrained(self.model_id)
tokens = tokenizer(**self.metas)["input_ids"]
return tokens
@slow
def test_sampling(self):
model = JukeboxModel.from_pretrained(self.model_id, min_duration=0).eval()
labels = self.prepare_inputs()
set_seed(0)
zs = [torch.zeros(1, 0, dtype=torch.long).cpu() for _ in range(3)]
zs = model._sample(zs, labels, [0], sample_length=40 * model.priors[0].raw_to_tokens, save_results=False)
self.assertIn(zs[0][0].detach().cpu().tolist(), [self.EXPECTED_OUTPUT_2, self.EXPECTED_OUTPUT_2_PT_2])
set_seed(0)
zs = model._sample(zs, labels, [1], sample_length=40 * model.priors[1].raw_to_tokens, save_results=False)
self.assertIn(zs[1][0].detach().cpu().tolist(), [self.EXPECTED_OUTPUT_1, self.EXPECTED_OUTPUT_1_PT_2])
set_seed(0)
zs = model._sample(zs, labels, [2], sample_length=40 * model.priors[2].raw_to_tokens, save_results=False)
self.assertIn(zs[2][0].detach().cpu().tolist(), [self.EXPECTED_OUTPUT_0, self.EXPECTED_OUTPUT_0_PT_2])
@slow
def test_conditioning(self):
torch.backends.cuda.matmul.allow_tf32 = False
torch.backends.cudnn.allow_tf32 = False
model = JukeboxModel.from_pretrained(self.model_id, min_duration=0).eval()
labels = self.prepare_inputs()
set_seed(0)
zs = [torch.zeros(1, 0, dtype=torch.long) for _ in range(3)]
top_prior = model.priors[0]
start = 0
music_token_conds = top_prior.get_music_tokens_conds(zs, start=start, end=start + top_prior.n_ctx)
metadata = top_prior.get_metadata(labels[0].clone(), start, 1058304, 0)
self.assertIsNone(music_token_conds)
self.assertListEqual(metadata.numpy()[0][:10].tolist(), self.EXPECTED_Y_COND)
audio_conditioning, metadata_conditioning, lyric_tokens = top_prior.get_cond(music_token_conds, metadata)
torch.testing.assert_allclose(
audio_conditioning[0][0][:30].detach(), torch.tensor(self.EXPECTED_AUDIO_COND), atol=1e-4, rtol=1e-4
)
torch.testing.assert_allclose(
metadata_conditioning[0][0][:30].detach(), torch.tensor(self.EXPECTED_META_COND), atol=1e-4, rtol=1e-4
)
torch.testing.assert_allclose(
lyric_tokens[0, :30].detach(), torch.tensor(self.EXPECTED_LYRIC_COND), atol=1e-4, rtol=1e-4
)
@slow
def test_primed_sampling(self):
torch.backends.cuda.matmul.allow_tf32 = False
torch.backends.cudnn.allow_tf32 = False
model = JukeboxModel.from_pretrained(self.model_id, min_duration=0).eval()
set_seed(0)
waveform = torch.rand((1, 5120, 1))
tokens = list(self.prepare_inputs())
zs = [model.vqvae.encode(waveform, start_level=2, bs_chunks=waveform.shape[0])[0], None, None]
zs = model._sample(
zs, tokens, sample_levels=[0], save_results=False, sample_length=40 * model.priors[0].raw_to_tokens
)
torch.testing.assert_allclose(zs[0][0][:40], torch.tensor(self.EXPECTED_PRIMED_0))
upper_2 = torch.cat((zs[0], torch.zeros(1, 2048 - zs[0].shape[-1])), dim=-1).long()
zs = [upper_2, model.vqvae.encode(waveform, start_level=1, bs_chunks=waveform.shape[0])[0], None]
zs = model._sample(
zs, tokens, sample_levels=[1], save_results=False, sample_length=40 * model.priors[1].raw_to_tokens
)
torch.testing.assert_allclose(zs[1][0][:40], torch.tensor(self.EXPECTED_PRIMED_1))
upper_1 = torch.cat((zs[1], torch.zeros(1, 2048 - zs[1].shape[-1])), dim=-1).long()
zs = [upper_2, upper_1, model.vqvae.encode(waveform, start_level=0, bs_chunks=waveform.shape[0])[0]]
zs = model._sample(
zs, tokens, sample_levels=[2], save_results=False, sample_length=40 * model.priors[2].raw_to_tokens
)
torch.testing.assert_allclose(zs[2][0][:40].cpu(), torch.tensor(self.EXPECTED_PRIMED_2))
@slow
def test_vqvae(self):
model = JukeboxModel.from_pretrained(self.model_id, min_duration=0).eval()
set_seed(0)
x = torch.rand((1, 5120, 1))
with torch.no_grad():
zs = model.vqvae.encode(x, start_level=2, bs_chunks=x.shape[0])
torch.testing.assert_allclose(zs[0][0], torch.tensor(self.EXPECTED_VQVAE_ENCODE))
with torch.no_grad():
x = model.vqvae.decode(zs, start_level=2, bs_chunks=x.shape[0])
torch.testing.assert_allclose(x[0, :40, 0], torch.tensor(self.EXPECTED_VQVAE_DECODE), atol=1e-4, rtol=1e-4)
@require_torch
class Jukebox5bModelTester(unittest.TestCase):
all_model_classes = (JukeboxModel,) if is_torch_available() else ()
model_id = "openai/jukebox-5b-lyrics"
metas = {
"artist": "Zac Brown Band",
"genres": "Country",
"lyrics": """I met a traveller from an antique land,
Who said "Two vast and trunkless legs of stone
Stand in the desert. . . . Near them, on the sand,
Half sunk a shattered visage lies, whose frown,
And wrinkled lip, and sneer of cold command,
Tell that its sculptor well those passions read
Which yet survive, stamped on these lifeless things,
The hand that mocked them, and the heart that fed;
And on the pedestal, these words appear:
My name is Ozymandias, King of Kings;
Look on my Works, ye Mighty, and despair!
Nothing beside remains. Round the decay
Of that colossal Wreck, boundless and bare
The lone and level sands stretch far away
""",
}
# fmt: off
EXPECTED_OUTPUT_2 = [
1489, 653, 653, 653, 653, 653, 653, 653, 653, 653, 653, 653,
653, 653, 653, 653, 653, 653, 653, 653, 653, 653, 653, 653,
653, 653, 653, 653, 653, 653, 653, 653, 653, 653, 653, 653,
653, 653, 653, 653, 653, 653, 653, 653, 653, 653, 653, 653,
1489, 1489, 1489, 1489, 1150, 1853, 1509, 1150, 1357, 1509, 6, 1272
]
EXPECTED_OUTPUT_2_PT_2 = [
1489, 653, 653, 653, 653, 653, 653, 653, 653, 653, 653, 653,
653, 653, 653, 653, 653, 653, 653, 653, 653, 653, 653, 653,
653, 653, 653, 653, 653, 653, 653, 653, 653, 653, 653, 653,
653, 653, 653, 653, 653, 653, 653, 653, 653, 653, 653, 653,
653, 653, 653, 653, 653, 653, 653, 653, 653, 653, 653, 653
]
EXPECTED_OUTPUT_1 = [
1125, 416, 1125, 1125, 1125, 1125, 1125, 416, 416, 416, 416, 416,
416, 416, 416, 416, 416, 416, 416, 416, 416, 416, 416, 416,
416, 416, 416, 416, 416, 416, 416, 416, 416, 416, 416, 416,
416, 416, 416, 416, 416, 416, 416, 416, 416, 416, 416, 416,
416, 416, 416, 416, 416, 416, 416, 416, 416, 416, 416, 416
]
EXPECTED_OUTPUT_1_PT_2 = [
416, 416, 1125, 1125, 416, 416, 416, 416, 416, 416, 416, 416, 416,
416, 416, 416, 416, 416, 416, 416, 416, 416, 416, 416, 416, 416,
416, 416, 416, 416, 416, 416, 416, 416, 416, 416, 416, 416, 416,
416, 416, 416, 416, 416, 416, 416, 416, 416, 416, 416, 416, 416,
416, 416, 416, 416, 416, 416, 416, 416
]
EXPECTED_OUTPUT_0 = [
1755, 1061, 234, 1755, 1061, 1755, 185, 290, 307, 307, 616, 616,
616, 616, 616, 616, 307, 290, 417, 1755, 234, 1755, 185, 290,
290, 290, 307, 616, 616, 616, 616, 616, 290, 234, 234, 1755,
234, 234, 1755, 234, 185, 185, 307, 616, 616, 616, 616, 290,
1755, 1755, 1755, 234, 234, 1755, 1572, 290, 307, 616, 34, 616
]
EXPECTED_OUTPUT_0_PT_2 = [
854, 842, 1353, 114, 1353, 842, 185, 842, 185, 114, 591, 842, 185,
417, 185, 842, 307, 842, 591, 842, 185, 842, 185, 842, 591, 842,
1353, 842, 185, 842, 591, 842, 591, 114, 591, 842, 185, 842, 591,
89, 591, 842, 591, 842, 591, 417, 1372, 842, 1372, 842, 34, 842,
185, 89, 591, 842, 185, 842, 591, 632
]
EXPECTED_GPU_OUTPUTS_2 = [
1489, 653, 653, 653, 653, 653, 653, 653, 653, 653, 653, 653,
653, 653, 653, 653, 653, 653, 653, 653, 653, 653, 653, 653,
653, 653, 653, 653, 653, 653, 653, 653, 653, 653, 653, 653,
653, 653, 653, 653, 653, 653, 653, 653, 653, 653, 653, 653,
653, 653, 653, 653, 653, 653, 653, 653, 653, 653, 653, 653
]
EXPECTED_GPU_OUTPUTS_2_PT_2 = [
1489, 653, 653, 653, 653, 653, 653, 653, 653, 653, 653, 653,
653, 653, 653, 653, 653, 653, 653, 653, 653, 653, 653, 653,
653, 653, 653, 653, 653, 653, 653, 1853, 1177, 1536, 1228,
710, 475, 1489, 1229, 1224, 231, 1224, 252, 1434, 653, 475,
1106, 1877, 1599, 1228, 1600, 1683, 1182, 1853, 475, 1864,
252, 1229, 1434, 2001
]
EXPECTED_GPU_OUTPUTS_1 = [
1125, 1125, 416, 1125, 1125, 416, 1125, 1125, 416, 416, 1125, 416,
416, 416, 416, 416, 416, 416, 416, 416, 416, 416, 416, 416,
416, 416, 416, 416, 416, 416, 416, 416, 416, 416, 416, 416,
416, 416, 416, 416, 416, 416, 416, 416, 416, 416, 416, 416,
416, 416, 416, 416, 416, 416, 416, 416, 416, 416, 416, 416
]
EXPECTED_GPU_OUTPUTS_0 = [
491, 1755, 34, 1613, 1755, 417, 992, 1613, 222, 842, 1353, 1613,
844, 632, 185, 1613, 844, 632, 185, 1613, 185, 842, 677, 1613,
185, 114, 1353, 1613, 307, 89, 844, 1613, 307, 1332, 234, 1979,
307, 89, 1353, 616, 34, 842, 185, 842, 34, 842, 185, 842,
307, 114, 185, 89, 34, 1268, 185, 89, 34, 842, 185, 89
]
# fmt: on
def prepare_inputs(self, model_id):
tokenizer = JukeboxTokenizer.from_pretrained(model_id)
tokens = tokenizer(**self.metas)["input_ids"]
return tokens
@slow
def test_sampling(self):
model = JukeboxModel.from_pretrained(self.model_id, min_duration=0).eval()
labels = self.prepare_inputs(self.model_id)
set_seed(0)
zs = [torch.zeros(1, 0, dtype=torch.long).cpu() for _ in range(3)]
zs = model._sample(zs, labels, [0], sample_length=60 * model.priors[0].raw_to_tokens, save_results=False)
self.assertIn(zs[0][0].detach().cpu().tolist(), [self.EXPECTED_OUTPUT_2, self.EXPECTED_OUTPUT_2_PT_2])
set_seed(0)
zs = model._sample(zs, labels, [1], sample_length=60 * model.priors[1].raw_to_tokens, save_results=False)
self.assertIn(zs[1][0].detach().cpu().tolist(), [self.EXPECTED_OUTPUT_1, self.EXPECTED_OUTPUT_1_PT_2])
set_seed(0)
zs = model._sample(zs, labels, [2], sample_length=60 * model.priors[2].raw_to_tokens, save_results=False)
self.assertIn(zs[2][0].detach().cpu().tolist(), [self.EXPECTED_OUTPUT_0, self.EXPECTED_OUTPUT_0_PT_2])
@slow
@require_torch_accelerator
@skip("Not enough GPU memory on CI runners")
def test_slow_sampling(self):
model = JukeboxModel.from_pretrained(self.model_id, min_duration=0).eval()
labels = [i.to(torch_device) for i in self.prepare_inputs(self.model_id)]
set_seed(0)
model.priors[0].to(torch_device)
zs = [torch.zeros(1, 0, dtype=torch.long).to(torch_device) for _ in range(3)]
zs = model._sample(zs, labels, [0], sample_length=60 * model.priors[0].raw_to_tokens, save_results=False)
torch.testing.assert_allclose(zs[0][0].cpu(), torch.tensor(self.EXPECTED_GPU_OUTPUTS_2))
model.priors[0].cpu()
set_seed(0)
model.priors[1].to(torch_device)
zs = model._sample(zs, labels, [1], sample_length=60 * model.priors[1].raw_to_tokens, save_results=False)
torch.testing.assert_allclose(zs[1][0].cpu(), torch.tensor(self.EXPECTED_GPU_OUTPUTS_1))
model.priors[1].cpu()
set_seed(0)
model.priors[2].to(torch_device)
zs = model._sample(zs, labels, [2], sample_length=60 * model.priors[2].raw_to_tokens, save_results=False)
torch.testing.assert_allclose(zs[2][0].cpu(), torch.tensor(self.EXPECTED_GPU_OUTPUTS_0))
@slow
@require_torch_accelerator
@require_torch_fp16
def test_fp16_slow_sampling(self):
prior_id = "ArthurZ/jukebox_prior_0"
model = JukeboxPrior.from_pretrained(prior_id, min_duration=0).eval().half().to(torch_device)
labels = self.prepare_inputs(prior_id)[0].to(torch_device)
metadata = model.get_metadata(labels, 0, 7680, 0)
set_seed(0)
outputs = model.sample(1, metadata=metadata, sample_tokens=60)
self.assertIn(outputs[0].cpu().tolist(), [self.EXPECTED_GPU_OUTPUTS_2, self.EXPECTED_GPU_OUTPUTS_2_PT_2])
| transformers/tests/models/jukebox/test_modeling_jukebox.py/0 | {
"file_path": "transformers/tests/models/jukebox/test_modeling_jukebox.py",
"repo_id": "transformers",
"token_count": 9327
} | 356 |
# coding=utf-8
# Copyright 2022 The HuggingFace Inc. team. All rights reserved.
#
# Licensed under the Apache License, Version 2.0 (the "License");
# you may not use this file except in compliance with the License.
# You may obtain a copy of the License at
#
# http://www.apache.org/licenses/LICENSE-2.0
#
# Unless required by applicable law or agreed to in writing, software
# distributed under the License is distributed on an "AS IS" BASIS,
# WITHOUT WARRANTIES OR CONDITIONS OF ANY KIND, either express or implied.
# See the License for the specific language governing permissions and
# limitations under the License.
""" Testing suite for the PyTorch LayoutLMv3 model. """
import copy
import unittest
from transformers.models.auto import get_values
from transformers.testing_utils import require_torch, slow, torch_device
from transformers.utils import cached_property, is_torch_available, is_vision_available
from ...test_configuration_common import ConfigTester
from ...test_modeling_common import ModelTesterMixin, floats_tensor, ids_tensor, random_attention_mask
from ...test_pipeline_mixin import PipelineTesterMixin
if is_torch_available():
import torch
from transformers import (
MODEL_FOR_MULTIPLE_CHOICE_MAPPING,
MODEL_FOR_QUESTION_ANSWERING_MAPPING,
MODEL_FOR_SEQUENCE_CLASSIFICATION_MAPPING,
MODEL_FOR_TOKEN_CLASSIFICATION_MAPPING,
LayoutLMv3Config,
LayoutLMv3ForQuestionAnswering,
LayoutLMv3ForSequenceClassification,
LayoutLMv3ForTokenClassification,
LayoutLMv3Model,
)
from transformers.models.layoutlmv3.modeling_layoutlmv3 import LAYOUTLMV3_PRETRAINED_MODEL_ARCHIVE_LIST
if is_vision_available():
from PIL import Image
from transformers import LayoutLMv3ImageProcessor
class LayoutLMv3ModelTester:
def __init__(
self,
parent,
batch_size=2,
num_channels=3,
image_size=4,
patch_size=2,
text_seq_length=7,
is_training=True,
use_input_mask=True,
use_token_type_ids=True,
use_labels=True,
vocab_size=99,
hidden_size=36,
num_hidden_layers=2,
num_attention_heads=4,
intermediate_size=37,
hidden_act="gelu",
hidden_dropout_prob=0.1,
attention_probs_dropout_prob=0.1,
max_position_embeddings=512,
type_vocab_size=16,
type_sequence_label_size=2,
initializer_range=0.02,
coordinate_size=6,
shape_size=6,
num_labels=3,
num_choices=4,
scope=None,
range_bbox=1000,
):
self.parent = parent
self.batch_size = batch_size
self.num_channels = num_channels
self.image_size = image_size
self.patch_size = patch_size
self.text_seq_length = text_seq_length
self.is_training = is_training
self.use_input_mask = use_input_mask
self.use_token_type_ids = use_token_type_ids
self.use_labels = use_labels
self.vocab_size = vocab_size
self.hidden_size = hidden_size
self.num_hidden_layers = num_hidden_layers
self.num_attention_heads = num_attention_heads
self.intermediate_size = intermediate_size
self.hidden_act = hidden_act
self.hidden_dropout_prob = hidden_dropout_prob
self.attention_probs_dropout_prob = attention_probs_dropout_prob
self.max_position_embeddings = max_position_embeddings
self.type_vocab_size = type_vocab_size
self.type_sequence_label_size = type_sequence_label_size
self.initializer_range = initializer_range
self.coordinate_size = coordinate_size
self.shape_size = shape_size
self.num_labels = num_labels
self.num_choices = num_choices
self.scope = scope
self.range_bbox = range_bbox
# LayoutLMv3's sequence length equals the number of text tokens + number of patches + 1 (we add 1 for the CLS token)
self.text_seq_length = text_seq_length
self.image_seq_length = (image_size // patch_size) ** 2 + 1
self.seq_length = self.text_seq_length + self.image_seq_length
def prepare_config_and_inputs(self):
input_ids = ids_tensor([self.batch_size, self.text_seq_length], self.vocab_size)
bbox = ids_tensor([self.batch_size, self.text_seq_length, 4], self.range_bbox)
# Ensure that bbox is legal
for i in range(bbox.shape[0]):
for j in range(bbox.shape[1]):
if bbox[i, j, 3] < bbox[i, j, 1]:
t = bbox[i, j, 3]
bbox[i, j, 3] = bbox[i, j, 1]
bbox[i, j, 1] = t
if bbox[i, j, 2] < bbox[i, j, 0]:
t = bbox[i, j, 2]
bbox[i, j, 2] = bbox[i, j, 0]
bbox[i, j, 0] = t
pixel_values = floats_tensor([self.batch_size, self.num_channels, self.image_size, self.image_size])
input_mask = None
if self.use_input_mask:
input_mask = random_attention_mask([self.batch_size, self.text_seq_length])
token_type_ids = None
if self.use_token_type_ids:
token_type_ids = ids_tensor([self.batch_size, self.text_seq_length], self.type_vocab_size)
sequence_labels = None
token_labels = None
if self.use_labels:
sequence_labels = ids_tensor([self.batch_size], self.type_sequence_label_size)
token_labels = ids_tensor([self.batch_size, self.text_seq_length], self.num_labels)
config = LayoutLMv3Config(
vocab_size=self.vocab_size,
hidden_size=self.hidden_size,
num_hidden_layers=self.num_hidden_layers,
num_attention_heads=self.num_attention_heads,
intermediate_size=self.intermediate_size,
hidden_act=self.hidden_act,
hidden_dropout_prob=self.hidden_dropout_prob,
attention_probs_dropout_prob=self.attention_probs_dropout_prob,
max_position_embeddings=self.max_position_embeddings,
type_vocab_size=self.type_vocab_size,
initializer_range=self.initializer_range,
coordinate_size=self.coordinate_size,
shape_size=self.shape_size,
input_size=self.image_size,
patch_size=self.patch_size,
)
return config, input_ids, bbox, pixel_values, token_type_ids, input_mask, sequence_labels, token_labels
def create_and_check_model(
self, config, input_ids, bbox, pixel_values, token_type_ids, input_mask, sequence_labels, token_labels
):
model = LayoutLMv3Model(config=config)
model.to(torch_device)
model.eval()
# text + image
result = model(input_ids, pixel_values=pixel_values)
result = model(
input_ids, bbox=bbox, pixel_values=pixel_values, attention_mask=input_mask, token_type_ids=token_type_ids
)
result = model(input_ids, bbox=bbox, pixel_values=pixel_values, token_type_ids=token_type_ids)
result = model(input_ids, bbox=bbox, pixel_values=pixel_values)
self.parent.assertEqual(result.last_hidden_state.shape, (self.batch_size, self.seq_length, self.hidden_size))
# text only
result = model(input_ids)
self.parent.assertEqual(
result.last_hidden_state.shape, (self.batch_size, self.text_seq_length, self.hidden_size)
)
# image only
result = model(pixel_values=pixel_values)
self.parent.assertEqual(
result.last_hidden_state.shape, (self.batch_size, self.image_seq_length, self.hidden_size)
)
def create_and_check_for_sequence_classification(
self, config, input_ids, bbox, pixel_values, token_type_ids, input_mask, sequence_labels, token_labels
):
config.num_labels = self.num_labels
model = LayoutLMv3ForSequenceClassification(config)
model.to(torch_device)
model.eval()
result = model(
input_ids,
bbox=bbox,
pixel_values=pixel_values,
attention_mask=input_mask,
token_type_ids=token_type_ids,
labels=sequence_labels,
)
self.parent.assertEqual(result.logits.shape, (self.batch_size, self.num_labels))
def create_and_check_for_token_classification(
self, config, input_ids, bbox, pixel_values, token_type_ids, input_mask, sequence_labels, token_labels
):
config.num_labels = self.num_labels
model = LayoutLMv3ForTokenClassification(config=config)
model.to(torch_device)
model.eval()
result = model(
input_ids,
bbox=bbox,
pixel_values=pixel_values,
attention_mask=input_mask,
token_type_ids=token_type_ids,
labels=token_labels,
)
self.parent.assertEqual(result.logits.shape, (self.batch_size, self.text_seq_length, self.num_labels))
def create_and_check_for_question_answering(
self, config, input_ids, bbox, pixel_values, token_type_ids, input_mask, sequence_labels, token_labels
):
model = LayoutLMv3ForQuestionAnswering(config=config)
model.to(torch_device)
model.eval()
result = model(
input_ids,
bbox=bbox,
pixel_values=pixel_values,
attention_mask=input_mask,
token_type_ids=token_type_ids,
start_positions=sequence_labels,
end_positions=sequence_labels,
)
self.parent.assertEqual(result.start_logits.shape, (self.batch_size, self.seq_length))
self.parent.assertEqual(result.end_logits.shape, (self.batch_size, self.seq_length))
def prepare_config_and_inputs_for_common(self):
config_and_inputs = self.prepare_config_and_inputs()
(
config,
input_ids,
bbox,
pixel_values,
token_type_ids,
input_mask,
sequence_labels,
token_labels,
) = config_and_inputs
inputs_dict = {
"input_ids": input_ids,
"bbox": bbox,
"pixel_values": pixel_values,
"token_type_ids": token_type_ids,
"attention_mask": input_mask,
}
return config, inputs_dict
@require_torch
class LayoutLMv3ModelTest(ModelTesterMixin, PipelineTesterMixin, unittest.TestCase):
test_pruning = False
test_torchscript = False
test_mismatched_shapes = False
all_model_classes = (
(
LayoutLMv3Model,
LayoutLMv3ForSequenceClassification,
LayoutLMv3ForTokenClassification,
LayoutLMv3ForQuestionAnswering,
)
if is_torch_available()
else ()
)
pipeline_model_mapping = (
{"document-question-answering": LayoutLMv3ForQuestionAnswering, "feature-extraction": LayoutLMv3Model}
if is_torch_available()
else {}
)
# TODO: Fix the failed tests
def is_pipeline_test_to_skip(
self, pipeline_test_casse_name, config_class, model_architecture, tokenizer_name, processor_name
):
# `DocumentQuestionAnsweringPipeline` is expected to work with this model, but it combines the text and visual
# embedding along the sequence dimension (dim 1), which causes an error during post-processing as `p_mask` has
# the sequence dimension of the text embedding only.
# (see the line `embedding_output = torch.cat([embedding_output, visual_embeddings], dim=1)`)
return True
def setUp(self):
self.model_tester = LayoutLMv3ModelTester(self)
self.config_tester = ConfigTester(self, config_class=LayoutLMv3Config, hidden_size=37)
def _prepare_for_class(self, inputs_dict, model_class, return_labels=False):
inputs_dict = copy.deepcopy(inputs_dict)
if model_class in get_values(MODEL_FOR_MULTIPLE_CHOICE_MAPPING):
inputs_dict = {
k: v.unsqueeze(1).expand(-1, self.model_tester.num_choices, -1).contiguous()
if isinstance(v, torch.Tensor) and v.ndim > 1
else v
for k, v in inputs_dict.items()
}
if return_labels:
if model_class in get_values(MODEL_FOR_MULTIPLE_CHOICE_MAPPING):
inputs_dict["labels"] = torch.ones(self.model_tester.batch_size, dtype=torch.long, device=torch_device)
elif model_class in get_values(MODEL_FOR_QUESTION_ANSWERING_MAPPING):
inputs_dict["start_positions"] = torch.zeros(
self.model_tester.batch_size, dtype=torch.long, device=torch_device
)
inputs_dict["end_positions"] = torch.zeros(
self.model_tester.batch_size, dtype=torch.long, device=torch_device
)
elif model_class in [
*get_values(MODEL_FOR_SEQUENCE_CLASSIFICATION_MAPPING),
]:
inputs_dict["labels"] = torch.zeros(
self.model_tester.batch_size, dtype=torch.long, device=torch_device
)
elif model_class in [
*get_values(MODEL_FOR_TOKEN_CLASSIFICATION_MAPPING),
]:
inputs_dict["labels"] = torch.zeros(
(self.model_tester.batch_size, self.model_tester.text_seq_length),
dtype=torch.long,
device=torch_device,
)
return inputs_dict
def test_config(self):
self.config_tester.run_common_tests()
def test_model(self):
config_and_inputs = self.model_tester.prepare_config_and_inputs()
self.model_tester.create_and_check_model(*config_and_inputs)
def test_model_various_embeddings(self):
config_and_inputs = self.model_tester.prepare_config_and_inputs()
for type in ["absolute", "relative_key", "relative_key_query"]:
config_and_inputs[0].position_embedding_type = type
self.model_tester.create_and_check_model(*config_and_inputs)
def test_for_sequence_classification(self):
config_and_inputs = self.model_tester.prepare_config_and_inputs()
self.model_tester.create_and_check_for_sequence_classification(*config_and_inputs)
def test_for_token_classification(self):
config_and_inputs = self.model_tester.prepare_config_and_inputs()
self.model_tester.create_and_check_for_token_classification(*config_and_inputs)
def test_for_question_answering(self):
config_and_inputs = self.model_tester.prepare_config_and_inputs()
self.model_tester.create_and_check_for_question_answering(*config_and_inputs)
@slow
def test_model_from_pretrained(self):
for model_name in LAYOUTLMV3_PRETRAINED_MODEL_ARCHIVE_LIST[:1]:
model = LayoutLMv3Model.from_pretrained(model_name)
self.assertIsNotNone(model)
# We will verify our results on an image of cute cats
def prepare_img():
image = Image.open("./tests/fixtures/tests_samples/COCO/000000039769.png")
return image
@require_torch
class LayoutLMv3ModelIntegrationTest(unittest.TestCase):
@cached_property
def default_image_processor(self):
return LayoutLMv3ImageProcessor(apply_ocr=False) if is_vision_available() else None
@slow
def test_inference_no_head(self):
model = LayoutLMv3Model.from_pretrained("microsoft/layoutlmv3-base").to(torch_device)
image_processor = self.default_image_processor
image = prepare_img()
pixel_values = image_processor(images=image, return_tensors="pt").pixel_values.to(torch_device)
input_ids = torch.tensor([[1, 2]])
bbox = torch.tensor([[1, 2, 3, 4], [5, 6, 7, 8]]).unsqueeze(0)
# forward pass
outputs = model(
input_ids=input_ids.to(torch_device),
bbox=bbox.to(torch_device),
pixel_values=pixel_values.to(torch_device),
)
# verify the logits
expected_shape = torch.Size((1, 199, 768))
self.assertEqual(outputs.last_hidden_state.shape, expected_shape)
expected_slice = torch.tensor(
[[-0.0529, 0.3618, 0.1632], [-0.1587, -0.1667, -0.0400], [-0.1557, -0.1671, -0.0505]]
).to(torch_device)
self.assertTrue(torch.allclose(outputs.last_hidden_state[0, :3, :3], expected_slice, atol=1e-4))
| transformers/tests/models/layoutlmv3/test_modeling_layoutlmv3.py/0 | {
"file_path": "transformers/tests/models/layoutlmv3/test_modeling_layoutlmv3.py",
"repo_id": "transformers",
"token_count": 7600
} | 357 |
# coding=utf-8
# Copyright 2023 The HuggingFace Team. All rights reserved.
#
# Licensed under the Apache License, Version 2.0 (the "License");
# you may not use this file except in compliance with the License.
# You may obtain a copy of the License at
#
# http://www.apache.org/licenses/LICENSE-2.0
#
# Unless required by applicable law or agreed to in writing, software
# distributed under the License is distributed on an "AS IS" BASIS,
# WITHOUT WARRANTIES OR CONDITIONS OF ANY KIND, either express or implied.
# See the License for the specific language governing permissions and
# limitations under the License.
import unittest
from transformers import MegaConfig, is_torch_available
from transformers.testing_utils import (
TestCasePlus,
is_flaky,
require_torch,
require_torch_fp16,
slow,
torch_device,
)
from ...generation.test_utils import GenerationTesterMixin
from ...test_configuration_common import ConfigTester
from ...test_modeling_common import ModelTesterMixin, floats_tensor, ids_tensor, random_attention_mask
from ...test_pipeline_mixin import PipelineTesterMixin
if is_torch_available():
import torch
from transformers import (
MegaForCausalLM,
MegaForMaskedLM,
MegaForMultipleChoice,
MegaForQuestionAnswering,
MegaForSequenceClassification,
MegaForTokenClassification,
MegaModel,
)
from transformers.models.mega.modeling_mega import MEGA_PRETRAINED_MODEL_ARCHIVE_LIST
class MegaModelTester:
def __init__(
self,
parent,
batch_size=13,
seq_length=7,
is_training=True,
use_input_mask=True,
use_labels=True,
vocab_size=99,
hidden_size=32,
num_hidden_layers=2,
intermediate_size=37,
hidden_dropout_prob=0.1,
attention_probs_dropout_prob=0.1,
max_positions=1024,
bidirectional=False, # needed for decoding, and can't modify common generation tests; test separately by overriding
ema_projection_size=16,
shared_representation_size=64,
use_chunking=False,
chunk_size=32,
attention_activation="softmax",
use_normalized_ffn=True,
nffn_hidden_size=24,
add_token_type_embeddings=True,
type_vocab_size=2,
type_sequence_label_size=2,
initializer_range=0.02,
num_labels=3,
num_choices=4,
scope=None,
):
self.parent = parent
self.batch_size = batch_size
self.seq_length = seq_length
self.is_training = is_training
self.use_input_mask = use_input_mask
self.add_token_type_embeddings = add_token_type_embeddings
self.use_labels = use_labels
self.vocab_size = vocab_size
self.hidden_size = hidden_size
self.num_hidden_layers = num_hidden_layers
self.intermediate_size = intermediate_size
self.hidden_dropout_prob = hidden_dropout_prob
self.attention_probs_dropout_prob = attention_probs_dropout_prob
self.max_positions = max_positions
self.bidirectional = bidirectional
self.ema_projection_size = ema_projection_size
self.shared_representation_size = shared_representation_size
self.use_chunking = use_chunking
self.chunk_size = chunk_size
self.attention_activation = attention_activation
self.use_normalized_ffn = use_normalized_ffn
self.nffn_hidden_size = nffn_hidden_size
self.type_vocab_size = type_vocab_size
self.type_sequence_label_size = type_sequence_label_size
self.initializer_range = initializer_range
self.num_labels = num_labels
self.num_choices = num_choices
self.scope = scope
self.num_attention_heads = 1
def prepare_config_and_inputs(self):
input_ids = ids_tensor([self.batch_size, self.seq_length], self.vocab_size)
input_mask = None
if self.use_input_mask:
input_mask = random_attention_mask([self.batch_size, self.seq_length])
token_type_ids = None
if self.add_token_type_embeddings:
token_type_ids = ids_tensor([self.batch_size, self.seq_length], self.type_vocab_size)
sequence_labels = None
token_labels = None
choice_labels = None
if self.use_labels:
sequence_labels = ids_tensor([self.batch_size], self.type_sequence_label_size)
token_labels = ids_tensor([self.batch_size, self.seq_length], self.num_labels)
choice_labels = ids_tensor([self.batch_size], self.num_choices)
config = self.get_config()
return config, input_ids, token_type_ids, input_mask, sequence_labels, token_labels, choice_labels
def get_config(self):
return MegaConfig(
vocab_size=self.vocab_size,
hidden_size=self.hidden_size,
num_hidden_layers=self.num_hidden_layers,
intermediate_size=self.intermediate_size,
hidden_dropout_prob=self.hidden_dropout_prob,
attention_probs_dropout_prob=self.attention_probs_dropout_prob,
type_vocab_size=self.type_vocab_size,
initializer_range=self.initializer_range,
# added args
add_token_type_embeddings=self.add_token_type_embeddings,
max_positions=self.max_positions,
bidirectional=self.bidirectional,
ema_projection_size=self.ema_projection_size,
shared_representation_size=self.shared_representation_size,
use_chunking=self.use_chunking,
chunk_size=self.chunk_size,
attention_activation=self.attention_activation,
use_normalized_ffn=self.use_normalized_ffn,
nffn_hidden_size=self.nffn_hidden_size,
)
def get_pipeline_config(self):
config = self.get_config()
config.vocab_size = 300
return config
def prepare_config_and_inputs_for_decoder(self):
(
config,
input_ids,
token_type_ids,
input_mask,
sequence_labels,
token_labels,
choice_labels,
) = self.prepare_config_and_inputs()
config.is_decoder = True
config.bidirectional = False
encoder_hidden_states = floats_tensor([self.batch_size, self.seq_length, self.hidden_size])
encoder_attention_mask = ids_tensor([self.batch_size, self.seq_length], vocab_size=2)
return (
config,
input_ids,
token_type_ids,
input_mask,
sequence_labels,
token_labels,
choice_labels,
encoder_hidden_states,
encoder_attention_mask,
)
def create_and_check_model(
self, config, input_ids, token_type_ids, input_mask, sequence_labels, token_labels, choice_labels
):
model = MegaModel(config=config)
model.to(torch_device)
model.eval()
result = model(input_ids, attention_mask=input_mask, token_type_ids=token_type_ids)
result = model(input_ids, token_type_ids=token_type_ids)
result = model(input_ids)
self.parent.assertEqual(result.last_hidden_state.shape, (self.batch_size, self.seq_length, self.hidden_size))
self.parent.assertEqual(result.pooler_output.shape, (self.batch_size, self.hidden_size))
def create_and_check_model_as_decoder(
self,
config,
input_ids,
token_type_ids,
input_mask,
sequence_labels,
token_labels,
choice_labels,
encoder_hidden_states,
encoder_attention_mask,
):
config.add_cross_attention = True
model = MegaModel(config)
model.to(torch_device)
model.eval()
result = model(
input_ids,
attention_mask=input_mask,
token_type_ids=token_type_ids,
encoder_hidden_states=encoder_hidden_states,
encoder_attention_mask=encoder_attention_mask,
)
result = model(
input_ids,
attention_mask=input_mask,
token_type_ids=token_type_ids,
encoder_hidden_states=encoder_hidden_states,
)
self.parent.assertEqual(result.last_hidden_state.shape, (self.batch_size, self.seq_length, self.hidden_size))
self.parent.assertEqual(result.pooler_output.shape, (self.batch_size, self.hidden_size))
def create_and_check_for_causal_lm(
self,
config,
input_ids,
token_type_ids,
input_mask,
sequence_labels,
token_labels,
choice_labels,
encoder_hidden_states,
encoder_attention_mask,
):
model = MegaForCausalLM(config=config)
model.to(torch_device)
model.eval()
result = model(input_ids, attention_mask=input_mask, token_type_ids=token_type_ids, labels=token_labels)
self.parent.assertEqual(result.logits.shape, (self.batch_size, self.seq_length, self.vocab_size))
def create_and_check_decoder_model_past_large_inputs(
self,
config,
input_ids,
token_type_ids,
input_mask,
sequence_labels,
token_labels,
choice_labels,
encoder_hidden_states,
encoder_attention_mask,
):
config.is_decoder = True
config.bidirectional = False
config.add_cross_attention = True
model = MegaForCausalLM(config=config).to(torch_device).eval()
# make sure that ids don't start with pad token
mask = input_ids.ne(config.pad_token_id).long()
input_ids = input_ids * mask
# first forward pass
outputs = model(
input_ids,
attention_mask=input_mask,
encoder_hidden_states=encoder_hidden_states,
encoder_attention_mask=encoder_attention_mask,
use_cache=True,
)
past_key_values = outputs.past_key_values
# create hypothetical multiple next token and extent to next_input_ids
next_tokens = ids_tensor((self.batch_size, 1), config.vocab_size)
# make sure that ids don't start with pad token
mask = next_tokens.ne(config.pad_token_id).long()
next_tokens = next_tokens * mask
next_mask = ids_tensor((self.batch_size, 1), vocab_size=2)
# append to next input_ids and
next_input_ids = torch.cat([input_ids, next_tokens], dim=-1)
next_attention_mask = torch.cat([input_mask, next_mask], dim=-1)
output_from_no_past = model(
next_input_ids,
attention_mask=next_attention_mask,
encoder_hidden_states=encoder_hidden_states,
encoder_attention_mask=encoder_attention_mask,
output_hidden_states=True,
)["hidden_states"][0]
output_from_past = model(
next_tokens,
attention_mask=next_mask,
encoder_hidden_states=encoder_hidden_states,
encoder_attention_mask=encoder_attention_mask,
past_key_values=past_key_values,
output_hidden_states=True,
)["hidden_states"][0]
# select random slice
random_slice_idx = ids_tensor((1,), output_from_past.shape[-1]).item()
output_from_no_past_slice = output_from_no_past[:, -1:, random_slice_idx].detach()
output_from_past_slice = output_from_past[:, :, random_slice_idx].detach()
self.parent.assertTrue(output_from_past_slice.shape[1] == next_tokens.shape[1])
# test that outputs are equal for slice
self.parent.assertTrue(torch.allclose(output_from_past_slice, output_from_no_past_slice, atol=1e-3))
def create_and_check_decoder_model_with_chunking(
self,
config,
input_ids,
token_type_ids,
input_mask,
sequence_labels,
token_labels,
choice_labels,
encoder_hidden_states,
encoder_attention_mask,
):
config.use_chunking = True
config.output_attentions = True
config.attention_activation = "laplace"
config.chunk_size = input_ids.size(1) * 2
model = MegaForCausalLM(config).to(torch_device).eval()
input_ids = input_ids.repeat(1, 8)
# multiply the sequence length by 8 since we repeat the same ids 8 times in input_ids
input_mask = random_attention_mask([self.batch_size, self.seq_length * 8])
result = model(input_ids, attention_mask=input_mask)
# test if the sequence length of attentions is same provided chunk_size
self.parent.assertEqual(result["attentions"][0].shape[-1], config.chunk_size)
def create_and_check_for_masked_lm(
self, config, input_ids, token_type_ids, input_mask, sequence_labels, token_labels, choice_labels
):
model = MegaForMaskedLM(config=config)
model.to(torch_device)
model.eval()
result = model(input_ids, attention_mask=input_mask, token_type_ids=token_type_ids, labels=token_labels)
self.parent.assertEqual(result.logits.shape, (self.batch_size, self.seq_length, self.vocab_size))
def create_and_check_for_token_classification(
self, config, input_ids, token_type_ids, input_mask, sequence_labels, token_labels, choice_labels
):
config.num_labels = self.num_labels
model = MegaForTokenClassification(config=config)
model.to(torch_device)
model.eval()
result = model(input_ids, attention_mask=input_mask, token_type_ids=token_type_ids, labels=token_labels)
self.parent.assertEqual(result.logits.shape, (self.batch_size, self.seq_length, self.num_labels))
def create_and_check_for_multiple_choice(
self, config, input_ids, token_type_ids, input_mask, sequence_labels, token_labels, choice_labels
):
config.num_choices = self.num_choices
model = MegaForMultipleChoice(config=config)
model.to(torch_device)
model.eval()
multiple_choice_inputs_ids = input_ids.unsqueeze(1).expand(-1, self.num_choices, -1).contiguous()
multiple_choice_token_type_ids = token_type_ids.unsqueeze(1).expand(-1, self.num_choices, -1).contiguous()
multiple_choice_input_mask = input_mask.unsqueeze(1).expand(-1, self.num_choices, -1).contiguous()
result = model(
multiple_choice_inputs_ids,
attention_mask=multiple_choice_input_mask,
token_type_ids=multiple_choice_token_type_ids,
labels=choice_labels,
)
self.parent.assertEqual(result.logits.shape, (self.batch_size, self.num_choices))
def create_and_check_for_question_answering(
self, config, input_ids, token_type_ids, input_mask, sequence_labels, token_labels, choice_labels
):
model = MegaForQuestionAnswering(config=config)
model.to(torch_device)
model.eval()
result = model(
input_ids,
attention_mask=input_mask,
token_type_ids=token_type_ids,
start_positions=sequence_labels,
end_positions=sequence_labels,
)
self.parent.assertEqual(result.start_logits.shape, (self.batch_size, self.seq_length))
self.parent.assertEqual(result.end_logits.shape, (self.batch_size, self.seq_length))
# extra checks for Mega-specific model functionality
def create_and_check_bidirectionality(
self, config, input_ids, token_type_ids, input_mask, sequence_labels, token_labels, choice_labels
):
config.bidirectional = True
model = MegaModel(config)
model.to(torch_device)
model.eval()
# no mask
result = model(input_ids)
# with mask & token types
result = model(input_ids, attention_mask=input_mask, token_type_ids=token_type_ids)
self.parent.assertEqual(result[0].shape, (self.batch_size, self.seq_length, self.hidden_size))
def check_chunking_shorter_sequence(
self, config, input_ids, token_type_ids, input_mask, sequence_labels, token_labels, choice_labels
):
config.use_chunking = True
config.chunk_size = input_ids.size(1) + 25
model = MegaModel(config)
model.to(torch_device)
model.eval()
result = model(input_ids, attention_mask=input_mask, token_type_ids=token_type_ids)
self.parent.assertEqual(result[0].shape, (self.batch_size, self.seq_length, self.hidden_size))
def check_chunking_longer_sequence(
self, config, input_ids, token_type_ids, input_mask, sequence_labels, token_labels, choice_labels
):
config.use_chunking = True
# we want the chunk size to be < sequence length, and the sequence length to be a multiple of chunk size
config.chunk_size = input_ids.size(1) * 2
model = MegaModel(config)
model.to(torch_device)
model.eval()
result = model(
input_ids.repeat(1, 8),
)
self.parent.assertEqual(result[0].shape, (self.batch_size, self.seq_length * 8, self.hidden_size))
def check_laplace_self_attention(
self, config, input_ids, token_type_ids, input_mask, sequence_labels, token_labels, choice_labels
):
config.attention_activation = "laplace"
model = MegaModel(config)
model.to(torch_device)
model.eval()
result = model(input_ids, attention_mask=input_mask, token_type_ids=token_type_ids)
self.parent.assertEqual(result[0].shape, (self.batch_size, self.seq_length, self.hidden_size))
def check_relu2_self_attention(
self, config, input_ids, token_type_ids, input_mask, sequence_labels, token_labels, choice_labels
):
config.attention_activation = "relu2"
model = MegaModel(config)
model.to(torch_device)
model.eval()
result = model(input_ids, attention_mask=input_mask, token_type_ids=token_type_ids)
self.parent.assertEqual(result[0].shape, (self.batch_size, self.seq_length, self.hidden_size))
def check_sequence_length_beyond_max_positions(
self, config, input_ids, token_type_ids, input_mask, sequence_labels, token_labels, choice_labels
):
config.max_positions = self.seq_length - 2
model = MegaModel(config)
model.to(torch_device)
model.eval()
result = model(input_ids, attention_mask=input_mask, token_type_ids=token_type_ids)
self.parent.assertEqual(result[0].shape, (self.batch_size, self.seq_length, self.hidden_size))
def prepare_config_and_inputs_for_common(self):
config_and_inputs = self.prepare_config_and_inputs()
(
config,
input_ids,
token_type_ids,
input_mask,
sequence_labels,
token_labels,
choice_labels,
) = config_and_inputs
inputs_dict = {"input_ids": input_ids, "token_type_ids": token_type_ids, "attention_mask": input_mask}
return config, inputs_dict
@require_torch
class MegaModelTest(ModelTesterMixin, GenerationTesterMixin, PipelineTesterMixin, unittest.TestCase):
all_model_classes = (
(
MegaForCausalLM,
MegaForMaskedLM,
MegaModel,
MegaForSequenceClassification,
MegaForTokenClassification,
MegaForMultipleChoice,
MegaForQuestionAnswering,
)
if is_torch_available()
else ()
)
all_generative_model_classes = (MegaForCausalLM,) if is_torch_available() else ()
pipeline_model_mapping = (
{
"feature-extraction": MegaModel,
"fill-mask": MegaForMaskedLM,
"question-answering": MegaForQuestionAnswering,
"text-classification": MegaForSequenceClassification,
"text-generation": MegaForCausalLM,
"token-classification": MegaForTokenClassification,
"zero-shot": MegaForSequenceClassification,
}
if is_torch_available()
else {}
)
fx_compatible = False
test_head_masking = False
test_pruning = False
def setUp(self):
self.model_tester = MegaModelTester(self)
self.config_tester = ConfigTester(self, config_class=MegaConfig, hidden_size=37)
# TODO: @ydshieh
@is_flaky(description="Sometimes gives `AssertionError` on expected outputs")
def test_pipeline_fill_mask(self):
super().test_pipeline_fill_mask()
# TODO: @ydshieh
@is_flaky(
description="Sometimes gives `RuntimeError: probability tensor contains either `inf`, `nan` or element < 0`"
)
def test_pipeline_text_generation(self):
super().test_pipeline_text_generation()
def test_config(self):
self.config_tester.run_common_tests()
def test_model(self):
config_and_inputs = self.model_tester.prepare_config_and_inputs()
self.model_tester.create_and_check_model(*config_and_inputs)
def test_model_as_decoder(self):
config_and_inputs = self.model_tester.prepare_config_and_inputs_for_decoder()
self.model_tester.create_and_check_model_as_decoder(*config_and_inputs)
def test_model_as_decoder_with_default_input_mask(self):
# This regression test was failing with PyTorch < 1.3
(
config,
input_ids,
token_type_ids,
input_mask,
sequence_labels,
token_labels,
choice_labels,
encoder_hidden_states,
encoder_attention_mask,
) = self.model_tester.prepare_config_and_inputs_for_decoder()
input_mask = None
self.model_tester.create_and_check_model_as_decoder(
config,
input_ids,
token_type_ids,
input_mask,
sequence_labels,
token_labels,
choice_labels,
encoder_hidden_states,
encoder_attention_mask,
)
def test_for_causal_lm(self):
config_and_inputs = self.model_tester.prepare_config_and_inputs_for_decoder()
self.model_tester.create_and_check_for_causal_lm(*config_and_inputs)
def test_decoder_model_past_with_large_inputs(self):
config_and_inputs = self.model_tester.prepare_config_and_inputs_for_decoder()
self.model_tester.create_and_check_decoder_model_past_large_inputs(*config_and_inputs)
def test_decoder_model_with_chunking(self):
config_and_inputs = self.model_tester.prepare_config_and_inputs_for_decoder()
self.model_tester.create_and_check_decoder_model_with_chunking(*config_and_inputs)
def test_for_masked_lm(self):
config_and_inputs = self.model_tester.prepare_config_and_inputs()
self.model_tester.create_and_check_for_masked_lm(*config_and_inputs)
def test_for_token_classification(self):
config_and_inputs = self.model_tester.prepare_config_and_inputs()
self.model_tester.create_and_check_for_token_classification(*config_and_inputs)
def test_for_multiple_choice(self):
config_and_inputs = self.model_tester.prepare_config_and_inputs()
self.model_tester.create_and_check_for_multiple_choice(*config_and_inputs)
def test_for_question_answering(self):
config_and_inputs = self.model_tester.prepare_config_and_inputs()
self.model_tester.create_and_check_for_question_answering(*config_and_inputs)
def test_for_bidirectionality(self):
config_and_inputs = self.model_tester.prepare_config_and_inputs()
self.model_tester.create_and_check_bidirectionality(*config_and_inputs)
def test_for_chunking_shorter_sequence(self):
config_and_inputs = self.model_tester.prepare_config_and_inputs()
self.model_tester.check_chunking_shorter_sequence(*config_and_inputs)
def test_for_chunking_longer_sequence(self):
config_and_inputs = self.model_tester.prepare_config_and_inputs()
self.model_tester.check_chunking_longer_sequence(*config_and_inputs)
def test_for_laplace_attention(self):
config_and_inputs = self.model_tester.prepare_config_and_inputs()
self.model_tester.check_laplace_self_attention(*config_and_inputs)
def test_for_relu2_attention(self):
config_and_inputs = self.model_tester.prepare_config_and_inputs()
self.model_tester.check_relu2_self_attention(*config_and_inputs)
def test_for_sequence_length_beyond_max_positions(self):
config_and_inputs = self.model_tester.prepare_config_and_inputs()
self.model_tester.check_sequence_length_beyond_max_positions(*config_and_inputs)
@require_torch_fp16
def test_generate_fp16(self):
config, input_ids, _, attention_mask, *_ = self.model_tester.prepare_config_and_inputs_for_decoder()
# attention_mask = torch.LongTensor(input_ids.ne(1)).to(torch_device)
model = MegaForCausalLM(config).eval().to(torch_device)
model.half()
model.generate(input_ids, attention_mask=attention_mask)
model.generate(num_beams=4, do_sample=True, early_stopping=False, num_return_sequences=3)
def test_sequence_classification_model(self):
config, input_ids, _, attention_mask, *_ = self.model_tester.prepare_config_and_inputs()
config.num_labels = self.model_tester.num_labels
sequence_labels = ids_tensor([self.model_tester.batch_size], self.model_tester.type_sequence_label_size)
model = MegaForSequenceClassification(config)
model.to(torch_device)
model.eval()
result = model(input_ids, attention_mask=attention_mask, labels=sequence_labels)
self.assertEqual(result.logits.shape, (self.model_tester.batch_size, self.model_tester.num_labels))
def test_sequence_classification_model_for_multi_label(self):
config, input_ids, _, attention_mask, *_ = self.model_tester.prepare_config_and_inputs()
config.num_labels = self.model_tester.num_labels
config.problem_type = "multi_label_classification"
sequence_labels = ids_tensor(
[self.model_tester.batch_size, config.num_labels], self.model_tester.type_sequence_label_size
).to(torch.float)
model = MegaForSequenceClassification(config)
model.to(torch_device)
model.eval()
result = model(input_ids, attention_mask=attention_mask, labels=sequence_labels)
self.assertEqual(result.logits.shape, (self.model_tester.batch_size, self.model_tester.num_labels))
@slow
def test_model_from_pretrained(self):
for model_name in MEGA_PRETRAINED_MODEL_ARCHIVE_LIST[:1]:
model = MegaModel.from_pretrained(model_name)
self.assertIsNotNone(model)
@unittest.skip(reason="Does not work on the tiny model as we keep hitting edge cases.")
def test_cpu_offload(self):
super().test_cpu_offload()
@unittest.skip(reason="Does not work on the tiny model as we keep hitting edge cases.")
def test_disk_offload(self):
super().test_disk_offload()
@unittest.skip(reason="Does not work on the tiny model as we keep hitting edge cases.")
def test_model_parallelism(self):
super().test_model_parallelism()
@unittest.skip(
reason=(
"Calling `self.attention_function` in `MegaMovingAverageGatedAttention.forward` changes the submodules on "
"device 1 to device 0 (also changes `requires_grad`). No idea how this could happen for now."
)
)
def test_multi_gpu_data_parallel_forward(self):
super().test_multi_gpu_data_parallel_forward()
@unittest.skip(reason="Tracing of the dynamically computed `MegaMultiDimensionDampedEma._kernel` doesn't work.")
def test_torchscript_simple(self):
super().test_torchscript_simple()
@unittest.skip(reason="Tracing of the dynamically computed `MegaMultiDimensionDampedEma._kernel` doesn't work.")
def test_torchscript_output_hidden_state(self):
super().test_torchscript_output_hidden_state()
@unittest.skip(reason="Tracing of the dynamically computed `MegaMultiDimensionDampedEma._kernel` doesn't work.")
def test_torchscript_output_attentions(self):
super().test_torchscript_output_attentions()
@require_torch
class MegaModelIntegrationTest(TestCasePlus):
@slow
def test_inference_masked_lm(self):
model = MegaForMaskedLM.from_pretrained("mnaylor/mega-base-wikitext")
input_ids = torch.tensor([[0, 31414, 232, 328, 740, 1140, 12695, 69, 46078, 1588, 2]])
with torch.no_grad():
output = model(input_ids)[0]
expected_shape = torch.Size((1, 11, 50265))
self.assertEqual(output.shape, expected_shape)
# compare the actual values for a slice.
expected_slice = torch.tensor(
[[[67.8389, 10.1470, -32.7148], [-11.1655, 29.1152, 23.1304], [-3.8015, 66.0397, 29.6733]]]
)
self.assertTrue(torch.allclose(output[:, :3, :3], expected_slice, atol=1e-4))
@slow
def test_inference_no_head(self):
model = MegaModel.from_pretrained("mnaylor/mega-base-wikitext")
input_ids = torch.tensor([[0, 31414, 232, 328, 740, 1140, 12695, 69, 46078, 1588, 2]])
with torch.no_grad():
output = model(input_ids)[0]
expected_shape = torch.Size((1, 11, 128))
self.assertEqual(output.shape, expected_shape)
# compare the actual values for a slice. taken from output[:, :3, :3]
expected_slice = torch.tensor(
[[[1.1767, -0.6349, 2.8494], [-0.5109, -0.7745, 1.9495], [-0.3287, -0.2111, 3.3367]]]
)
self.assertTrue(torch.allclose(output[:, :3, :3], expected_slice, atol=1e-4))
| transformers/tests/models/mega/test_modeling_mega.py/0 | {
"file_path": "transformers/tests/models/mega/test_modeling_mega.py",
"repo_id": "transformers",
"token_count": 13179
} | 358 |
# coding=utf-8
# Copyright 2021, The HuggingFace Inc. team. All rights reserved.
#
# Licensed under the Apache License, Version 2.0 (the "License");
# you may not use this file except in compliance with the License.
# You may obtain a copy of the License at
#
# http://www.apache.org/licenses/LICENSE-2.0
#
# Unless required by applicable law or agreed to in writing, software
# distributed under the License is distributed on an "AS IS" BASIS,
# WITHOUT WARRANTIES OR CONDITIONS OF ANY KIND, either express or implied.
# See the License for the specific language governing permissions and
# limitations under the License.
""" Testing suite for the PyTorch MVP model. """
import copy
import tempfile
import unittest
import timeout_decorator # noqa
from transformers import MvpConfig, is_torch_available
from transformers.testing_utils import (
require_sentencepiece,
require_tokenizers,
require_torch,
require_torch_fp16,
slow,
torch_device,
)
from transformers.utils import cached_property
from ...generation.test_utils import GenerationTesterMixin
from ...test_configuration_common import ConfigTester
from ...test_modeling_common import ModelTesterMixin, floats_tensor, ids_tensor
from ...test_pipeline_mixin import PipelineTesterMixin
if is_torch_available():
import torch
from transformers import (
MvpForCausalLM,
MvpForConditionalGeneration,
MvpForQuestionAnswering,
MvpForSequenceClassification,
MvpModel,
MvpTokenizer,
)
from transformers.models.mvp.modeling_mvp import MvpDecoder, MvpEncoder, shift_tokens_right
def prepare_mvp_inputs_dict(
config,
input_ids,
decoder_input_ids=None,
attention_mask=None,
decoder_attention_mask=None,
head_mask=None,
decoder_head_mask=None,
cross_attn_head_mask=None,
):
if attention_mask is None:
attention_mask = input_ids.ne(config.pad_token_id)
if decoder_attention_mask is None:
decoder_attention_mask = decoder_input_ids.ne(config.pad_token_id)
if head_mask is None:
head_mask = torch.ones(config.encoder_layers, config.encoder_attention_heads, device=torch_device)
if decoder_head_mask is None:
decoder_head_mask = torch.ones(config.decoder_layers, config.decoder_attention_heads, device=torch_device)
if cross_attn_head_mask is None:
cross_attn_head_mask = torch.ones(config.decoder_layers, config.decoder_attention_heads, device=torch_device)
return {
"input_ids": input_ids,
"decoder_input_ids": decoder_input_ids,
"attention_mask": attention_mask,
"decoder_attention_mask": attention_mask,
"head_mask": head_mask,
"decoder_head_mask": decoder_head_mask,
"cross_attn_head_mask": cross_attn_head_mask,
}
class MvpModelTester:
def __init__(
self,
parent,
batch_size=13,
seq_length=7,
is_training=True,
use_labels=False,
vocab_size=99,
hidden_size=16,
num_hidden_layers=2,
num_attention_heads=4,
intermediate_size=4,
hidden_act="gelu",
hidden_dropout_prob=0.1,
attention_probs_dropout_prob=0.1,
max_position_embeddings=20,
eos_token_id=2,
pad_token_id=1,
bos_token_id=0,
):
self.parent = parent
self.batch_size = batch_size
self.seq_length = seq_length
self.is_training = is_training
self.use_labels = use_labels
self.vocab_size = vocab_size
self.hidden_size = hidden_size
self.num_hidden_layers = num_hidden_layers
self.num_attention_heads = num_attention_heads
self.intermediate_size = intermediate_size
self.hidden_act = hidden_act
self.hidden_dropout_prob = hidden_dropout_prob
self.attention_probs_dropout_prob = attention_probs_dropout_prob
self.max_position_embeddings = max_position_embeddings
self.eos_token_id = eos_token_id
self.pad_token_id = pad_token_id
self.bos_token_id = bos_token_id
def prepare_config_and_inputs(self):
input_ids = ids_tensor([self.batch_size, self.seq_length], self.vocab_size)
input_ids = ids_tensor([self.batch_size, self.seq_length], self.vocab_size).clamp(
3,
)
input_ids[:, -1] = self.eos_token_id # Eos Token
decoder_input_ids = ids_tensor([self.batch_size, self.seq_length], self.vocab_size)
config = self.get_config()
inputs_dict = prepare_mvp_inputs_dict(config, input_ids, decoder_input_ids)
return config, inputs_dict
def get_config(self):
return MvpConfig(
vocab_size=self.vocab_size,
d_model=self.hidden_size,
encoder_layers=self.num_hidden_layers,
decoder_layers=self.num_hidden_layers,
encoder_attention_heads=self.num_attention_heads,
decoder_attention_heads=self.num_attention_heads,
encoder_ffn_dim=self.intermediate_size,
decoder_ffn_dim=self.intermediate_size,
dropout=self.hidden_dropout_prob,
attention_dropout=self.attention_probs_dropout_prob,
max_position_embeddings=self.max_position_embeddings,
eos_token_id=self.eos_token_id,
bos_token_id=self.bos_token_id,
pad_token_id=self.pad_token_id,
)
def get_pipeline_config(self):
config = self.get_config()
config.max_position_embeddings = 100
config.vocab_size = 300
return config
def prepare_config_and_inputs_for_common(self):
config, inputs_dict = self.prepare_config_and_inputs()
return config, inputs_dict
def create_and_check_decoder_model_past_large_inputs(self, config, inputs_dict):
model = MvpModel(config=config).get_decoder().to(torch_device).eval()
input_ids = inputs_dict["input_ids"]
attention_mask = inputs_dict["attention_mask"]
head_mask = inputs_dict["head_mask"]
# first forward pass
outputs = model(input_ids, attention_mask=attention_mask, head_mask=head_mask, use_cache=True)
output, past_key_values = outputs.to_tuple()
# create hypothetical multiple next token and extent to next_input_ids
next_tokens = ids_tensor((self.batch_size, 3), config.vocab_size)
next_attn_mask = ids_tensor((self.batch_size, 3), 2)
# append to next input_ids and
next_input_ids = torch.cat([input_ids, next_tokens], dim=-1)
next_attention_mask = torch.cat([attention_mask, next_attn_mask], dim=-1)
output_from_no_past = model(next_input_ids, attention_mask=next_attention_mask)["last_hidden_state"]
output_from_past = model(next_tokens, attention_mask=next_attention_mask, past_key_values=past_key_values)[
"last_hidden_state"
]
# select random slice
random_slice_idx = ids_tensor((1,), output_from_past.shape[-1]).item()
output_from_no_past_slice = output_from_no_past[:, -3:, random_slice_idx].detach()
output_from_past_slice = output_from_past[:, :, random_slice_idx].detach()
self.parent.assertTrue(output_from_past_slice.shape[1] == next_tokens.shape[1])
# test that outputs are equal for slice
self.parent.assertTrue(torch.allclose(output_from_past_slice, output_from_no_past_slice, atol=1e-3))
def check_encoder_decoder_model_standalone(self, config, inputs_dict):
model = MvpModel(config=config).to(torch_device).eval()
outputs = model(**inputs_dict)
encoder_last_hidden_state = outputs.encoder_last_hidden_state
last_hidden_state = outputs.last_hidden_state
with tempfile.TemporaryDirectory() as tmpdirname:
encoder = model.get_encoder()
encoder.save_pretrained(tmpdirname)
encoder = MvpEncoder.from_pretrained(tmpdirname).to(torch_device)
encoder_last_hidden_state_2 = encoder(inputs_dict["input_ids"], attention_mask=inputs_dict["attention_mask"])[
0
]
self.parent.assertTrue((encoder_last_hidden_state_2 - encoder_last_hidden_state).abs().max().item() < 1e-3)
with tempfile.TemporaryDirectory() as tmpdirname:
decoder = model.get_decoder()
decoder.save_pretrained(tmpdirname)
decoder = MvpDecoder.from_pretrained(tmpdirname).to(torch_device)
last_hidden_state_2 = decoder(
input_ids=inputs_dict["decoder_input_ids"],
attention_mask=inputs_dict["decoder_attention_mask"],
encoder_hidden_states=encoder_last_hidden_state,
encoder_attention_mask=inputs_dict["attention_mask"],
)[0]
self.parent.assertTrue((last_hidden_state_2 - last_hidden_state).abs().max().item() < 1e-3)
@require_torch
class MvpHeadTests(unittest.TestCase):
vocab_size = 99
def _get_config_and_data(self):
input_ids = torch.tensor(
[
[71, 82, 18, 33, 46, 91, 2],
[68, 34, 26, 58, 30, 82, 2],
[5, 97, 17, 39, 94, 40, 2],
[76, 83, 94, 25, 70, 78, 2],
[87, 59, 41, 35, 48, 66, 2],
[55, 13, 16, 58, 5, 2, 1], # note padding
[64, 27, 31, 51, 12, 75, 2],
[52, 64, 86, 17, 83, 39, 2],
[48, 61, 9, 24, 71, 82, 2],
[26, 1, 60, 48, 22, 13, 2],
[21, 5, 62, 28, 14, 76, 2],
[45, 98, 37, 86, 59, 48, 2],
[70, 70, 50, 9, 28, 0, 2],
],
dtype=torch.long,
device=torch_device,
)
batch_size = input_ids.shape[0]
config = MvpConfig(
vocab_size=self.vocab_size,
d_model=24,
encoder_layers=2,
decoder_layers=2,
encoder_attention_heads=2,
decoder_attention_heads=2,
encoder_ffn_dim=32,
decoder_ffn_dim=32,
max_position_embeddings=48,
eos_token_id=2,
pad_token_id=1,
bos_token_id=0,
)
return config, input_ids, batch_size
def test_sequence_classification_forward(self):
config, input_ids, batch_size = self._get_config_and_data()
labels = _long_tensor([2] * batch_size).to(torch_device)
config.num_labels = 3
model = MvpForSequenceClassification(config)
model.to(torch_device)
outputs = model(input_ids=input_ids, decoder_input_ids=input_ids, labels=labels)
expected_shape = torch.Size((batch_size, config.num_labels))
self.assertEqual(outputs["logits"].shape, expected_shape)
self.assertIsInstance(outputs["loss"].item(), float)
def test_question_answering_forward(self):
config, input_ids, batch_size = self._get_config_and_data()
sequence_labels = ids_tensor([batch_size], 2).to(torch_device)
model = MvpForQuestionAnswering(config)
model.to(torch_device)
outputs = model(
input_ids=input_ids,
start_positions=sequence_labels,
end_positions=sequence_labels,
)
self.assertEqual(outputs["start_logits"].shape, input_ids.shape)
self.assertEqual(outputs["end_logits"].shape, input_ids.shape)
self.assertIsInstance(outputs["loss"].item(), float)
@timeout_decorator.timeout(1)
def test_lm_forward(self):
config, input_ids, batch_size = self._get_config_and_data()
lm_labels = ids_tensor([batch_size, input_ids.shape[1]], self.vocab_size).to(torch_device)
lm_model = MvpForConditionalGeneration(config)
lm_model.to(torch_device)
outputs = lm_model(input_ids=input_ids, labels=lm_labels)
expected_shape = (batch_size, input_ids.shape[1], config.vocab_size)
self.assertEqual(outputs["logits"].shape, expected_shape)
self.assertIsInstance(outputs["loss"].item(), float)
def test_lm_uneven_forward(self):
config = MvpConfig(
vocab_size=self.vocab_size,
d_model=14,
encoder_layers=2,
decoder_layers=2,
encoder_attention_heads=2,
decoder_attention_heads=2,
encoder_ffn_dim=8,
decoder_ffn_dim=8,
max_position_embeddings=48,
)
lm_model = MvpForConditionalGeneration(config).to(torch_device)
context = torch.tensor(
[[71, 82, 18, 33, 46, 91, 2], [68, 34, 26, 58, 30, 2, 1]], device=torch_device, dtype=torch.long
)
summary = torch.tensor([[82, 71, 82, 18, 2], [58, 68, 2, 1, 1]], device=torch_device, dtype=torch.long)
outputs = lm_model(input_ids=context, decoder_input_ids=summary, labels=summary)
expected_shape = (*summary.shape, config.vocab_size)
self.assertEqual(outputs["logits"].shape, expected_shape)
def test_generate_beam_search(self):
input_ids = torch.tensor([[71, 82, 2], [68, 34, 2]], device=torch_device, dtype=torch.long)
config = MvpConfig(
vocab_size=self.vocab_size,
d_model=24,
encoder_layers=2,
decoder_layers=2,
encoder_attention_heads=2,
decoder_attention_heads=2,
encoder_ffn_dim=32,
decoder_ffn_dim=32,
max_position_embeddings=48,
eos_token_id=2,
pad_token_id=1,
bos_token_id=0,
)
lm_model = MvpForConditionalGeneration(config).to(torch_device)
lm_model.eval()
max_length = 5
generated_ids = lm_model.generate(
input_ids.clone(),
do_sample=True,
num_return_sequences=1,
num_beams=2,
no_repeat_ngram_size=3,
max_length=max_length,
)
self.assertEqual(generated_ids.shape, (input_ids.shape[0], max_length))
def test_shift_tokens_right(self):
input_ids = torch.tensor([[71, 82, 18, 33, 2, 1, 1], [68, 34, 26, 58, 30, 82, 2]], dtype=torch.long)
shifted = shift_tokens_right(input_ids, 1, 2)
n_pad_before = input_ids.eq(1).float().sum()
n_pad_after = shifted.eq(1).float().sum()
self.assertEqual(shifted.shape, input_ids.shape)
self.assertEqual(n_pad_after, n_pad_before - 1)
self.assertTrue(torch.eq(shifted[:, 0], 2).all())
@slow
def test_tokenization(self):
tokenizer = MvpTokenizer.from_pretrained("RUCAIBox/mvp")
examples = [" Hello world", " DomDramg"] # need leading spaces for equality
fairseq_results = [
torch.tensor([0, 20920, 232, 2]),
torch.tensor([0, 11349, 495, 4040, 571, 2]),
]
for ex, desired_result in zip(examples, fairseq_results):
mvp_toks = tokenizer.encode(ex, return_tensors="pt").squeeze()
assert_tensors_close(desired_result.long(), mvp_toks, prefix=ex)
@require_torch_fp16
def test_generate_fp16(self):
config, input_ids, batch_size = self._get_config_and_data()
attention_mask = input_ids.ne(1).to(torch_device)
model = MvpForConditionalGeneration(config).eval().to(torch_device)
model.half()
model.generate(input_ids, attention_mask=attention_mask)
model.generate(num_beams=4, do_sample=True, early_stopping=False, num_return_sequences=3)
def test_dummy_inputs(self):
config, *_ = self._get_config_and_data()
model = MvpForConditionalGeneration(config).eval().to(torch_device)
model(**model.dummy_inputs)
def test_resize_tokens_embeddings_more(self):
config, input_ids, _ = self._get_config_and_data()
def _get_embs(m):
return (m.get_input_embeddings().weight.data.clone(), m.get_output_embeddings().weight.data.clone())
model = MvpForConditionalGeneration(config).eval().to(torch_device)
input, output = _get_embs(model)
self.assertTrue(torch.eq(input, output).all())
new_vocab_size = 45
model.resize_token_embeddings(new_vocab_size)
input_new, output_new = _get_embs(model)
self.assertEqual(input_new.shape, (new_vocab_size, config.d_model))
self.assertEqual(output_new.shape, (new_vocab_size, config.d_model))
self.assertTrue(torch.eq(input_new, output_new).all())
@require_torch
class MvpModelTest(ModelTesterMixin, GenerationTesterMixin, PipelineTesterMixin, unittest.TestCase):
all_model_classes = (
(MvpModel, MvpForConditionalGeneration, MvpForSequenceClassification, MvpForQuestionAnswering)
if is_torch_available()
else ()
)
all_generative_model_classes = (MvpForConditionalGeneration,) if is_torch_available() else ()
pipeline_model_mapping = (
{
"conversational": MvpForConditionalGeneration,
"feature-extraction": MvpModel,
"fill-mask": MvpForConditionalGeneration,
"question-answering": MvpForQuestionAnswering,
"summarization": MvpForConditionalGeneration,
"text-classification": MvpForSequenceClassification,
"text-generation": MvpForCausalLM,
"text2text-generation": MvpForConditionalGeneration,
"translation": MvpForConditionalGeneration,
"zero-shot": MvpForSequenceClassification,
}
if is_torch_available()
else {}
)
is_encoder_decoder = True
fx_compatible = False
test_pruning = False
test_missing_keys = False
# TODO: Fix the failed tests
def is_pipeline_test_to_skip(
self, pipeline_test_casse_name, config_class, model_architecture, tokenizer_name, processor_name
):
if (
pipeline_test_casse_name == "QAPipelineTests"
and tokenizer_name is not None
and not tokenizer_name.endswith("Fast")
):
# `QAPipelineTests` fails for a few models when the slower tokenizer are used.
# (The slower tokenizers were never used for pipeline tests before the pipeline testing rework)
# TODO: check (and possibly fix) the `QAPipelineTests` with slower tokenizer
return True
return False
def setUp(self):
self.model_tester = MvpModelTester(self)
self.config_tester = ConfigTester(self, config_class=MvpConfig)
def test_config(self):
self.config_tester.run_common_tests()
def test_save_load_strict(self):
config, inputs_dict = self.model_tester.prepare_config_and_inputs()
for model_class in self.all_model_classes:
model = model_class(config)
with tempfile.TemporaryDirectory() as tmpdirname:
model.save_pretrained(tmpdirname)
model2, info = model_class.from_pretrained(tmpdirname, output_loading_info=True)
self.assertEqual(info["missing_keys"], [])
def test_decoder_model_past_with_large_inputs(self):
config_and_inputs = self.model_tester.prepare_config_and_inputs()
self.model_tester.create_and_check_decoder_model_past_large_inputs(*config_and_inputs)
def test_encoder_decoder_model_standalone(self):
config_and_inputs = self.model_tester.prepare_config_and_inputs_for_common()
self.model_tester.check_encoder_decoder_model_standalone(*config_and_inputs)
# MvpForSequenceClassification does not support inputs_embeds
def test_inputs_embeds(self):
config, inputs_dict = self.model_tester.prepare_config_and_inputs_for_common()
for model_class in (MvpModel, MvpForConditionalGeneration, MvpForQuestionAnswering):
model = model_class(config)
model.to(torch_device)
model.eval()
inputs = copy.deepcopy(self._prepare_for_class(inputs_dict, model_class))
if not self.is_encoder_decoder:
input_ids = inputs["input_ids"]
del inputs["input_ids"]
else:
encoder_input_ids = inputs["input_ids"]
decoder_input_ids = inputs.get("decoder_input_ids", encoder_input_ids)
del inputs["input_ids"]
inputs.pop("decoder_input_ids", None)
wte = model.get_input_embeddings()
if not self.is_encoder_decoder:
inputs["inputs_embeds"] = wte(input_ids)
else:
inputs["inputs_embeds"] = wte(encoder_input_ids)
inputs["decoder_inputs_embeds"] = wte(decoder_input_ids)
with torch.no_grad():
model(**inputs)[0]
@require_torch_fp16
def test_generate_fp16(self):
config, input_dict = self.model_tester.prepare_config_and_inputs()
input_ids = input_dict["input_ids"]
attention_mask = input_ids.ne(1).to(torch_device)
model = MvpForConditionalGeneration(config).eval().to(torch_device)
model.half()
model.generate(input_ids, attention_mask=attention_mask)
model.generate(num_beams=4, do_sample=True, early_stopping=False, num_return_sequences=3)
def assert_tensors_close(a, b, atol=1e-12, prefix=""):
"""If tensors have different shapes, different values or a and b are not both tensors, raise a nice Assertion error."""
if a is None and b is None:
return True
try:
if torch.allclose(a, b, atol=atol):
return True
raise
except Exception:
pct_different = (torch.gt((a - b).abs(), atol)).float().mean().item()
if a.numel() > 100:
msg = f"tensor values are {pct_different:.1%} percent different."
else:
msg = f"{a} != {b}"
if prefix:
msg = prefix + ": " + msg
raise AssertionError(msg)
def _long_tensor(tok_lst):
return torch.tensor(tok_lst, dtype=torch.long, device=torch_device)
@require_torch
@require_sentencepiece
@require_tokenizers
class MvpModelIntegrationTests(unittest.TestCase):
@cached_property
def default_tokenizer(self):
return MvpTokenizer.from_pretrained("RUCAIBox/mvp")
@slow
def test_inference_no_head(self):
model = MvpModel.from_pretrained("RUCAIBox/mvp").to(torch_device)
input_ids = _long_tensor([[0, 31414, 232, 328, 740, 1140, 12695, 69, 46078, 1588, 2]])
attention_mask = input_ids.ne(model.config.pad_token_id)
with torch.no_grad():
output = model(input_ids=input_ids, attention_mask=attention_mask).last_hidden_state
expected_shape = torch.Size((1, 11, 1024))
self.assertEqual(output.shape, expected_shape)
expected_slice = torch.tensor(
[[0.3461, 0.3624, 0.2689], [0.3461, 0.3624, 0.2689], [-0.1562, 1.1637, -0.3784]], device=torch_device
)
self.assertTrue(torch.allclose(output[:, :3, :3], expected_slice, atol=1e-3))
@slow
def test_summarization_inference(self):
model = MvpForConditionalGeneration.from_pretrained("RUCAIBox/mvp").to(torch_device)
tok = self.default_tokenizer
PGE_ARTICLE = """ Listen to local radio broadcasts for advertisements that reference casinos in your area.\nIf none are in your area, listen to national radio broadcasts for advertisements of casinos in other areas.\nNote the location that is mentioned in each advertisement that involves a casino.\nIf no locations are mentioned, note any additional contact information, such as a website or phone number. Use that information to find out where the casinos are.;\n,\n\nIf you learn about more than 1 casino on the radio, use the Internet to search the distance between your location and each casino. Sites such as maps.google.com or mapquest.com will help you in this search.'""" # fmt: skip
EXPECTED_SUMMARY = "Listen to the radio.\nUse the Internet."
dct = tok.batch_encode_plus(
[PGE_ARTICLE],
return_tensors="pt",
).to(torch_device)
hypotheses_batch = model.generate(**dct)
decoded = tok.batch_decode(hypotheses_batch, skip_special_tokens=True)
self.assertEqual(EXPECTED_SUMMARY, decoded[0])
class MvpStandaloneDecoderModelTester:
def __init__(
self,
parent,
vocab_size=99,
batch_size=13,
d_model=16,
decoder_seq_length=7,
is_training=True,
is_decoder=True,
use_attention_mask=True,
use_cache=False,
use_labels=True,
decoder_start_token_id=2,
decoder_ffn_dim=32,
decoder_layers=2,
encoder_attention_heads=4,
decoder_attention_heads=4,
max_position_embeddings=30,
is_encoder_decoder=False,
pad_token_id=0,
bos_token_id=1,
eos_token_id=2,
scope=None,
):
self.parent = parent
self.batch_size = batch_size
self.decoder_seq_length = decoder_seq_length
# For common tests
self.seq_length = self.decoder_seq_length
self.is_training = is_training
self.use_attention_mask = use_attention_mask
self.use_labels = use_labels
self.vocab_size = vocab_size
self.d_model = d_model
self.hidden_size = d_model
self.num_hidden_layers = decoder_layers
self.decoder_layers = decoder_layers
self.decoder_ffn_dim = decoder_ffn_dim
self.encoder_attention_heads = encoder_attention_heads
self.decoder_attention_heads = decoder_attention_heads
self.num_attention_heads = decoder_attention_heads
self.eos_token_id = eos_token_id
self.bos_token_id = bos_token_id
self.pad_token_id = pad_token_id
self.decoder_start_token_id = decoder_start_token_id
self.use_cache = use_cache
self.max_position_embeddings = max_position_embeddings
self.is_encoder_decoder = is_encoder_decoder
self.scope = None
self.decoder_key_length = decoder_seq_length
self.base_model_out_len = 2
self.decoder_attention_idx = 1
def prepare_config_and_inputs(self):
input_ids = ids_tensor([self.batch_size, self.decoder_seq_length], self.vocab_size)
attention_mask = None
if self.use_attention_mask:
attention_mask = ids_tensor([self.batch_size, self.decoder_seq_length], vocab_size=2)
lm_labels = None
if self.use_labels:
lm_labels = ids_tensor([self.batch_size, self.decoder_seq_length], self.vocab_size)
config = MvpConfig(
vocab_size=self.vocab_size,
d_model=self.d_model,
encoder_layers=self.decoder_layers,
decoder_layers=self.decoder_layers,
decoder_ffn_dim=self.decoder_ffn_dim,
encoder_attention_heads=self.encoder_attention_heads,
decoder_attention_heads=self.decoder_attention_heads,
eos_token_id=self.eos_token_id,
bos_token_id=self.bos_token_id,
use_cache=self.use_cache,
pad_token_id=self.pad_token_id,
decoder_start_token_id=self.decoder_start_token_id,
max_position_embeddings=self.max_position_embeddings,
is_encoder_decoder=self.is_encoder_decoder,
)
return (
config,
input_ids,
attention_mask,
lm_labels,
)
def prepare_config_and_inputs_for_decoder(self):
(
config,
input_ids,
attention_mask,
lm_labels,
) = self.prepare_config_and_inputs()
encoder_hidden_states = floats_tensor([self.batch_size, self.decoder_seq_length, self.hidden_size])
encoder_attention_mask = ids_tensor([self.batch_size, self.decoder_seq_length], vocab_size=2)
return (
config,
input_ids,
attention_mask,
encoder_hidden_states,
encoder_attention_mask,
lm_labels,
)
def create_and_check_decoder_model_past(
self,
config,
input_ids,
attention_mask,
lm_labels,
):
config.use_cache = True
model = MvpDecoder(config=config).to(torch_device).eval()
# first forward pass
outputs = model(input_ids, use_cache=True)
outputs_use_cache_conf = model(input_ids)
outputs_no_past = model(input_ids, use_cache=False)
self.parent.assertTrue(len(outputs) == len(outputs_use_cache_conf))
self.parent.assertTrue(len(outputs) == len(outputs_no_past) + 1)
past_key_values = outputs["past_key_values"]
# create hypothetical next token and extent to next_input_ids
next_tokens = ids_tensor((self.batch_size, 1), config.vocab_size)
# append to next input_ids and
next_input_ids = torch.cat([input_ids, next_tokens], dim=-1)
output_from_no_past = model(next_input_ids)["last_hidden_state"]
output_from_past = model(next_tokens, past_key_values=past_key_values)["last_hidden_state"]
# select random slice
random_slice_idx = ids_tensor((1,), output_from_past.shape[-1]).item()
output_from_no_past_slice = output_from_no_past[:, next_input_ids.shape[-1] - 1, random_slice_idx].detach()
output_from_past_slice = output_from_past[:, 0, random_slice_idx].detach()
# test that outputs are equal for slice
assert torch.allclose(output_from_past_slice, output_from_no_past_slice, atol=1e-3)
def create_and_check_decoder_model_attention_mask_past(
self,
config,
input_ids,
attention_mask,
lm_labels,
):
model = MvpDecoder(config=config).to(torch_device).eval()
# create attention mask
attn_mask = torch.ones(input_ids.shape, dtype=torch.long, device=torch_device)
half_seq_length = input_ids.shape[-1] // 2
attn_mask[:, half_seq_length:] = 0
# first forward pass
past_key_values = model(input_ids, attention_mask=attn_mask, use_cache=True)["past_key_values"]
# create hypothetical next token and extent to next_input_ids
next_tokens = ids_tensor((self.batch_size, 1), config.vocab_size)
# change a random masked slice from input_ids
random_seq_idx_to_change = ids_tensor((1,), half_seq_length).item() + 1
random_other_next_tokens = ids_tensor((self.batch_size, 1), config.vocab_size).squeeze(-1)
input_ids[:, -random_seq_idx_to_change] = random_other_next_tokens
# append to next input_ids and attn_mask
next_input_ids = torch.cat([input_ids, next_tokens], dim=-1)
attn_mask = torch.cat(
[attn_mask, torch.ones((attn_mask.shape[0], 1), dtype=torch.long, device=torch_device)],
dim=1,
)
# get two different outputs
output_from_no_past = model(next_input_ids, attention_mask=attn_mask)["last_hidden_state"]
output_from_past = model(next_tokens, attention_mask=attn_mask, past_key_values=past_key_values)[
"last_hidden_state"
]
# select random slice
random_slice_idx = ids_tensor((1,), output_from_past.shape[-1]).item()
output_from_no_past_slice = output_from_no_past[:, next_input_ids.shape[-1] - 1, random_slice_idx].detach()
output_from_past_slice = output_from_past[:, 0, random_slice_idx].detach()
# test that outputs are equal for slice
assert torch.allclose(output_from_past_slice, output_from_no_past_slice, atol=1e-3)
def prepare_config_and_inputs_for_common(self):
config_and_inputs = self.prepare_config_and_inputs()
(
config,
input_ids,
attention_mask,
lm_labels,
) = config_and_inputs
inputs_dict = {
"input_ids": input_ids,
"attention_mask": attention_mask,
}
return config, inputs_dict
@require_torch
class MvpStandaloneDecoderModelTest(ModelTesterMixin, GenerationTesterMixin, unittest.TestCase):
all_model_classes = (MvpDecoder, MvpForCausalLM) if is_torch_available() else ()
all_generative_model_classes = (MvpForCausalLM,) if is_torch_available() else ()
fx_comptatible = True
test_pruning = False
is_encoder_decoder = False
def setUp(
self,
):
self.model_tester = MvpStandaloneDecoderModelTester(self, is_training=False)
self.config_tester = ConfigTester(self, config_class=MvpConfig)
def test_config(self):
self.config_tester.run_common_tests()
def test_decoder_model_past(self):
config_and_inputs = self.model_tester.prepare_config_and_inputs()
self.model_tester.create_and_check_decoder_model_past(*config_and_inputs)
def test_decoder_model_attn_mask_past(self):
config_and_inputs = self.model_tester.prepare_config_and_inputs()
self.model_tester.create_and_check_decoder_model_attention_mask_past(*config_and_inputs)
def test_retain_grad_hidden_states_attentions(self):
# decoder cannot keep gradients
return
@unittest.skip("The model doesn't support left padding") # and it's not used enough to be worth fixing :)
def test_left_padding_compatibility(self):
pass
| transformers/tests/models/mvp/test_modeling_mvp.py/0 | {
"file_path": "transformers/tests/models/mvp/test_modeling_mvp.py",
"repo_id": "transformers",
"token_count": 15265
} | 359 |
# coding=utf-8
# Copyright 2022 HuggingFace Inc.
#
# Licensed under the Apache License, Version 2.0 (the "License");
# you may not use this file except in compliance with the License.
# You may obtain a copy of the License at
#
# http://www.apache.org/licenses/LICENSE-2.0
#
# Unless required by applicable law or agreed to in writing, software
# distributed under the License is distributed on an "AS IS" BASIS,
# WITHOUT WARRANTIES OR CONDITIONS OF ANY KIND, either express or implied.
# See the License for the specific language governing permissions and
# limitations under the License.
import json
import os
import tempfile
import unittest
import numpy as np
from transformers.testing_utils import require_torch, require_vision
from transformers.utils import is_torch_available, is_vision_available
from ...test_image_processing_common import ImageProcessingTestMixin, prepare_image_inputs
if is_torch_available():
import torch
if is_vision_available():
from transformers import OneFormerImageProcessor
from transformers.models.oneformer.image_processing_oneformer import binary_mask_to_rle, prepare_metadata
from transformers.models.oneformer.modeling_oneformer import OneFormerForUniversalSegmentationOutput
if is_vision_available():
from PIL import Image
class OneFormerImageProcessorTester(unittest.TestCase):
def __init__(
self,
parent,
batch_size=7,
num_channels=3,
min_resolution=30,
max_resolution=400,
size=None,
do_resize=True,
do_normalize=True,
image_mean=[0.5, 0.5, 0.5],
image_std=[0.5, 0.5, 0.5],
num_labels=10,
do_reduce_labels=False,
ignore_index=255,
repo_path="shi-labs/oneformer_demo",
class_info_file="ade20k_panoptic.json",
num_text=10,
):
self.parent = parent
self.batch_size = batch_size
self.num_channels = num_channels
self.min_resolution = min_resolution
self.max_resolution = max_resolution
self.do_resize = do_resize
self.size = {"shortest_edge": 32, "longest_edge": 1333} if size is None else size
self.do_normalize = do_normalize
self.image_mean = image_mean
self.image_std = image_std
self.class_info_file = class_info_file
self.num_text = num_text
self.repo_path = repo_path
# for the post_process_functions
self.batch_size = 2
self.num_queries = 10
self.num_classes = 10
self.height = 3
self.width = 4
self.num_labels = num_labels
self.do_reduce_labels = do_reduce_labels
self.ignore_index = ignore_index
def prepare_image_processor_dict(self):
return {
"do_resize": self.do_resize,
"size": self.size,
"do_normalize": self.do_normalize,
"image_mean": self.image_mean,
"image_std": self.image_std,
"num_labels": self.num_labels,
"do_reduce_labels": self.do_reduce_labels,
"ignore_index": self.ignore_index,
"class_info_file": self.class_info_file,
"num_text": self.num_text,
}
def get_expected_values(self, image_inputs, batched=False):
"""
This function computes the expected height and width when providing images to OneFormerImageProcessor,
assuming do_resize is set to True with a scalar size.
"""
if not batched:
image = image_inputs[0]
if isinstance(image, Image.Image):
w, h = image.size
else:
h, w = image.shape[1], image.shape[2]
if w < h:
expected_height = int(self.size["shortest_edge"] * h / w)
expected_width = self.size["shortest_edge"]
elif w > h:
expected_height = self.size["shortest_edge"]
expected_width = int(self.size["shortest_edge"] * w / h)
else:
expected_height = self.size["shortest_edge"]
expected_width = self.size["shortest_edge"]
else:
expected_values = []
for image in image_inputs:
expected_height, expected_width = self.get_expected_values([image])
expected_values.append((expected_height, expected_width))
expected_height = max(expected_values, key=lambda item: item[0])[0]
expected_width = max(expected_values, key=lambda item: item[1])[1]
return expected_height, expected_width
def get_fake_oneformer_outputs(self):
return OneFormerForUniversalSegmentationOutput(
# +1 for null class
class_queries_logits=torch.randn((self.batch_size, self.num_queries, self.num_classes + 1)),
masks_queries_logits=torch.randn((self.batch_size, self.num_queries, self.height, self.width)),
)
def expected_output_image_shape(self, images):
height, width = self.get_expected_values(images, batched=True)
return self.num_channels, height, width
def prepare_image_inputs(self, equal_resolution=False, numpify=False, torchify=False):
return prepare_image_inputs(
batch_size=self.batch_size,
num_channels=self.num_channels,
min_resolution=self.min_resolution,
max_resolution=self.max_resolution,
equal_resolution=equal_resolution,
numpify=numpify,
torchify=torchify,
)
@require_torch
@require_vision
class OneFormerImageProcessingTest(ImageProcessingTestMixin, unittest.TestCase):
image_processing_class = OneFormerImageProcessor if (is_vision_available() and is_torch_available()) else None
# only for test_image_processing_common.test_image_proc_to_json_string
image_processing_class = image_processing_class
def setUp(self):
self.image_processor_tester = OneFormerImageProcessorTester(self)
@property
def image_processor_dict(self):
return self.image_processor_tester.prepare_image_processor_dict()
def test_image_proc_properties(self):
image_processor = self.image_processing_class(**self.image_processor_dict)
self.assertTrue(hasattr(image_processor, "image_mean"))
self.assertTrue(hasattr(image_processor, "image_std"))
self.assertTrue(hasattr(image_processor, "do_normalize"))
self.assertTrue(hasattr(image_processor, "do_resize"))
self.assertTrue(hasattr(image_processor, "size"))
self.assertTrue(hasattr(image_processor, "ignore_index"))
self.assertTrue(hasattr(image_processor, "class_info_file"))
self.assertTrue(hasattr(image_processor, "num_text"))
self.assertTrue(hasattr(image_processor, "repo_path"))
self.assertTrue(hasattr(image_processor, "metadata"))
self.assertTrue(hasattr(image_processor, "do_reduce_labels"))
def comm_get_image_processor_inputs(
self, with_segmentation_maps=False, is_instance_map=False, segmentation_type="np"
):
image_processor = self.image_processing_class(**self.image_processor_dict)
# prepare image and target
num_labels = self.image_processor_tester.num_labels
annotations = None
instance_id_to_semantic_id = None
image_inputs = self.image_processor_tester.prepare_image_inputs(equal_resolution=False)
if with_segmentation_maps:
high = num_labels
if is_instance_map:
labels_expanded = list(range(num_labels)) * 2
instance_id_to_semantic_id = dict(enumerate(labels_expanded))
annotations = [
np.random.randint(0, high * 2, (img.size[1], img.size[0])).astype(np.uint8) for img in image_inputs
]
if segmentation_type == "pil":
annotations = [Image.fromarray(annotation) for annotation in annotations]
inputs = image_processor(
image_inputs,
["semantic"] * len(image_inputs),
annotations,
return_tensors="pt",
instance_id_to_semantic_id=instance_id_to_semantic_id,
pad_and_return_pixel_mask=True,
)
return inputs
def test_init_without_params(self):
pass
def test_call_with_segmentation_maps(self):
def common(is_instance_map=False, segmentation_type=None):
inputs = self.comm_get_image_processor_inputs(
with_segmentation_maps=True, is_instance_map=is_instance_map, segmentation_type=segmentation_type
)
mask_labels = inputs["mask_labels"]
class_labels = inputs["class_labels"]
pixel_values = inputs["pixel_values"]
text_inputs = inputs["text_inputs"]
# check the batch_size
for mask_label, class_label, text_input in zip(mask_labels, class_labels, text_inputs):
self.assertEqual(mask_label.shape[0], class_label.shape[0])
# this ensure padding has happened
self.assertEqual(mask_label.shape[1:], pixel_values.shape[2:])
self.assertEqual(len(text_input), self.image_processor_tester.num_text)
common()
common(is_instance_map=True)
common(is_instance_map=False, segmentation_type="pil")
common(is_instance_map=True, segmentation_type="pil")
def test_binary_mask_to_rle(self):
fake_binary_mask = np.zeros((20, 50))
fake_binary_mask[0, 20:] = 1
fake_binary_mask[1, :15] = 1
fake_binary_mask[5, :10] = 1
rle = binary_mask_to_rle(fake_binary_mask)
self.assertEqual(len(rle), 4)
self.assertEqual(rle[0], 21)
self.assertEqual(rle[1], 45)
def test_post_process_semantic_segmentation(self):
fature_extractor = self.image_processing_class(
num_labels=self.image_processor_tester.num_classes,
max_seq_length=77,
task_seq_length=77,
class_info_file="ade20k_panoptic.json",
num_text=self.image_processor_tester.num_text,
repo_path="shi-labs/oneformer_demo",
)
outputs = self.image_processor_tester.get_fake_oneformer_outputs()
segmentation = fature_extractor.post_process_semantic_segmentation(outputs)
self.assertEqual(len(segmentation), self.image_processor_tester.batch_size)
self.assertEqual(
segmentation[0].shape,
(
self.image_processor_tester.height,
self.image_processor_tester.width,
),
)
target_sizes = [(1, 4) for i in range(self.image_processor_tester.batch_size)]
segmentation = fature_extractor.post_process_semantic_segmentation(outputs, target_sizes=target_sizes)
self.assertEqual(segmentation[0].shape, target_sizes[0])
def test_post_process_instance_segmentation(self):
image_processor = self.image_processing_class(
num_labels=self.image_processor_tester.num_classes,
max_seq_length=77,
task_seq_length=77,
class_info_file="ade20k_panoptic.json",
num_text=self.image_processor_tester.num_text,
repo_path="shi-labs/oneformer_demo",
)
outputs = self.image_processor_tester.get_fake_oneformer_outputs()
segmentation = image_processor.post_process_instance_segmentation(outputs, threshold=0)
self.assertTrue(len(segmentation) == self.image_processor_tester.batch_size)
for el in segmentation:
self.assertTrue("segmentation" in el)
self.assertTrue("segments_info" in el)
self.assertEqual(type(el["segments_info"]), list)
self.assertEqual(
el["segmentation"].shape, (self.image_processor_tester.height, self.image_processor_tester.width)
)
def test_post_process_panoptic_segmentation(self):
image_processor = self.image_processing_class(
num_labels=self.image_processor_tester.num_classes,
max_seq_length=77,
task_seq_length=77,
class_info_file="ade20k_panoptic.json",
num_text=self.image_processor_tester.num_text,
repo_path="shi-labs/oneformer_demo",
)
outputs = self.image_processor_tester.get_fake_oneformer_outputs()
segmentation = image_processor.post_process_panoptic_segmentation(outputs, threshold=0)
self.assertTrue(len(segmentation) == self.image_processor_tester.batch_size)
for el in segmentation:
self.assertTrue("segmentation" in el)
self.assertTrue("segments_info" in el)
self.assertEqual(type(el["segments_info"]), list)
self.assertEqual(
el["segmentation"].shape, (self.image_processor_tester.height, self.image_processor_tester.width)
)
def test_can_load_with_local_metadata(self):
# Create a temporary json file
class_info = {
"0": {"isthing": 0, "name": "foo"},
"1": {"isthing": 0, "name": "bar"},
"2": {"isthing": 1, "name": "baz"},
}
metadata = prepare_metadata(class_info)
with tempfile.TemporaryDirectory() as tmpdirname:
metadata_path = os.path.join(tmpdirname, "metadata.json")
with open(metadata_path, "w") as f:
json.dump(class_info, f)
config_dict = self.image_processor_dict
config_dict["class_info_file"] = metadata_path
config_dict["repo_path"] = tmpdirname
image_processor = self.image_processing_class(**config_dict)
self.assertEqual(image_processor.metadata, metadata)
| transformers/tests/models/oneformer/test_image_processing_oneformer.py/0 | {
"file_path": "transformers/tests/models/oneformer/test_image_processing_oneformer.py",
"repo_id": "transformers",
"token_count": 6134
} | 360 |
# coding=utf-8
# Copyright 2022 The HuggingFace Inc. team. All rights reserved.
#
# Licensed under the Apache License, Version 2.0 (the "License");
# you may not use this file except in compliance with the License.
# You may obtain a copy of the License at
#
# http://www.apache.org/licenses/LICENSE-2.0
#
# Unless required by applicable law or agreed to in writing, software
# distributed under the License is distributed on an "AS IS" BASIS,
# WITHOUT WARRANTIES OR CONDITIONS OF ANY KIND, either express or implied.
# See the License for the specific language governing permissions and
# limitations under the License.
""" Testing suite for the PyTorch OwlViT model. """
import inspect
import os
import tempfile
import unittest
import numpy as np
import requests
from transformers import OwlViTConfig, OwlViTTextConfig, OwlViTVisionConfig
from transformers.testing_utils import (
require_torch,
require_torch_accelerator,
require_torch_fp16,
require_vision,
slow,
torch_device,
)
from transformers.utils import is_torch_available, is_vision_available
from ...test_configuration_common import ConfigTester
from ...test_modeling_common import (
ModelTesterMixin,
_config_zero_init,
floats_tensor,
ids_tensor,
random_attention_mask,
)
from ...test_pipeline_mixin import PipelineTesterMixin
if is_torch_available():
import torch
from torch import nn
from transformers import OwlViTForObjectDetection, OwlViTModel, OwlViTTextModel, OwlViTVisionModel
from transformers.models.owlvit.modeling_owlvit import OWLVIT_PRETRAINED_MODEL_ARCHIVE_LIST
if is_vision_available():
from PIL import Image
from transformers import OwlViTProcessor
class OwlViTVisionModelTester:
def __init__(
self,
parent,
batch_size=12,
image_size=32,
patch_size=2,
num_channels=3,
is_training=True,
hidden_size=32,
num_hidden_layers=2,
num_attention_heads=4,
intermediate_size=37,
dropout=0.1,
attention_dropout=0.1,
initializer_range=0.02,
scope=None,
):
self.parent = parent
self.batch_size = batch_size
self.image_size = image_size
self.patch_size = patch_size
self.num_channels = num_channels
self.is_training = is_training
self.hidden_size = hidden_size
self.num_hidden_layers = num_hidden_layers
self.num_attention_heads = num_attention_heads
self.intermediate_size = intermediate_size
self.dropout = dropout
self.attention_dropout = attention_dropout
self.initializer_range = initializer_range
self.scope = scope
# in ViT, the seq length equals the number of patches + 1 (we add 1 for the [CLS] token)
num_patches = (image_size // patch_size) ** 2
self.seq_length = num_patches + 1
def prepare_config_and_inputs(self):
pixel_values = floats_tensor([self.batch_size, self.num_channels, self.image_size, self.image_size])
config = self.get_config()
return config, pixel_values
def get_config(self):
return OwlViTVisionConfig(
image_size=self.image_size,
patch_size=self.patch_size,
num_channels=self.num_channels,
hidden_size=self.hidden_size,
num_hidden_layers=self.num_hidden_layers,
num_attention_heads=self.num_attention_heads,
intermediate_size=self.intermediate_size,
dropout=self.dropout,
attention_dropout=self.attention_dropout,
initializer_range=self.initializer_range,
)
def create_and_check_model(self, config, pixel_values):
model = OwlViTVisionModel(config=config).to(torch_device)
model.eval()
pixel_values = pixel_values.to(torch.float32)
with torch.no_grad():
result = model(pixel_values)
# expected sequence length = num_patches + 1 (we add 1 for the [CLS] token)
num_patches = (self.image_size // self.patch_size) ** 2
self.parent.assertEqual(result.last_hidden_state.shape, (self.batch_size, num_patches + 1, self.hidden_size))
self.parent.assertEqual(result.pooler_output.shape, (self.batch_size, self.hidden_size))
def prepare_config_and_inputs_for_common(self):
config_and_inputs = self.prepare_config_and_inputs()
config, pixel_values = config_and_inputs
inputs_dict = {"pixel_values": pixel_values}
return config, inputs_dict
@require_torch
class OwlViTVisionModelTest(ModelTesterMixin, unittest.TestCase):
"""
Here we also overwrite some of the tests of test_modeling_common.py, as OWLVIT does not use input_ids, inputs_embeds,
attention_mask and seq_length.
"""
all_model_classes = (OwlViTVisionModel,) if is_torch_available() else ()
fx_compatible = False
test_pruning = False
test_resize_embeddings = False
test_head_masking = False
def setUp(self):
self.model_tester = OwlViTVisionModelTester(self)
self.config_tester = ConfigTester(
self, config_class=OwlViTVisionConfig, has_text_modality=False, hidden_size=37
)
def test_config(self):
self.config_tester.run_common_tests()
@unittest.skip(reason="OWLVIT does not use inputs_embeds")
def test_inputs_embeds(self):
pass
def test_model_common_attributes(self):
config, _ = self.model_tester.prepare_config_and_inputs_for_common()
for model_class in self.all_model_classes:
model = model_class(config)
self.assertIsInstance(model.get_input_embeddings(), (nn.Module))
x = model.get_output_embeddings()
self.assertTrue(x is None or isinstance(x, nn.Linear))
def test_forward_signature(self):
config, _ = self.model_tester.prepare_config_and_inputs_for_common()
for model_class in self.all_model_classes:
model = model_class(config)
signature = inspect.signature(model.forward)
# signature.parameters is an OrderedDict => so arg_names order is deterministic
arg_names = [*signature.parameters.keys()]
expected_arg_names = ["pixel_values"]
self.assertListEqual(arg_names[:1], expected_arg_names)
def test_model(self):
config_and_inputs = self.model_tester.prepare_config_and_inputs()
self.model_tester.create_and_check_model(*config_and_inputs)
@unittest.skip(reason="OWL-ViT does not support training yet")
def test_training(self):
pass
@unittest.skip(reason="OWL-ViT does not support training yet")
def test_training_gradient_checkpointing(self):
pass
@unittest.skip(
reason="This architecure seem to not compute gradients properly when using GC, check: https://github.com/huggingface/transformers/pull/27124"
)
def test_training_gradient_checkpointing_use_reentrant(self):
pass
@unittest.skip(
reason="This architecure seem to not compute gradients properly when using GC, check: https://github.com/huggingface/transformers/pull/27124"
)
def test_training_gradient_checkpointing_use_reentrant_false(self):
pass
@unittest.skip(reason="OwlViTVisionModel has no base class and is not available in MODEL_MAPPING")
def test_save_load_fast_init_from_base(self):
pass
@unittest.skip(reason="OwlViTVisionModel has no base class and is not available in MODEL_MAPPING")
def test_save_load_fast_init_to_base(self):
pass
@slow
def test_model_from_pretrained(self):
for model_name in OWLVIT_PRETRAINED_MODEL_ARCHIVE_LIST[:1]:
model = OwlViTVisionModel.from_pretrained(model_name)
self.assertIsNotNone(model)
class OwlViTTextModelTester:
def __init__(
self,
parent,
batch_size=12,
num_queries=4,
seq_length=16,
is_training=True,
use_input_mask=True,
use_labels=True,
vocab_size=99,
hidden_size=64,
num_hidden_layers=12,
num_attention_heads=4,
intermediate_size=37,
dropout=0.1,
attention_dropout=0.1,
max_position_embeddings=16,
initializer_range=0.02,
scope=None,
):
self.parent = parent
self.batch_size = batch_size
self.num_queries = num_queries
self.seq_length = seq_length
self.is_training = is_training
self.use_input_mask = use_input_mask
self.use_labels = use_labels
self.vocab_size = vocab_size
self.hidden_size = hidden_size
self.num_hidden_layers = num_hidden_layers
self.num_attention_heads = num_attention_heads
self.intermediate_size = intermediate_size
self.dropout = dropout
self.attention_dropout = attention_dropout
self.max_position_embeddings = max_position_embeddings
self.initializer_range = initializer_range
self.scope = scope
def prepare_config_and_inputs(self):
input_ids = ids_tensor([self.batch_size * self.num_queries, self.seq_length], self.vocab_size)
input_mask = None
if self.use_input_mask:
input_mask = random_attention_mask([self.batch_size * self.num_queries, self.seq_length])
if input_mask is not None:
num_text, seq_length = input_mask.shape
rnd_start_indices = np.random.randint(1, seq_length - 1, size=(num_text,))
for idx, start_index in enumerate(rnd_start_indices):
input_mask[idx, :start_index] = 1
input_mask[idx, start_index:] = 0
config = self.get_config()
return config, input_ids, input_mask
def get_config(self):
return OwlViTTextConfig(
vocab_size=self.vocab_size,
hidden_size=self.hidden_size,
num_hidden_layers=self.num_hidden_layers,
num_attention_heads=self.num_attention_heads,
intermediate_size=self.intermediate_size,
dropout=self.dropout,
attention_dropout=self.attention_dropout,
max_position_embeddings=self.max_position_embeddings,
initializer_range=self.initializer_range,
)
def create_and_check_model(self, config, input_ids, input_mask):
model = OwlViTTextModel(config=config).to(torch_device)
model.eval()
with torch.no_grad():
result = model(input_ids=input_ids, attention_mask=input_mask)
self.parent.assertEqual(
result.last_hidden_state.shape, (self.batch_size * self.num_queries, self.seq_length, self.hidden_size)
)
self.parent.assertEqual(result.pooler_output.shape, (self.batch_size * self.num_queries, self.hidden_size))
def prepare_config_and_inputs_for_common(self):
config_and_inputs = self.prepare_config_and_inputs()
config, input_ids, input_mask = config_and_inputs
inputs_dict = {"input_ids": input_ids, "attention_mask": input_mask}
return config, inputs_dict
@require_torch
class OwlViTTextModelTest(ModelTesterMixin, unittest.TestCase):
all_model_classes = (OwlViTTextModel,) if is_torch_available() else ()
fx_compatible = False
test_pruning = False
test_head_masking = False
def setUp(self):
self.model_tester = OwlViTTextModelTester(self)
self.config_tester = ConfigTester(self, config_class=OwlViTTextConfig, hidden_size=37)
def test_config(self):
self.config_tester.run_common_tests()
def test_model(self):
config_and_inputs = self.model_tester.prepare_config_and_inputs()
self.model_tester.create_and_check_model(*config_and_inputs)
@unittest.skip(reason="OWL-ViT does not support training yet")
def test_training(self):
pass
@unittest.skip(reason="OWL-ViT does not support training yet")
def test_training_gradient_checkpointing(self):
pass
@unittest.skip(
reason="This architecure seem to not compute gradients properly when using GC, check: https://github.com/huggingface/transformers/pull/27124"
)
def test_training_gradient_checkpointing_use_reentrant(self):
pass
@unittest.skip(
reason="This architecure seem to not compute gradients properly when using GC, check: https://github.com/huggingface/transformers/pull/27124"
)
def test_training_gradient_checkpointing_use_reentrant_false(self):
pass
@unittest.skip(reason="OWLVIT does not use inputs_embeds")
def test_inputs_embeds(self):
pass
@unittest.skip(reason="OwlViTTextModel has no base class and is not available in MODEL_MAPPING")
def test_save_load_fast_init_from_base(self):
pass
@unittest.skip(reason="OwlViTTextModel has no base class and is not available in MODEL_MAPPING")
def test_save_load_fast_init_to_base(self):
pass
@slow
def test_model_from_pretrained(self):
for model_name in OWLVIT_PRETRAINED_MODEL_ARCHIVE_LIST[:1]:
model = OwlViTTextModel.from_pretrained(model_name)
self.assertIsNotNone(model)
class OwlViTModelTester:
def __init__(self, parent, text_kwargs=None, vision_kwargs=None, is_training=True):
if text_kwargs is None:
text_kwargs = {}
if vision_kwargs is None:
vision_kwargs = {}
self.parent = parent
self.text_model_tester = OwlViTTextModelTester(parent, **text_kwargs)
self.vision_model_tester = OwlViTVisionModelTester(parent, **vision_kwargs)
self.is_training = is_training
self.text_config = self.text_model_tester.get_config().to_dict()
self.vision_config = self.vision_model_tester.get_config().to_dict()
def prepare_config_and_inputs(self):
text_config, input_ids, attention_mask = self.text_model_tester.prepare_config_and_inputs()
vision_config, pixel_values = self.vision_model_tester.prepare_config_and_inputs()
config = self.get_config()
return config, input_ids, attention_mask, pixel_values
def get_config(self):
return OwlViTConfig.from_text_vision_configs(self.text_config, self.vision_config, projection_dim=64)
def create_and_check_model(self, config, input_ids, attention_mask, pixel_values):
model = OwlViTModel(config).to(torch_device).eval()
with torch.no_grad():
result = model(
input_ids=input_ids,
pixel_values=pixel_values,
attention_mask=attention_mask,
)
image_logits_size = (
self.vision_model_tester.batch_size,
self.text_model_tester.batch_size * self.text_model_tester.num_queries,
)
text_logits_size = (
self.text_model_tester.batch_size * self.text_model_tester.num_queries,
self.vision_model_tester.batch_size,
)
self.parent.assertEqual(result.logits_per_image.shape, image_logits_size)
self.parent.assertEqual(result.logits_per_text.shape, text_logits_size)
def prepare_config_and_inputs_for_common(self):
config_and_inputs = self.prepare_config_and_inputs()
config, input_ids, attention_mask, pixel_values = config_and_inputs
inputs_dict = {
"pixel_values": pixel_values,
"input_ids": input_ids,
"attention_mask": attention_mask,
"return_loss": False,
}
return config, inputs_dict
@require_torch
class OwlViTModelTest(ModelTesterMixin, PipelineTesterMixin, unittest.TestCase):
all_model_classes = (OwlViTModel,) if is_torch_available() else ()
pipeline_model_mapping = (
{"feature-extraction": OwlViTModel, "zero-shot-object-detection": OwlViTForObjectDetection}
if is_torch_available()
else {}
)
fx_compatible = False
test_head_masking = False
test_pruning = False
test_resize_embeddings = False
test_attention_outputs = False
def setUp(self):
self.model_tester = OwlViTModelTester(self)
def test_model(self):
config_and_inputs = self.model_tester.prepare_config_and_inputs()
self.model_tester.create_and_check_model(*config_and_inputs)
@unittest.skip(reason="Hidden_states is tested in individual model tests")
def test_hidden_states_output(self):
pass
@unittest.skip(reason="Inputs_embeds is tested in individual model tests")
def test_inputs_embeds(self):
pass
@unittest.skip(reason="Retain_grad is tested in individual model tests")
def test_retain_grad_hidden_states_attentions(self):
pass
@unittest.skip(reason="OwlViTModel does not have input/output embeddings")
def test_model_common_attributes(self):
pass
# override as the `logit_scale` parameter initilization is different for OWLVIT
def test_initialization(self):
config, inputs_dict = self.model_tester.prepare_config_and_inputs_for_common()
configs_no_init = _config_zero_init(config)
for model_class in self.all_model_classes:
model = model_class(config=configs_no_init)
for name, param in model.named_parameters():
if param.requires_grad:
# check if `logit_scale` is initilized as per the original implementation
if name == "logit_scale":
self.assertAlmostEqual(
param.data.item(),
np.log(1 / 0.07),
delta=1e-3,
msg=f"Parameter {name} of model {model_class} seems not properly initialized",
)
else:
self.assertIn(
((param.data.mean() * 1e9).round() / 1e9).item(),
[0.0, 1.0],
msg=f"Parameter {name} of model {model_class} seems not properly initialized",
)
def _create_and_check_torchscript(self, config, inputs_dict):
if not self.test_torchscript:
return
configs_no_init = _config_zero_init(config) # To be sure we have no Nan
configs_no_init.torchscript = True
configs_no_init.return_dict = False
for model_class in self.all_model_classes:
model = model_class(config=configs_no_init).to(torch_device)
model.eval()
try:
input_ids = inputs_dict["input_ids"]
pixel_values = inputs_dict["pixel_values"] # OWLVIT needs pixel_values
traced_model = torch.jit.trace(model, (input_ids, pixel_values))
except RuntimeError:
self.fail("Couldn't trace module.")
with tempfile.TemporaryDirectory() as tmp_dir_name:
pt_file_name = os.path.join(tmp_dir_name, "traced_model.pt")
try:
torch.jit.save(traced_model, pt_file_name)
except Exception:
self.fail("Couldn't save module.")
try:
loaded_model = torch.jit.load(pt_file_name)
except Exception:
self.fail("Couldn't load module.")
loaded_model = loaded_model.to(torch_device)
loaded_model.eval()
model_state_dict = model.state_dict()
loaded_model_state_dict = loaded_model.state_dict()
non_persistent_buffers = {}
for key in loaded_model_state_dict.keys():
if key not in model_state_dict.keys():
non_persistent_buffers[key] = loaded_model_state_dict[key]
loaded_model_state_dict = {
key: value for key, value in loaded_model_state_dict.items() if key not in non_persistent_buffers
}
self.assertEqual(set(model_state_dict.keys()), set(loaded_model_state_dict.keys()))
model_buffers = list(model.buffers())
for non_persistent_buffer in non_persistent_buffers.values():
found_buffer = False
for i, model_buffer in enumerate(model_buffers):
if torch.equal(non_persistent_buffer, model_buffer):
found_buffer = True
break
self.assertTrue(found_buffer)
model_buffers.pop(i)
models_equal = True
for layer_name, p1 in model_state_dict.items():
p2 = loaded_model_state_dict[layer_name]
if p1.data.ne(p2.data).sum() > 0:
models_equal = False
self.assertTrue(models_equal)
def test_load_vision_text_config(self):
config, inputs_dict = self.model_tester.prepare_config_and_inputs_for_common()
# Save OwlViTConfig and check if we can load OwlViTVisionConfig from it
with tempfile.TemporaryDirectory() as tmp_dir_name:
config.save_pretrained(tmp_dir_name)
vision_config = OwlViTVisionConfig.from_pretrained(tmp_dir_name)
self.assertDictEqual(config.vision_config.to_dict(), vision_config.to_dict())
# Save OwlViTConfig and check if we can load OwlViTTextConfig from it
with tempfile.TemporaryDirectory() as tmp_dir_name:
config.save_pretrained(tmp_dir_name)
text_config = OwlViTTextConfig.from_pretrained(tmp_dir_name)
self.assertDictEqual(config.text_config.to_dict(), text_config.to_dict())
@slow
def test_model_from_pretrained(self):
for model_name in OWLVIT_PRETRAINED_MODEL_ARCHIVE_LIST[:1]:
model = OwlViTModel.from_pretrained(model_name)
self.assertIsNotNone(model)
class OwlViTForObjectDetectionTester:
def __init__(self, parent, is_training=True):
self.parent = parent
self.text_model_tester = OwlViTTextModelTester(parent)
self.vision_model_tester = OwlViTVisionModelTester(parent)
self.is_training = is_training
self.text_config = self.text_model_tester.get_config().to_dict()
self.vision_config = self.vision_model_tester.get_config().to_dict()
def prepare_config_and_inputs(self):
text_config, input_ids, attention_mask = self.text_model_tester.prepare_config_and_inputs()
vision_config, pixel_values = self.vision_model_tester.prepare_config_and_inputs()
config = self.get_config()
return config, pixel_values, input_ids, attention_mask
def get_config(self):
return OwlViTConfig.from_text_vision_configs(self.text_config, self.vision_config, projection_dim=64)
def create_and_check_model(self, config, pixel_values, input_ids, attention_mask):
model = OwlViTForObjectDetection(config).to(torch_device).eval()
with torch.no_grad():
result = model(
pixel_values=pixel_values,
input_ids=input_ids,
attention_mask=attention_mask,
return_dict=True,
)
pred_boxes_size = (
self.vision_model_tester.batch_size,
(self.vision_model_tester.image_size // self.vision_model_tester.patch_size) ** 2,
4,
)
pred_logits_size = (
self.vision_model_tester.batch_size,
(self.vision_model_tester.image_size // self.vision_model_tester.patch_size) ** 2,
4,
)
pred_class_embeds_size = (
self.vision_model_tester.batch_size,
(self.vision_model_tester.image_size // self.vision_model_tester.patch_size) ** 2,
self.text_model_tester.hidden_size,
)
self.parent.assertEqual(result.pred_boxes.shape, pred_boxes_size)
self.parent.assertEqual(result.logits.shape, pred_logits_size)
self.parent.assertEqual(result.class_embeds.shape, pred_class_embeds_size)
def prepare_config_and_inputs_for_common(self):
config_and_inputs = self.prepare_config_and_inputs()
config, pixel_values, input_ids, attention_mask = config_and_inputs
inputs_dict = {
"pixel_values": pixel_values,
"input_ids": input_ids,
"attention_mask": attention_mask,
}
return config, inputs_dict
@require_torch
class OwlViTForObjectDetectionTest(ModelTesterMixin, unittest.TestCase):
all_model_classes = (OwlViTForObjectDetection,) if is_torch_available() else ()
fx_compatible = False
test_head_masking = False
test_pruning = False
test_resize_embeddings = False
test_attention_outputs = False
def setUp(self):
self.model_tester = OwlViTForObjectDetectionTester(self)
def test_model(self):
config_and_inputs = self.model_tester.prepare_config_and_inputs()
self.model_tester.create_and_check_model(*config_and_inputs)
@unittest.skip(reason="Hidden_states is tested in individual model tests")
def test_hidden_states_output(self):
pass
@unittest.skip(reason="Inputs_embeds is tested in individual model tests")
def test_inputs_embeds(self):
pass
@unittest.skip(reason="Retain_grad is tested in individual model tests")
def test_retain_grad_hidden_states_attentions(self):
pass
@unittest.skip(reason="OwlViTModel does not have input/output embeddings")
def test_model_common_attributes(self):
pass
@unittest.skip(reason="Test_initialization is tested in individual model tests")
def test_initialization(self):
pass
@unittest.skip(reason="Test_forward_signature is tested in individual model tests")
def test_forward_signature(self):
pass
@unittest.skip(reason="Test_save_load_fast_init_from_base is tested in individual model tests")
def test_save_load_fast_init_from_base(self):
pass
@unittest.skip(reason="OWL-ViT does not support training yet")
def test_training(self):
pass
@unittest.skip(reason="OWL-ViT does not support training yet")
def test_training_gradient_checkpointing(self):
pass
@unittest.skip(
reason="This architecure seem to not compute gradients properly when using GC, check: https://github.com/huggingface/transformers/pull/27124"
)
def test_training_gradient_checkpointing_use_reentrant(self):
pass
@unittest.skip(
reason="This architecure seem to not compute gradients properly when using GC, check: https://github.com/huggingface/transformers/pull/27124"
)
def test_training_gradient_checkpointing_use_reentrant_false(self):
pass
def _create_and_check_torchscript(self, config, inputs_dict):
if not self.test_torchscript:
return
configs_no_init = _config_zero_init(config) # To be sure we have no Nan
configs_no_init.torchscript = True
configs_no_init.return_dict = False
for model_class in self.all_model_classes:
model = model_class(config=configs_no_init).to(torch_device)
model.eval()
try:
input_ids = inputs_dict["input_ids"]
pixel_values = inputs_dict["pixel_values"] # OWLVIT needs pixel_values
traced_model = torch.jit.trace(model, (input_ids, pixel_values))
except RuntimeError:
self.fail("Couldn't trace module.")
with tempfile.TemporaryDirectory() as tmp_dir_name:
pt_file_name = os.path.join(tmp_dir_name, "traced_model.pt")
try:
torch.jit.save(traced_model, pt_file_name)
except Exception:
self.fail("Couldn't save module.")
try:
loaded_model = torch.jit.load(pt_file_name)
except Exception:
self.fail("Couldn't load module.")
loaded_model = loaded_model.to(torch_device)
loaded_model.eval()
model_state_dict = model.state_dict()
loaded_model_state_dict = loaded_model.state_dict()
non_persistent_buffers = {}
for key in loaded_model_state_dict.keys():
if key not in model_state_dict.keys():
non_persistent_buffers[key] = loaded_model_state_dict[key]
loaded_model_state_dict = {
key: value for key, value in loaded_model_state_dict.items() if key not in non_persistent_buffers
}
self.assertEqual(set(model_state_dict.keys()), set(loaded_model_state_dict.keys()))
model_buffers = list(model.buffers())
for non_persistent_buffer in non_persistent_buffers.values():
found_buffer = False
for i, model_buffer in enumerate(model_buffers):
if torch.equal(non_persistent_buffer, model_buffer):
found_buffer = True
break
self.assertTrue(found_buffer)
model_buffers.pop(i)
models_equal = True
for layer_name, p1 in model_state_dict.items():
p2 = loaded_model_state_dict[layer_name]
if p1.data.ne(p2.data).sum() > 0:
models_equal = False
self.assertTrue(models_equal)
@slow
def test_model_from_pretrained(self):
for model_name in OWLVIT_PRETRAINED_MODEL_ARCHIVE_LIST[:1]:
model = OwlViTForObjectDetection.from_pretrained(model_name)
self.assertIsNotNone(model)
# We will verify our results on an image of cute cats
def prepare_img():
url = "http://images.cocodataset.org/val2017/000000039769.jpg"
im = Image.open(requests.get(url, stream=True).raw)
return im
@require_vision
@require_torch
class OwlViTModelIntegrationTest(unittest.TestCase):
@slow
def test_inference(self):
model_name = "google/owlvit-base-patch32"
model = OwlViTModel.from_pretrained(model_name).to(torch_device)
processor = OwlViTProcessor.from_pretrained(model_name)
image = prepare_img()
inputs = processor(
text=[["a photo of a cat", "a photo of a dog"]],
images=image,
max_length=16,
padding="max_length",
return_tensors="pt",
).to(torch_device)
# forward pass
with torch.no_grad():
outputs = model(**inputs)
# verify the logits
self.assertEqual(
outputs.logits_per_image.shape,
torch.Size((inputs.pixel_values.shape[0], inputs.input_ids.shape[0])),
)
self.assertEqual(
outputs.logits_per_text.shape,
torch.Size((inputs.input_ids.shape[0], inputs.pixel_values.shape[0])),
)
expected_logits = torch.tensor([[3.4613, 0.9403]], device=torch_device)
self.assertTrue(torch.allclose(outputs.logits_per_image, expected_logits, atol=1e-3))
@slow
def test_inference_object_detection(self):
model_name = "google/owlvit-base-patch32"
model = OwlViTForObjectDetection.from_pretrained(model_name).to(torch_device)
processor = OwlViTProcessor.from_pretrained(model_name)
image = prepare_img()
inputs = processor(
text=[["a photo of a cat", "a photo of a dog"]],
images=image,
max_length=16,
padding="max_length",
return_tensors="pt",
).to(torch_device)
with torch.no_grad():
outputs = model(**inputs)
num_queries = int((model.config.vision_config.image_size / model.config.vision_config.patch_size) ** 2)
self.assertEqual(outputs.pred_boxes.shape, torch.Size((1, num_queries, 4)))
expected_slice_boxes = torch.tensor(
[[0.0691, 0.0445, 0.1373], [0.1592, 0.0456, 0.3192], [0.1632, 0.0423, 0.2478]]
).to(torch_device)
self.assertTrue(torch.allclose(outputs.pred_boxes[0, :3, :3], expected_slice_boxes, atol=1e-4))
@slow
def test_inference_one_shot_object_detection(self):
model_name = "google/owlvit-base-patch32"
model = OwlViTForObjectDetection.from_pretrained(model_name).to(torch_device)
processor = OwlViTProcessor.from_pretrained(model_name)
image = prepare_img()
query_image = prepare_img()
inputs = processor(
images=image,
query_images=query_image,
max_length=16,
padding="max_length",
return_tensors="pt",
).to(torch_device)
with torch.no_grad():
outputs = model.image_guided_detection(**inputs)
num_queries = int((model.config.vision_config.image_size / model.config.vision_config.patch_size) ** 2)
self.assertEqual(outputs.target_pred_boxes.shape, torch.Size((1, num_queries, 4)))
expected_slice_boxes = torch.tensor(
[[0.0691, 0.0445, 0.1373], [0.1592, 0.0456, 0.3192], [0.1632, 0.0423, 0.2478]]
).to(torch_device)
self.assertTrue(torch.allclose(outputs.target_pred_boxes[0, :3, :3], expected_slice_boxes, atol=1e-4))
@slow
@require_torch_accelerator
@require_torch_fp16
def test_inference_one_shot_object_detection_fp16(self):
model_name = "google/owlvit-base-patch32"
model = OwlViTForObjectDetection.from_pretrained(model_name, torch_dtype=torch.float16).to(torch_device)
processor = OwlViTProcessor.from_pretrained(model_name)
image = prepare_img()
query_image = prepare_img()
inputs = processor(
images=image,
query_images=query_image,
max_length=16,
padding="max_length",
return_tensors="pt",
).to(torch_device)
with torch.no_grad():
outputs = model.image_guided_detection(**inputs)
# No need to check the logits, we just check inference runs fine.
num_queries = int((model.config.vision_config.image_size / model.config.vision_config.patch_size) ** 2)
self.assertEqual(outputs.target_pred_boxes.shape, torch.Size((1, num_queries, 4)))
| transformers/tests/models/owlvit/test_modeling_owlvit.py/0 | {
"file_path": "transformers/tests/models/owlvit/test_modeling_owlvit.py",
"repo_id": "transformers",
"token_count": 15259
} | 361 |
# coding=utf-8
# Copyright 2022 The HuggingFace Team. All rights reserved.
#
# Licensed under the Apache License, Version 2.0 (the "License");
# you may not use this file except in compliance with the License.
# You may obtain a copy of the License at
#
# http://www.apache.org/licenses/LICENSE-2.0
#
# Unless required by applicable law or agreed to in writing, software
# distributed under the License is distributed on an "AS IS" BASIS,
# WITHOUT WARRANTIES OR CONDITIONS OF ANY KIND, either express or implied.
# See the License for the specific language governing permissions and
# limitations under the License.
from __future__ import annotations
import unittest
from transformers import RobertaPreLayerNormConfig, is_tf_available
from transformers.testing_utils import require_sentencepiece, require_tf, require_tokenizers, slow
from ...test_configuration_common import ConfigTester
from ...test_modeling_tf_common import TFModelTesterMixin, floats_tensor, ids_tensor, random_attention_mask
from ...test_pipeline_mixin import PipelineTesterMixin
if is_tf_available():
import numpy
import tensorflow as tf
from transformers.models.roberta_prelayernorm.modeling_tf_roberta_prelayernorm import (
TF_ROBERTA_PRELAYERNORM_PRETRAINED_MODEL_ARCHIVE_LIST,
TFRobertaPreLayerNormForCausalLM,
TFRobertaPreLayerNormForMaskedLM,
TFRobertaPreLayerNormForMultipleChoice,
TFRobertaPreLayerNormForQuestionAnswering,
TFRobertaPreLayerNormForSequenceClassification,
TFRobertaPreLayerNormForTokenClassification,
TFRobertaPreLayerNormModel,
)
# Copied from tests.models.roberta.test_modeling_tf_roberta.TFRobertaModelTester with Roberta->RobertaPreLayerNorm
class TFRobertaPreLayerNormModelTester:
def __init__(
self,
parent,
):
self.parent = parent
self.batch_size = 13
self.seq_length = 7
self.is_training = True
self.use_input_mask = True
self.use_token_type_ids = True
self.use_labels = True
self.vocab_size = 99
self.hidden_size = 32
self.num_hidden_layers = 2
self.num_attention_heads = 4
self.intermediate_size = 37
self.hidden_act = "gelu"
self.hidden_dropout_prob = 0.1
self.attention_probs_dropout_prob = 0.1
self.max_position_embeddings = 512
self.type_vocab_size = 16
self.type_sequence_label_size = 2
self.initializer_range = 0.02
self.num_labels = 3
self.num_choices = 4
self.scope = None
def prepare_config_and_inputs(self):
input_ids = ids_tensor([self.batch_size, self.seq_length], self.vocab_size)
input_mask = None
if self.use_input_mask:
input_mask = random_attention_mask([self.batch_size, self.seq_length])
token_type_ids = None
if self.use_token_type_ids:
token_type_ids = ids_tensor([self.batch_size, self.seq_length], self.type_vocab_size)
sequence_labels = None
token_labels = None
choice_labels = None
if self.use_labels:
sequence_labels = ids_tensor([self.batch_size], self.type_sequence_label_size)
token_labels = ids_tensor([self.batch_size, self.seq_length], self.num_labels)
choice_labels = ids_tensor([self.batch_size], self.num_choices)
config = RobertaPreLayerNormConfig(
vocab_size=self.vocab_size,
hidden_size=self.hidden_size,
num_hidden_layers=self.num_hidden_layers,
num_attention_heads=self.num_attention_heads,
intermediate_size=self.intermediate_size,
hidden_act=self.hidden_act,
hidden_dropout_prob=self.hidden_dropout_prob,
attention_probs_dropout_prob=self.attention_probs_dropout_prob,
max_position_embeddings=self.max_position_embeddings,
type_vocab_size=self.type_vocab_size,
initializer_range=self.initializer_range,
)
return config, input_ids, token_type_ids, input_mask, sequence_labels, token_labels, choice_labels
def prepare_config_and_inputs_for_decoder(self):
(
config,
input_ids,
token_type_ids,
input_mask,
sequence_labels,
token_labels,
choice_labels,
) = self.prepare_config_and_inputs()
config.is_decoder = True
encoder_hidden_states = floats_tensor([self.batch_size, self.seq_length, self.hidden_size])
encoder_attention_mask = ids_tensor([self.batch_size, self.seq_length], vocab_size=2)
return (
config,
input_ids,
token_type_ids,
input_mask,
sequence_labels,
token_labels,
choice_labels,
encoder_hidden_states,
encoder_attention_mask,
)
def create_and_check_model(
self, config, input_ids, token_type_ids, input_mask, sequence_labels, token_labels, choice_labels
):
model = TFRobertaPreLayerNormModel(config=config)
inputs = {"input_ids": input_ids, "attention_mask": input_mask, "token_type_ids": token_type_ids}
result = model(inputs)
inputs = [input_ids, input_mask]
result = model(inputs)
result = model(input_ids)
self.parent.assertEqual(result.last_hidden_state.shape, (self.batch_size, self.seq_length, self.hidden_size))
def create_and_check_causal_lm_base_model(
self, config, input_ids, token_type_ids, input_mask, sequence_labels, token_labels, choice_labels
):
config.is_decoder = True
model = TFRobertaPreLayerNormModel(config=config)
inputs = {"input_ids": input_ids, "attention_mask": input_mask, "token_type_ids": token_type_ids}
result = model(inputs)
inputs = [input_ids, input_mask]
result = model(inputs)
result = model(input_ids)
self.parent.assertEqual(result.last_hidden_state.shape, (self.batch_size, self.seq_length, self.hidden_size))
def create_and_check_model_as_decoder(
self,
config,
input_ids,
token_type_ids,
input_mask,
sequence_labels,
token_labels,
choice_labels,
encoder_hidden_states,
encoder_attention_mask,
):
config.add_cross_attention = True
model = TFRobertaPreLayerNormModel(config=config)
inputs = {
"input_ids": input_ids,
"attention_mask": input_mask,
"token_type_ids": token_type_ids,
"encoder_hidden_states": encoder_hidden_states,
"encoder_attention_mask": encoder_attention_mask,
}
result = model(inputs)
inputs = [input_ids, input_mask]
result = model(inputs, token_type_ids=token_type_ids, encoder_hidden_states=encoder_hidden_states)
# Also check the case where encoder outputs are not passed
result = model(input_ids, attention_mask=input_mask, token_type_ids=token_type_ids)
self.parent.assertEqual(result.last_hidden_state.shape, (self.batch_size, self.seq_length, self.hidden_size))
def create_and_check_causal_lm_model(
self, config, input_ids, token_type_ids, input_mask, sequence_labels, token_labels, choice_labels
):
config.is_decoder = True
model = TFRobertaPreLayerNormForCausalLM(config=config)
inputs = {
"input_ids": input_ids,
"attention_mask": input_mask,
"token_type_ids": token_type_ids,
}
prediction_scores = model(inputs)["logits"]
self.parent.assertListEqual(
list(prediction_scores.numpy().shape), [self.batch_size, self.seq_length, self.vocab_size]
)
def create_and_check_causal_lm_model_as_decoder(
self,
config,
input_ids,
token_type_ids,
input_mask,
sequence_labels,
token_labels,
choice_labels,
encoder_hidden_states,
encoder_attention_mask,
):
config.add_cross_attention = True
model = TFRobertaPreLayerNormForCausalLM(config=config)
inputs = {
"input_ids": input_ids,
"attention_mask": input_mask,
"token_type_ids": token_type_ids,
"encoder_hidden_states": encoder_hidden_states,
"encoder_attention_mask": encoder_attention_mask,
}
result = model(inputs)
inputs = [input_ids, input_mask]
result = model(inputs, token_type_ids=token_type_ids, encoder_hidden_states=encoder_hidden_states)
prediction_scores = result["logits"]
self.parent.assertListEqual(
list(prediction_scores.numpy().shape), [self.batch_size, self.seq_length, self.vocab_size]
)
def create_and_check_causal_lm_model_past(
self,
config,
input_ids,
token_type_ids,
input_mask,
sequence_labels,
token_labels,
choice_labels,
):
config.is_decoder = True
model = TFRobertaPreLayerNormForCausalLM(config=config)
# special to `RobertaPreLayerNormEmbeddings` in `RobertaPreLayerNorm`:
# - its `padding_idx` and its effect on `position_ids`
# (TFRobertaPreLayerNormEmbeddings.create_position_ids_from_input_ids)
# - `1` here is `TFRobertaPreLayerNormEmbeddings.padding_idx`
input_ids = tf.where(input_ids == 1, 2, input_ids)
# first forward pass
outputs = model(input_ids, use_cache=True)
outputs_use_cache_conf = model(input_ids)
outputs_no_past = model(input_ids, use_cache=False)
self.parent.assertTrue(len(outputs) == len(outputs_use_cache_conf))
self.parent.assertTrue(len(outputs) == len(outputs_no_past) + 1)
past_key_values = outputs.past_key_values
# create hypothetical next token and extent to next_input_ids
next_tokens = ids_tensor((self.batch_size, 1), config.vocab_size)
# append to next input_ids and attn_mask
next_input_ids = tf.concat([input_ids, next_tokens], axis=-1)
output_from_no_past = model(next_input_ids, output_hidden_states=True).hidden_states[0]
output_from_past = model(
next_tokens, past_key_values=past_key_values, output_hidden_states=True
).hidden_states[0]
# select random slice
random_slice_idx = int(ids_tensor((1,), output_from_past.shape[-1]))
output_from_no_past_slice = output_from_no_past[:, -1, random_slice_idx]
output_from_past_slice = output_from_past[:, 0, random_slice_idx]
# test that outputs are equal for slice
tf.debugging.assert_near(output_from_past_slice, output_from_no_past_slice, rtol=1e-6)
def create_and_check_causal_lm_model_past_with_attn_mask(
self,
config,
input_ids,
token_type_ids,
input_mask,
sequence_labels,
token_labels,
choice_labels,
):
config.is_decoder = True
model = TFRobertaPreLayerNormForCausalLM(config=config)
# special to `RobertaPreLayerNormEmbeddings` in `RobertaPreLayerNorm`:
# - its `padding_idx` and its effect on `position_ids`
# (TFRobertaPreLayerNormEmbeddings.create_position_ids_from_input_ids)
# - `1` here is `TFRobertaPreLayerNormEmbeddings.padding_idx`
# avoid `padding_idx` in the past
input_ids = tf.where(input_ids == 1, 2, input_ids)
# create attention mask
half_seq_length = self.seq_length // 2
attn_mask_begin = tf.ones((self.batch_size, half_seq_length), dtype=tf.int32)
attn_mask_end = tf.zeros((self.batch_size, self.seq_length - half_seq_length), dtype=tf.int32)
attn_mask = tf.concat([attn_mask_begin, attn_mask_end], axis=1)
# first forward pass
outputs = model(input_ids, attention_mask=attn_mask, use_cache=True)
# create hypothetical next token and extent to next_input_ids
next_tokens = ids_tensor((self.batch_size, 1), config.vocab_size)
past_key_values = outputs.past_key_values
# change a random masked slice from input_ids
random_seq_idx_to_change = ids_tensor((1,), half_seq_length).numpy() + 1
random_other_next_tokens = ids_tensor((self.batch_size, self.seq_length), config.vocab_size)
vector_condition = tf.range(self.seq_length) == (self.seq_length - random_seq_idx_to_change)
condition = tf.transpose(
tf.broadcast_to(tf.expand_dims(vector_condition, -1), (self.seq_length, self.batch_size))
)
input_ids = tf.where(condition, random_other_next_tokens, input_ids)
# avoid `padding_idx` in the past
input_ids = tf.where(input_ids == 1, 2, input_ids)
# append to next input_ids and
next_input_ids = tf.concat([input_ids, next_tokens], axis=-1)
attn_mask = tf.concat(
[attn_mask, tf.ones((attn_mask.shape[0], 1), dtype=tf.int32)],
axis=1,
)
output_from_no_past = model(
next_input_ids,
attention_mask=attn_mask,
output_hidden_states=True,
).hidden_states[0]
output_from_past = model(
next_tokens, past_key_values=past_key_values, attention_mask=attn_mask, output_hidden_states=True
).hidden_states[0]
# select random slice
random_slice_idx = int(ids_tensor((1,), output_from_past.shape[-1]))
output_from_no_past_slice = output_from_no_past[:, -1, random_slice_idx]
output_from_past_slice = output_from_past[:, 0, random_slice_idx]
# test that outputs are equal for slice
tf.debugging.assert_near(output_from_past_slice, output_from_no_past_slice, rtol=1e-6)
def create_and_check_causal_lm_model_past_large_inputs(
self,
config,
input_ids,
token_type_ids,
input_mask,
sequence_labels,
token_labels,
choice_labels,
):
config.is_decoder = True
model = TFRobertaPreLayerNormForCausalLM(config=config)
# special to `RobertaPreLayerNormEmbeddings` in `RobertaPreLayerNorm`:
# - its `padding_idx` and its effect on `position_ids`
# (TFRobertaPreLayerNormEmbeddings.create_position_ids_from_input_ids)
# - `1` here is `TFRobertaPreLayerNormEmbeddings.padding_idx`
# avoid `padding_idx` in the past
input_ids = tf.where(input_ids == 1, 2, input_ids)
input_ids = input_ids[:1, :]
input_mask = input_mask[:1, :]
self.batch_size = 1
# first forward pass
outputs = model(input_ids, attention_mask=input_mask, use_cache=True)
past_key_values = outputs.past_key_values
# create hypothetical next token and extent to next_input_ids
next_tokens = ids_tensor((self.batch_size, 3), config.vocab_size)
next_attn_mask = ids_tensor((self.batch_size, 3), 2)
# append to next input_ids and
next_input_ids = tf.concat([input_ids, next_tokens], axis=-1)
next_attention_mask = tf.concat([input_mask, next_attn_mask], axis=-1)
output_from_no_past = model(
next_input_ids,
attention_mask=next_attention_mask,
output_hidden_states=True,
).hidden_states[0]
output_from_past = model(
next_tokens,
attention_mask=next_attention_mask,
past_key_values=past_key_values,
output_hidden_states=True,
).hidden_states[0]
self.parent.assertEqual(next_tokens.shape[1], output_from_past.shape[1])
# select random slice
random_slice_idx = int(ids_tensor((1,), output_from_past.shape[-1]))
output_from_no_past_slice = output_from_no_past[:, -3:, random_slice_idx]
output_from_past_slice = output_from_past[:, :, random_slice_idx]
# test that outputs are equal for slice
tf.debugging.assert_near(output_from_past_slice, output_from_no_past_slice, rtol=1e-3)
def create_and_check_decoder_model_past_large_inputs(
self,
config,
input_ids,
token_type_ids,
input_mask,
sequence_labels,
token_labels,
choice_labels,
encoder_hidden_states,
encoder_attention_mask,
):
config.add_cross_attention = True
model = TFRobertaPreLayerNormForCausalLM(config=config)
# special to `RobertaPreLayerNormEmbeddings` in `RobertaPreLayerNorm`:
# - its `padding_idx` and its effect on `position_ids`
# (TFRobertaPreLayerNormEmbeddings.create_position_ids_from_input_ids)
# - `1` here is `TFRobertaPreLayerNormEmbeddings.padding_idx`
# avoid `padding_idx` in the past
input_ids = tf.where(input_ids == 1, 2, input_ids)
input_ids = input_ids[:1, :]
input_mask = input_mask[:1, :]
encoder_hidden_states = encoder_hidden_states[:1, :, :]
encoder_attention_mask = encoder_attention_mask[:1, :]
self.batch_size = 1
# first forward pass
outputs = model(
input_ids,
attention_mask=input_mask,
encoder_hidden_states=encoder_hidden_states,
encoder_attention_mask=encoder_attention_mask,
use_cache=True,
)
past_key_values = outputs.past_key_values
# create hypothetical next token and extent to next_input_ids
next_tokens = ids_tensor((self.batch_size, 3), config.vocab_size)
next_attn_mask = ids_tensor((self.batch_size, 3), 2)
# append to next input_ids and
next_input_ids = tf.concat([input_ids, next_tokens], axis=-1)
next_attention_mask = tf.concat([input_mask, next_attn_mask], axis=-1)
output_from_no_past = model(
next_input_ids,
attention_mask=next_attention_mask,
encoder_hidden_states=encoder_hidden_states,
encoder_attention_mask=encoder_attention_mask,
output_hidden_states=True,
).hidden_states[0]
output_from_past = model(
next_tokens,
attention_mask=next_attention_mask,
encoder_hidden_states=encoder_hidden_states,
encoder_attention_mask=encoder_attention_mask,
past_key_values=past_key_values,
output_hidden_states=True,
).hidden_states[0]
self.parent.assertEqual(next_tokens.shape[1], output_from_past.shape[1])
# select random slice
random_slice_idx = int(ids_tensor((1,), output_from_past.shape[-1]))
output_from_no_past_slice = output_from_no_past[:, -3:, random_slice_idx]
output_from_past_slice = output_from_past[:, :, random_slice_idx]
# test that outputs are equal for slice
tf.debugging.assert_near(output_from_past_slice, output_from_no_past_slice, rtol=1e-3)
def create_and_check_for_masked_lm(
self, config, input_ids, token_type_ids, input_mask, sequence_labels, token_labels, choice_labels
):
model = TFRobertaPreLayerNormForMaskedLM(config=config)
result = model([input_ids, input_mask, token_type_ids])
self.parent.assertEqual(result.logits.shape, (self.batch_size, self.seq_length, self.vocab_size))
def create_and_check_for_token_classification(
self, config, input_ids, token_type_ids, input_mask, sequence_labels, token_labels, choice_labels
):
config.num_labels = self.num_labels
model = TFRobertaPreLayerNormForTokenClassification(config=config)
inputs = {"input_ids": input_ids, "attention_mask": input_mask, "token_type_ids": token_type_ids}
result = model(inputs)
self.parent.assertEqual(result.logits.shape, (self.batch_size, self.seq_length, self.num_labels))
def create_and_check_for_question_answering(
self, config, input_ids, token_type_ids, input_mask, sequence_labels, token_labels, choice_labels
):
model = TFRobertaPreLayerNormForQuestionAnswering(config=config)
inputs = {"input_ids": input_ids, "attention_mask": input_mask, "token_type_ids": token_type_ids}
result = model(inputs)
self.parent.assertEqual(result.start_logits.shape, (self.batch_size, self.seq_length))
self.parent.assertEqual(result.end_logits.shape, (self.batch_size, self.seq_length))
def create_and_check_for_multiple_choice(
self, config, input_ids, token_type_ids, input_mask, sequence_labels, token_labels, choice_labels
):
config.num_choices = self.num_choices
model = TFRobertaPreLayerNormForMultipleChoice(config=config)
multiple_choice_inputs_ids = tf.tile(tf.expand_dims(input_ids, 1), (1, self.num_choices, 1))
multiple_choice_input_mask = tf.tile(tf.expand_dims(input_mask, 1), (1, self.num_choices, 1))
multiple_choice_token_type_ids = tf.tile(tf.expand_dims(token_type_ids, 1), (1, self.num_choices, 1))
inputs = {
"input_ids": multiple_choice_inputs_ids,
"attention_mask": multiple_choice_input_mask,
"token_type_ids": multiple_choice_token_type_ids,
}
result = model(inputs)
self.parent.assertEqual(result.logits.shape, (self.batch_size, self.num_choices))
def prepare_config_and_inputs_for_common(self):
config_and_inputs = self.prepare_config_and_inputs()
(
config,
input_ids,
token_type_ids,
input_mask,
sequence_labels,
token_labels,
choice_labels,
) = config_and_inputs
inputs_dict = {"input_ids": input_ids, "token_type_ids": token_type_ids, "attention_mask": input_mask}
return config, inputs_dict
@require_tf
# Copied from tests.models.roberta.test_modeling_tf_roberta.TFRobertaModelTest with ROBERTA->ROBERTA_PRELAYERNORM,Roberta->RobertaPreLayerNorm
class TFRobertaPreLayerNormModelTest(TFModelTesterMixin, PipelineTesterMixin, unittest.TestCase):
all_model_classes = (
(
TFRobertaPreLayerNormModel,
TFRobertaPreLayerNormForCausalLM,
TFRobertaPreLayerNormForMaskedLM,
TFRobertaPreLayerNormForSequenceClassification,
TFRobertaPreLayerNormForTokenClassification,
TFRobertaPreLayerNormForQuestionAnswering,
)
if is_tf_available()
else ()
)
pipeline_model_mapping = (
{
"feature-extraction": TFRobertaPreLayerNormModel,
"fill-mask": TFRobertaPreLayerNormForMaskedLM,
"question-answering": TFRobertaPreLayerNormForQuestionAnswering,
"text-classification": TFRobertaPreLayerNormForSequenceClassification,
"text-generation": TFRobertaPreLayerNormForCausalLM,
"token-classification": TFRobertaPreLayerNormForTokenClassification,
"zero-shot": TFRobertaPreLayerNormForSequenceClassification,
}
if is_tf_available()
else {}
)
test_head_masking = False
test_onnx = False
def setUp(self):
self.model_tester = TFRobertaPreLayerNormModelTester(self)
self.config_tester = ConfigTester(self, config_class=RobertaPreLayerNormConfig, hidden_size=37)
def test_config(self):
self.config_tester.run_common_tests()
def test_model(self):
"""Test the base model"""
config_and_inputs = self.model_tester.prepare_config_and_inputs()
self.model_tester.create_and_check_model(*config_and_inputs)
def test_causal_lm_base_model(self):
"""Test the base model of the causal LM model
is_deocder=True, no cross_attention, no encoder outputs
"""
config_and_inputs = self.model_tester.prepare_config_and_inputs()
self.model_tester.create_and_check_causal_lm_base_model(*config_and_inputs)
def test_model_as_decoder(self):
"""Test the base model as a decoder (of an encoder-decoder architecture)
is_deocder=True + cross_attention + pass encoder outputs
"""
config_and_inputs = self.model_tester.prepare_config_and_inputs_for_decoder()
self.model_tester.create_and_check_model_as_decoder(*config_and_inputs)
def test_for_masked_lm(self):
config_and_inputs = self.model_tester.prepare_config_and_inputs()
self.model_tester.create_and_check_for_masked_lm(*config_and_inputs)
def test_for_causal_lm(self):
"""Test the causal LM model"""
config_and_inputs = self.model_tester.prepare_config_and_inputs()
self.model_tester.create_and_check_causal_lm_model(*config_and_inputs)
def test_causal_lm_model_as_decoder(self):
"""Test the causal LM model as a decoder"""
config_and_inputs = self.model_tester.prepare_config_and_inputs_for_decoder()
self.model_tester.create_and_check_causal_lm_model_as_decoder(*config_and_inputs)
def test_causal_lm_model_past(self):
"""Test causal LM model with `past_key_values`"""
config_and_inputs = self.model_tester.prepare_config_and_inputs()
self.model_tester.create_and_check_causal_lm_model_past(*config_and_inputs)
def test_causal_lm_model_past_with_attn_mask(self):
"""Test the causal LM model with `past_key_values` and `attention_mask`"""
config_and_inputs = self.model_tester.prepare_config_and_inputs()
self.model_tester.create_and_check_causal_lm_model_past_with_attn_mask(*config_and_inputs)
def test_causal_lm_model_past_with_large_inputs(self):
"""Test the causal LM model with `past_key_values` and a longer decoder sequence length"""
config_and_inputs = self.model_tester.prepare_config_and_inputs()
self.model_tester.create_and_check_causal_lm_model_past_large_inputs(*config_and_inputs)
def test_decoder_model_past_with_large_inputs(self):
"""Similar to `test_causal_lm_model_past_with_large_inputs` but with cross-attention"""
config_and_inputs = self.model_tester.prepare_config_and_inputs_for_decoder()
self.model_tester.create_and_check_decoder_model_past_large_inputs(*config_and_inputs)
def test_for_token_classification(self):
config_and_inputs = self.model_tester.prepare_config_and_inputs()
self.model_tester.create_and_check_for_token_classification(*config_and_inputs)
def test_for_question_answering(self):
config_and_inputs = self.model_tester.prepare_config_and_inputs()
self.model_tester.create_and_check_for_question_answering(*config_and_inputs)
def test_for_multiple_choice(self):
config_and_inputs = self.model_tester.prepare_config_and_inputs()
self.model_tester.create_and_check_for_multiple_choice(*config_and_inputs)
@slow
def test_model_from_pretrained(self):
for model_name in TF_ROBERTA_PRELAYERNORM_PRETRAINED_MODEL_ARCHIVE_LIST[:1]:
model = TFRobertaPreLayerNormModel.from_pretrained(model_name)
self.assertIsNotNone(model)
@require_tf
@require_sentencepiece
@require_tokenizers
class TFRobertaPreLayerNormModelIntegrationTest(unittest.TestCase):
@slow
def test_inference_masked_lm(self):
model = TFRobertaPreLayerNormForMaskedLM.from_pretrained("andreasmadsen/efficient_mlm_m0.40")
input_ids = tf.constant([[0, 31414, 232, 328, 740, 1140, 12695, 69, 46078, 1588, 2]])
output = model(input_ids)[0]
expected_shape = [1, 11, 50265]
self.assertEqual(list(output.numpy().shape), expected_shape)
# compare the actual values for a slice.
EXPECTED_SLICE = tf.constant(
[[[40.4880, 18.0199, -5.2367], [-1.8877, -4.0885, 10.7085], [-2.2613, -5.6110, 7.2665]]]
)
self.assertTrue(numpy.allclose(output[:, :3, :3].numpy(), EXPECTED_SLICE.numpy(), atol=1e-4))
@slow
def test_inference_no_head(self):
model = TFRobertaPreLayerNormModel.from_pretrained("andreasmadsen/efficient_mlm_m0.40")
input_ids = tf.constant([[0, 31414, 232, 328, 740, 1140, 12695, 69, 46078, 1588, 2]])
output = model(input_ids)[0]
# compare the actual values for a slice.
EXPECTED_SLICE = tf.constant(
[[[0.0208, -0.0356, 0.0237], [-0.1569, -0.0411, -0.2626], [0.1879, 0.0125, -0.0089]]]
)
self.assertTrue(numpy.allclose(output[:, :3, :3].numpy(), EXPECTED_SLICE.numpy(), atol=1e-4))
| transformers/tests/models/roberta_prelayernorm/test_modeling_tf_roberta_prelayernorm.py/0 | {
"file_path": "transformers/tests/models/roberta_prelayernorm/test_modeling_tf_roberta_prelayernorm.py",
"repo_id": "transformers",
"token_count": 12806
} | 362 |
# coding=utf-8
# Copyright 2023 HuggingFace Inc.
#
# Licensed under the Apache License, Version 2.0 (the "License");
# you may not use this file except in compliance with the License.
# You may obtain a copy of the License at
#
# http://www.apache.org/licenses/LICENSE-2.0
#
# Unless required by applicable law or agreed to in writing, software
# distributed under the License is distributed on an "AS IS" BASIS,
# WITHOUT WARRANTIES OR CONDITIONS OF ANY KIND, either express or implied.
# See the License for the specific language governing permissions and
# limitations under the License.
import itertools
import os
import random
import tempfile
import unittest
import numpy as np
from datasets import load_dataset
from transformers import SeamlessM4TFeatureExtractor, is_speech_available
from transformers.testing_utils import check_json_file_has_correct_format, require_torch
from transformers.utils.import_utils import is_torch_available
from ...test_sequence_feature_extraction_common import SequenceFeatureExtractionTestMixin
if is_torch_available():
import torch
global_rng = random.Random()
# Copied from tests.models.whisper.test_feature_extraction_whisper.floats_list
def floats_list(shape, scale=1.0, rng=None, name=None):
"""Creates a random float32 tensor"""
if rng is None:
rng = global_rng
values = []
for batch_idx in range(shape[0]):
values.append([])
for _ in range(shape[1]):
values[-1].append(rng.random() * scale)
return values
@require_torch
class SeamlessM4TFeatureExtractionTester(unittest.TestCase):
def __init__(
self,
parent,
batch_size=7,
min_seq_length=400,
max_seq_length=2000,
feature_size=10,
padding_value=0.0,
sampling_rate=4_000,
return_attention_mask=True,
do_normalize=True,
stride=2,
):
self.parent = parent
self.batch_size = batch_size
self.min_seq_length = min_seq_length
self.max_seq_length = max_seq_length
self.seq_length_diff = (self.max_seq_length - self.min_seq_length) // (self.batch_size - 1)
self.padding_value = padding_value
self.sampling_rate = sampling_rate
self.return_attention_mask = return_attention_mask
self.do_normalize = do_normalize
self.feature_size = feature_size
self.stride = stride
self.num_mel_bins = feature_size
def prepare_feat_extract_dict(self):
return {
"feature_size": self.feature_size,
"num_mel_bins": self.num_mel_bins,
"padding_value": self.padding_value,
"sampling_rate": self.sampling_rate,
"stride": self.stride,
"return_attention_mask": self.return_attention_mask,
"do_normalize": self.do_normalize,
}
# Copied from tests.models.whisper.test_feature_extraction_whisper.WhisperFeatureExtractionTester.prepare_inputs_for_common
def prepare_inputs_for_common(self, equal_length=False, numpify=False):
def _flatten(list_of_lists):
return list(itertools.chain(*list_of_lists))
if equal_length:
speech_inputs = [floats_list((self.max_seq_length, self.feature_size)) for _ in range(self.batch_size)]
else:
# make sure that inputs increase in size
speech_inputs = [
floats_list((x, self.feature_size))
for x in range(self.min_seq_length, self.max_seq_length, self.seq_length_diff)
]
if numpify:
speech_inputs = [np.asarray(x) for x in speech_inputs]
return speech_inputs
@require_torch
class SeamlessM4TFeatureExtractionTest(SequenceFeatureExtractionTestMixin, unittest.TestCase):
feature_extraction_class = SeamlessM4TFeatureExtractor if is_speech_available() else None
def setUp(self):
self.feat_extract_tester = SeamlessM4TFeatureExtractionTester(self)
def test_feat_extract_from_and_save_pretrained(self):
feat_extract_first = self.feature_extraction_class(**self.feat_extract_dict)
with tempfile.TemporaryDirectory() as tmpdirname:
saved_file = feat_extract_first.save_pretrained(tmpdirname)[0]
check_json_file_has_correct_format(saved_file)
feat_extract_second = self.feature_extraction_class.from_pretrained(tmpdirname)
dict_first = feat_extract_first.to_dict()
dict_second = feat_extract_second.to_dict()
self.assertDictEqual(dict_first, dict_second)
def test_feat_extract_to_json_file(self):
feat_extract_first = self.feature_extraction_class(**self.feat_extract_dict)
with tempfile.TemporaryDirectory() as tmpdirname:
json_file_path = os.path.join(tmpdirname, "feat_extract.json")
feat_extract_first.to_json_file(json_file_path)
feat_extract_second = self.feature_extraction_class.from_json_file(json_file_path)
dict_first = feat_extract_first.to_dict()
dict_second = feat_extract_second.to_dict()
self.assertEqual(dict_first, dict_second)
def test_call_numpy(self):
# Tests that all call wrap to encode_plus and batch_encode_plus
feature_extractor = self.feature_extraction_class(**self.feat_extract_tester.prepare_feat_extract_dict())
# create three inputs of length 800, 1000, and 1200
speech_inputs = [floats_list((1, x))[0] for x in range(800, 1400, 200)]
np_speech_inputs = [np.asarray(speech_input) for speech_input in speech_inputs]
# Test feature size
input_features = feature_extractor(np_speech_inputs, padding=True, return_tensors="np").input_features
self.assertTrue(input_features.ndim == 3)
self.assertTrue(input_features.shape[0] == 3)
self.assertTrue(input_features.shape[-1] == feature_extractor.feature_size * feature_extractor.stride)
# Test not batched input
encoded_sequences_1 = feature_extractor(speech_inputs[0], return_tensors="np").input_features
encoded_sequences_2 = feature_extractor(np_speech_inputs[0], return_tensors="np").input_features
self.assertTrue(np.allclose(encoded_sequences_1, encoded_sequences_2, atol=1e-3))
# Test batched
encoded_sequences_1 = feature_extractor(speech_inputs, return_tensors="np").input_features
encoded_sequences_2 = feature_extractor(np_speech_inputs, return_tensors="np").input_features
for enc_seq_1, enc_seq_2 in zip(encoded_sequences_1, encoded_sequences_2):
self.assertTrue(np.allclose(enc_seq_1, enc_seq_2, atol=1e-3))
# Test 2-D numpy arrays are batched.
speech_inputs = [floats_list((1, x))[0] for x in (800, 800, 800)]
np_speech_inputs = np.asarray(speech_inputs)
encoded_sequences_1 = feature_extractor(speech_inputs, return_tensors="np").input_features
encoded_sequences_2 = feature_extractor(np_speech_inputs, return_tensors="np").input_features
for enc_seq_1, enc_seq_2 in zip(encoded_sequences_1, encoded_sequences_2):
self.assertTrue(np.allclose(enc_seq_1, enc_seq_2, atol=1e-3))
def test_call_without_attention_mask(self):
feature_extractor_args = self.feat_extract_tester.prepare_feat_extract_dict()
feature_extractor = self.feature_extraction_class(**feature_extractor_args)
# create three inputs of length 800, 1000, and 1200
speech_inputs = [floats_list((1, x))[0] for x in range(800, 1400, 200)]
np_speech_inputs = [np.asarray(speech_input) for speech_input in speech_inputs]
# Test attention mask when passing no attention mask to forward call
output = feature_extractor(np_speech_inputs, padding=True, return_tensors="np", return_attention_mask=False)
self.assertTrue("attention_mask" not in output)
# Test attention mask when no attention mask by default
feature_extractor_args["return_attention_mask"] = False
feature_extractor = self.feature_extraction_class(**feature_extractor_args)
output = feature_extractor(np_speech_inputs, padding=True, return_tensors="np", return_attention_mask=False)
self.assertTrue("attention_mask" not in output)
def test_attention_mask(self):
# test attention mask has the right output shape
feature_extractor_args = self.feat_extract_tester.prepare_feat_extract_dict()
feature_extractor = self.feature_extraction_class(**feature_extractor_args)
# create three inputs of length 800, 1000, and 1200
speech_inputs = [floats_list((1, x))[0] for x in range(800, 1400, 200)]
np_speech_inputs = [np.asarray(speech_input) for speech_input in speech_inputs]
# Test attention mask when passing it to forward call
output = feature_extractor(np_speech_inputs, padding=True, return_tensors="np")
input_features = output.input_features
attention_mask = output.attention_mask
self.assertTrue(attention_mask.ndim == 2)
self.assertTrue(attention_mask.shape[0] == 3)
self.assertTrue(attention_mask.shape[-1] == input_features.shape[1])
@require_torch
def test_call_torch(self):
import torch
# Tests that all call wrap to encode_plus and batch_encode_plus
feature_extractor = self.feature_extraction_class(**self.feat_extract_tester.prepare_feat_extract_dict())
# create three inputs of length 800, 1000, and 1200
speech_inputs = [floats_list((1, x))[0] for x in range(800, 1400, 200)]
pt_speech_inputs = [torch.tensor(speech_input) for speech_input in speech_inputs]
# Test feature size
input_features = feature_extractor(pt_speech_inputs, padding=True, return_tensors="pt").input_features
self.assertTrue(input_features.ndim == 3)
self.assertTrue(input_features.shape[0] == 3)
self.assertTrue(input_features.shape[-1] == feature_extractor.feature_size * feature_extractor.stride)
# Test not batched input
encoded_sequences_1 = feature_extractor(speech_inputs[0], return_tensors="pt").input_features
encoded_sequences_2 = feature_extractor(pt_speech_inputs[0], return_tensors="pt").input_features
self.assertTrue(torch.allclose(encoded_sequences_1, encoded_sequences_2, atol=1e-3))
# Test batched
encoded_sequences_1 = feature_extractor(speech_inputs, return_tensors="pt").input_features
encoded_sequences_2 = feature_extractor(pt_speech_inputs, return_tensors="pt").input_features
for enc_seq_1, enc_seq_2 in zip(encoded_sequences_1, encoded_sequences_2):
self.assertTrue(torch.allclose(enc_seq_1, enc_seq_2, atol=1e-3))
# Test 2-D numpy arrays are batched.
speech_inputs = [floats_list((1, x))[0] for x in (800, 800, 800)]
pt_speech_inputs = torch.tensor(speech_inputs)
encoded_sequences_1 = feature_extractor(speech_inputs, return_tensors="pt").input_features
encoded_sequences_2 = feature_extractor(pt_speech_inputs, return_tensors="pt").input_features
for enc_seq_1, enc_seq_2 in zip(encoded_sequences_1, encoded_sequences_2):
self.assertTrue(torch.allclose(enc_seq_1, enc_seq_2, atol=1e-3))
@require_torch
# Copied from tests.models.whisper.test_feature_extraction_whisper.WhisperFeatureExtractionTest.test_double_precision_pad
def test_double_precision_pad(self):
import torch
feature_extractor = self.feature_extraction_class(**self.feat_extract_tester.prepare_feat_extract_dict())
np_speech_inputs = np.random.rand(100, 32).astype(np.float64)
py_speech_inputs = np_speech_inputs.tolist()
for inputs in [py_speech_inputs, np_speech_inputs]:
np_processed = feature_extractor.pad([{"input_features": inputs}], return_tensors="np")
self.assertTrue(np_processed.input_features.dtype == np.float32)
pt_processed = feature_extractor.pad([{"input_features": inputs}], return_tensors="pt")
self.assertTrue(pt_processed.input_features.dtype == torch.float32)
def _load_datasample(self, id):
ds = load_dataset("hf-internal-testing/librispeech_asr_dummy", "clean", split="validation")
# automatic decoding with librispeech
speech_sample = ds.sort("id")[id]["audio"]["array"]
return torch.from_numpy(speech_sample).unsqueeze(0)
def test_integration(self):
# fmt: off
EXPECTED_INPUT_FEATURES = torch.tensor(
[
-1.5621, -1.4236, -1.3335, -1.3991, -1.2881, -1.1133, -0.9710, -0.8895,
-0.8280, -0.7376, -0.7194, -0.6896, -0.6849, -0.6788, -0.6545, -0.6610,
-0.6566, -0.5738, -0.5252, -0.5533, -0.5887, -0.6116, -0.5971, -0.4956,
-0.2881, -0.1512, 0.0299, 0.1762, 0.2728, 0.2236
]
)
# fmt: on
input_speech = self._load_datasample(10)
feature_extractor = SeamlessM4TFeatureExtractor()
input_features = feature_extractor(input_speech, return_tensors="pt").input_features
feature_extractor(input_speech, return_tensors="pt").input_features[0, 5, :30]
self.assertEqual(input_features.shape, (1, 279, 160))
self.assertTrue(torch.allclose(input_features[0, 5, :30], EXPECTED_INPUT_FEATURES, atol=1e-4))
def test_zero_mean_unit_variance_normalization_trunc_np_longest(self):
feat_extract = self.feature_extraction_class(**self.feat_extract_tester.prepare_feat_extract_dict())
audio = self._load_datasample(1)
audio = ((audio - audio.min()) / (audio.max() - audio.min())) * 65535 # Rescale to [0, 65535] to show issue
audio = feat_extract.zero_mean_unit_var_norm([audio], attention_mask=None)[0]
self.assertTrue((audio.mean() < 1e-3).all())
self.assertTrue(((audio.var() - 1).abs() < 1e-3).all())
| transformers/tests/models/seamless_m4t/test_feature_extraction_seamless_m4t.py/0 | {
"file_path": "transformers/tests/models/seamless_m4t/test_feature_extraction_seamless_m4t.py",
"repo_id": "transformers",
"token_count": 5810
} | 363 |
# coding=utf-8
# Copyright 2024 The HuggingFace Inc. team. All rights reserved.
#
# Licensed under the Apache License, Version 2.0 (the "License");
# you may not use this file except in compliance with the License.
# You may obtain a copy of the License at
#
# http://www.apache.org/licenses/LICENSE-2.0
#
# Unless required by applicable law or agreed to in writing, software
# distributed under the License is distributed on an "AS IS" BASIS,
# WITHOUT WARRANTIES OR CONDITIONS OF ANY KIND, either express or implied.
# See the License for the specific language governing permissions and
# limitations under the License.
""" Testing suite for the PyTorch Siglip model. """
import inspect
import os
import tempfile
import unittest
import numpy as np
import requests
from transformers import SiglipConfig, SiglipTextConfig, SiglipVisionConfig
from transformers.testing_utils import (
require_torch,
require_vision,
slow,
torch_device,
)
from transformers.utils import is_torch_available, is_vision_available
from ...test_configuration_common import ConfigTester
from ...test_modeling_common import (
ModelTesterMixin,
_config_zero_init,
floats_tensor,
ids_tensor,
random_attention_mask,
)
from ...test_pipeline_mixin import PipelineTesterMixin
if is_torch_available():
import torch
from torch import nn
from transformers import SiglipModel, SiglipTextModel, SiglipVisionModel
from transformers.models.siglip.modeling_siglip import SIGLIP_PRETRAINED_MODEL_ARCHIVE_LIST
if is_vision_available():
from PIL import Image
from transformers import SiglipProcessor
class SiglipVisionModelTester:
def __init__(
self,
parent,
batch_size=12,
image_size=30,
patch_size=2,
num_channels=3,
is_training=True,
hidden_size=32,
num_hidden_layers=2,
num_attention_heads=4,
intermediate_size=37,
dropout=0.1,
attention_dropout=0.1,
initializer_range=0.02,
scope=None,
):
self.parent = parent
self.batch_size = batch_size
self.image_size = image_size
self.patch_size = patch_size
self.num_channels = num_channels
self.is_training = is_training
self.hidden_size = hidden_size
self.num_hidden_layers = num_hidden_layers
self.num_attention_heads = num_attention_heads
self.intermediate_size = intermediate_size
self.dropout = dropout
self.attention_dropout = attention_dropout
self.initializer_range = initializer_range
self.scope = scope
# in ViT, the seq length equals the number of patches
num_patches = (image_size // patch_size) ** 2
self.seq_length = num_patches
# Copied from tests.models.clip.test_modeling_clip.CLIPVisionModelTester.prepare_config_and_inputs
def prepare_config_and_inputs(self):
pixel_values = floats_tensor([self.batch_size, self.num_channels, self.image_size, self.image_size])
config = self.get_config()
return config, pixel_values
def get_config(self):
return SiglipVisionConfig(
image_size=self.image_size,
patch_size=self.patch_size,
num_channels=self.num_channels,
hidden_size=self.hidden_size,
num_hidden_layers=self.num_hidden_layers,
num_attention_heads=self.num_attention_heads,
intermediate_size=self.intermediate_size,
dropout=self.dropout,
attention_dropout=self.attention_dropout,
initializer_range=self.initializer_range,
)
def create_and_check_model(self, config, pixel_values):
model = SiglipVisionModel(config=config)
model.to(torch_device)
model.eval()
with torch.no_grad():
result = model(pixel_values)
# expected sequence length = num_patches + 1 (we add 1 for the [CLS] token)
image_size = (self.image_size, self.image_size)
patch_size = (self.patch_size, self.patch_size)
num_patches = (image_size[1] // patch_size[1]) * (image_size[0] // patch_size[0])
self.parent.assertEqual(result.last_hidden_state.shape, (self.batch_size, num_patches, self.hidden_size))
self.parent.assertEqual(result.pooler_output.shape, (self.batch_size, self.hidden_size))
# Copied from tests.models.clip.test_modeling_clip.CLIPVisionModelTester.prepare_config_and_inputs_for_common
def prepare_config_and_inputs_for_common(self):
config_and_inputs = self.prepare_config_and_inputs()
config, pixel_values = config_and_inputs
inputs_dict = {"pixel_values": pixel_values}
return config, inputs_dict
@require_torch
class SiglipVisionModelTest(ModelTesterMixin, unittest.TestCase):
"""
Here we also overwrite some of the tests of test_modeling_common.py, as SIGLIP does not use input_ids, inputs_embeds,
attention_mask and seq_length.
"""
all_model_classes = (SiglipVisionModel,) if is_torch_available() else ()
fx_compatible = False
test_pruning = False
test_resize_embeddings = False
test_head_masking = False
def setUp(self):
self.model_tester = SiglipVisionModelTester(self)
self.config_tester = ConfigTester(
self, config_class=SiglipVisionConfig, has_text_modality=False, hidden_size=37
)
def test_config(self):
self.config_tester.run_common_tests()
@unittest.skip(reason="SIGLIP does not use inputs_embeds")
def test_inputs_embeds(self):
pass
def test_model_common_attributes(self):
config, _ = self.model_tester.prepare_config_and_inputs_for_common()
for model_class in self.all_model_classes:
model = model_class(config)
self.assertIsInstance(model.get_input_embeddings(), (nn.Module))
x = model.get_output_embeddings()
self.assertTrue(x is None or isinstance(x, nn.Linear))
def test_forward_signature(self):
config, _ = self.model_tester.prepare_config_and_inputs_for_common()
for model_class in self.all_model_classes:
model = model_class(config)
signature = inspect.signature(model.forward)
# signature.parameters is an OrderedDict => so arg_names order is deterministic
arg_names = [*signature.parameters.keys()]
expected_arg_names = ["pixel_values"]
self.assertListEqual(arg_names[:1], expected_arg_names)
def test_model(self):
config_and_inputs = self.model_tester.prepare_config_and_inputs()
self.model_tester.create_and_check_model(*config_and_inputs)
@unittest.skip(reason="SiglipVisionModel does not support standalone training")
def test_training(self):
pass
@unittest.skip(reason="SiglipVisionModel does not support standalone training")
def test_training_gradient_checkpointing(self):
pass
@unittest.skip(reason="SiglipVisionModel does not support standalone training")
def test_training_gradient_checkpointing_use_reentrant(self):
pass
@unittest.skip(reason="SiglipVisionModel does not support standalone training")
def test_training_gradient_checkpointing_use_reentrant_false(self):
pass
@unittest.skip(reason="SiglipVisionModel has no base class and is not available in MODEL_MAPPING")
def test_save_load_fast_init_from_base(self):
pass
@unittest.skip(reason="SiglipVisionModel has no base class and is not available in MODEL_MAPPING")
def test_save_load_fast_init_to_base(self):
pass
@unittest.skip(reason="Siglip uses the same initialization scheme as the Flax original implementation")
def test_initialization(self):
pass
@slow
def test_model_from_pretrained(self):
for model_name in SIGLIP_PRETRAINED_MODEL_ARCHIVE_LIST[:1]:
model = SiglipVisionModel.from_pretrained(model_name)
self.assertIsNotNone(model)
class SiglipTextModelTester:
def __init__(
self,
parent,
batch_size=12,
seq_length=7,
is_training=True,
use_input_mask=True,
use_labels=True,
vocab_size=99,
hidden_size=32,
num_hidden_layers=2,
num_attention_heads=4,
intermediate_size=37,
dropout=0.1,
attention_dropout=0.1,
max_position_embeddings=512,
initializer_range=0.02,
scope=None,
):
self.parent = parent
self.batch_size = batch_size
self.seq_length = seq_length
self.is_training = is_training
self.use_input_mask = use_input_mask
self.use_labels = use_labels
self.vocab_size = vocab_size
self.hidden_size = hidden_size
self.num_hidden_layers = num_hidden_layers
self.num_attention_heads = num_attention_heads
self.intermediate_size = intermediate_size
self.dropout = dropout
self.attention_dropout = attention_dropout
self.max_position_embeddings = max_position_embeddings
self.initializer_range = initializer_range
self.scope = scope
# Copied from tests.models.clip.test_modeling_clip.CLIPTextModelTester.prepare_config_and_inputs
def prepare_config_and_inputs(self):
input_ids = ids_tensor([self.batch_size, self.seq_length], self.vocab_size)
input_mask = None
if self.use_input_mask:
input_mask = random_attention_mask([self.batch_size, self.seq_length])
if input_mask is not None:
batch_size, seq_length = input_mask.shape
rnd_start_indices = np.random.randint(1, seq_length - 1, size=(batch_size,))
for batch_idx, start_index in enumerate(rnd_start_indices):
input_mask[batch_idx, :start_index] = 1
input_mask[batch_idx, start_index:] = 0
config = self.get_config()
return config, input_ids, input_mask
def get_config(self):
return SiglipTextConfig(
vocab_size=self.vocab_size,
hidden_size=self.hidden_size,
num_hidden_layers=self.num_hidden_layers,
num_attention_heads=self.num_attention_heads,
intermediate_size=self.intermediate_size,
dropout=self.dropout,
attention_dropout=self.attention_dropout,
max_position_embeddings=self.max_position_embeddings,
initializer_range=self.initializer_range,
)
def create_and_check_model(self, config, input_ids, input_mask):
model = SiglipTextModel(config=config)
model.to(torch_device)
model.eval()
with torch.no_grad():
result = model(input_ids, attention_mask=input_mask)
result = model(input_ids)
self.parent.assertEqual(result.last_hidden_state.shape, (self.batch_size, self.seq_length, self.hidden_size))
self.parent.assertEqual(result.pooler_output.shape, (self.batch_size, self.hidden_size))
# Copied from tests.models.clip.test_modeling_clip.CLIPTextModelTester.prepare_config_and_inputs_for_common
def prepare_config_and_inputs_for_common(self):
config_and_inputs = self.prepare_config_and_inputs()
config, input_ids, input_mask = config_and_inputs
inputs_dict = {"input_ids": input_ids, "attention_mask": input_mask}
return config, inputs_dict
@require_torch
class SiglipTextModelTest(ModelTesterMixin, unittest.TestCase):
all_model_classes = (SiglipTextModel,) if is_torch_available() else ()
fx_compatible = False
test_pruning = False
test_head_masking = False
model_split_percents = [0.5, 0.8, 0.9]
# Copied from tests.models.clip.test_modeling_clip.CLIPTextModelTest.setUp with CLIP->Siglip
def setUp(self):
self.model_tester = SiglipTextModelTester(self)
self.config_tester = ConfigTester(self, config_class=SiglipTextConfig, hidden_size=37)
# Copied from tests.models.clip.test_modeling_clip.CLIPTextModelTest.test_config
def test_config(self):
self.config_tester.run_common_tests()
# Copied from tests.models.clip.test_modeling_clip.CLIPTextModelTest.test_model
def test_model(self):
config_and_inputs = self.model_tester.prepare_config_and_inputs()
self.model_tester.create_and_check_model(*config_and_inputs)
# Copied from tests.models.clip.test_modeling_clip.CLIPTextModelTest.test_training
def test_training(self):
pass
# Copied from tests.models.clip.test_modeling_clip.CLIPTextModelTest.test_training_gradient_checkpointing
def test_training_gradient_checkpointing(self):
pass
@unittest.skip(
reason="This architecure seem to not compute gradients properly when using GC, check: https://github.com/huggingface/transformers/pull/27124"
)
# Copied from tests.models.clip.test_modeling_clip.CLIPTextModelTest.test_training_gradient_checkpointing_use_reentrant
def test_training_gradient_checkpointing_use_reentrant(self):
pass
@unittest.skip(
reason="This architecure seem to not compute gradients properly when using GC, check: https://github.com/huggingface/transformers/pull/27124"
)
# Copied from tests.models.clip.test_modeling_clip.CLIPTextModelTest.test_training_gradient_checkpointing_use_reentrant_false
def test_training_gradient_checkpointing_use_reentrant_false(self):
pass
@unittest.skip(reason="Siglip does not use inputs_embeds")
# Copied from tests.models.clip.test_modeling_clip.CLIPTextModelTest.test_inputs_embeds
def test_inputs_embeds(self):
pass
@unittest.skip(reason="SiglipTextModel has no base class and is not available in MODEL_MAPPING")
# Copied from tests.models.clip.test_modeling_clip.CLIPTextModelTest.test_save_load_fast_init_from_base
def test_save_load_fast_init_from_base(self):
pass
@unittest.skip(reason="SiglipTextModel has no base class and is not available in MODEL_MAPPING")
# Copied from tests.models.clip.test_modeling_clip.CLIPTextModelTest.test_save_load_fast_init_to_base
def test_save_load_fast_init_to_base(self):
pass
@unittest.skip(reason="Siglip uses the same initialization scheme as the Flax original implementation")
def test_initialization(self):
pass
@slow
def test_model_from_pretrained(self):
for model_name in SIGLIP_PRETRAINED_MODEL_ARCHIVE_LIST[:1]:
model = SiglipTextModel.from_pretrained(model_name)
self.assertIsNotNone(model)
class SiglipModelTester:
def __init__(self, parent, text_kwargs=None, vision_kwargs=None, is_training=True):
if text_kwargs is None:
text_kwargs = {}
if vision_kwargs is None:
vision_kwargs = {}
self.parent = parent
self.text_model_tester = SiglipTextModelTester(parent, **text_kwargs)
self.vision_model_tester = SiglipVisionModelTester(parent, **vision_kwargs)
self.is_training = is_training
# Copied from tests.models.clip.test_modeling_clip.CLIPModelTester.prepare_config_and_inputs
def prepare_config_and_inputs(self):
text_config, input_ids, attention_mask = self.text_model_tester.prepare_config_and_inputs()
vision_config, pixel_values = self.vision_model_tester.prepare_config_and_inputs()
config = self.get_config()
return config, input_ids, attention_mask, pixel_values
def get_config(self):
return SiglipConfig.from_text_vision_configs(
self.text_model_tester.get_config(),
self.vision_model_tester.get_config(),
)
def create_and_check_model(self, config, input_ids, attention_mask, pixel_values):
model = SiglipModel(config).to(torch_device).eval()
with torch.no_grad():
result = model(input_ids, pixel_values, attention_mask)
self.parent.assertEqual(
result.logits_per_image.shape, (self.vision_model_tester.batch_size, self.text_model_tester.batch_size)
)
self.parent.assertEqual(
result.logits_per_text.shape, (self.text_model_tester.batch_size, self.vision_model_tester.batch_size)
)
def prepare_config_and_inputs_for_common(self):
config_and_inputs = self.prepare_config_and_inputs()
config, input_ids, attention_mask, pixel_values = config_and_inputs
inputs_dict = {
"input_ids": input_ids,
"attention_mask": attention_mask,
"pixel_values": pixel_values,
"return_loss": False,
}
return config, inputs_dict
@require_torch
class SiglipModelTest(ModelTesterMixin, PipelineTesterMixin, unittest.TestCase):
all_model_classes = (SiglipModel,) if is_torch_available() else ()
pipeline_model_mapping = {"feature-extraction": SiglipModel} if is_torch_available() else {}
fx_compatible = False
test_head_masking = False
test_pruning = False
test_resize_embeddings = False
test_attention_outputs = False
# Copied from tests.models.clip.test_modeling_clip.CLIPModelTest.setUp with CLIP->Siglip
def setUp(self):
self.model_tester = SiglipModelTester(self)
# Copied from tests.models.clip.test_modeling_clip.CLIPModelTest.test_model
def test_model(self):
config_and_inputs = self.model_tester.prepare_config_and_inputs()
self.model_tester.create_and_check_model(*config_and_inputs)
@unittest.skip(reason="Hidden_states is tested in individual model tests")
# Copied from tests.models.clip.test_modeling_clip.CLIPModelTest.test_hidden_states_output
def test_hidden_states_output(self):
pass
@unittest.skip(reason="Inputs_embeds is tested in individual model tests")
# Copied from tests.models.clip.test_modeling_clip.CLIPModelTest.test_inputs_embeds
def test_inputs_embeds(self):
pass
@unittest.skip(reason="Retain_grad is tested in individual model tests")
# Copied from tests.models.clip.test_modeling_clip.CLIPModelTest.test_retain_grad_hidden_states_attentions
def test_retain_grad_hidden_states_attentions(self):
pass
@unittest.skip(reason="SiglipModel does not have input/output embeddings")
# Copied from tests.models.clip.test_modeling_clip.CLIPModelTest.test_model_common_attributes
def test_model_common_attributes(self):
pass
@unittest.skip(reason="SiglipModel does not support training")
def test_training(self):
pass
@unittest.skip(reason="SiglipModel does not support training")
def test_training_gradient_checkpointing(self):
pass
@unittest.skip(reason="SiglipModel does not support training")
def test_training_gradient_checkpointing_use_reentrant(self):
pass
@unittest.skip(reason="SiglipModel does not support training")
def test_training_gradient_checkpointing_use_reentrant_false(self):
pass
@unittest.skip(reason="Siglip uses the same initialization scheme as the Flax original implementation")
def test_initialization(self):
pass
# Copied from tests.models.clip.test_modeling_clip.CLIPModelTest._create_and_check_torchscript with CLIP->Siglip
def _create_and_check_torchscript(self, config, inputs_dict):
if not self.test_torchscript:
return
configs_no_init = _config_zero_init(config) # To be sure we have no Nan
configs_no_init.torchscript = True
configs_no_init.return_dict = False
for model_class in self.all_model_classes:
model = model_class(config=configs_no_init)
model.to(torch_device)
model.eval()
try:
input_ids = inputs_dict["input_ids"]
pixel_values = inputs_dict["pixel_values"] # Siglip needs pixel_values
traced_model = torch.jit.trace(model, (input_ids, pixel_values))
except RuntimeError:
self.fail("Couldn't trace module.")
with tempfile.TemporaryDirectory() as tmp_dir_name:
pt_file_name = os.path.join(tmp_dir_name, "traced_model.pt")
try:
torch.jit.save(traced_model, pt_file_name)
except Exception:
self.fail("Couldn't save module.")
try:
loaded_model = torch.jit.load(pt_file_name)
except Exception:
self.fail("Couldn't load module.")
model.to(torch_device)
model.eval()
loaded_model.to(torch_device)
loaded_model.eval()
model_state_dict = model.state_dict()
loaded_model_state_dict = loaded_model.state_dict()
non_persistent_buffers = {}
for key in loaded_model_state_dict.keys():
if key not in model_state_dict.keys():
non_persistent_buffers[key] = loaded_model_state_dict[key]
loaded_model_state_dict = {
key: value for key, value in loaded_model_state_dict.items() if key not in non_persistent_buffers
}
self.assertEqual(set(model_state_dict.keys()), set(loaded_model_state_dict.keys()))
model_buffers = list(model.buffers())
for non_persistent_buffer in non_persistent_buffers.values():
found_buffer = False
for i, model_buffer in enumerate(model_buffers):
if torch.equal(non_persistent_buffer, model_buffer):
found_buffer = True
break
self.assertTrue(found_buffer)
model_buffers.pop(i)
models_equal = True
for layer_name, p1 in model_state_dict.items():
p2 = loaded_model_state_dict[layer_name]
if p1.data.ne(p2.data).sum() > 0:
models_equal = False
self.assertTrue(models_equal)
# Copied from tests.models.clip.test_modeling_clip.CLIPModelTest.test_load_vision_text_config with CLIP->Siglip
def test_load_vision_text_config(self):
config, inputs_dict = self.model_tester.prepare_config_and_inputs_for_common()
# Save SiglipConfig and check if we can load SiglipVisionConfig from it
with tempfile.TemporaryDirectory() as tmp_dir_name:
config.save_pretrained(tmp_dir_name)
vision_config = SiglipVisionConfig.from_pretrained(tmp_dir_name)
self.assertDictEqual(config.vision_config.to_dict(), vision_config.to_dict())
# Save SiglipConfig and check if we can load SiglipTextConfig from it
with tempfile.TemporaryDirectory() as tmp_dir_name:
config.save_pretrained(tmp_dir_name)
text_config = SiglipTextConfig.from_pretrained(tmp_dir_name)
self.assertDictEqual(config.text_config.to_dict(), text_config.to_dict())
@slow
# Copied from tests.models.clip.test_modeling_clip.CLIPModelTest.test_model_from_pretrained with CLIPModel->SiglipModel, CLIP->SIGLIP
def test_model_from_pretrained(self):
for model_name in SIGLIP_PRETRAINED_MODEL_ARCHIVE_LIST[:1]:
model = SiglipModel.from_pretrained(model_name)
self.assertIsNotNone(model)
# We will verify our results on an image of cute cats
def prepare_img():
url = "http://images.cocodataset.org/val2017/000000039769.jpg"
image = Image.open(requests.get(url, stream=True).raw)
return image
@require_vision
@require_torch
class SiglipModelIntegrationTest(unittest.TestCase):
@slow
def test_inference(self):
model_name = "google/siglip-base-patch16-224"
model = SiglipModel.from_pretrained(model_name).to(torch_device)
processor = SiglipProcessor.from_pretrained(model_name)
image = prepare_img()
inputs = processor(
text=["a photo of 2 cats", "a photo of 2 dogs"], images=image, padding="max_length", return_tensors="pt"
).to(torch_device)
# forward pass
with torch.no_grad():
outputs = model(**inputs)
logits_per_image = outputs.logits_per_image
logits_per_text = outputs.logits_per_text
# verify the logits
self.assertEqual(
logits_per_image.shape,
torch.Size((inputs.pixel_values.shape[0], inputs.input_ids.shape[0])),
)
self.assertEqual(
logits_per_text.shape,
torch.Size((inputs.input_ids.shape[0], inputs.pixel_values.shape[0])),
)
expected_logits = torch.tensor([[-0.7567, -10.3354]], device=torch_device)
self.assertTrue(torch.allclose(outputs.logits_per_image, expected_logits, atol=1e-3))
# verify the probs
probs = torch.sigmoid(logits_per_image) # these are the probabilities
expected_probs = torch.tensor([[3.1937e-01, 3.2463e-05]], device=torch_device)
self.assertTrue(torch.allclose(probs, expected_probs, atol=1e-3))
| transformers/tests/models/siglip/test_modeling_siglip.py/0 | {
"file_path": "transformers/tests/models/siglip/test_modeling_siglip.py",
"repo_id": "transformers",
"token_count": 10564
} | 364 |
# coding=utf-8
# Copyright 2022 The HuggingFace Inc. team. All rights reserved.
#
# Licensed under the Apache License, Version 2.0 (the "License");
# you may not use this file except in compliance with the License.
# You may obtain a copy of the License at
#
# http://www.apache.org/licenses/LICENSE-2.0
#
# Unless required by applicable law or agreed to in writing, software
# distributed under the License is distributed on an "AS IS" BASIS,
# WITHOUT WARRANTIES OR CONDITIONS OF ANY KIND, either express or implied.
# See the License for the specific language governing permissions and
# limitations under the License.
""" Testing suite for the PyTorch SpeechT5 model. """
import copy
import inspect
import tempfile
import unittest
from transformers import SpeechT5Config, SpeechT5HifiGanConfig
from transformers.testing_utils import (
is_torch_available,
require_sentencepiece,
require_tokenizers,
require_torch,
slow,
torch_device,
)
from transformers.trainer_utils import set_seed
from transformers.utils import cached_property
from ...test_configuration_common import ConfigTester
from ...test_modeling_common import (
ModelTesterMixin,
_config_zero_init,
floats_tensor,
ids_tensor,
random_attention_mask,
)
from ...test_pipeline_mixin import PipelineTesterMixin
if is_torch_available():
import torch
from transformers import (
SpeechT5ForSpeechToSpeech,
SpeechT5ForSpeechToText,
SpeechT5ForTextToSpeech,
SpeechT5HifiGan,
SpeechT5Model,
SpeechT5Processor,
)
def prepare_inputs_dict(
config,
input_ids=None,
input_values=None,
decoder_input_ids=None,
decoder_input_values=None,
attention_mask=None,
decoder_attention_mask=None,
head_mask=None,
decoder_head_mask=None,
cross_attn_head_mask=None,
):
if input_ids is not None:
encoder_dict = {"input_ids": input_ids}
else:
encoder_dict = {"input_values": input_values}
if decoder_input_ids is not None:
decoder_dict = {"decoder_input_ids": decoder_input_ids}
else:
decoder_dict = {"decoder_input_values": decoder_input_values}
if head_mask is None:
head_mask = torch.ones(config.encoder_layers, config.encoder_attention_heads, device=torch_device)
if decoder_head_mask is None:
decoder_head_mask = torch.ones(config.decoder_layers, config.decoder_attention_heads, device=torch_device)
if cross_attn_head_mask is None:
cross_attn_head_mask = torch.ones(config.decoder_layers, config.decoder_attention_heads, device=torch_device)
return {
**encoder_dict,
**decoder_dict,
"attention_mask": attention_mask,
"decoder_attention_mask": decoder_attention_mask,
"head_mask": head_mask,
"decoder_head_mask": decoder_head_mask,
"cross_attn_head_mask": cross_attn_head_mask,
}
@require_torch
class SpeechT5ModelTester:
def __init__(
self,
parent,
batch_size=13,
seq_length=7,
is_training=False,
vocab_size=81,
hidden_size=24,
num_hidden_layers=2,
num_attention_heads=2,
intermediate_size=4,
):
self.parent = parent
self.batch_size = batch_size
self.seq_length = seq_length
self.is_training = is_training
self.vocab_size = vocab_size
self.hidden_size = hidden_size
self.num_hidden_layers = num_hidden_layers
self.num_attention_heads = num_attention_heads
self.intermediate_size = intermediate_size
def prepare_config_and_inputs(self):
input_values = floats_tensor([self.batch_size, self.seq_length, self.hidden_size], scale=1.0)
attention_mask = random_attention_mask([self.batch_size, self.seq_length])
decoder_input_values = floats_tensor([self.batch_size, self.seq_length, self.hidden_size], scale=1.0)
decoder_attention_mask = random_attention_mask([self.batch_size, self.seq_length])
config = self.get_config()
inputs_dict = prepare_inputs_dict(
config,
input_values=input_values,
decoder_input_values=decoder_input_values,
attention_mask=attention_mask,
decoder_attention_mask=decoder_attention_mask,
)
return config, inputs_dict
def prepare_config_and_inputs_for_common(self):
config, inputs_dict = self.prepare_config_and_inputs()
return config, inputs_dict
def get_config(self):
return SpeechT5Config(
vocab_size=self.vocab_size,
hidden_size=self.hidden_size,
encoder_layers=self.num_hidden_layers,
decoder_layers=self.num_hidden_layers,
encoder_attention_heads=self.num_attention_heads,
decoder_attention_heads=self.num_attention_heads,
encoder_ffn_dim=self.intermediate_size,
decoder_ffn_dim=self.intermediate_size,
)
def create_and_check_model_forward(self, config, inputs_dict):
model = SpeechT5Model(config=config).to(torch_device).eval()
input_values = inputs_dict["input_values"]
attention_mask = inputs_dict["attention_mask"]
decoder_input_values = inputs_dict["decoder_input_values"]
result = model(input_values, attention_mask=attention_mask, decoder_input_values=decoder_input_values)
self.parent.assertEqual(result.last_hidden_state.shape, (self.batch_size, self.seq_length, self.hidden_size))
@require_torch
class SpeechT5ModelTest(ModelTesterMixin, PipelineTesterMixin, unittest.TestCase):
all_model_classes = (SpeechT5Model,) if is_torch_available() else ()
pipeline_model_mapping = (
{"automatic-speech-recognition": SpeechT5ForSpeechToText, "feature-extraction": SpeechT5Model}
if is_torch_available()
else {}
)
is_encoder_decoder = True
test_pruning = False
test_headmasking = False
test_resize_embeddings = False
input_name = "input_values"
def setUp(self):
self.model_tester = SpeechT5ModelTester(self)
self.config_tester = ConfigTester(self, config_class=SpeechT5Config, hidden_size=37)
def test_config(self):
self.config_tester.run_common_tests()
def test_model_forward(self):
config_and_inputs = self.model_tester.prepare_config_and_inputs()
self.model_tester.create_and_check_model_forward(*config_and_inputs)
def test_forward_signature(self):
config, _ = self.model_tester.prepare_config_and_inputs_for_common()
for model_class in self.all_model_classes:
model = model_class(config)
signature = inspect.signature(model.forward)
# signature.parameters is an OrderedDict => so arg_names order is deterministic
arg_names = [*signature.parameters.keys()]
expected_arg_names = [
"input_values",
"attention_mask",
"decoder_input_values",
"decoder_attention_mask",
]
expected_arg_names.extend(
["head_mask", "decoder_head_mask", "cross_attn_head_mask", "encoder_outputs"]
if "head_mask" and "decoder_head_mask" and "cross_attn_head_mask" in arg_names
else ["encoder_outputs"]
)
self.assertListEqual(arg_names[: len(expected_arg_names)], expected_arg_names)
# this model has no inputs_embeds
def test_inputs_embeds(self):
pass
# this model has no input embeddings
def test_model_common_attributes(self):
pass
def test_retain_grad_hidden_states_attentions(self):
# decoder cannot keep gradients
pass
@slow
def test_torchscript_output_attentions(self):
# disabled because this model doesn't have decoder_input_ids
pass
@slow
def test_torchscript_output_hidden_state(self):
# disabled because this model doesn't have decoder_input_ids
pass
@slow
def test_torchscript_simple(self):
# disabled because this model doesn't have decoder_input_ids
pass
@require_torch
class SpeechT5ForSpeechToTextTester:
def __init__(
self,
parent,
batch_size=13,
encoder_seq_length=1024, # speech is longer
decoder_seq_length=7,
is_training=False,
hidden_size=24,
num_hidden_layers=2,
num_attention_heads=2,
intermediate_size=4,
conv_dim=(32, 32, 32),
conv_stride=(4, 4, 4),
conv_kernel=(8, 8, 8),
conv_bias=False,
num_conv_pos_embeddings=16,
num_conv_pos_embedding_groups=2,
vocab_size=81,
):
self.parent = parent
self.batch_size = batch_size
self.encoder_seq_length = encoder_seq_length
self.decoder_seq_length = decoder_seq_length
self.is_training = is_training
self.hidden_size = hidden_size
self.num_hidden_layers = num_hidden_layers
self.num_attention_heads = num_attention_heads
self.intermediate_size = intermediate_size
self.conv_dim = conv_dim
self.conv_stride = conv_stride
self.conv_kernel = conv_kernel
self.conv_bias = conv_bias
self.num_conv_pos_embeddings = num_conv_pos_embeddings
self.num_conv_pos_embedding_groups = num_conv_pos_embedding_groups
self.vocab_size = vocab_size
def prepare_config_and_inputs(self):
input_values = floats_tensor([self.batch_size, self.encoder_seq_length], scale=1.0)
attention_mask = random_attention_mask([self.batch_size, self.encoder_seq_length])
decoder_input_ids = ids_tensor([self.batch_size, self.decoder_seq_length], self.vocab_size).clamp(2)
decoder_attention_mask = random_attention_mask([self.batch_size, self.decoder_seq_length])
config = self.get_config()
inputs_dict = prepare_inputs_dict(
config,
input_values=input_values,
decoder_input_ids=decoder_input_ids,
attention_mask=attention_mask,
decoder_attention_mask=decoder_attention_mask,
)
return config, inputs_dict
def prepare_config_and_inputs_for_common(self):
config, inputs_dict = self.prepare_config_and_inputs()
return config, inputs_dict
def get_config(self):
return SpeechT5Config(
hidden_size=self.hidden_size,
encoder_layers=self.num_hidden_layers,
decoder_layers=self.num_hidden_layers,
encoder_attention_heads=self.num_attention_heads,
decoder_attention_heads=self.num_attention_heads,
encoder_ffn_dim=self.intermediate_size,
decoder_ffn_dim=self.intermediate_size,
conv_dim=self.conv_dim,
conv_stride=self.conv_stride,
conv_kernel=self.conv_kernel,
conv_bias=self.conv_bias,
num_conv_pos_embeddings=self.num_conv_pos_embeddings,
num_conv_pos_embedding_groups=self.num_conv_pos_embedding_groups,
vocab_size=self.vocab_size,
)
def create_and_check_model_forward(self, config, inputs_dict):
model = SpeechT5ForSpeechToText(config=config).to(torch_device).eval()
input_values = inputs_dict["input_values"]
attention_mask = inputs_dict["attention_mask"]
decoder_input_ids = inputs_dict["decoder_input_ids"]
result = model(input_values, attention_mask=attention_mask, decoder_input_ids=decoder_input_ids)
self.parent.assertEqual(result.logits.shape, (self.batch_size, self.decoder_seq_length, self.vocab_size))
def create_and_check_decoder_model_past_large_inputs(self, config, inputs_dict):
model = SpeechT5ForSpeechToText(config=config).get_decoder().to(torch_device).eval()
input_ids = inputs_dict["decoder_input_ids"]
attention_mask = inputs_dict["decoder_attention_mask"]
# first forward pass
outputs = model(input_ids, attention_mask=attention_mask, use_cache=True)
output, past_key_values = outputs.to_tuple()
# create hypothetical multiple next token and extent to next_input_ids
next_tokens = ids_tensor((self.batch_size, 3), config.vocab_size).clamp(2)
next_attn_mask = ids_tensor((self.batch_size, 3), 2)
# append to next input_ids and
next_input_ids = torch.cat([input_ids, next_tokens], dim=-1)
next_attention_mask = torch.cat([attention_mask, next_attn_mask], dim=-1)
output_from_no_past = model(next_input_ids, attention_mask=next_attention_mask)["last_hidden_state"]
output_from_past = model(next_tokens, attention_mask=next_attention_mask, past_key_values=past_key_values)[
"last_hidden_state"
]
# select random slice
random_slice_idx = ids_tensor((1,), output_from_past.shape[-1]).item()
output_from_no_past_slice = output_from_no_past[:, -3:, random_slice_idx].detach()
output_from_past_slice = output_from_past[:, :, random_slice_idx].detach()
self.parent.assertTrue(output_from_past_slice.shape[1] == next_tokens.shape[1])
# test that outputs are equal for slice
self.parent.assertTrue(torch.allclose(output_from_past_slice, output_from_no_past_slice, atol=1e-2))
@require_torch
class SpeechT5ForSpeechToTextTest(ModelTesterMixin, unittest.TestCase):
all_model_classes = (SpeechT5ForSpeechToText,) if is_torch_available() else ()
all_generative_model_classes = (SpeechT5ForSpeechToText,) if is_torch_available() else ()
is_encoder_decoder = True
test_pruning = False
test_headmasking = False
input_name = "input_values"
def setUp(self):
self.model_tester = SpeechT5ForSpeechToTextTester(self)
self.config_tester = ConfigTester(self, config_class=SpeechT5Config, hidden_size=37)
def test_config(self):
self.config_tester.run_common_tests()
def test_save_load_strict(self):
config, inputs_dict = self.model_tester.prepare_config_and_inputs()
for model_class in self.all_model_classes:
model = model_class(config)
with tempfile.TemporaryDirectory() as tmpdirname:
model.save_pretrained(tmpdirname)
model2, info = model_class.from_pretrained(tmpdirname, output_loading_info=True)
self.assertEqual(info["missing_keys"], [])
def test_model_forward(self):
config_and_inputs = self.model_tester.prepare_config_and_inputs()
self.model_tester.create_and_check_model_forward(*config_and_inputs)
def test_decoder_model_past_with_large_inputs(self):
config_and_inputs = self.model_tester.prepare_config_and_inputs()
self.model_tester.create_and_check_decoder_model_past_large_inputs(*config_and_inputs)
def test_attention_outputs(self):
config, inputs_dict = self.model_tester.prepare_config_and_inputs_for_common()
config.return_dict = True
seq_len = getattr(self.model_tester, "seq_length", None)
decoder_seq_length = getattr(self.model_tester, "decoder_seq_length", seq_len)
encoder_seq_length = getattr(self.model_tester, "encoder_seq_length", seq_len)
decoder_key_length = getattr(self.model_tester, "decoder_key_length", decoder_seq_length)
encoder_key_length = getattr(self.model_tester, "key_length", encoder_seq_length)
for model_class in self.all_model_classes:
inputs_dict["output_attentions"] = True
inputs_dict["output_hidden_states"] = False
config.return_dict = True
model = model_class(config)
model.to(torch_device)
model.eval()
subsampled_encoder_seq_length = model.speecht5.encoder.prenet._get_feat_extract_output_lengths(
encoder_seq_length
)
subsampled_encoder_key_length = model.speecht5.encoder.prenet._get_feat_extract_output_lengths(
encoder_key_length
)
with torch.no_grad():
outputs = model(**self._prepare_for_class(inputs_dict, model_class))
attentions = outputs.encoder_attentions if config.is_encoder_decoder else outputs.attentions
self.assertEqual(len(attentions), self.model_tester.num_hidden_layers)
# check that output_attentions also work using config
del inputs_dict["output_attentions"]
config.output_attentions = True
model = model_class(config)
model.to(torch_device)
model.eval()
with torch.no_grad():
outputs = model(**self._prepare_for_class(inputs_dict, model_class))
attentions = outputs.encoder_attentions if config.is_encoder_decoder else outputs.attentions
self.assertEqual(len(attentions), self.model_tester.num_hidden_layers)
self.assertListEqual(
list(attentions[0].shape[-3:]),
[self.model_tester.num_attention_heads, subsampled_encoder_seq_length, subsampled_encoder_key_length],
)
out_len = len(outputs)
correct_outlen = 5
# loss is at first position
if "labels" in inputs_dict:
correct_outlen += 1 # loss is added to beginning
if "past_key_values" in outputs:
correct_outlen += 1 # past_key_values have been returned
self.assertEqual(out_len, correct_outlen)
# decoder attentions
decoder_attentions = outputs.decoder_attentions
self.assertIsInstance(decoder_attentions, (list, tuple))
self.assertEqual(len(decoder_attentions), self.model_tester.num_hidden_layers)
self.assertListEqual(
list(decoder_attentions[0].shape[-3:]),
[self.model_tester.num_attention_heads, decoder_seq_length, decoder_key_length],
)
# cross attentions
cross_attentions = outputs.cross_attentions
self.assertIsInstance(cross_attentions, (list, tuple))
self.assertEqual(len(cross_attentions), self.model_tester.num_hidden_layers)
self.assertListEqual(
list(cross_attentions[0].shape[-3:]),
[
self.model_tester.num_attention_heads,
decoder_seq_length,
subsampled_encoder_key_length,
],
)
# Check attention is always last and order is fine
inputs_dict["output_attentions"] = True
inputs_dict["output_hidden_states"] = True
model = model_class(config)
model.to(torch_device)
model.eval()
with torch.no_grad():
outputs = model(**self._prepare_for_class(inputs_dict, model_class))
added_hidden_states = 2
self.assertEqual(out_len + added_hidden_states, len(outputs))
self_attentions = outputs.encoder_attentions if config.is_encoder_decoder else outputs.attentions
self.assertEqual(len(self_attentions), self.model_tester.num_hidden_layers)
self.assertListEqual(
list(self_attentions[0].shape[-3:]),
[self.model_tester.num_attention_heads, subsampled_encoder_seq_length, subsampled_encoder_key_length],
)
def test_forward_signature(self):
config, _ = self.model_tester.prepare_config_and_inputs_for_common()
for model_class in self.all_model_classes:
model = model_class(config)
signature = inspect.signature(model.forward)
# signature.parameters is an OrderedDict => so arg_names order is deterministic
arg_names = [*signature.parameters.keys()]
expected_arg_names = [
"input_values",
"attention_mask",
"decoder_input_ids",
"decoder_attention_mask",
]
expected_arg_names.extend(
["head_mask", "decoder_head_mask", "cross_attn_head_mask", "encoder_outputs"]
if "head_mask" and "decoder_head_mask" and "cross_attn_head_mask" in arg_names
else ["encoder_outputs"]
)
self.assertListEqual(arg_names[: len(expected_arg_names)], expected_arg_names)
def test_hidden_states_output(self):
def check_hidden_states_output(inputs_dict, config, model_class):
model = model_class(config)
model.to(torch_device)
model.eval()
with torch.no_grad():
outputs = model(**self._prepare_for_class(inputs_dict, model_class))
hidden_states = outputs.encoder_hidden_states if config.is_encoder_decoder else outputs.hidden_states
expected_num_layers = getattr(
self.model_tester, "expected_num_hidden_layers", self.model_tester.num_hidden_layers + 1
)
self.assertEqual(len(hidden_states), expected_num_layers)
if hasattr(self.model_tester, "encoder_seq_length"):
seq_length = self.model_tester.encoder_seq_length
else:
seq_length = self.model_tester.seq_length
subsampled_seq_length = model.speecht5.encoder.prenet._get_feat_extract_output_lengths(seq_length)
self.assertListEqual(
list(hidden_states[0].shape[-2:]),
[subsampled_seq_length, self.model_tester.hidden_size],
)
if config.is_encoder_decoder:
hidden_states = outputs.decoder_hidden_states
self.assertIsInstance(hidden_states, (list, tuple))
self.assertEqual(len(hidden_states), expected_num_layers)
seq_len = getattr(self.model_tester, "seq_length", None)
decoder_seq_length = getattr(self.model_tester, "decoder_seq_length", seq_len)
self.assertListEqual(
list(hidden_states[0].shape[-2:]),
[decoder_seq_length, self.model_tester.hidden_size],
)
config, inputs_dict = self.model_tester.prepare_config_and_inputs_for_common()
for model_class in self.all_model_classes:
inputs_dict["output_hidden_states"] = True
check_hidden_states_output(inputs_dict, config, model_class)
# check that output_hidden_states also work using config
del inputs_dict["output_hidden_states"]
config.output_hidden_states = True
check_hidden_states_output(inputs_dict, config, model_class)
def test_initialization(self):
config, inputs_dict = self.model_tester.prepare_config_and_inputs_for_common()
configs_no_init = _config_zero_init(config)
for model_class in self.all_model_classes:
model = model_class(config=configs_no_init)
for name, param in model.named_parameters():
uniform_init_parms = [
"conv.weight",
"conv.parametrizations.weight",
"masked_spec_embed",
"feature_projection.projection.weight",
"feature_projection.projection.bias",
]
if param.requires_grad:
if any(x in name for x in uniform_init_parms):
self.assertTrue(
-1.0 <= ((param.data.mean() * 1e9).round() / 1e9).item() <= 1.0,
msg=f"Parameter {name} of model {model_class} seems not properly initialized",
)
else:
self.assertIn(
((param.data.mean() * 1e9).round() / 1e9).item(),
[0.0, 1.0],
msg=f"Parameter {name} of model {model_class} seems not properly initialized",
)
# this model has no inputs_embeds
def test_inputs_embeds(self):
pass
def test_resize_embeddings_untied(self):
original_config, inputs_dict = self.model_tester.prepare_config_and_inputs_for_common()
if not self.test_resize_embeddings:
return
original_config.tie_word_embeddings = False
# if model cannot untied embeddings -> leave test
if original_config.tie_word_embeddings:
return
for model_class in self.all_model_classes:
config = copy.deepcopy(original_config)
model = model_class(config).to(torch_device)
# if no output embeddings -> leave test
if model.get_output_embeddings() is None:
continue
# Check that resizing the token embeddings with a larger vocab size increases the model's vocab size
model_vocab_size = config.vocab_size
model.resize_token_embeddings(model_vocab_size + 10)
self.assertEqual(model.config.vocab_size, model_vocab_size + 10)
output_embeds = model.get_output_embeddings()
self.assertEqual(output_embeds.weight.shape[0], model_vocab_size + 10)
# Check bias if present
if output_embeds.bias is not None:
self.assertEqual(output_embeds.bias.shape[0], model_vocab_size + 10)
# Check that the model can still do a forward pass successfully (every parameter should be resized)
model(**self._prepare_for_class(inputs_dict, model_class))
# Check that resizing the token embeddings with a smaller vocab size decreases the model's vocab size
model.resize_token_embeddings(model_vocab_size - 15)
self.assertEqual(model.config.vocab_size, model_vocab_size - 15)
# Check that it actually resizes the embeddings matrix
output_embeds = model.get_output_embeddings()
self.assertEqual(output_embeds.weight.shape[0], model_vocab_size - 15)
# Check bias if present
if output_embeds.bias is not None:
self.assertEqual(output_embeds.bias.shape[0], model_vocab_size - 15)
# Check that the model can still do a forward pass successfully (every parameter should be resized)
if "decoder_input_ids" in inputs_dict:
inputs_dict["decoder_input_ids"].clamp_(max=model_vocab_size - 15 - 1)
# Check that the model can still do a forward pass successfully (every parameter should be resized)
model(**self._prepare_for_class(inputs_dict, model_class))
def test_resize_tokens_embeddings(self):
original_config, inputs_dict = self.model_tester.prepare_config_and_inputs_for_common()
if not self.test_resize_embeddings:
return
for model_class in self.all_model_classes:
config = copy.deepcopy(original_config)
model = model_class(config)
model.to(torch_device)
if self.model_tester.is_training is False:
model.eval()
model_vocab_size = config.vocab_size
# Retrieve the embeddings and clone theme
model_embed = model.resize_token_embeddings(model_vocab_size)
cloned_embeddings = model_embed.weight.clone()
# Check that resizing the token embeddings with a larger vocab size increases the model's vocab size
model_embed = model.resize_token_embeddings(model_vocab_size + 10)
self.assertEqual(model.config.vocab_size, model_vocab_size + 10)
# Check that it actually resizes the embeddings matrix
self.assertEqual(model_embed.weight.shape[0], cloned_embeddings.shape[0] + 10)
# Check that the model can still do a forward pass successfully (every parameter should be resized)
model(**self._prepare_for_class(inputs_dict, model_class))
# Check that resizing the token embeddings with a smaller vocab size decreases the model's vocab size
model_embed = model.resize_token_embeddings(model_vocab_size - 15)
self.assertEqual(model.config.vocab_size, model_vocab_size - 15)
# Check that it actually resizes the embeddings matrix
self.assertEqual(model_embed.weight.shape[0], cloned_embeddings.shape[0] - 15)
# make sure that decoder_input_ids are resized
if "decoder_input_ids" in inputs_dict:
inputs_dict["decoder_input_ids"].clamp_(max=model_vocab_size - 15 - 1)
model(**self._prepare_for_class(inputs_dict, model_class))
# Check that adding and removing tokens has not modified the first part of the embedding matrix.
models_equal = True
for p1, p2 in zip(cloned_embeddings, model_embed.weight):
if p1.data.ne(p2.data).sum() > 0:
models_equal = False
self.assertTrue(models_equal)
def test_retain_grad_hidden_states_attentions(self):
# decoder cannot keep gradients
pass
# training is not supported yet
def test_training(self):
pass
def test_training_gradient_checkpointing(self):
pass
@unittest.skip(
reason="This architecure seem to not compute gradients properly when using GC, check: https://github.com/huggingface/transformers/pull/27124"
)
def test_training_gradient_checkpointing_use_reentrant(self):
pass
@unittest.skip(
reason="This architecure seem to not compute gradients properly when using GC, check: https://github.com/huggingface/transformers/pull/27124"
)
def test_training_gradient_checkpointing_use_reentrant_false(self):
pass
# overwrite from test_modeling_common
def _mock_init_weights(self, module):
if hasattr(module, "weight") and module.weight is not None:
module.weight.data.fill_(3)
if hasattr(module, "weight_g") and module.weight_g is not None:
module.weight_g.data.fill_(3)
if hasattr(module, "weight_v") and module.weight_v is not None:
module.weight_v.data.fill_(3)
if hasattr(module, "bias") and module.bias is not None:
module.bias.data.fill_(3)
if hasattr(module, "masked_spec_embed") and module.masked_spec_embed is not None:
module.masked_spec_embed.data.fill_(3)
@require_torch
@require_sentencepiece
@require_tokenizers
@slow
class SpeechT5ForSpeechToTextIntegrationTests(unittest.TestCase):
@cached_property
def default_processor(self):
return SpeechT5Processor.from_pretrained("microsoft/speecht5_asr")
def _load_datasamples(self, num_samples):
from datasets import load_dataset
ds = load_dataset("hf-internal-testing/librispeech_asr_dummy", "clean", split="validation")
# automatic decoding with librispeech
speech_samples = ds.sort("id").select(range(num_samples))[:num_samples]["audio"]
return [x["array"] for x in speech_samples]
def test_generation_librispeech(self):
model = SpeechT5ForSpeechToText.from_pretrained("microsoft/speecht5_asr")
model.to(torch_device)
processor = self.default_processor
input_speech = self._load_datasamples(1)
input_values = processor(audio=input_speech, return_tensors="pt").input_values.to(torch_device)
generated_ids = model.generate(input_values)
generated_transcript = processor.batch_decode(generated_ids, skip_special_tokens=True)
EXPECTED_TRANSCRIPTIONS = [
"mister quilter is the apostle of the middle classes and we are glad to welcome his gospel"
]
self.assertListEqual(generated_transcript, EXPECTED_TRANSCRIPTIONS)
def test_generation_librispeech_batched(self):
model = SpeechT5ForSpeechToText.from_pretrained("microsoft/speecht5_asr")
model.to(torch_device)
processor = self.default_processor
input_speech = self._load_datasamples(4)
inputs = processor(audio=input_speech, return_tensors="pt", padding=True)
input_values = inputs.input_values.to(torch_device)
attention_mask = inputs.attention_mask.to(torch_device)
generated_ids = model.generate(input_values, attention_mask=attention_mask)
generated_transcripts = processor.batch_decode(generated_ids, skip_special_tokens=True)
EXPECTED_TRANSCRIPTIONS = [
"mister quilter is the apostle of the middle classes and we are glad to welcome his gospel",
"nor is mister quilter's manner less interesting than his matter",
"he tells us that at this festive season of the year with christmas and rosebeaf looming before us"
" similars drawn from eating and its results occur most readily to the mind",
"he has grave doubts whether sir frederick latin's work is really greek after all and can discover in it"
" but little of rocky ithica",
]
self.assertListEqual(generated_transcripts, EXPECTED_TRANSCRIPTIONS)
@require_torch
class SpeechT5ForTextToSpeechTester:
def __init__(
self,
parent,
batch_size=13,
encoder_seq_length=7,
decoder_seq_length=1024, # speech is longer
is_training=False,
hidden_size=24,
num_hidden_layers=2,
num_attention_heads=2,
intermediate_size=4,
vocab_size=81,
num_mel_bins=20,
reduction_factor=2,
speech_decoder_postnet_layers=2,
speech_decoder_postnet_units=32,
speech_decoder_prenet_units=32,
):
self.parent = parent
self.batch_size = batch_size
self.encoder_seq_length = encoder_seq_length
self.decoder_seq_length = decoder_seq_length
self.is_training = is_training
self.hidden_size = hidden_size
self.num_hidden_layers = num_hidden_layers
self.num_attention_heads = num_attention_heads
self.intermediate_size = intermediate_size
self.vocab_size = vocab_size
self.num_mel_bins = num_mel_bins
self.reduction_factor = reduction_factor
self.speech_decoder_postnet_layers = speech_decoder_postnet_layers
self.speech_decoder_postnet_units = speech_decoder_postnet_units
self.speech_decoder_prenet_units = speech_decoder_prenet_units
def prepare_config_and_inputs(self):
input_ids = ids_tensor([self.batch_size, self.encoder_seq_length], self.vocab_size).clamp(2)
attention_mask = random_attention_mask([self.batch_size, self.encoder_seq_length])
decoder_input_values = floats_tensor([self.batch_size, self.decoder_seq_length, self.num_mel_bins], scale=1.0)
decoder_attention_mask = random_attention_mask([self.batch_size, self.decoder_seq_length])
config = self.get_config()
inputs_dict = prepare_inputs_dict(
config,
input_ids=input_ids,
decoder_input_values=decoder_input_values,
attention_mask=attention_mask,
decoder_attention_mask=decoder_attention_mask,
)
return config, inputs_dict
def prepare_config_and_inputs_for_common(self):
config, inputs_dict = self.prepare_config_and_inputs()
return config, inputs_dict
def get_config(self):
return SpeechT5Config(
hidden_size=self.hidden_size,
encoder_layers=self.num_hidden_layers,
decoder_layers=self.num_hidden_layers,
encoder_attention_heads=self.num_attention_heads,
decoder_attention_heads=self.num_attention_heads,
encoder_ffn_dim=self.intermediate_size,
decoder_ffn_dim=self.intermediate_size,
vocab_size=self.vocab_size,
num_mel_bins=self.num_mel_bins,
reduction_factor=self.reduction_factor,
speech_decoder_postnet_layers=self.speech_decoder_postnet_layers,
speech_decoder_postnet_units=self.speech_decoder_postnet_units,
speech_decoder_prenet_units=self.speech_decoder_prenet_units,
)
def create_and_check_model_forward(self, config, inputs_dict):
model = SpeechT5ForTextToSpeech(config=config).to(torch_device).eval()
input_ids = inputs_dict["input_ids"]
attention_mask = inputs_dict["attention_mask"]
decoder_input_values = inputs_dict["decoder_input_values"]
result = model(input_ids, attention_mask=attention_mask, decoder_input_values=decoder_input_values)
self.parent.assertEqual(
result.spectrogram.shape,
(self.batch_size, self.decoder_seq_length * self.reduction_factor, self.num_mel_bins),
)
@require_torch
class SpeechT5ForTextToSpeechTest(ModelTesterMixin, unittest.TestCase):
all_model_classes = (SpeechT5ForTextToSpeech,) if is_torch_available() else ()
all_generative_model_classes = (SpeechT5ForTextToSpeech,) if is_torch_available() else ()
is_encoder_decoder = True
test_pruning = False
test_headmasking = False
input_name = "input_ids"
def setUp(self):
self.model_tester = SpeechT5ForTextToSpeechTester(self)
self.config_tester = ConfigTester(self, config_class=SpeechT5Config, hidden_size=37)
def test_config(self):
self.config_tester.run_common_tests()
def test_save_load_strict(self):
config, inputs_dict = self.model_tester.prepare_config_and_inputs()
for model_class in self.all_model_classes:
model = model_class(config)
with tempfile.TemporaryDirectory() as tmpdirname:
model.save_pretrained(tmpdirname)
model2, info = model_class.from_pretrained(tmpdirname, output_loading_info=True)
self.assertEqual(info["missing_keys"], [])
def test_model_forward(self):
config_and_inputs = self.model_tester.prepare_config_and_inputs()
self.model_tester.create_and_check_model_forward(*config_and_inputs)
# skipped because there is always dropout in SpeechT5SpeechDecoderPrenet
def test_decoder_model_past_with_large_inputs(self):
pass
# skipped because there is always dropout in SpeechT5SpeechDecoderPrenet
def test_determinism(self):
pass
def test_forward_signature(self):
config, _ = self.model_tester.prepare_config_and_inputs_for_common()
for model_class in self.all_model_classes:
model = model_class(config)
signature = inspect.signature(model.forward)
# signature.parameters is an OrderedDict => so arg_names order is deterministic
arg_names = [*signature.parameters.keys()]
expected_arg_names = [
"input_ids",
"attention_mask",
"decoder_input_values",
"decoder_attention_mask",
]
expected_arg_names.extend(
["head_mask", "decoder_head_mask", "cross_attn_head_mask", "encoder_outputs"]
if "head_mask" and "decoder_head_mask" and "cross_attn_head_mask" in arg_names
else ["encoder_outputs"]
)
self.assertListEqual(arg_names[: len(expected_arg_names)], expected_arg_names)
def test_initialization(self):
config, inputs_dict = self.model_tester.prepare_config_and_inputs_for_common()
configs_no_init = _config_zero_init(config)
for model_class in self.all_model_classes:
model = model_class(config=configs_no_init)
for name, param in model.named_parameters():
uniform_init_parms = [
"conv.weight",
]
if param.requires_grad:
if any(x in name for x in uniform_init_parms):
self.assertTrue(
-1.0 <= ((param.data.mean() * 1e9).round() / 1e9).item() <= 1.0,
msg=f"Parameter {name} of model {model_class} seems not properly initialized",
)
else:
self.assertIn(
((param.data.mean() * 1e9).round() / 1e9).item(),
[0.0, 1.0],
msg=f"Parameter {name} of model {model_class} seems not properly initialized",
)
# this model has no inputs_embeds
def test_inputs_embeds(self):
pass
# skipped because there is always dropout in SpeechT5SpeechDecoderPrenet
def test_model_outputs_equivalence(self):
pass
# skipped because there is always dropout in SpeechT5SpeechDecoderPrenet
def test_save_load(self):
pass
def test_retain_grad_hidden_states_attentions(self):
# decoder cannot keep gradients
pass
@slow
def test_torchscript_output_attentions(self):
# disabled because this model doesn't have decoder_input_ids
pass
@slow
def test_torchscript_output_hidden_state(self):
# disabled because this model doesn't have decoder_input_ids
pass
@slow
def test_torchscript_simple(self):
# disabled because this model doesn't have decoder_input_ids
pass
# training is not supported yet
def test_training(self):
pass
def test_training_gradient_checkpointing(self):
pass
@unittest.skip(
reason="This architecure seem to not compute gradients properly when using GC, check: https://github.com/huggingface/transformers/pull/27124"
)
def test_training_gradient_checkpointing_use_reentrant(self):
pass
@unittest.skip(
reason="This architecure seem to not compute gradients properly when using GC, check: https://github.com/huggingface/transformers/pull/27124"
)
def test_training_gradient_checkpointing_use_reentrant_false(self):
pass
# overwrite from test_modeling_common
def _mock_init_weights(self, module):
if hasattr(module, "weight") and module.weight is not None:
module.weight.data.fill_(3)
if hasattr(module, "weight_g") and module.weight_g is not None:
module.weight_g.data.fill_(3)
if hasattr(module, "weight_v") and module.weight_v is not None:
module.weight_v.data.fill_(3)
if hasattr(module, "bias") and module.bias is not None:
module.bias.data.fill_(3)
@require_torch
@require_sentencepiece
@require_tokenizers
class SpeechT5ForTextToSpeechIntegrationTests(unittest.TestCase):
@cached_property
def default_model(self):
return SpeechT5ForTextToSpeech.from_pretrained("microsoft/speecht5_tts").to(torch_device)
@cached_property
def default_processor(self):
return SpeechT5Processor.from_pretrained("microsoft/speecht5_tts")
@cached_property
def default_vocoder(self):
return SpeechT5HifiGan.from_pretrained("microsoft/speecht5_hifigan").to(torch_device)
def test_generation(self):
model = self.default_model
processor = self.default_processor
input_text = "Mister Quilter is the apostle of the middle classes, and we are glad to welcome his gospel."
input_ids = processor(text=input_text, return_tensors="pt").input_ids.to(torch_device)
speaker_embeddings = torch.zeros((1, 512), device=torch_device)
# Generate speech and validate output dimensions
set_seed(555) # Ensure deterministic behavior
generated_speech = model.generate_speech(input_ids, speaker_embeddings=speaker_embeddings)
num_mel_bins = model.config.num_mel_bins
self.assertEqual(
generated_speech.shape[1], num_mel_bins, "Generated speech output has an unexpected number of mel bins."
)
# Validate generation with additional kwargs using model.generate;
# same method than generate_speech
set_seed(555) # Reset seed for consistent results
generated_speech_with_generate = model.generate(
input_ids, attention_mask=None, speaker_embeddings=speaker_embeddings
)
self.assertEqual(
generated_speech_with_generate.shape,
generated_speech.shape,
"Shape mismatch between generate_speech and generate methods.",
)
def test_one_to_many_generation(self):
model = self.default_model
processor = self.default_processor
vocoder = self.default_vocoder
input_text = [
"mister quilter is the apostle of the middle classes and we are glad to welcome his gospel",
"nor is mister quilter's manner less interesting than his matter",
"he tells us that at this festive season of the year with christmas and rosebeaf looming before us",
]
inputs = processor(text=input_text, padding="max_length", max_length=128, return_tensors="pt").to(torch_device)
speaker_embeddings = torch.zeros((1, 512), device=torch_device)
# Generate spectrograms
set_seed(555) # Ensure deterministic behavior
spectrograms, spectrogram_lengths = model.generate_speech(
input_ids=inputs["input_ids"],
speaker_embeddings=speaker_embeddings,
attention_mask=inputs["attention_mask"],
return_output_lengths=True,
)
# Validate generated spectrogram dimensions
expected_batch_size = len(input_text)
num_mel_bins = model.config.num_mel_bins
actual_batch_size, _, actual_num_mel_bins = spectrograms.shape
self.assertEqual(actual_batch_size, expected_batch_size, "Batch size of generated spectrograms is incorrect.")
self.assertEqual(
actual_num_mel_bins, num_mel_bins, "Number of mel bins in batch generated spectrograms is incorrect."
)
# Generate waveforms using the vocoder
waveforms = vocoder(spectrograms)
waveform_lengths = [int(waveforms.size(1) / max(spectrogram_lengths)) * i for i in spectrogram_lengths]
# Validate generation with integrated vocoder
set_seed(555) # Reset seed for consistent results
waveforms_with_vocoder, waveform_lengths_with_vocoder = model.generate_speech(
input_ids=inputs["input_ids"],
speaker_embeddings=speaker_embeddings,
attention_mask=inputs["attention_mask"],
vocoder=vocoder,
return_output_lengths=True,
)
# Check consistency between waveforms generated with and without standalone vocoder
self.assertTrue(
torch.allclose(waveforms, waveforms_with_vocoder, atol=1e-8),
"Mismatch in waveforms generated with and without the standalone vocoder.",
)
self.assertEqual(
waveform_lengths,
waveform_lengths_with_vocoder,
"Waveform lengths differ between standalone and integrated vocoder generation.",
)
# Test generation consistency without returning lengths
set_seed(555) # Reset seed for consistent results
waveforms_with_vocoder_no_lengths = model.generate_speech(
input_ids=inputs["input_ids"],
speaker_embeddings=speaker_embeddings,
attention_mask=inputs["attention_mask"],
vocoder=vocoder,
return_output_lengths=False,
)
# Validate waveform consistency without length information
self.assertTrue(
torch.allclose(waveforms_with_vocoder_no_lengths, waveforms_with_vocoder, atol=1e-8),
"Waveforms differ when generated with and without length information.",
)
# Validate batch vs. single instance generation consistency
for i, text in enumerate(input_text):
inputs = processor(text=text, padding="max_length", max_length=128, return_tensors="pt").to(torch_device)
set_seed(555) # Reset seed for consistent results
spectrogram = model.generate_speech(
input_ids=inputs["input_ids"],
speaker_embeddings=speaker_embeddings,
)
# Check spectrogram shape consistency
self.assertEqual(
spectrogram.shape,
spectrograms[i][: spectrogram_lengths[i]].shape,
"Mismatch in spectrogram shape between batch and single instance generation.",
)
# Generate and validate waveform for single instance
waveform = vocoder(spectrogram)
self.assertEqual(
waveform.shape,
waveforms[i][: waveform_lengths[i]].shape,
"Mismatch in waveform shape between batch and single instance generation.",
)
# Check waveform consistency with integrated vocoder
set_seed(555) # Reset seed for consistent results
waveform_with_integrated_vocoder = model.generate_speech(
input_ids=inputs["input_ids"],
speaker_embeddings=speaker_embeddings,
vocoder=vocoder,
)
self.assertTrue(
torch.allclose(waveform, waveform_with_integrated_vocoder, atol=1e-8),
"Mismatch in waveform between standalone and integrated vocoder for single instance generation.",
)
def test_batch_generation(self):
model = self.default_model
processor = self.default_processor
vocoder = self.default_vocoder
input_text = [
"mister quilter is the apostle of the middle classes and we are glad to welcome his gospel",
"nor is mister quilter's manner less interesting than his matter",
"he tells us that at this festive season of the year with christmas and rosebeaf looming before us",
]
inputs = processor(text=input_text, padding="max_length", max_length=128, return_tensors="pt").to(torch_device)
set_seed(555) # Ensure deterministic behavior
speaker_embeddings = torch.randn((len(input_text), 512), device=torch_device)
# Generate spectrograms
set_seed(555) # Reset seed for consistent results
spectrograms, spectrogram_lengths = model.generate_speech(
input_ids=inputs["input_ids"],
speaker_embeddings=speaker_embeddings,
attention_mask=inputs["attention_mask"],
return_output_lengths=True,
)
# Validate generated spectrogram dimensions
expected_batch_size = len(input_text)
num_mel_bins = model.config.num_mel_bins
actual_batch_size, _, actual_num_mel_bins = spectrograms.shape
self.assertEqual(
actual_batch_size,
expected_batch_size,
"Batch size of generated spectrograms is incorrect.",
)
self.assertEqual(
actual_num_mel_bins,
num_mel_bins,
"Number of mel bins in batch generated spectrograms is incorrect.",
)
# Generate waveforms using the vocoder
waveforms = vocoder(spectrograms)
waveform_lengths = [int(waveforms.size(1) / max(spectrogram_lengths)) * i for i in spectrogram_lengths]
# Validate generation with integrated vocoder
set_seed(555) # Reset seed for consistent results
waveforms_with_vocoder, waveform_lengths_with_vocoder = model.generate_speech(
input_ids=inputs["input_ids"],
speaker_embeddings=speaker_embeddings,
attention_mask=inputs["attention_mask"],
vocoder=vocoder,
return_output_lengths=True,
)
# Check consistency between waveforms generated with and without standalone vocoder
self.assertTrue(
torch.allclose(waveforms, waveforms_with_vocoder, atol=1e-8),
"Mismatch in waveforms generated with and without the standalone vocoder.",
)
self.assertEqual(
waveform_lengths,
waveform_lengths_with_vocoder,
"Waveform lengths differ between standalone and integrated vocoder generation.",
)
# Test generation consistency without returning lengths
set_seed(555) # Reset seed for consistent results
waveforms_with_vocoder_no_lengths = model.generate_speech(
input_ids=inputs["input_ids"],
speaker_embeddings=speaker_embeddings,
attention_mask=inputs["attention_mask"],
vocoder=vocoder,
return_output_lengths=False,
)
# Validate waveform consistency without length information
self.assertTrue(
torch.allclose(waveforms_with_vocoder_no_lengths, waveforms_with_vocoder, atol=1e-8),
"Waveforms differ when generated with and without length information.",
)
# Validate batch vs. single instance generation consistency
for i, text in enumerate(input_text):
inputs = processor(text=text, padding="max_length", max_length=128, return_tensors="pt").to(torch_device)
current_speaker_embedding = speaker_embeddings[i].unsqueeze(0)
set_seed(555) # Reset seed for consistent results
spectrogram = model.generate_speech(
input_ids=inputs["input_ids"],
speaker_embeddings=current_speaker_embedding,
)
# Check spectrogram shape consistency
self.assertEqual(
spectrogram.shape,
spectrograms[i][: spectrogram_lengths[i]].shape,
"Mismatch in spectrogram shape between batch and single instance generation.",
)
# Generate and validate waveform for single instance
waveform = vocoder(spectrogram)
self.assertEqual(
waveform.shape,
waveforms[i][: waveform_lengths[i]].shape,
"Mismatch in waveform shape between batch and single instance generation.",
)
# Check waveform consistency with integrated vocoder
set_seed(555) # Reset seed for consistent results
waveform_with_integrated_vocoder = model.generate_speech(
input_ids=inputs["input_ids"],
speaker_embeddings=current_speaker_embedding,
vocoder=vocoder,
)
self.assertTrue(
torch.allclose(waveform, waveform_with_integrated_vocoder, atol=1e-8),
"Mismatch in waveform between standalone and integrated vocoder for single instance generation.",
)
@require_torch
class SpeechT5ForSpeechToSpeechTester:
def __init__(
self,
parent,
batch_size=13,
encoder_seq_length=1024, # speech is longer
decoder_seq_length=1024,
is_training=False,
hidden_size=24,
num_hidden_layers=2,
num_attention_heads=2,
intermediate_size=4,
conv_dim=(32, 32, 32),
conv_stride=(4, 4, 4),
conv_kernel=(8, 8, 8),
conv_bias=False,
num_conv_pos_embeddings=16,
num_conv_pos_embedding_groups=2,
vocab_size=81,
num_mel_bins=20,
reduction_factor=2,
speech_decoder_postnet_layers=2,
speech_decoder_postnet_units=32,
speech_decoder_prenet_units=32,
):
self.parent = parent
self.batch_size = batch_size
self.encoder_seq_length = encoder_seq_length
self.decoder_seq_length = decoder_seq_length
self.is_training = is_training
self.hidden_size = hidden_size
self.num_hidden_layers = num_hidden_layers
self.num_attention_heads = num_attention_heads
self.intermediate_size = intermediate_size
self.conv_dim = conv_dim
self.conv_stride = conv_stride
self.conv_kernel = conv_kernel
self.conv_bias = conv_bias
self.num_conv_pos_embeddings = num_conv_pos_embeddings
self.num_conv_pos_embedding_groups = num_conv_pos_embedding_groups
self.vocab_size = vocab_size
self.num_mel_bins = num_mel_bins
self.reduction_factor = reduction_factor
self.speech_decoder_postnet_layers = speech_decoder_postnet_layers
self.speech_decoder_postnet_units = speech_decoder_postnet_units
self.speech_decoder_prenet_units = speech_decoder_prenet_units
def prepare_config_and_inputs(self):
input_values = floats_tensor([self.batch_size, self.encoder_seq_length], scale=1.0)
attention_mask = random_attention_mask([self.batch_size, self.encoder_seq_length])
decoder_input_values = floats_tensor([self.batch_size, self.decoder_seq_length, self.num_mel_bins], scale=1.0)
decoder_attention_mask = random_attention_mask([self.batch_size, self.decoder_seq_length])
config = self.get_config()
inputs_dict = prepare_inputs_dict(
config,
input_values=input_values,
decoder_input_values=decoder_input_values,
attention_mask=attention_mask,
decoder_attention_mask=decoder_attention_mask,
)
return config, inputs_dict
def prepare_config_and_inputs_for_common(self):
config, inputs_dict = self.prepare_config_and_inputs()
return config, inputs_dict
def get_config(self):
return SpeechT5Config(
hidden_size=self.hidden_size,
encoder_layers=self.num_hidden_layers,
decoder_layers=self.num_hidden_layers,
encoder_attention_heads=self.num_attention_heads,
decoder_attention_heads=self.num_attention_heads,
encoder_ffn_dim=self.intermediate_size,
decoder_ffn_dim=self.intermediate_size,
conv_dim=self.conv_dim,
conv_stride=self.conv_stride,
conv_kernel=self.conv_kernel,
conv_bias=self.conv_bias,
num_conv_pos_embeddings=self.num_conv_pos_embeddings,
num_conv_pos_embedding_groups=self.num_conv_pos_embedding_groups,
vocab_size=self.vocab_size,
num_mel_bins=self.num_mel_bins,
reduction_factor=self.reduction_factor,
speech_decoder_postnet_layers=self.speech_decoder_postnet_layers,
speech_decoder_postnet_units=self.speech_decoder_postnet_units,
speech_decoder_prenet_units=self.speech_decoder_prenet_units,
)
def create_and_check_model_forward(self, config, inputs_dict):
model = SpeechT5ForSpeechToSpeech(config=config).to(torch_device).eval()
input_values = inputs_dict["input_values"]
attention_mask = inputs_dict["attention_mask"]
decoder_input_values = inputs_dict["decoder_input_values"]
result = model(input_values, attention_mask=attention_mask, decoder_input_values=decoder_input_values)
self.parent.assertEqual(
result.spectrogram.shape,
(self.batch_size, self.decoder_seq_length * self.reduction_factor, self.num_mel_bins),
)
@require_torch
class SpeechT5ForSpeechToSpeechTest(ModelTesterMixin, unittest.TestCase):
all_model_classes = (SpeechT5ForSpeechToSpeech,) if is_torch_available() else ()
all_generative_model_classes = (SpeechT5ForSpeechToSpeech,) if is_torch_available() else ()
is_encoder_decoder = True
test_pruning = False
test_headmasking = False
test_resize_embeddings = False
input_name = "input_values"
def setUp(self):
self.model_tester = SpeechT5ForSpeechToSpeechTester(self)
self.config_tester = ConfigTester(self, config_class=SpeechT5Config, hidden_size=37)
def test_config(self):
self.config_tester.run_common_tests()
def test_save_load_strict(self):
config, inputs_dict = self.model_tester.prepare_config_and_inputs()
for model_class in self.all_model_classes:
model = model_class(config)
with tempfile.TemporaryDirectory() as tmpdirname:
model.save_pretrained(tmpdirname)
model2, info = model_class.from_pretrained(tmpdirname, output_loading_info=True)
self.assertEqual(info["missing_keys"], [])
def test_model_forward(self):
config_and_inputs = self.model_tester.prepare_config_and_inputs()
self.model_tester.create_and_check_model_forward(*config_and_inputs)
# skipped because there is always dropout in SpeechT5SpeechDecoderPrenet
def test_decoder_model_past_with_large_inputs(self):
pass
# skipped because there is always dropout in SpeechT5SpeechDecoderPrenet
def test_determinism(self):
pass
def test_attention_outputs(self):
config, inputs_dict = self.model_tester.prepare_config_and_inputs_for_common()
config.return_dict = True
seq_len = getattr(self.model_tester, "seq_length", None)
decoder_seq_length = getattr(self.model_tester, "decoder_seq_length", seq_len)
encoder_seq_length = getattr(self.model_tester, "encoder_seq_length", seq_len)
decoder_key_length = getattr(self.model_tester, "decoder_key_length", decoder_seq_length)
encoder_key_length = getattr(self.model_tester, "key_length", encoder_seq_length)
for model_class in self.all_model_classes:
inputs_dict["output_attentions"] = True
inputs_dict["output_hidden_states"] = False
config.return_dict = True
model = model_class(config)
model.to(torch_device)
model.eval()
subsampled_encoder_seq_length = model.speecht5.encoder.prenet._get_feat_extract_output_lengths(
encoder_seq_length
)
subsampled_encoder_key_length = model.speecht5.encoder.prenet._get_feat_extract_output_lengths(
encoder_key_length
)
with torch.no_grad():
outputs = model(**self._prepare_for_class(inputs_dict, model_class))
attentions = outputs.encoder_attentions if config.is_encoder_decoder else outputs.attentions
self.assertEqual(len(attentions), self.model_tester.num_hidden_layers)
# check that output_attentions also work using config
del inputs_dict["output_attentions"]
config.output_attentions = True
model = model_class(config)
model.to(torch_device)
model.eval()
with torch.no_grad():
outputs = model(**self._prepare_for_class(inputs_dict, model_class))
attentions = outputs.encoder_attentions if config.is_encoder_decoder else outputs.attentions
self.assertEqual(len(attentions), self.model_tester.num_hidden_layers)
self.assertListEqual(
list(attentions[0].shape[-3:]),
[self.model_tester.num_attention_heads, subsampled_encoder_seq_length, subsampled_encoder_key_length],
)
out_len = len(outputs)
correct_outlen = 5
# loss is at first position
if "labels" in inputs_dict:
correct_outlen += 1 # loss is added to beginning
if "past_key_values" in outputs:
correct_outlen += 1 # past_key_values have been returned
self.assertEqual(out_len, correct_outlen)
# decoder attentions
decoder_attentions = outputs.decoder_attentions
self.assertIsInstance(decoder_attentions, (list, tuple))
self.assertEqual(len(decoder_attentions), self.model_tester.num_hidden_layers)
self.assertListEqual(
list(decoder_attentions[0].shape[-3:]),
[self.model_tester.num_attention_heads, decoder_seq_length, decoder_key_length],
)
# cross attentions
cross_attentions = outputs.cross_attentions
self.assertIsInstance(cross_attentions, (list, tuple))
self.assertEqual(len(cross_attentions), self.model_tester.num_hidden_layers)
self.assertListEqual(
list(cross_attentions[0].shape[-3:]),
[
self.model_tester.num_attention_heads,
decoder_seq_length,
subsampled_encoder_key_length,
],
)
# Check attention is always last and order is fine
inputs_dict["output_attentions"] = True
inputs_dict["output_hidden_states"] = True
model = model_class(config)
model.to(torch_device)
model.eval()
with torch.no_grad():
outputs = model(**self._prepare_for_class(inputs_dict, model_class))
added_hidden_states = 2
self.assertEqual(out_len + added_hidden_states, len(outputs))
self_attentions = outputs.encoder_attentions if config.is_encoder_decoder else outputs.attentions
self.assertEqual(len(self_attentions), self.model_tester.num_hidden_layers)
self.assertListEqual(
list(self_attentions[0].shape[-3:]),
[self.model_tester.num_attention_heads, subsampled_encoder_seq_length, subsampled_encoder_key_length],
)
def test_forward_signature(self):
config, _ = self.model_tester.prepare_config_and_inputs_for_common()
for model_class in self.all_model_classes:
model = model_class(config)
signature = inspect.signature(model.forward)
# signature.parameters is an OrderedDict => so arg_names order is deterministic
arg_names = [*signature.parameters.keys()]
expected_arg_names = [
"input_values",
"attention_mask",
"decoder_input_values",
"decoder_attention_mask",
]
expected_arg_names.extend(
["head_mask", "decoder_head_mask", "cross_attn_head_mask", "encoder_outputs"]
if "head_mask" and "decoder_head_mask" and "cross_attn_head_mask" in arg_names
else ["encoder_outputs"]
)
self.assertListEqual(arg_names[: len(expected_arg_names)], expected_arg_names)
def test_hidden_states_output(self):
def check_hidden_states_output(inputs_dict, config, model_class):
model = model_class(config)
model.to(torch_device)
model.eval()
with torch.no_grad():
outputs = model(**self._prepare_for_class(inputs_dict, model_class))
hidden_states = outputs.encoder_hidden_states if config.is_encoder_decoder else outputs.hidden_states
expected_num_layers = getattr(
self.model_tester, "expected_num_hidden_layers", self.model_tester.num_hidden_layers + 1
)
self.assertEqual(len(hidden_states), expected_num_layers)
if hasattr(self.model_tester, "encoder_seq_length"):
seq_length = self.model_tester.encoder_seq_length
else:
seq_length = self.model_tester.seq_length
subsampled_seq_length = model.speecht5.encoder.prenet._get_feat_extract_output_lengths(seq_length)
self.assertListEqual(
list(hidden_states[0].shape[-2:]),
[subsampled_seq_length, self.model_tester.hidden_size],
)
if config.is_encoder_decoder:
hidden_states = outputs.decoder_hidden_states
self.assertIsInstance(hidden_states, (list, tuple))
self.assertEqual(len(hidden_states), expected_num_layers)
seq_len = getattr(self.model_tester, "seq_length", None)
decoder_seq_length = getattr(self.model_tester, "decoder_seq_length", seq_len)
self.assertListEqual(
list(hidden_states[0].shape[-2:]),
[decoder_seq_length, self.model_tester.hidden_size],
)
config, inputs_dict = self.model_tester.prepare_config_and_inputs_for_common()
for model_class in self.all_model_classes:
inputs_dict["output_hidden_states"] = True
check_hidden_states_output(inputs_dict, config, model_class)
# check that output_hidden_states also work using config
del inputs_dict["output_hidden_states"]
config.output_hidden_states = True
check_hidden_states_output(inputs_dict, config, model_class)
def test_initialization(self):
config, inputs_dict = self.model_tester.prepare_config_and_inputs_for_common()
configs_no_init = _config_zero_init(config)
for model_class in self.all_model_classes:
model = model_class(config=configs_no_init)
for name, param in model.named_parameters():
uniform_init_parms = [
"conv.weight",
"conv.parametrizations.weight",
"masked_spec_embed",
"feature_projection.projection.weight",
"feature_projection.projection.bias",
]
if param.requires_grad:
if any(x in name for x in uniform_init_parms):
self.assertTrue(
-1.0 <= ((param.data.mean() * 1e9).round() / 1e9).item() <= 1.0,
msg=f"Parameter {name} of model {model_class} seems not properly initialized",
)
else:
self.assertIn(
((param.data.mean() * 1e9).round() / 1e9).item(),
[0.0, 1.0],
msg=f"Parameter {name} of model {model_class} seems not properly initialized",
)
# this model has no inputs_embeds
def test_inputs_embeds(self):
pass
# this model has no input embeddings
def test_model_common_attributes(self):
pass
# skipped because there is always dropout in SpeechT5SpeechDecoderPrenet
def test_model_outputs_equivalence(self):
pass
def test_retain_grad_hidden_states_attentions(self):
# decoder cannot keep gradients
pass
# skipped because there is always dropout in SpeechT5SpeechDecoderPrenet
def test_save_load(self):
pass
@slow
def test_torchscript_output_attentions(self):
# disabled because this model doesn't have decoder_input_ids
pass
@slow
def test_torchscript_output_hidden_state(self):
# disabled because this model doesn't have decoder_input_ids
pass
@slow
def test_torchscript_simple(self):
# disabled because this model doesn't have decoder_input_ids
pass
# training is not supported yet
def test_training(self):
pass
def test_training_gradient_checkpointing(self):
pass
@unittest.skip(
reason="This architecure seem to not compute gradients properly when using GC, check: https://github.com/huggingface/transformers/pull/27124"
)
def test_training_gradient_checkpointing_use_reentrant(self):
pass
@unittest.skip(
reason="This architecure seem to not compute gradients properly when using GC, check: https://github.com/huggingface/transformers/pull/27124"
)
def test_training_gradient_checkpointing_use_reentrant_false(self):
pass
# overwrite from test_modeling_common
def _mock_init_weights(self, module):
if hasattr(module, "weight") and module.weight is not None:
module.weight.data.fill_(3)
if hasattr(module, "weight_g") and module.weight_g is not None:
module.weight_g.data.fill_(3)
if hasattr(module, "weight_v") and module.weight_v is not None:
module.weight_v.data.fill_(3)
if hasattr(module, "bias") and module.bias is not None:
module.bias.data.fill_(3)
if hasattr(module, "masked_spec_embed") and module.masked_spec_embed is not None:
module.masked_spec_embed.data.fill_(3)
@require_torch
@require_sentencepiece
@require_tokenizers
@slow
class SpeechT5ForSpeechToSpeechIntegrationTests(unittest.TestCase):
@cached_property
def default_processor(self):
return SpeechT5Processor.from_pretrained("microsoft/speecht5_vc")
def _load_datasamples(self, num_samples):
from datasets import load_dataset
ds = load_dataset("hf-internal-testing/librispeech_asr_dummy", "clean", split="validation")
# automatic decoding with librispeech
speech_samples = ds.sort("id").select(range(num_samples))[:num_samples]["audio"]
return [x["array"] for x in speech_samples]
def test_generation_librispeech(self):
model = SpeechT5ForSpeechToSpeech.from_pretrained("microsoft/speecht5_vc")
model.to(torch_device)
processor = self.default_processor
input_speech = self._load_datasamples(1)
input_values = processor(audio=input_speech, return_tensors="pt").input_values.to(torch_device)
speaker_embeddings = torch.zeros((1, 512), device=torch_device)
generated_speech = model.generate_speech(input_values, speaker_embeddings=speaker_embeddings)
self.assertEqual(generated_speech.shape[1], model.config.num_mel_bins)
self.assertGreaterEqual(generated_speech.shape[0], 300)
self.assertLessEqual(generated_speech.shape[0], 310)
class SpeechT5HifiGanTester:
def __init__(
self,
parent,
batch_size=13,
seq_length=7,
is_training=False,
num_mel_bins=20,
):
self.parent = parent
self.batch_size = batch_size
self.seq_length = seq_length
self.is_training = is_training
self.num_mel_bins = num_mel_bins
def prepare_config_and_inputs(self):
input_values = floats_tensor([self.seq_length, self.num_mel_bins], scale=1.0)
config = self.get_config()
return config, input_values
def get_config(self):
return SpeechT5HifiGanConfig(
model_in_dim=self.num_mel_bins,
upsample_initial_channel=32,
)
def create_and_check_model(self, config, input_values):
model = SpeechT5HifiGan(config=config).to(torch_device).eval()
result = model(input_values)
self.parent.assertEqual(result.shape, (self.seq_length * 256,))
def prepare_config_and_inputs_for_common(self):
config, input_values = self.prepare_config_and_inputs()
inputs_dict = {"spectrogram": input_values}
return config, inputs_dict
@require_torch
class SpeechT5HifiGanTest(ModelTesterMixin, unittest.TestCase):
all_model_classes = (SpeechT5HifiGan,) if is_torch_available() else ()
test_torchscript = False
test_pruning = False
test_resize_embeddings = False
test_resize_position_embeddings = False
test_head_masking = False
test_mismatched_shapes = False
test_missing_keys = False
test_model_parallel = False
is_encoder_decoder = False
has_attentions = False
input_name = "spectrogram"
def setUp(self):
self.model_tester = SpeechT5HifiGanTester(self)
self.config_tester = ConfigTester(self, config_class=SpeechT5HifiGanConfig)
def test_config(self):
self.config_tester.create_and_test_config_to_json_string()
self.config_tester.create_and_test_config_to_json_file()
self.config_tester.create_and_test_config_from_and_save_pretrained()
self.config_tester.create_and_test_config_from_and_save_pretrained_subfolder()
self.config_tester.create_and_test_config_with_num_labels()
self.config_tester.check_config_can_be_init_without_params()
self.config_tester.check_config_arguments_init()
def test_model(self):
config_and_inputs = self.model_tester.prepare_config_and_inputs()
self.model_tester.create_and_check_model(*config_and_inputs)
def test_forward_signature(self):
config, _ = self.model_tester.prepare_config_and_inputs_for_common()
for model_class in self.all_model_classes:
model = model_class(config)
signature = inspect.signature(model.forward)
# signature.parameters is an OrderedDict => so arg_names order is deterministic
arg_names = [*signature.parameters.keys()]
expected_arg_names = [
"spectrogram",
]
self.assertListEqual(arg_names[: len(expected_arg_names)], expected_arg_names)
# this model does not output hidden states
def test_hidden_states_output(self):
pass
# skip
def test_initialization(self):
pass
# this model has no inputs_embeds
def test_inputs_embeds(self):
pass
# this model has no input embeddings
def test_model_common_attributes(self):
pass
# skip as this model doesn't support all arguments tested
def test_model_outputs_equivalence(self):
pass
# this model does not output hidden states
def test_retain_grad_hidden_states_attentions(self):
pass
# skip because it fails on automapping of SpeechT5HifiGanConfig
def test_save_load_fast_init_from_base(self):
pass
# skip because it fails on automapping of SpeechT5HifiGanConfig
def test_save_load_fast_init_to_base(self):
pass
def test_batched_inputs_outputs(self):
config, inputs = self.model_tester.prepare_config_and_inputs_for_common()
for model_class in self.all_model_classes:
model = model_class(config)
model.to(torch_device)
model.eval()
batched_inputs = inputs["spectrogram"].unsqueeze(0).repeat(2, 1, 1)
with torch.no_grad():
batched_outputs = model(batched_inputs.to(torch_device))
self.assertEqual(
batched_inputs.shape[0], batched_outputs.shape[0], msg="Got different batch dims for input and output"
)
def test_unbatched_inputs_outputs(self):
config, inputs = self.model_tester.prepare_config_and_inputs_for_common()
for model_class in self.all_model_classes:
model = model_class(config)
model.to(torch_device)
model.eval()
with torch.no_grad():
outputs = model(inputs["spectrogram"].to(torch_device))
self.assertTrue(outputs.dim() == 1, msg="Got un-batched inputs but batched output")
| transformers/tests/models/speecht5/test_modeling_speecht5.py/0 | {
"file_path": "transformers/tests/models/speecht5/test_modeling_speecht5.py",
"repo_id": "transformers",
"token_count": 35083
} | 365 |
# coding=utf-8
# Copyright 2022 The HuggingFace Inc. team. All rights reserved.
#
# Licensed under the Apache License, Version 2.0 (the "License");
# you may not use this file except in compliance with the License.
# You may obtain a copy of the License at
#
# http://www.apache.org/licenses/LICENSE-2.0
#
# Unless required by applicable law or agreed to in writing, software
# distributed under the License is distributed on an "AS IS" BASIS,
# WITHOUT WARRANTIES OR CONDITIONS OF ANY KIND, either express or implied.
# See the License for the specific language governing permissions and
# limitations under the License.
""" Testing suite for the PyTorch TimeSeriesTransformer model. """
import inspect
import tempfile
import unittest
from huggingface_hub import hf_hub_download
from parameterized import parameterized
from transformers import is_torch_available
from transformers.testing_utils import is_flaky, require_torch, slow, torch_device
from ...test_configuration_common import ConfigTester
from ...test_modeling_common import ModelTesterMixin, floats_tensor, ids_tensor
from ...test_pipeline_mixin import PipelineTesterMixin
TOLERANCE = 1e-4
if is_torch_available():
import torch
from transformers import (
TimeSeriesTransformerConfig,
TimeSeriesTransformerForPrediction,
TimeSeriesTransformerModel,
)
from transformers.models.time_series_transformer.modeling_time_series_transformer import (
TimeSeriesTransformerDecoder,
TimeSeriesTransformerEncoder,
)
@require_torch
class TimeSeriesTransformerModelTester:
def __init__(
self,
parent,
batch_size=13,
prediction_length=7,
context_length=14,
cardinality=19,
embedding_dimension=5,
num_time_features=4,
is_training=True,
hidden_size=64,
num_hidden_layers=2,
num_attention_heads=4,
intermediate_size=4,
hidden_act="gelu",
hidden_dropout_prob=0.1,
attention_probs_dropout_prob=0.1,
lags_sequence=[1, 2, 3, 4, 5],
):
self.parent = parent
self.batch_size = batch_size
self.prediction_length = prediction_length
self.context_length = context_length
self.cardinality = cardinality
self.num_time_features = num_time_features
self.lags_sequence = lags_sequence
self.embedding_dimension = embedding_dimension
self.is_training = is_training
self.hidden_size = hidden_size
self.num_hidden_layers = num_hidden_layers
self.num_attention_heads = num_attention_heads
self.intermediate_size = intermediate_size
self.hidden_act = hidden_act
self.hidden_dropout_prob = hidden_dropout_prob
self.attention_probs_dropout_prob = attention_probs_dropout_prob
self.encoder_seq_length = context_length
self.decoder_seq_length = prediction_length
def get_config(self):
return TimeSeriesTransformerConfig(
encoder_layers=self.num_hidden_layers,
decoder_layers=self.num_hidden_layers,
encoder_attention_heads=self.num_attention_heads,
decoder_attention_heads=self.num_attention_heads,
encoder_ffn_dim=self.intermediate_size,
decoder_ffn_dim=self.intermediate_size,
dropout=self.hidden_dropout_prob,
attention_dropout=self.attention_probs_dropout_prob,
prediction_length=self.prediction_length,
context_length=self.context_length,
lags_sequence=self.lags_sequence,
num_time_features=self.num_time_features,
num_static_real_features=1,
num_static_categorical_features=1,
cardinality=[self.cardinality],
embedding_dimension=[self.embedding_dimension],
)
def prepare_time_series_transformer_inputs_dict(self, config):
_past_length = config.context_length + max(config.lags_sequence)
static_categorical_features = ids_tensor([self.batch_size, 1], config.cardinality[0])
static_real_features = floats_tensor([self.batch_size, 1])
past_time_features = floats_tensor([self.batch_size, _past_length, config.num_time_features])
past_values = floats_tensor([self.batch_size, _past_length])
past_observed_mask = floats_tensor([self.batch_size, _past_length]) > 0.5
# decoder inputs
future_time_features = floats_tensor([self.batch_size, config.prediction_length, config.num_time_features])
future_values = floats_tensor([self.batch_size, config.prediction_length])
inputs_dict = {
"past_values": past_values,
"static_categorical_features": static_categorical_features,
"static_real_features": static_real_features,
"past_time_features": past_time_features,
"past_observed_mask": past_observed_mask,
"future_time_features": future_time_features,
"future_values": future_values,
}
return inputs_dict
def prepare_config_and_inputs(self):
config = self.get_config()
inputs_dict = self.prepare_time_series_transformer_inputs_dict(config)
return config, inputs_dict
def prepare_config_and_inputs_for_common(self):
config, inputs_dict = self.prepare_config_and_inputs()
return config, inputs_dict
def check_encoder_decoder_model_standalone(self, config, inputs_dict):
model = TimeSeriesTransformerModel(config=config).to(torch_device).eval()
outputs = model(**inputs_dict)
encoder_last_hidden_state = outputs.encoder_last_hidden_state
last_hidden_state = outputs.last_hidden_state
with tempfile.TemporaryDirectory() as tmpdirname:
encoder = model.get_encoder()
encoder.save_pretrained(tmpdirname)
encoder = TimeSeriesTransformerEncoder.from_pretrained(tmpdirname).to(torch_device)
transformer_inputs, _, _, _ = model.create_network_inputs(**inputs_dict)
enc_input = transformer_inputs[:, : config.context_length, ...]
dec_input = transformer_inputs[:, config.context_length :, ...]
encoder_last_hidden_state_2 = encoder(inputs_embeds=enc_input)[0]
self.parent.assertTrue((encoder_last_hidden_state_2 - encoder_last_hidden_state).abs().max().item() < 1e-3)
with tempfile.TemporaryDirectory() as tmpdirname:
decoder = model.get_decoder()
decoder.save_pretrained(tmpdirname)
decoder = TimeSeriesTransformerDecoder.from_pretrained(tmpdirname).to(torch_device)
last_hidden_state_2 = decoder(
inputs_embeds=dec_input,
encoder_hidden_states=encoder_last_hidden_state,
)[0]
self.parent.assertTrue((last_hidden_state_2 - last_hidden_state).abs().max().item() < 1e-3)
@require_torch
class TimeSeriesTransformerModelTest(ModelTesterMixin, PipelineTesterMixin, unittest.TestCase):
all_model_classes = (
(TimeSeriesTransformerModel, TimeSeriesTransformerForPrediction) if is_torch_available() else ()
)
all_generative_model_classes = (TimeSeriesTransformerForPrediction,) if is_torch_available() else ()
pipeline_model_mapping = {"feature-extraction": TimeSeriesTransformerModel} if is_torch_available() else {}
is_encoder_decoder = True
test_pruning = False
test_head_masking = False
test_missing_keys = False
test_torchscript = False
test_inputs_embeds = False
test_model_common_attributes = False
def setUp(self):
self.model_tester = TimeSeriesTransformerModelTester(self)
self.config_tester = ConfigTester(
self,
config_class=TimeSeriesTransformerConfig,
has_text_modality=False,
prediction_length=self.model_tester.prediction_length,
)
def test_config(self):
self.config_tester.run_common_tests()
def test_save_load_strict(self):
config, _ = self.model_tester.prepare_config_and_inputs()
for model_class in self.all_model_classes:
model = model_class(config)
with tempfile.TemporaryDirectory() as tmpdirname:
model.save_pretrained(tmpdirname)
model2, info = model_class.from_pretrained(tmpdirname, output_loading_info=True)
self.assertEqual(info["missing_keys"], [])
def test_encoder_decoder_model_standalone(self):
config_and_inputs = self.model_tester.prepare_config_and_inputs_for_common()
self.model_tester.check_encoder_decoder_model_standalone(*config_and_inputs)
# Ignore since we have no tokens embeddings
def test_resize_tokens_embeddings(self):
pass
# # Input is 'static_categorical_features' not 'input_ids'
def test_model_main_input_name(self):
model_signature = inspect.signature(getattr(TimeSeriesTransformerModel, "forward"))
# The main input is the name of the argument after `self`
observed_main_input_name = list(model_signature.parameters.keys())[1]
self.assertEqual(TimeSeriesTransformerModel.main_input_name, observed_main_input_name)
def test_forward_signature(self):
config, _ = self.model_tester.prepare_config_and_inputs_for_common()
for model_class in self.all_model_classes:
model = model_class(config)
signature = inspect.signature(model.forward)
# signature.parameters is an OrderedDict => so arg_names order is deterministic
arg_names = [*signature.parameters.keys()]
expected_arg_names = [
"past_values",
"past_time_features",
"past_observed_mask",
"static_categorical_features",
"static_real_features",
"future_values",
"future_time_features",
]
expected_arg_names.extend(
[
"future_observed_mask",
"decoder_attention_mask",
"head_mask",
"decoder_head_mask",
"cross_attn_head_mask",
"encoder_outputs",
"past_key_values",
"output_hidden_states",
"output_attentions",
"use_cache",
"return_dict",
]
if "future_observed_mask" in arg_names
else [
"decoder_attention_mask",
"head_mask",
"decoder_head_mask",
"cross_attn_head_mask",
"encoder_outputs",
"past_key_values",
"output_hidden_states",
"output_attentions",
"use_cache",
"return_dict",
]
)
self.assertListEqual(arg_names[: len(expected_arg_names)], expected_arg_names)
def test_attention_outputs(self):
config, inputs_dict = self.model_tester.prepare_config_and_inputs_for_common()
config.return_dict = True
seq_len = getattr(self.model_tester, "seq_length", None)
decoder_seq_length = getattr(self.model_tester, "decoder_seq_length", seq_len)
encoder_seq_length = getattr(self.model_tester, "encoder_seq_length", seq_len)
for model_class in self.all_model_classes:
inputs_dict["output_attentions"] = True
inputs_dict["output_hidden_states"] = False
config.return_dict = True
model = model_class(config)
model.to(torch_device)
model.eval()
with torch.no_grad():
outputs = model(**self._prepare_for_class(inputs_dict, model_class))
attentions = outputs.encoder_attentions if config.is_encoder_decoder else outputs.attentions
self.assertEqual(len(attentions), self.model_tester.num_hidden_layers)
# check that output_attentions also work using config
del inputs_dict["output_attentions"]
config.output_attentions = True
model = model_class(config)
model.to(torch_device)
model.eval()
with torch.no_grad():
outputs = model(**self._prepare_for_class(inputs_dict, model_class))
attentions = outputs.encoder_attentions
self.assertEqual(len(attentions), self.model_tester.num_hidden_layers)
self.assertListEqual(
list(attentions[0].shape[-3:]),
[self.model_tester.num_attention_heads, encoder_seq_length, encoder_seq_length],
)
out_len = len(outputs)
correct_outlen = 7
if "last_hidden_state" in outputs:
correct_outlen += 1
if "past_key_values" in outputs:
correct_outlen += 1 # past_key_values have been returned
if "loss" in outputs:
correct_outlen += 1
if "params" in outputs:
correct_outlen += 1
self.assertEqual(out_len, correct_outlen)
# decoder attentions
decoder_attentions = outputs.decoder_attentions
self.assertIsInstance(decoder_attentions, (list, tuple))
self.assertEqual(len(decoder_attentions), self.model_tester.num_hidden_layers)
self.assertListEqual(
list(decoder_attentions[0].shape[-3:]),
[self.model_tester.num_attention_heads, decoder_seq_length, decoder_seq_length],
)
# cross attentions
cross_attentions = outputs.cross_attentions
self.assertIsInstance(cross_attentions, (list, tuple))
self.assertEqual(len(cross_attentions), self.model_tester.num_hidden_layers)
self.assertListEqual(
list(cross_attentions[0].shape[-3:]),
[
self.model_tester.num_attention_heads,
decoder_seq_length,
encoder_seq_length,
],
)
# Check attention is always last and order is fine
inputs_dict["output_attentions"] = True
inputs_dict["output_hidden_states"] = True
model = model_class(config)
model.to(torch_device)
model.eval()
with torch.no_grad():
outputs = model(**self._prepare_for_class(inputs_dict, model_class))
self.assertEqual(out_len + 2, len(outputs))
self_attentions = outputs.encoder_attentions if config.is_encoder_decoder else outputs.attentions
self.assertEqual(len(self_attentions), self.model_tester.num_hidden_layers)
self.assertListEqual(
list(self_attentions[0].shape[-3:]),
[self.model_tester.num_attention_heads, encoder_seq_length, encoder_seq_length],
)
@unittest.skip(
reason="This architecure seem to not compute gradients properly when using GC, check: https://github.com/huggingface/transformers/pull/27124"
)
def test_training_gradient_checkpointing(self):
pass
@unittest.skip(
reason="This architecure seem to not compute gradients properly when using GC, check: https://github.com/huggingface/transformers/pull/27124"
)
def test_training_gradient_checkpointing_use_reentrant(self):
pass
@unittest.skip(
reason="This architecure seem to not compute gradients properly when using GC, check: https://github.com/huggingface/transformers/pull/27124"
)
def test_training_gradient_checkpointing_use_reentrant_false(self):
pass
@parameterized.expand(
[
(1, 5, [1]),
(1, 5, [1, 10, 15]),
(1, 5, [3, 6, 9, 10]),
(2, 5, [1, 2, 7]),
(2, 5, [2, 3, 4, 6]),
(4, 5, [1, 5, 9, 11]),
(4, 5, [7, 8, 13, 14]),
],
)
def test_create_network_inputs(self, prediction_length, context_length, lags_sequence):
history_length = max(lags_sequence) + context_length
config = TimeSeriesTransformerConfig(
prediction_length=prediction_length,
context_length=context_length,
lags_sequence=lags_sequence,
scaling=False,
num_parallel_samples=10,
num_static_categorical_features=1,
cardinality=[1],
embedding_dimension=[2],
num_static_real_features=1,
)
model = TimeSeriesTransformerModel(config)
batch = {
"static_categorical_features": torch.tensor([[0]], dtype=torch.int64),
"static_real_features": torch.tensor([[0.0]], dtype=torch.float32),
"past_time_features": torch.arange(history_length, dtype=torch.float32).view(1, history_length, 1),
"past_values": torch.arange(history_length, dtype=torch.float32).view(1, history_length),
"past_observed_mask": torch.arange(history_length, dtype=torch.float32).view(1, history_length),
}
# test with no future_target (only one step prediction)
batch["future_time_features"] = torch.arange(history_length, history_length + 1, dtype=torch.float32).view(
1, 1, 1
)
transformer_inputs, loc, scale, _ = model.create_network_inputs(**batch)
self.assertTrue((scale == 1.0).all())
assert (loc == 0.0).all()
ref = torch.arange(max(lags_sequence), history_length, dtype=torch.float32)
for idx, lag in enumerate(lags_sequence):
assert torch.isclose(ref - lag, transformer_inputs[0, :, idx]).all()
# test with all future data
batch["future_time_features"] = torch.arange(
history_length, history_length + prediction_length, dtype=torch.float32
).view(1, prediction_length, 1)
batch["future_values"] = torch.arange(
history_length, history_length + prediction_length, dtype=torch.float32
).view(1, prediction_length)
transformer_inputs, loc, scale, _ = model.create_network_inputs(**batch)
assert (scale == 1.0).all()
assert (loc == 0.0).all()
ref = torch.arange(max(lags_sequence), history_length + prediction_length, dtype=torch.float32)
for idx, lag in enumerate(lags_sequence):
assert torch.isclose(ref - lag, transformer_inputs[0, :, idx]).all()
# test for generation
batch.pop("future_values")
transformer_inputs, loc, scale, _ = model.create_network_inputs(**batch)
lagged_sequence = model.get_lagged_subsequences(
sequence=batch["past_values"],
subsequences_length=1,
shift=1,
)
# assert that the last element of the lagged sequence is the one after the encoders input
assert transformer_inputs[0, ..., 0][-1] + 1 == lagged_sequence[0, ..., 0][-1]
future_values = torch.arange(history_length, history_length + prediction_length, dtype=torch.float32).view(
1, prediction_length
)
# assert that the first element of the future_values is offset by lag after the decoders input
assert lagged_sequence[0, ..., 0][-1] + lags_sequence[0] == future_values[0, ..., 0]
@is_flaky()
def test_retain_grad_hidden_states_attentions(self):
super().test_retain_grad_hidden_states_attentions()
def prepare_batch(filename="train-batch.pt"):
file = hf_hub_download(repo_id="hf-internal-testing/tourism-monthly-batch", filename=filename, repo_type="dataset")
batch = torch.load(file, map_location=torch_device)
return batch
@require_torch
@slow
class TimeSeriesTransformerModelIntegrationTests(unittest.TestCase):
def test_inference_no_head(self):
model = TimeSeriesTransformerModel.from_pretrained("huggingface/time-series-transformer-tourism-monthly").to(
torch_device
)
batch = prepare_batch()
with torch.no_grad():
output = model(
past_values=batch["past_values"],
past_time_features=batch["past_time_features"],
past_observed_mask=batch["past_observed_mask"],
static_categorical_features=batch["static_categorical_features"],
static_real_features=batch["static_real_features"],
future_values=batch["future_values"],
future_time_features=batch["future_time_features"],
).last_hidden_state
expected_shape = torch.Size((64, model.config.context_length, model.config.d_model))
self.assertEqual(output.shape, expected_shape)
expected_slice = torch.tensor(
[[0.8196, -1.5131, 1.4620], [1.1268, -1.3238, 1.5997], [1.5098, -1.0715, 1.7359]], device=torch_device
)
self.assertTrue(torch.allclose(output[0, :3, :3], expected_slice, atol=TOLERANCE))
def test_inference_head(self):
model = TimeSeriesTransformerForPrediction.from_pretrained(
"huggingface/time-series-transformer-tourism-monthly"
).to(torch_device)
batch = prepare_batch("val-batch.pt")
with torch.no_grad():
output = model(
past_values=batch["past_values"],
past_time_features=batch["past_time_features"],
past_observed_mask=batch["past_observed_mask"],
static_categorical_features=batch["static_categorical_features"],
static_real_features=batch["static_real_features"],
future_time_features=batch["future_time_features"],
).encoder_last_hidden_state
expected_shape = torch.Size((64, model.config.context_length, model.config.d_model))
self.assertEqual(output.shape, expected_shape)
expected_slice = torch.tensor(
[[-1.2957, -1.0280, -0.6045], [-0.7017, -0.8193, -0.3717], [-1.0449, -0.8149, 0.1405]], device=torch_device
)
self.assertTrue(torch.allclose(output[0, :3, :3], expected_slice, atol=TOLERANCE))
def test_seq_to_seq_generation(self):
model = TimeSeriesTransformerForPrediction.from_pretrained(
"huggingface/time-series-transformer-tourism-monthly"
).to(torch_device)
batch = prepare_batch("val-batch.pt")
with torch.no_grad():
outputs = model.generate(
static_categorical_features=batch["static_categorical_features"],
static_real_features=batch["static_real_features"],
past_time_features=batch["past_time_features"],
past_values=batch["past_values"],
future_time_features=batch["future_time_features"],
past_observed_mask=batch["past_observed_mask"],
)
expected_shape = torch.Size((64, model.config.num_parallel_samples, model.config.prediction_length))
self.assertEqual(outputs.sequences.shape, expected_shape)
expected_slice = torch.tensor([2825.2749, 3584.9207, 6763.9951], device=torch_device)
mean_prediction = outputs.sequences.mean(dim=1)
self.assertTrue(torch.allclose(mean_prediction[0, -3:], expected_slice, rtol=1e-1))
| transformers/tests/models/time_series_transformer/test_modeling_time_series_transformer.py/0 | {
"file_path": "transformers/tests/models/time_series_transformer/test_modeling_time_series_transformer.py",
"repo_id": "transformers",
"token_count": 10424
} | 366 |
# Copyright 2023 The HuggingFace Team. All rights reserved.
#
# Licensed under the Apache License, Version 2.0 (the "License");
# you may not use this file except in compliance with the License.
# You may obtain a copy of the License at
#
# http://www.apache.org/licenses/LICENSE-2.0
#
# Unless required by applicable law or agreed to in writing, software
# distributed under the License is distributed on an "AS IS" BASIS,
# WITHOUT WARRANTIES OR CONDITIONS OF ANY KIND, either express or implied.
# See the License for the specific language governing permissions and
# limitations under the License.
import copy
import os
import pickle
import tempfile
import unittest
from transformers import UMT5Config, is_torch_available
from transformers.models.auto.modeling_auto import MODEL_FOR_SEQUENCE_CLASSIFICATION_MAPPING_NAMES
from transformers.testing_utils import (
require_sentencepiece,
require_tokenizers,
require_torch,
slow,
torch_device,
)
from transformers.utils import is_torch_fx_available
from ...generation.test_utils import GenerationTesterMixin
from ...test_configuration_common import ConfigTester
from ...test_modeling_common import ModelTesterMixin, _config_zero_init, ids_tensor
from ...test_pipeline_mixin import PipelineTesterMixin
if is_torch_fx_available():
from transformers.utils.fx import symbolic_trace
if is_torch_available():
import torch
from transformers import (
AutoTokenizer,
UMT5EncoderModel,
UMT5ForConditionalGeneration,
UMT5ForQuestionAnswering,
UMT5ForSequenceClassification,
UMT5ForTokenClassification,
UMT5Model,
)
# Copied from test.models.t5.test_modeling_t5.T5ModelTester with T5->UMT5
class UMT5ModelTester:
def __init__(
self,
parent,
vocab_size=99,
batch_size=13,
encoder_seq_length=7,
decoder_seq_length=7,
# For common tests
is_training=True,
use_attention_mask=True,
use_labels=False,
hidden_size=32,
num_hidden_layers=2,
num_attention_heads=4,
d_ff=37,
relative_attention_num_buckets=8,
dropout_rate=0.1,
initializer_factor=0.002,
eos_token_id=1,
pad_token_id=0,
decoder_start_token_id=0,
scope=None,
decoder_layers=None,
):
self.parent = parent
self.batch_size = batch_size
self.encoder_seq_length = encoder_seq_length
self.decoder_seq_length = decoder_seq_length
# For common tests
self.seq_length = self.decoder_seq_length
self.is_training = is_training
self.use_attention_mask = use_attention_mask
self.use_labels = use_labels
self.vocab_size = vocab_size
self.hidden_size = hidden_size
self.num_hidden_layers = num_hidden_layers
self.num_attention_heads = num_attention_heads
self.d_ff = d_ff
self.relative_attention_num_buckets = relative_attention_num_buckets
self.dropout_rate = dropout_rate
self.initializer_factor = initializer_factor
self.eos_token_id = eos_token_id
self.pad_token_id = pad_token_id
self.decoder_start_token_id = decoder_start_token_id
self.scope = None
self.decoder_layers = decoder_layers
def get_large_model_config(self):
return UMT5Config.from_pretrained("google/umt5-base")
def prepare_inputs_dict(
self,
config,
input_ids,
decoder_input_ids,
attention_mask=None,
decoder_attention_mask=None,
head_mask=None,
decoder_head_mask=None,
cross_attn_head_mask=None,
):
if attention_mask is None:
attention_mask = input_ids.ne(config.pad_token_id)
if decoder_attention_mask is None:
decoder_attention_mask = decoder_input_ids.ne(config.pad_token_id)
if head_mask is None:
head_mask = torch.ones(config.num_hidden_layers, config.num_attention_heads, device=torch_device)
if decoder_head_mask is None:
decoder_head_mask = torch.ones(config.num_decoder_layers, config.num_attention_heads, device=torch_device)
if cross_attn_head_mask is None:
cross_attn_head_mask = torch.ones(
config.num_decoder_layers, config.num_attention_heads, device=torch_device
)
return {
"input_ids": input_ids,
"decoder_input_ids": decoder_input_ids,
"attention_mask": attention_mask,
"decoder_attention_mask": decoder_attention_mask,
"head_mask": head_mask,
"decoder_head_mask": decoder_head_mask,
"cross_attn_head_mask": cross_attn_head_mask,
}
def prepare_config_and_inputs(self):
input_ids = ids_tensor([self.batch_size, self.encoder_seq_length], self.vocab_size)
decoder_input_ids = ids_tensor([self.batch_size, self.decoder_seq_length], self.vocab_size)
# we need to clamp the input ids here to avoid having pad token in between
# this is because for NllbMoe the position_ids are prepared such that
# all pad tokens have pos id = 2 and rest are between 2..seq_length
# and the seq_length here is seq_length - num_pad_tokens
# but when using past, there is no way of knowing if the past input ids had
# pad tokens in them, which results in incorrect seq_lenth and which in turn results in
# position_ids being off by num_pad_tokens in past input
input_ids = input_ids.clamp(self.pad_token_id + 2)
input_ids[:, -1] = self.eos_token_id # Eos Token
decoder_input_ids = decoder_input_ids.clamp(self.pad_token_id + 1)
config = self.get_config()
config.encoder_attention_heads = config.num_attention_heads
input_dict = self.prepare_inputs_dict(config, input_ids, decoder_input_ids)
return config, input_dict
def prepare_config_and_inputs_for_common(self):
config, inputs_dict = self.prepare_config_and_inputs()
return config, inputs_dict
def get_pipeline_config(self):
return UMT5Config(
vocab_size=166, # t5 forces 100 extra tokens
d_model=self.hidden_size,
d_ff=self.d_ff,
d_kv=self.hidden_size // self.num_attention_heads,
num_layers=self.num_hidden_layers,
num_decoder_layers=self.decoder_layers,
num_heads=self.num_attention_heads,
relative_attention_num_buckets=self.relative_attention_num_buckets,
dropout_rate=self.dropout_rate,
initializer_factor=self.initializer_factor,
eos_token_id=self.eos_token_id,
bos_token_id=self.pad_token_id,
pad_token_id=self.pad_token_id,
decoder_start_token_id=self.decoder_start_token_id,
)
def get_config(self):
return UMT5Config(
vocab_size=self.vocab_size,
d_model=self.hidden_size,
d_ff=self.d_ff,
d_kv=self.hidden_size // self.num_attention_heads,
num_layers=self.num_hidden_layers,
num_decoder_layers=self.decoder_layers,
num_heads=self.num_attention_heads,
relative_attention_num_buckets=self.relative_attention_num_buckets,
dropout_rate=self.dropout_rate,
initializer_factor=self.initializer_factor,
eos_token_id=self.eos_token_id,
bos_token_id=self.pad_token_id,
pad_token_id=self.pad_token_id,
decoder_start_token_id=self.decoder_start_token_id,
)
def create_and_check_model(
self,
config,
input_ids,
decoder_input_ids,
attention_mask,
decoder_attention_mask,
lm_labels,
):
model = UMT5Model(config=config)
model.to(torch_device)
model.eval()
result = model(
input_ids=input_ids,
decoder_input_ids=decoder_input_ids,
attention_mask=attention_mask,
decoder_attention_mask=decoder_attention_mask,
)
result = model(input_ids=input_ids, decoder_input_ids=decoder_input_ids)
decoder_output = result.last_hidden_state
decoder_past = result.past_key_values
encoder_output = result.encoder_last_hidden_state
self.parent.assertEqual(encoder_output.size(), (self.batch_size, self.encoder_seq_length, self.hidden_size))
self.parent.assertEqual(decoder_output.size(), (self.batch_size, self.decoder_seq_length, self.hidden_size))
# There should be `num_layers` key value embeddings stored in decoder_past
self.parent.assertEqual(len(decoder_past), config.num_layers)
# There should be a self attn key, a self attn value, a cross attn key and a cross attn value stored in each decoder_past tuple
self.parent.assertEqual(len(decoder_past[0]), 4)
def create_and_check_decoder_model_past(
self,
config,
input_ids,
decoder_input_ids,
attention_mask,
decoder_attention_mask,
lm_labels,
):
model = UMT5Model(config=config).get_decoder().to(torch_device).eval()
# first forward pass
outputs = model(input_ids, use_cache=True)
outputs_use_cache_conf = model(input_ids)
outputs_no_past = model(input_ids, use_cache=False)
self.parent.assertTrue(len(outputs) == len(outputs_use_cache_conf))
self.parent.assertTrue(len(outputs) == len(outputs_no_past) + 1)
output, past_key_values = outputs.to_tuple()
# create hypothetical next token and extent to next_input_ids
next_tokens = ids_tensor((self.batch_size, 1), config.vocab_size)
# append to next input_ids and
next_input_ids = torch.cat([input_ids, next_tokens], dim=-1)
output_from_no_past = model(next_input_ids)["last_hidden_state"]
output_from_past = model(next_tokens, past_key_values=past_key_values)["last_hidden_state"]
# select random slice
random_slice_idx = ids_tensor((1,), output_from_past.shape[-1]).item()
output_from_no_past_slice = output_from_no_past[:, -1, random_slice_idx].detach()
output_from_past_slice = output_from_past[:, 0, random_slice_idx].detach()
# test that outputs are equal for slice
self.parent.assertTrue(torch.allclose(output_from_past_slice, output_from_no_past_slice, atol=1e-3))
def create_and_check_model_fp16_forward(
self,
config,
input_dict,
):
model = UMT5Model(config=config).to(torch_device).half().eval()
output = model(**input_dict)["last_hidden_state"]
self.parent.assertFalse(torch.isnan(output).any().item())
def create_and_check_with_sequence_classification_head(
self,
config,
input_dict,
):
labels = torch.tensor([1] * self.batch_size, dtype=torch.long, device=torch_device)
model = UMT5ForSequenceClassification(config=config).to(torch_device).eval()
outputs = model(**input_dict, labels=labels)
# self.parent.assertEqual(len(outputs), 4)
self.parent.assertEqual(outputs["logits"].size(), (self.batch_size, config.num_labels))
self.parent.assertEqual(outputs["loss"].size(), ())
@require_torch
class UMT5ModelTest(ModelTesterMixin, GenerationTesterMixin, PipelineTesterMixin, unittest.TestCase):
all_model_classes = (
(UMT5Model, UMT5ForConditionalGeneration, UMT5ForSequenceClassification, UMT5ForQuestionAnswering)
if is_torch_available()
else ()
)
all_generative_model_classes = (UMT5ForConditionalGeneration,) if is_torch_available() else ()
pipeline_model_mapping = (
{
"conversational": UMT5ForConditionalGeneration,
"feature-extraction": UMT5Model,
"question-answering": UMT5ForQuestionAnswering,
"summarization": UMT5ForConditionalGeneration,
"text-classification": UMT5ForSequenceClassification,
"text2text-generation": UMT5ForConditionalGeneration,
"translation": UMT5ForConditionalGeneration,
"zero-shot": UMT5ForSequenceClassification,
}
if is_torch_available()
else {}
)
is_encoder_decoder = True
fx_compatible = False
test_pruning = False
test_missing_keys = True
test_torchscript = True
# The small UMT5 model needs higher percentages for CPU/MP tests
model_split_percents = [0.8, 0.9]
def setUp(self):
self.model_tester = UMT5ModelTester(self)
# `QAPipelineTests` is not working well with slow tokenizers (for some models) and we don't want to touch the file
# `src/transformers/data/processors/squad.py` (where this test fails for this model)
def is_pipeline_test_to_skip(
self, pipeline_test_casse_name, config_class, model_architecture, tokenizer_name, processor_name
):
if pipeline_test_casse_name == "QAPipelineTests" and not tokenizer_name.endswith("Fast"):
return True
return False
def _create_and_check_torch_fx_tracing(self, config, inputs_dict, output_loss=False):
if not is_torch_fx_available() or not self.fx_compatible:
return
configs_no_init = _config_zero_init(config) # To be sure we have no Nan
configs_no_init.return_dict = False
for model_class in self.all_model_classes:
if model_class.__name__ == "UMT5ForSequenceClassification":
continue
model = model_class(config=configs_no_init)
model.to(torch_device)
model.eval()
inputs = self._prepare_for_class(inputs_dict, model_class, return_labels=output_loss)
try:
if model.config.is_encoder_decoder:
model.config.use_cache = False # FSTM still requires this hack -> FSTM should probably be refactored similar to BART afterward
labels = inputs.get("labels", None)
input_names = [
"attention_mask",
"decoder_attention_mask",
"decoder_input_ids",
"input_features",
"input_ids",
"input_values",
]
if labels is not None:
input_names.append("labels")
filtered_inputs = {k: v for (k, v) in inputs.items() if k in input_names}
input_names = list(filtered_inputs.keys())
model_output = model(**filtered_inputs)
traced_model = symbolic_trace(model, input_names)
traced_output = traced_model(**filtered_inputs)
else:
input_names = [
"attention_mask",
"bbox",
"input_features",
"input_ids",
"input_values",
"pixel_values",
"token_type_ids",
"visual_feats",
"visual_pos",
]
labels = inputs.get("labels", None)
start_positions = inputs.get("start_positions", None)
end_positions = inputs.get("end_positions", None)
if labels is not None:
input_names.append("labels")
if start_positions is not None:
input_names.append("start_positions")
if end_positions is not None:
input_names.append("end_positions")
filtered_inputs = {k: v for (k, v) in inputs.items() if k in input_names}
input_names = list(filtered_inputs.keys())
if model.__class__.__name__ in set(MODEL_FOR_SEQUENCE_CLASSIFICATION_MAPPING_NAMES.values()) and (
not hasattr(model.config, "problem_type") or model.config.problem_type is None
):
model.config.problem_type = "single_label_classification"
traced_model = symbolic_trace(model, input_names)
traced_output = traced_model(**filtered_inputs)
model_output = model(**filtered_inputs)
except Exception as e:
self.fail(f"Couldn't trace module: {e}")
def flatten_output(output):
flatten = []
for x in output:
if isinstance(x, (tuple, list)):
flatten += flatten_output(x)
elif not isinstance(x, torch.Tensor):
continue
else:
flatten.append(x)
return flatten
model_output = flatten_output(model_output)
traced_output = flatten_output(traced_output)
num_outputs = len(model_output)
for i in range(num_outputs):
self.assertTrue(
torch.allclose(model_output[i], traced_output[i]),
f"traced {i}th output doesn't match model {i}th output for {model_class}",
)
# Test that the model can be serialized and restored properly
with tempfile.TemporaryDirectory() as tmp_dir_name:
pkl_file_name = os.path.join(tmp_dir_name, "model.pkl")
try:
with open(pkl_file_name, "wb") as f:
pickle.dump(traced_model, f)
with open(pkl_file_name, "rb") as f:
loaded = pickle.load(f)
except Exception as e:
self.fail(f"Couldn't serialize / deserialize the traced model: {e}")
loaded_output = loaded(**filtered_inputs)
loaded_output = flatten_output(loaded_output)
for i in range(num_outputs):
self.assertTrue(
torch.allclose(model_output[i], loaded_output[i]),
f"serialized model {i}th output doesn't match model {i}th output for {model_class}",
)
# Avoid memory leak. Without this, each call increase RAM usage by ~20MB.
# (Even with this call, there are still memory leak by ~0.04MB)
self.clear_torch_jit_class_registry()
# UMT5ForSequenceClassification does not support inputs_embeds
def test_inputs_embeds(self):
config, inputs_dict = self.model_tester.prepare_config_and_inputs_for_common()
for model_class in (UMT5Model, UMT5ForConditionalGeneration, UMT5ForQuestionAnswering):
model = model_class(config)
model.to(torch_device)
model.eval()
inputs = copy.deepcopy(self._prepare_for_class(inputs_dict, model_class))
if not self.is_encoder_decoder:
input_ids = inputs["input_ids"]
del inputs["input_ids"]
else:
encoder_input_ids = inputs["input_ids"]
decoder_input_ids = inputs.get("decoder_input_ids", encoder_input_ids)
del inputs["input_ids"]
inputs.pop("decoder_input_ids", None)
wte = model.get_input_embeddings()
if not self.is_encoder_decoder:
inputs["inputs_embeds"] = wte(input_ids)
else:
inputs["inputs_embeds"] = wte(encoder_input_ids)
inputs["decoder_inputs_embeds"] = wte(decoder_input_ids)
with torch.no_grad():
model(**inputs)[0]
def test_with_sequence_classification_head(self):
config_and_inputs = self.model_tester.prepare_config_and_inputs()
self.model_tester.create_and_check_with_sequence_classification_head(*config_and_inputs)
@unittest.skip("Test has a segmentation fault on torch 1.8.0")
def test_export_to_onnx(self):
config_and_inputs = self.model_tester.prepare_config_and_inputs()
model = UMT5Model(config_and_inputs[0]).to(torch_device)
with tempfile.TemporaryDirectory() as tmpdirname:
torch.onnx.export(
model,
(config_and_inputs[1], config_and_inputs[3], config_and_inputs[2]),
f"{tmpdirname}/t5_test.onnx",
export_params=True,
opset_version=9,
input_names=["input_ids", "decoder_input_ids"],
)
@unittest.skipIf(torch_device == "cpu", "Cant do half precision")
def test_model_fp16_forward(self):
config_and_inputs = self.model_tester.prepare_config_and_inputs()
self.model_tester.create_and_check_model_fp16_forward(*config_and_inputs)
def test_generate_with_head_masking(self):
attention_names = ["encoder_attentions", "decoder_attentions", "cross_attentions"]
config_and_inputs = self.model_tester.prepare_config_and_inputs()
config = config_and_inputs[0]
model = UMT5ForConditionalGeneration(config).eval()
model.to(torch_device)
head_masking = {
"head_mask": torch.zeros(config.num_layers, config.num_heads, device=torch_device),
"decoder_head_mask": torch.zeros(config.num_decoder_layers, config.num_heads, device=torch_device),
"cross_attn_head_mask": torch.zeros(config.num_decoder_layers, config.num_heads, device=torch_device),
}
for attn_name, (name, mask) in zip(attention_names, head_masking.items()):
head_masks = {name: mask}
# Explicitly pass decoder_head_mask as it is required from T5 model when head_mask specified
if name == "head_mask":
head_masks["decoder_head_mask"] = torch.ones(
config.num_decoder_layers, config.num_heads, device=torch_device
)
out = model.generate(
config_and_inputs[1]["input_ids"],
num_beams=1,
max_length=3,
output_attentions=True,
return_dict_in_generate=True,
**head_masks,
)
# We check the state of decoder_attentions and cross_attentions just from the last step
attn_weights = out[attn_name] if attn_name == attention_names[0] else out[attn_name][-1]
self.assertEqual(sum([w.sum().item() for w in attn_weights]), 0.0)
@unittest.skip("Does not work on the tiny model as we keep hitting edge cases.")
def test_disk_offload(self):
pass
@unittest.skip(
reason="This architecure seem to not compute gradients properly when using GC, check: https://github.com/huggingface/transformers/pull/27124"
)
def test_training_gradient_checkpointing(self):
pass
@unittest.skip(
reason="This architecure seem to not compute gradients properly when using GC, check: https://github.com/huggingface/transformers/pull/27124"
)
def test_training_gradient_checkpointing_use_reentrant(self):
pass
@unittest.skip(
reason="This architecure seem to not compute gradients properly when using GC, check: https://github.com/huggingface/transformers/pull/27124"
)
def test_training_gradient_checkpointing_use_reentrant_false(self):
pass
# Copied from tests.models.t5.test_modeling_t5.T5EncoderOnlyModelTester with T5->UMT5
class UMT5EncoderOnlyModelTester:
def __init__(
self,
parent,
vocab_size=99,
batch_size=13,
encoder_seq_length=7,
# For common tests
use_attention_mask=True,
hidden_size=32,
num_hidden_layers=2,
num_attention_heads=4,
d_ff=37,
relative_attention_num_buckets=8,
is_training=False,
dropout_rate=0.1,
initializer_factor=0.002,
is_encoder_decoder=False,
eos_token_id=1,
pad_token_id=0,
scope=None,
):
self.parent = parent
self.batch_size = batch_size
self.encoder_seq_length = encoder_seq_length
# For common tests
self.seq_length = self.encoder_seq_length
self.use_attention_mask = use_attention_mask
self.vocab_size = vocab_size
self.hidden_size = hidden_size
self.num_hidden_layers = num_hidden_layers
self.num_attention_heads = num_attention_heads
self.d_ff = d_ff
self.relative_attention_num_buckets = relative_attention_num_buckets
self.dropout_rate = dropout_rate
self.initializer_factor = initializer_factor
self.eos_token_id = eos_token_id
self.pad_token_id = pad_token_id
self.is_encoder_decoder = is_encoder_decoder
self.scope = None
self.is_training = is_training
def get_large_model_config(self):
return UMT5Config.from_pretrained("t5-base")
def prepare_config_and_inputs(self):
input_ids = ids_tensor([self.batch_size, self.encoder_seq_length], self.vocab_size)
attention_mask = None
if self.use_attention_mask:
attention_mask = ids_tensor([self.batch_size, self.encoder_seq_length], vocab_size=2)
config = UMT5Config(
vocab_size=self.vocab_size,
d_model=self.hidden_size,
d_ff=self.d_ff,
d_kv=self.hidden_size // self.num_attention_heads,
num_layers=self.num_hidden_layers,
num_heads=self.num_attention_heads,
relative_attention_num_buckets=self.relative_attention_num_buckets,
dropout_rate=self.dropout_rate,
initializer_factor=self.initializer_factor,
eos_token_id=self.eos_token_id,
bos_token_id=self.pad_token_id,
pad_token_id=self.pad_token_id,
is_encoder_decoder=self.is_encoder_decoder,
)
return (
config,
input_ids,
attention_mask,
)
def create_and_check_model(
self,
config,
input_ids,
attention_mask,
):
model = UMT5EncoderModel(config=config)
model.to(torch_device)
model.eval()
result = model(
input_ids=input_ids,
attention_mask=attention_mask,
)
result = model(input_ids=input_ids)
encoder_output = result.last_hidden_state
self.parent.assertEqual(encoder_output.size(), (self.batch_size, self.encoder_seq_length, self.hidden_size))
def create_and_check_model_fp16_forward(
self,
config,
input_ids,
attention_mask,
):
model = UMT5EncoderModel(config=config).to(torch_device).half().eval()
output = model(input_ids, attention_mask=attention_mask)["last_hidden_state"]
self.parent.assertFalse(torch.isnan(output).any().item())
def create_and_check_with_token_classification_head(
self,
config,
input_ids,
attention_mask,
):
labels = torch.tensor([1] * self.seq_length * self.batch_size, dtype=torch.long, device=torch_device)
model = UMT5ForTokenClassification(config=config).to(torch_device).eval()
outputs = model(
input_ids=input_ids,
labels=labels,
attention_mask=attention_mask,
)
self.parent.assertEqual(outputs["logits"].size(), (self.batch_size, self.seq_length, config.num_labels))
self.parent.assertEqual(outputs["loss"].size(), ())
def prepare_config_and_inputs_for_common(self):
config_and_inputs = self.prepare_config_and_inputs()
(
config,
input_ids,
attention_mask,
) = config_and_inputs
inputs_dict = {
"input_ids": input_ids,
"attention_mask": attention_mask,
}
return config, inputs_dict
# Copied from tests.models.t5.test_modeling_t5.T5EncoderOnlyModelTest with T5->UMT5
class UMT5EncoderOnlyModelTest(ModelTesterMixin, PipelineTesterMixin, unittest.TestCase):
all_model_classes = (UMT5EncoderModel, UMT5ForTokenClassification) if is_torch_available() else ()
test_pruning = False
test_resize_embeddings = False
test_model_parallel = True
pipeline_model_mapping = (
{
"token-classification": UMT5ForTokenClassification,
}
if is_torch_available()
else {}
)
all_parallelizable_model_classes = (UMT5EncoderModel,) if is_torch_available() else ()
def setUp(self):
self.model_tester = UMT5EncoderOnlyModelTester(self)
self.config_tester = ConfigTester(self, config_class=UMT5Config, d_model=37)
def test_config(self):
self.config_tester.run_common_tests()
def test_model(self):
config_and_inputs = self.model_tester.prepare_config_and_inputs()
self.model_tester.create_and_check_model(*config_and_inputs)
@unittest.skipIf(torch_device == "cpu", "Cant do half precision")
def test_model_fp16_forward(self):
config_and_inputs = self.model_tester.prepare_config_and_inputs()
self.model_tester.create_and_check_model_fp16_forward(*config_and_inputs)
def test_with_token_classification_head(self):
config_and_inputs = self.model_tester.prepare_config_and_inputs()
self.model_tester.create_and_check_with_token_classification_head(*config_and_inputs)
@require_torch
@require_sentencepiece
@require_tokenizers
class Umt5IntegrationTest(unittest.TestCase):
@slow
@unittest.skip(
"Unless we stop stripping left and right by default for all special tokens, the expected ids obtained here will not match the original ones. Wait for https://github.com/huggingface/transformers/pull/23909 to be merged"
)
def test_small_integration_test(self):
"""
For comparison run the kaggle notbook available here : https://www.kaggle.com/arthurzucker/umt5-inference
"""
model = UMT5ForConditionalGeneration.from_pretrained("google/umt5-small", return_dict=True).to(torch_device)
tokenizer = AutoTokenizer.from_pretrained("google/umt5-small", use_fast=False, legacy=False)
input_text = [
"Bonjour monsieur <extra_id_0> bien <extra_id_1>.",
"No se como puedo <extra_id_0>.",
"This is the reason why we <extra_id_0> them.",
"The <extra_id_0> walks in <extra_id_1>, seats",
"A <extra_id_0> walks into a bar and orders a <extra_id_1> with <extra_id_2> pinch of <extra_id_3>.",
]
input_ids = tokenizer(input_text, return_tensors="pt", padding=True).input_ids
# fmt: off
EXPECTED_IDS = torch.tensor(
[
[ 38530, 210703, 256299, 1410, 256298, 274, 1, 0,0, 0, 0, 0, 0, 0, 0, 0,0, 0],
[ 826, 321, 671, 25922, 256299, 274, 1, 0,0, 0, 0, 0, 0, 0, 0, 0,0, 0],
[ 1460, 339, 312, 19014, 10620, 758, 256299, 2355,274, 1, 0, 0, 0, 0, 0, 0,0, 0],
[ 517, 256299, 14869, 281, 301, 256298, 275, 119983,1, 0, 0, 0, 0, 0, 0, 0,0, 0],
[ 320, 256299, 14869, 281, 2234, 289, 2275, 333,61391, 289, 256298, 543, 256297, 168714, 329, 256296,274, 1],
]
)
# fmt: on
torch.testing.assert_allclose(input_ids, EXPECTED_IDS)
generated_ids = model.generate(input_ids.to(torch_device))
EXPECTED_FILLING = [
"<pad><extra_id_0> et<extra_id_1> [eod] <extra_id_2><extra_id_55>.. [eod] 💐 💐 💐 💐 💐 💐 💐 💐 💐 💐 💐 <extra_id_56>ajšietosto<extra_id_56>lleux<extra_id_19><extra_id_6>ajšie</s>",
"<pad><extra_id_0>.<extra_id_1>.,<0x0A>...spech <0x0A><extra_id_20> <extra_id_21></s><pad><pad><pad><pad><pad><pad><pad><pad><pad><pad><pad><pad><pad><pad><pad><pad><pad><pad><pad><pad><pad><pad><pad><pad><pad><pad><pad><pad><pad><pad><pad>",
"<pad><extra_id_0> are not going to be a part of the world. We are not going to be a part of<extra_id_1> and<extra_id_2><0x0A><extra_id_48>.<extra_id_48></s><pad><pad><pad><pad><pad><pad><pad><pad><pad><pad><pad><pad><pad><pad><pad><pad>",
"<pad><extra_id_0> door<extra_id_1>, the door<extra_id_2> 피해[/</s><pad><pad><pad><pad><pad><pad><pad><pad><pad><pad><pad><pad><pad><pad><pad><pad><pad><pad><pad><pad><pad><pad><pad><pad><pad><pad><pad><pad><pad><pad><pad><pad><pad><pad><pad>",
"<pad><extra_id_0>nyone who<extra_id_1> drink<extra_id_2> a<extra_id_3> alcohol<extra_id_4> A<extra_id_5> A. This<extra_id_6> I<extra_id_7><extra_id_52><extra_id_53></s><pad><pad><pad><pad><pad><pad><pad><pad><pad><pad><pad><pad><pad><pad><pad><pad><pad><pad><pad><pad><pad><pad><pad><pad>",
]
filling = tokenizer.batch_decode(generated_ids)
self.assertEqual(filling, EXPECTED_FILLING)
| transformers/tests/models/umt5/test_modeling_umt5.py/0 | {
"file_path": "transformers/tests/models/umt5/test_modeling_umt5.py",
"repo_id": "transformers",
"token_count": 15614
} | 367 |
# coding=utf-8
# Copyright 2021 The HuggingFace Inc. team. All rights reserved.
#
# Licensed under the Apache License, Version 2.0 (the "License");
# you may not use this file except in compliance with the License.
# You may obtain a copy of the License at
#
# http://www.apache.org/licenses/LICENSE-2.0
#
# Unless required by applicable law or agreed to in writing, software
# distributed under the License is distributed on an "AS IS" BASIS,
# WITHOUT WARRANTIES OR CONDITIONS OF ANY KIND, either express or implied.
# See the License for the specific language governing permissions and
# limitations under the License.
""" Testing suite for the TensorFlow ViT model. """
from __future__ import annotations
import inspect
import unittest
from transformers import ViTConfig
from transformers.testing_utils import require_tf, require_vision, slow
from transformers.utils import cached_property, is_tf_available, is_vision_available
from ...test_configuration_common import ConfigTester
from ...test_modeling_tf_common import TFModelTesterMixin, floats_tensor, ids_tensor
from ...test_pipeline_mixin import PipelineTesterMixin
if is_tf_available():
import tensorflow as tf
from transformers import TFViTForImageClassification, TFViTModel
from transformers.modeling_tf_utils import keras
if is_vision_available():
from PIL import Image
from transformers import ViTImageProcessor
class TFViTModelTester:
def __init__(
self,
parent,
batch_size=13,
image_size=30,
patch_size=2,
num_channels=3,
is_training=True,
use_labels=True,
hidden_size=32,
num_hidden_layers=2,
num_attention_heads=4,
intermediate_size=37,
hidden_act="gelu",
hidden_dropout_prob=0.1,
attention_probs_dropout_prob=0.1,
type_sequence_label_size=10,
initializer_range=0.02,
num_labels=3,
scope=None,
):
self.parent = parent
self.batch_size = batch_size
self.image_size = image_size
self.patch_size = patch_size
self.num_channels = num_channels
self.is_training = is_training
self.use_labels = use_labels
self.hidden_size = hidden_size
self.num_hidden_layers = num_hidden_layers
self.num_attention_heads = num_attention_heads
self.intermediate_size = intermediate_size
self.hidden_act = hidden_act
self.hidden_dropout_prob = hidden_dropout_prob
self.attention_probs_dropout_prob = attention_probs_dropout_prob
self.type_sequence_label_size = type_sequence_label_size
self.initializer_range = initializer_range
self.scope = scope
# in ViT, the seq length equals the number of patches + 1 (we add 1 for the [CLS] token)
num_patches = (image_size // patch_size) ** 2
self.seq_length = num_patches + 1
def prepare_config_and_inputs(self):
pixel_values = floats_tensor([self.batch_size, self.num_channels, self.image_size, self.image_size])
labels = None
if self.use_labels:
labels = ids_tensor([self.batch_size], self.type_sequence_label_size)
config = self.get_config()
return config, pixel_values, labels
def get_config(self):
return ViTConfig(
image_size=self.image_size,
patch_size=self.patch_size,
num_channels=self.num_channels,
hidden_size=self.hidden_size,
num_hidden_layers=self.num_hidden_layers,
num_attention_heads=self.num_attention_heads,
intermediate_size=self.intermediate_size,
hidden_act=self.hidden_act,
hidden_dropout_prob=self.hidden_dropout_prob,
attention_probs_dropout_prob=self.attention_probs_dropout_prob,
is_decoder=False,
initializer_range=self.initializer_range,
)
def create_and_check_model(self, config, pixel_values, labels):
model = TFViTModel(config=config)
result = model(pixel_values, training=False)
self.parent.assertEqual(result.last_hidden_state.shape, (self.batch_size, self.seq_length, self.hidden_size))
# Test with an image with different size than the one specified in config.
image_size = self.image_size // 2
pixel_values = pixel_values[:, :, :image_size, :image_size]
result = model(pixel_values, interpolate_pos_encoding=True, training=False)
seq_length = (image_size // self.patch_size) ** 2 + 1
self.parent.assertEqual(result.last_hidden_state.shape, (self.batch_size, seq_length, self.hidden_size))
def create_and_check_for_image_classification(self, config, pixel_values, labels):
config.num_labels = self.type_sequence_label_size
model = TFViTForImageClassification(config)
result = model(pixel_values, labels=labels, training=False)
self.parent.assertEqual(result.logits.shape, (self.batch_size, self.type_sequence_label_size))
# Test with an image with different size than the one specified in config.
image_size = self.image_size // 2
pixel_values = pixel_values[:, :, :image_size, :image_size]
result = model(pixel_values, interpolate_pos_encoding=True, training=False)
self.parent.assertEqual(result.logits.shape, (self.batch_size, self.type_sequence_label_size))
# test greyscale images
config.num_channels = 1
model = TFViTForImageClassification(config)
pixel_values = floats_tensor([self.batch_size, 1, self.image_size, self.image_size])
result = model(pixel_values)
self.parent.assertEqual(result.logits.shape, (self.batch_size, self.type_sequence_label_size))
def prepare_config_and_inputs_for_common(self):
config_and_inputs = self.prepare_config_and_inputs()
config, pixel_values, labels = config_and_inputs
inputs_dict = {"pixel_values": pixel_values}
return config, inputs_dict
@require_tf
class TFViTModelTest(TFModelTesterMixin, PipelineTesterMixin, unittest.TestCase):
"""
Here we also overwrite some of the tests of test_modeling_tf_common.py, as ViT does not use input_ids, inputs_embeds,
attention_mask and seq_length.
"""
all_model_classes = (TFViTModel, TFViTForImageClassification) if is_tf_available() else ()
pipeline_model_mapping = (
{"feature-extraction": TFViTModel, "image-classification": TFViTForImageClassification}
if is_tf_available()
else {}
)
test_resize_embeddings = False
test_head_masking = False
test_onnx = False
def setUp(self):
self.model_tester = TFViTModelTester(self)
self.config_tester = ConfigTester(self, config_class=ViTConfig, has_text_modality=False, hidden_size=37)
def test_config(self):
self.config_tester.run_common_tests()
@unittest.skip(reason="ViT does not use inputs_embeds")
def test_inputs_embeds(self):
pass
@unittest.skip(reason="ViT does not use inputs_embeds")
def test_graph_mode_with_inputs_embeds(self):
pass
def test_model_common_attributes(self):
config, _ = self.model_tester.prepare_config_and_inputs_for_common()
for model_class in self.all_model_classes:
model = model_class(config)
self.assertIsInstance(model.get_input_embeddings(), (keras.layers.Layer))
x = model.get_output_embeddings()
self.assertTrue(x is None or isinstance(x, keras.layers.Layer))
def test_forward_signature(self):
config, _ = self.model_tester.prepare_config_and_inputs_for_common()
for model_class in self.all_model_classes:
model = model_class(config)
signature = inspect.signature(model.call)
# signature.parameters is an OrderedDict => so arg_names order is deterministic
arg_names = [*signature.parameters.keys()]
expected_arg_names = ["pixel_values"]
self.assertListEqual(arg_names[:1], expected_arg_names)
def test_model(self):
config_and_inputs = self.model_tester.prepare_config_and_inputs()
self.model_tester.create_and_check_model(*config_and_inputs)
def test_for_image_classification(self):
config_and_inputs = self.model_tester.prepare_config_and_inputs()
self.model_tester.create_and_check_for_image_classification(*config_and_inputs)
@slow
def test_model_from_pretrained(self):
model = TFViTModel.from_pretrained("google/vit-base-patch16-224")
self.assertIsNotNone(model)
# We will verify our results on an image of cute cats
def prepare_img():
image = Image.open("./tests/fixtures/tests_samples/COCO/000000039769.png")
return image
@require_tf
@require_vision
class TFViTModelIntegrationTest(unittest.TestCase):
@cached_property
def default_image_processor(self):
return ViTImageProcessor.from_pretrained("google/vit-base-patch16-224") if is_vision_available() else None
@slow
def test_inference_image_classification_head(self):
model = TFViTForImageClassification.from_pretrained("google/vit-base-patch16-224")
image_processor = self.default_image_processor
image = prepare_img()
inputs = image_processor(images=image, return_tensors="tf")
# forward pass
outputs = model(**inputs)
# verify the logits
expected_shape = tf.TensorShape((1, 1000))
self.assertEqual(outputs.logits.shape, expected_shape)
expected_slice = tf.constant([-0.2744, 0.8215, -0.0836])
tf.debugging.assert_near(outputs.logits[0, :3], expected_slice, atol=1e-4)
| transformers/tests/models/vit/test_modeling_tf_vit.py/0 | {
"file_path": "transformers/tests/models/vit/test_modeling_tf_vit.py",
"repo_id": "transformers",
"token_count": 3920
} | 368 |
# coding=utf-8
# Copyright 2021 The HuggingFace Inc. team. All rights reserved.
#
# Licensed under the Apache License, Version 2.0 (the "License");
# you may not use this file except in compliance with the License.
# You may obtain a copy of the License at
#
# http://www.apache.org/licenses/LICENSE-2.0
#
# Unless required by applicable law or agreed to in writing, software
# distributed under the License is distributed on an "AS IS" BASIS,
# WITHOUT WARRANTIES OR CONDITIONS OF ANY KIND, either express or implied.
# See the License for the specific language governing permissions and
# limitations under the License.
import tempfile
import unittest
import transformers
from transformers import XGLMConfig, XGLMTokenizer, is_flax_available, is_torch_available
from transformers.testing_utils import is_pt_flax_cross_test, require_flax, require_sentencepiece, slow
from ...generation.test_flax_utils import FlaxGenerationTesterMixin
from ...test_modeling_flax_common import FlaxModelTesterMixin, floats_tensor, ids_tensor, random_attention_mask
if is_flax_available():
import jax
import jax.numpy as jnp
import numpy as np
from transformers.modeling_flax_pytorch_utils import (
convert_pytorch_state_dict_to_flax,
load_flax_weights_in_pytorch_model,
)
from transformers.models.xglm.modeling_flax_xglm import FlaxXGLMForCausalLM, FlaxXGLMModel
if is_torch_available():
import torch
@require_flax
class FlaxXGLMModelTester:
def __init__(
self,
parent,
batch_size=14,
seq_length=7,
is_training=True,
use_input_mask=True,
use_labels=True,
vocab_size=99,
d_model=32,
num_hidden_layers=2,
num_attention_heads=4,
ffn_dim=37,
activation_function="gelu",
activation_dropout=0.1,
attention_dropout=0.1,
max_position_embeddings=512,
initializer_range=0.02,
scope=None,
):
self.parent = parent
self.batch_size = batch_size
self.seq_length = seq_length
self.is_training = is_training
self.use_input_mask = use_input_mask
self.use_labels = use_labels
self.vocab_size = vocab_size
self.hidden_size = d_model
self.num_hidden_layers = num_hidden_layers
self.num_attention_heads = num_attention_heads
self.ffn_dim = ffn_dim
self.activation_function = activation_function
self.activation_dropout = activation_dropout
self.attention_dropout = attention_dropout
self.max_position_embeddings = max_position_embeddings
self.initializer_range = initializer_range
self.scope = None
self.bos_token_id = 0
self.eos_token_id = 2
self.pad_token_id = 1
def prepare_config_and_inputs(self):
input_ids = np.clip(ids_tensor([self.batch_size, self.seq_length], self.vocab_size), 3, self.vocab_size)
input_mask = None
if self.use_input_mask:
input_mask = random_attention_mask([self.batch_size, self.seq_length])
config = XGLMConfig(
vocab_size=self.vocab_size,
d_model=self.hidden_size,
num_layers=self.num_hidden_layers,
attention_heads=self.num_attention_heads,
ffn_dim=self.ffn_dim,
activation_function=self.activation_function,
activation_dropout=self.activation_dropout,
attention_dropout=self.attention_dropout,
max_position_embeddings=self.max_position_embeddings,
initializer_range=self.initializer_range,
use_cache=True,
bos_token_id=self.bos_token_id,
eos_token_id=self.eos_token_id,
pad_token_id=self.pad_token_id,
)
return (config, input_ids, input_mask)
def prepare_config_and_inputs_for_common(self):
config_and_inputs = self.prepare_config_and_inputs()
config, input_ids, attention_mask = config_and_inputs
inputs_dict = {"input_ids": input_ids, "attention_mask": attention_mask}
return config, inputs_dict
def prepare_config_and_inputs_for_decoder(self):
config, input_ids, attention_mask = self.prepare_config_and_inputs()
encoder_hidden_states = floats_tensor([self.batch_size, self.seq_length, self.hidden_size])
encoder_attention_mask = ids_tensor([self.batch_size, self.seq_length], vocab_size=2)
return (
config,
input_ids,
attention_mask,
encoder_hidden_states,
encoder_attention_mask,
)
def check_use_cache_forward(self, model_class_name, config, input_ids, attention_mask):
max_decoder_length = 20
model = model_class_name(config)
past_key_values = model.init_cache(input_ids.shape[0], max_decoder_length)
attention_mask = jnp.ones((input_ids.shape[0], max_decoder_length), dtype="i4")
position_ids = jnp.broadcast_to(
jnp.arange(input_ids.shape[-1] - 1)[None, :], (input_ids.shape[0], input_ids.shape[-1] - 1)
)
outputs_cache = model(
input_ids[:, :-1],
attention_mask=attention_mask,
past_key_values=past_key_values,
position_ids=position_ids,
)
position_ids = jnp.array(input_ids.shape[0] * [[input_ids.shape[-1] - 1]], dtype="i4")
outputs_cache_next = model(
input_ids[:, -1:],
attention_mask=attention_mask,
past_key_values=outputs_cache.past_key_values,
position_ids=position_ids,
)
outputs = model(input_ids)
diff = np.max(np.abs((outputs_cache_next[0][:, -1, :5] - outputs[0][:, -1, :5])))
self.parent.assertTrue(diff < 1e-3, msg=f"Max diff is {diff}")
def check_use_cache_forward_with_attn_mask(self, model_class_name, config, input_ids, attention_mask):
max_decoder_length = 20
model = model_class_name(config)
attention_mask_cache = jnp.concatenate(
[attention_mask, jnp.zeros((attention_mask.shape[0], max_decoder_length - attention_mask.shape[1]))],
axis=-1,
)
past_key_values = model.init_cache(input_ids.shape[0], max_decoder_length)
position_ids = jnp.broadcast_to(
jnp.arange(input_ids.shape[-1] - 1)[None, :], (input_ids.shape[0], input_ids.shape[-1] - 1)
)
outputs_cache = model(
input_ids[:, :-1],
attention_mask=attention_mask_cache,
past_key_values=past_key_values,
position_ids=position_ids,
)
position_ids = jnp.array(input_ids.shape[0] * [[input_ids.shape[-1] - 1]], dtype="i4")
outputs_cache_next = model(
input_ids[:, -1:],
past_key_values=outputs_cache.past_key_values,
attention_mask=attention_mask_cache,
position_ids=position_ids,
)
outputs = model(input_ids, attention_mask=attention_mask)
diff = np.max(np.abs((outputs_cache_next[0][:, -1, :5] - outputs[0][:, -1, :5])))
self.parent.assertTrue(diff < 1e-3, msg=f"Max diff is {diff}")
@require_sentencepiece
@require_flax
class FlaxXGLMModelTest(FlaxModelTesterMixin, FlaxGenerationTesterMixin, unittest.TestCase):
all_model_classes = (FlaxXGLMModel, FlaxXGLMForCausalLM) if is_flax_available() else ()
all_generative_model_classes = (FlaxXGLMForCausalLM,) if is_flax_available() else ()
def setUp(self):
self.model_tester = FlaxXGLMModelTester(self)
def test_use_cache_forward(self):
for model_class_name in self.all_model_classes:
config, input_ids, attention_mask = self.model_tester.prepare_config_and_inputs()
self.model_tester.check_use_cache_forward(model_class_name, config, input_ids, attention_mask)
def test_use_cache_forward_with_attn_mask(self):
for model_class_name in self.all_model_classes:
config, input_ids, attention_mask = self.model_tester.prepare_config_and_inputs()
self.model_tester.check_use_cache_forward_with_attn_mask(
model_class_name, config, input_ids, attention_mask
)
@slow
def test_batch_generation(self):
tokenizer = XGLMTokenizer.from_pretrained("XGLM", padding_side="left")
inputs = tokenizer(["Hello this is a long string", "Hey"], return_tensors="np", padding=True, truncation=True)
model = FlaxXGLMForCausalLM.from_pretrained("facebook/xglm-564M")
model.config.num_beams = 1
model.config.do_sample = False
jit_generate = jax.jit(model.generate)
output_sequences = jit_generate(inputs["input_ids"], attention_mask=inputs["attention_mask"]).sequences
output_string = tokenizer.batch_decode(output_sequences, skip_special_tokens=True)
expected_string = [
"Hello this is a long string of questions, but I'm not sure if I'm",
"Hey, I'm a newbie to the forum and I'",
]
self.assertListEqual(output_string, expected_string)
# overwrite from common since `attention_mask` in combination
# with `causal_mask` behaves slighly differently
@is_pt_flax_cross_test
def test_equivalence_pt_to_flax(self):
config, inputs_dict = self.model_tester.prepare_config_and_inputs_for_common()
for model_class in self.all_model_classes:
with self.subTest(model_class.__name__):
# prepare inputs
prepared_inputs_dict = self._prepare_for_class(inputs_dict, model_class)
pt_inputs = {k: torch.tensor(v.tolist()) for k, v in prepared_inputs_dict.items()}
# load corresponding PyTorch class
pt_model_class_name = model_class.__name__[4:] # Skip the "Flax" at the beginning
pt_model_class = getattr(transformers, pt_model_class_name)
batch_size, seq_length = pt_inputs["input_ids"].shape
rnd_start_indices = np.random.randint(0, seq_length - 1, size=(batch_size,))
for batch_idx, start_index in enumerate(rnd_start_indices):
pt_inputs["attention_mask"][batch_idx, :start_index] = 0
pt_inputs["attention_mask"][batch_idx, start_index:] = 1
prepared_inputs_dict["attention_mask"][batch_idx, :start_index] = 0
prepared_inputs_dict["attention_mask"][batch_idx, start_index:] = 1
pt_model = pt_model_class(config).eval()
# Flax models don't use the `use_cache` option and cache is not returned as a default.
# So we disable `use_cache` here for PyTorch model.
pt_model.config.use_cache = False
fx_model = model_class(config, dtype=jnp.float32)
fx_state = convert_pytorch_state_dict_to_flax(pt_model.state_dict(), fx_model)
fx_model.params = fx_state
with torch.no_grad():
pt_outputs = pt_model(**pt_inputs).to_tuple()
fx_outputs = fx_model(**prepared_inputs_dict).to_tuple()
self.assertEqual(len(fx_outputs), len(pt_outputs), "Output lengths differ between Flax and PyTorch")
for fx_output, pt_output in zip(fx_outputs, pt_outputs):
self.assert_almost_equals(fx_output[:, -1], pt_output[:, -1].numpy(), 4e-2)
with tempfile.TemporaryDirectory() as tmpdirname:
pt_model.save_pretrained(tmpdirname)
fx_model_loaded = model_class.from_pretrained(tmpdirname, from_pt=True)
fx_outputs_loaded = fx_model_loaded(**prepared_inputs_dict).to_tuple()
self.assertEqual(
len(fx_outputs_loaded), len(pt_outputs), "Output lengths differ between Flax and PyTorch"
)
for fx_output_loaded, pt_output in zip(fx_outputs_loaded, pt_outputs):
self.assert_almost_equals(fx_output_loaded[:, -1], pt_output[:, -1].numpy(), 4e-2)
# overwrite from common since `attention_mask` in combination
# with `causal_mask` behaves slighly differently
@is_pt_flax_cross_test
def test_equivalence_flax_to_pt(self):
config, inputs_dict = self.model_tester.prepare_config_and_inputs_for_common()
for model_class in self.all_model_classes:
with self.subTest(model_class.__name__):
# prepare inputs
prepared_inputs_dict = self._prepare_for_class(inputs_dict, model_class)
pt_inputs = {k: torch.tensor(v.tolist()) for k, v in prepared_inputs_dict.items()}
# load corresponding PyTorch class
pt_model_class_name = model_class.__name__[4:] # Skip the "Flax" at the beginning
pt_model_class = getattr(transformers, pt_model_class_name)
pt_model = pt_model_class(config).eval()
pt_model.config.use_cache = False
fx_model = model_class(config, dtype=jnp.float32)
pt_model = load_flax_weights_in_pytorch_model(pt_model, fx_model.params)
batch_size, seq_length = pt_inputs["input_ids"].shape
rnd_start_indices = np.random.randint(0, seq_length - 1, size=(batch_size,))
for batch_idx, start_index in enumerate(rnd_start_indices):
pt_inputs["attention_mask"][batch_idx, :start_index] = 0
pt_inputs["attention_mask"][batch_idx, start_index:] = 1
prepared_inputs_dict["attention_mask"][batch_idx, :start_index] = 0
prepared_inputs_dict["attention_mask"][batch_idx, start_index:] = 1
# make sure weights are tied in PyTorch
pt_model.tie_weights()
with torch.no_grad():
pt_outputs = pt_model(**pt_inputs).to_tuple()
fx_outputs = fx_model(**prepared_inputs_dict).to_tuple()
self.assertEqual(len(fx_outputs), len(pt_outputs), "Output lengths differ between Flax and PyTorch")
for fx_output, pt_output in zip(fx_outputs, pt_outputs):
self.assert_almost_equals(fx_output[:, -1], pt_output[:, -1].numpy(), 4e-2)
with tempfile.TemporaryDirectory() as tmpdirname:
fx_model.save_pretrained(tmpdirname)
pt_model_loaded = pt_model_class.from_pretrained(tmpdirname, from_flax=True)
with torch.no_grad():
pt_outputs_loaded = pt_model_loaded(**pt_inputs).to_tuple()
self.assertEqual(
len(fx_outputs), len(pt_outputs_loaded), "Output lengths differ between Flax and PyTorch"
)
for fx_output, pt_output in zip(fx_outputs, pt_outputs_loaded):
self.assert_almost_equals(fx_output[:, -1], pt_output[:, -1].numpy(), 4e-2)
@slow
def test_model_from_pretrained(self):
for model_class_name in self.all_model_classes:
model = model_class_name.from_pretrained("facebook/xglm-564M")
outputs = model(np.ones((1, 1)))
self.assertIsNotNone(outputs)
| transformers/tests/models/xglm/test_modeling_flax_xglm.py/0 | {
"file_path": "transformers/tests/models/xglm/test_modeling_flax_xglm.py",
"repo_id": "transformers",
"token_count": 7114
} | 369 |
# coding=utf-8
# Copyright 2023 The HuggingFace Inc. team. All rights reserved.
#
# Licensed under the Apache License, Version 2.0 (the "License");
# you may not use this file except in compliance with the License.
# You may obtain a copy of the License at
#
# http://www.apache.org/licenses/LICENSE-2.0
#
# Unless required by applicable law or agreed to in writing, software
# distributed under the License is distributed on an "AS IS" BASIS,
# WITHOUT WARRANTIES OR CONDITIONS OF ANY KIND, either express or implied.
# See the License for the specific language governing permissions and
# limitations under the License.
import os
import tempfile
import unittest
from huggingface_hub import hf_hub_download
from transformers import AutoModelForCausalLM, OPTForCausalLM
from transformers.testing_utils import require_peft, require_torch, require_torch_gpu, slow, torch_device
from transformers.utils import is_torch_available
if is_torch_available():
import torch
@require_peft
@require_torch
class PeftTesterMixin:
peft_test_model_ids = ("peft-internal-testing/tiny-OPTForCausalLM-lora",)
transformers_test_model_ids = ("hf-internal-testing/tiny-random-OPTForCausalLM",)
transformers_test_model_classes = (AutoModelForCausalLM, OPTForCausalLM)
# TODO: run it with CI after PEFT release.
@slow
class PeftIntegrationTester(unittest.TestCase, PeftTesterMixin):
"""
A testing suite that makes sure that the PeftModel class is correctly integrated into the transformers library.
"""
def _check_lora_correctly_converted(self, model):
"""
Utility method to check if the model has correctly adapters injected on it.
"""
from peft.tuners.tuners_utils import BaseTunerLayer
is_peft_loaded = False
for _, m in model.named_modules():
if isinstance(m, BaseTunerLayer):
is_peft_loaded = True
break
return is_peft_loaded
def test_peft_from_pretrained(self):
"""
Simple test that tests the basic usage of PEFT model through `from_pretrained`.
This checks if we pass a remote folder that contains an adapter config and adapter weights, it
should correctly load a model that has adapters injected on it.
"""
for model_id in self.peft_test_model_ids:
for transformers_class in self.transformers_test_model_classes:
peft_model = transformers_class.from_pretrained(model_id).to(torch_device)
self.assertTrue(self._check_lora_correctly_converted(peft_model))
self.assertTrue(peft_model._hf_peft_config_loaded)
# dummy generation
_ = peft_model.generate(input_ids=torch.LongTensor([[0, 1, 2, 3, 4, 5, 6, 7]]).to(torch_device))
def test_peft_state_dict(self):
"""
Simple test that checks if the returned state dict of `get_adapter_state_dict()` method contains
the expected keys.
"""
for model_id in self.peft_test_model_ids:
for transformers_class in self.transformers_test_model_classes:
peft_model = transformers_class.from_pretrained(model_id).to(torch_device)
state_dict = peft_model.get_adapter_state_dict()
for key in state_dict.keys():
self.assertTrue("lora" in key)
def test_peft_save_pretrained(self):
"""
Test that checks various combinations of `save_pretrained` with a model that has adapters loaded
on it. This checks if the saved model contains the expected files (adapter weights and adapter config).
"""
for model_id in self.peft_test_model_ids:
for transformers_class in self.transformers_test_model_classes:
peft_model = transformers_class.from_pretrained(model_id).to(torch_device)
with tempfile.TemporaryDirectory() as tmpdirname:
peft_model.save_pretrained(tmpdirname)
self.assertTrue("adapter_model.safetensors" in os.listdir(tmpdirname))
self.assertTrue("adapter_config.json" in os.listdir(tmpdirname))
self.assertTrue("config.json" not in os.listdir(tmpdirname))
self.assertTrue("pytorch_model.bin" not in os.listdir(tmpdirname))
self.assertTrue("model.safetensors" not in os.listdir(tmpdirname))
peft_model = transformers_class.from_pretrained(tmpdirname).to(torch_device)
self.assertTrue(self._check_lora_correctly_converted(peft_model))
peft_model.save_pretrained(tmpdirname, safe_serialization=False)
self.assertTrue("adapter_model.bin" in os.listdir(tmpdirname))
self.assertTrue("adapter_config.json" in os.listdir(tmpdirname))
peft_model = transformers_class.from_pretrained(tmpdirname).to(torch_device)
self.assertTrue(self._check_lora_correctly_converted(peft_model))
def test_peft_enable_disable_adapters(self):
"""
A test that checks if `enable_adapters` and `disable_adapters` methods work as expected.
"""
from peft import LoraConfig
dummy_input = torch.LongTensor([[0, 1, 2, 3, 4, 5, 6, 7]]).to(torch_device)
for model_id in self.transformers_test_model_ids:
for transformers_class in self.transformers_test_model_classes:
peft_model = transformers_class.from_pretrained(model_id).to(torch_device)
peft_config = LoraConfig(init_lora_weights=False)
peft_model.add_adapter(peft_config)
peft_logits = peft_model(dummy_input).logits
peft_model.disable_adapters()
peft_logits_disabled = peft_model(dummy_input).logits
peft_model.enable_adapters()
peft_logits_enabled = peft_model(dummy_input).logits
self.assertTrue(torch.allclose(peft_logits, peft_logits_enabled, atol=1e-12, rtol=1e-12))
self.assertFalse(torch.allclose(peft_logits_enabled, peft_logits_disabled, atol=1e-12, rtol=1e-12))
def test_peft_add_adapter(self):
"""
Simple test that tests if `add_adapter` works as expected
"""
from peft import LoraConfig
for model_id in self.transformers_test_model_ids:
for transformers_class in self.transformers_test_model_classes:
model = transformers_class.from_pretrained(model_id).to(torch_device)
peft_config = LoraConfig(init_lora_weights=False)
model.add_adapter(peft_config)
self.assertTrue(self._check_lora_correctly_converted(model))
# dummy generation
_ = model.generate(input_ids=torch.LongTensor([[0, 1, 2, 3, 4, 5, 6, 7]]).to(torch_device))
def test_peft_add_adapter_from_pretrained(self):
"""
Simple test that tests if `add_adapter` works as expected
"""
from peft import LoraConfig
for model_id in self.transformers_test_model_ids:
for transformers_class in self.transformers_test_model_classes:
model = transformers_class.from_pretrained(model_id).to(torch_device)
peft_config = LoraConfig(init_lora_weights=False)
model.add_adapter(peft_config)
self.assertTrue(self._check_lora_correctly_converted(model))
with tempfile.TemporaryDirectory() as tmpdirname:
model.save_pretrained(tmpdirname)
model_from_pretrained = transformers_class.from_pretrained(tmpdirname).to(torch_device)
self.assertTrue(self._check_lora_correctly_converted(model_from_pretrained))
def test_peft_add_adapter_modules_to_save(self):
"""
Simple test that tests if `add_adapter` works as expected when training with
modules to save.
"""
from peft import LoraConfig
from peft.utils import ModulesToSaveWrapper
for model_id in self.transformers_test_model_ids:
for transformers_class in self.transformers_test_model_classes:
dummy_input = torch.LongTensor([[0, 1, 2, 3, 4, 5, 6, 7]]).to(torch_device)
model = transformers_class.from_pretrained(model_id).to(torch_device)
peft_config = LoraConfig(init_lora_weights=False, modules_to_save=["lm_head"])
model.add_adapter(peft_config)
self._check_lora_correctly_converted(model)
_has_modules_to_save_wrapper = False
for name, module in model.named_modules():
if isinstance(module, ModulesToSaveWrapper):
_has_modules_to_save_wrapper = True
self.assertTrue(module.modules_to_save.default.weight.requires_grad)
self.assertTrue("lm_head" in name)
break
self.assertTrue(_has_modules_to_save_wrapper)
state_dict = model.get_adapter_state_dict()
self.assertTrue("lm_head.weight" in state_dict.keys())
logits = model(dummy_input).logits
loss = logits.mean()
loss.backward()
for _, param in model.named_parameters():
if param.requires_grad:
self.assertTrue(param.grad is not None)
def test_peft_add_adapter_training_gradient_checkpointing(self):
"""
Simple test that tests if `add_adapter` works as expected when training with
gradient checkpointing.
"""
from peft import LoraConfig
for model_id in self.transformers_test_model_ids:
for transformers_class in self.transformers_test_model_classes:
model = transformers_class.from_pretrained(model_id).to(torch_device)
peft_config = LoraConfig(init_lora_weights=False)
model.add_adapter(peft_config)
self.assertTrue(self._check_lora_correctly_converted(model))
# When attaching adapters the input embeddings will stay frozen, this will
# lead to the output embedding having requires_grad=False.
dummy_input = torch.LongTensor([[0, 1, 2, 3, 4, 5, 6, 7]]).to(torch_device)
frozen_output = model.get_input_embeddings()(dummy_input)
self.assertTrue(frozen_output.requires_grad is False)
model.gradient_checkpointing_enable()
# Since here we attached the hook, the input should have requires_grad to set
# properly
non_frozen_output = model.get_input_embeddings()(dummy_input)
self.assertTrue(non_frozen_output.requires_grad is True)
# To repro the Trainer issue
dummy_input.requires_grad = False
for name, param in model.named_parameters():
if "lora" in name.lower():
self.assertTrue(param.requires_grad)
logits = model(dummy_input).logits
loss = logits.mean()
loss.backward()
for name, param in model.named_parameters():
if param.requires_grad:
self.assertTrue("lora" in name.lower())
self.assertTrue(param.grad is not None)
def test_peft_add_multi_adapter(self):
"""
Simple test that tests the basic usage of PEFT model through `from_pretrained`. This test tests if
add_adapter works as expected in multi-adapter setting.
"""
from peft import LoraConfig
from peft.tuners.tuners_utils import BaseTunerLayer
dummy_input = torch.LongTensor([[0, 1, 2, 3, 4, 5, 6, 7]]).to(torch_device)
for model_id in self.transformers_test_model_ids:
for transformers_class in self.transformers_test_model_classes:
is_peft_loaded = False
model = transformers_class.from_pretrained(model_id).to(torch_device)
logits_original_model = model(dummy_input).logits
peft_config = LoraConfig(init_lora_weights=False)
model.add_adapter(peft_config)
logits_adapter_1 = model(dummy_input)
model.add_adapter(peft_config, adapter_name="adapter-2")
logits_adapter_2 = model(dummy_input)
for _, m in model.named_modules():
if isinstance(m, BaseTunerLayer):
is_peft_loaded = True
break
self.assertTrue(is_peft_loaded)
# dummy generation
_ = model.generate(input_ids=dummy_input)
model.set_adapter("default")
self.assertTrue(model.active_adapters() == ["default"])
self.assertTrue(model.active_adapter() == "default")
model.set_adapter("adapter-2")
self.assertTrue(model.active_adapters() == ["adapter-2"])
self.assertTrue(model.active_adapter() == "adapter-2")
# Logits comparison
self.assertFalse(
torch.allclose(logits_adapter_1.logits, logits_adapter_2.logits, atol=1e-6, rtol=1e-6)
)
self.assertFalse(torch.allclose(logits_original_model, logits_adapter_2.logits, atol=1e-6, rtol=1e-6))
model.set_adapter(["adapter-2", "default"])
self.assertTrue(model.active_adapters() == ["adapter-2", "default"])
self.assertTrue(model.active_adapter() == "adapter-2")
logits_adapter_mixed = model(dummy_input)
self.assertFalse(
torch.allclose(logits_adapter_1.logits, logits_adapter_mixed.logits, atol=1e-6, rtol=1e-6)
)
self.assertFalse(
torch.allclose(logits_adapter_2.logits, logits_adapter_mixed.logits, atol=1e-6, rtol=1e-6)
)
# multi active adapter saving not supported
with self.assertRaises(ValueError), tempfile.TemporaryDirectory() as tmpdirname:
model.save_pretrained(tmpdirname)
@require_torch_gpu
def test_peft_from_pretrained_kwargs(self):
"""
Simple test that tests the basic usage of PEFT model through `from_pretrained` + additional kwargs
and see if the integraiton behaves as expected.
"""
for model_id in self.peft_test_model_ids:
for transformers_class in self.transformers_test_model_classes:
peft_model = transformers_class.from_pretrained(model_id, load_in_8bit=True, device_map="auto")
module = peft_model.model.decoder.layers[0].self_attn.v_proj
self.assertTrue(module.__class__.__name__ == "Linear8bitLt")
self.assertTrue(peft_model.hf_device_map is not None)
# dummy generation
_ = peft_model.generate(input_ids=torch.LongTensor([[0, 1, 2, 3, 4, 5, 6, 7]]).to(torch_device))
@require_torch_gpu
def test_peft_save_quantized(self):
"""
Simple test that tests the basic usage of PEFT model save_pretrained with quantized base models
"""
# 4bit
for model_id in self.peft_test_model_ids:
for transformers_class in self.transformers_test_model_classes:
peft_model = transformers_class.from_pretrained(model_id, load_in_4bit=True, device_map="auto")
module = peft_model.model.decoder.layers[0].self_attn.v_proj
self.assertTrue(module.__class__.__name__ == "Linear4bit")
self.assertTrue(peft_model.hf_device_map is not None)
with tempfile.TemporaryDirectory() as tmpdirname:
peft_model.save_pretrained(tmpdirname)
self.assertTrue("adapter_model.safetensors" in os.listdir(tmpdirname))
self.assertTrue("adapter_config.json" in os.listdir(tmpdirname))
self.assertTrue("pytorch_model.bin" not in os.listdir(tmpdirname))
self.assertTrue("model.safetensors" not in os.listdir(tmpdirname))
# 8-bit
for model_id in self.peft_test_model_ids:
for transformers_class in self.transformers_test_model_classes:
peft_model = transformers_class.from_pretrained(model_id, load_in_8bit=True, device_map="auto")
module = peft_model.model.decoder.layers[0].self_attn.v_proj
self.assertTrue(module.__class__.__name__ == "Linear8bitLt")
self.assertTrue(peft_model.hf_device_map is not None)
with tempfile.TemporaryDirectory() as tmpdirname:
peft_model.save_pretrained(tmpdirname)
self.assertTrue("adapter_model.safetensors" in os.listdir(tmpdirname))
self.assertTrue("adapter_config.json" in os.listdir(tmpdirname))
self.assertTrue("pytorch_model.bin" not in os.listdir(tmpdirname))
self.assertTrue("model.safetensors" not in os.listdir(tmpdirname))
@require_torch_gpu
def test_peft_save_quantized_regression(self):
"""
Simple test that tests the basic usage of PEFT model save_pretrained with quantized base models
Regression test to make sure everything works as expected before the safetensors integration.
"""
# 4bit
for model_id in self.peft_test_model_ids:
for transformers_class in self.transformers_test_model_classes:
peft_model = transformers_class.from_pretrained(model_id, load_in_4bit=True, device_map="auto")
module = peft_model.model.decoder.layers[0].self_attn.v_proj
self.assertTrue(module.__class__.__name__ == "Linear4bit")
self.assertTrue(peft_model.hf_device_map is not None)
with tempfile.TemporaryDirectory() as tmpdirname:
peft_model.save_pretrained(tmpdirname, safe_serialization=False)
self.assertTrue("adapter_model.bin" in os.listdir(tmpdirname))
self.assertTrue("adapter_config.json" in os.listdir(tmpdirname))
self.assertTrue("pytorch_model.bin" not in os.listdir(tmpdirname))
self.assertTrue("model.safetensors" not in os.listdir(tmpdirname))
# 8-bit
for model_id in self.peft_test_model_ids:
for transformers_class in self.transformers_test_model_classes:
peft_model = transformers_class.from_pretrained(model_id, load_in_8bit=True, device_map="auto")
module = peft_model.model.decoder.layers[0].self_attn.v_proj
self.assertTrue(module.__class__.__name__ == "Linear8bitLt")
self.assertTrue(peft_model.hf_device_map is not None)
with tempfile.TemporaryDirectory() as tmpdirname:
peft_model.save_pretrained(tmpdirname, safe_serialization=False)
self.assertTrue("adapter_model.bin" in os.listdir(tmpdirname))
self.assertTrue("adapter_config.json" in os.listdir(tmpdirname))
self.assertTrue("pytorch_model.bin" not in os.listdir(tmpdirname))
self.assertTrue("model.safetensors" not in os.listdir(tmpdirname))
def test_peft_pipeline(self):
"""
Simple test that tests the basic usage of PEFT model + pipeline
"""
from transformers import pipeline
for model_id in self.peft_test_model_ids:
pipe = pipeline("text-generation", model_id)
_ = pipe("Hello")
def test_peft_add_adapter_with_state_dict(self):
"""
Simple test that tests the basic usage of PEFT model through `from_pretrained`. This test tests if
add_adapter works as expected with a state_dict being passed.
"""
from peft import LoraConfig
dummy_input = torch.LongTensor([[0, 1, 2, 3, 4, 5, 6, 7]]).to(torch_device)
for model_id, peft_model_id in zip(self.transformers_test_model_ids, self.peft_test_model_ids):
for transformers_class in self.transformers_test_model_classes:
model = transformers_class.from_pretrained(model_id).to(torch_device)
peft_config = LoraConfig(init_lora_weights=False)
with self.assertRaises(ValueError):
model.load_adapter(peft_model_id=None)
state_dict_path = hf_hub_download(peft_model_id, "adapter_model.bin")
dummy_state_dict = torch.load(state_dict_path)
model.load_adapter(adapter_state_dict=dummy_state_dict, peft_config=peft_config)
with self.assertRaises(ValueError):
model.load_adapter(model.load_adapter(adapter_state_dict=dummy_state_dict, peft_config=None))
self.assertTrue(self._check_lora_correctly_converted(model))
# dummy generation
_ = model.generate(input_ids=dummy_input)
def test_peft_from_pretrained_hub_kwargs(self):
"""
Tests different combinations of PEFT model + from_pretrained + hub kwargs
"""
peft_model_id = "peft-internal-testing/tiny-opt-lora-revision"
# This should not work
with self.assertRaises(OSError):
_ = AutoModelForCausalLM.from_pretrained(peft_model_id)
adapter_kwargs = {"revision": "test"}
# This should work
model = AutoModelForCausalLM.from_pretrained(peft_model_id, adapter_kwargs=adapter_kwargs)
self.assertTrue(self._check_lora_correctly_converted(model))
model = OPTForCausalLM.from_pretrained(peft_model_id, adapter_kwargs=adapter_kwargs)
self.assertTrue(self._check_lora_correctly_converted(model))
adapter_kwargs = {"revision": "main", "subfolder": "test_subfolder"}
model = AutoModelForCausalLM.from_pretrained(peft_model_id, adapter_kwargs=adapter_kwargs)
self.assertTrue(self._check_lora_correctly_converted(model))
model = OPTForCausalLM.from_pretrained(peft_model_id, adapter_kwargs=adapter_kwargs)
self.assertTrue(self._check_lora_correctly_converted(model))
| transformers/tests/peft_integration/test_peft_integration.py/0 | {
"file_path": "transformers/tests/peft_integration/test_peft_integration.py",
"repo_id": "transformers",
"token_count": 10392
} | 370 |
# coding=utf-8
# Copyright 2023 The HuggingFace Team. All rights reserved.
#
# Licensed under the Apache License, Version 2.0 (the "License");
# you may not use this file except in compliance with the License.
# You may obtain a copy of the License at
#
# http://www.apache.org/licenses/LICENSE-2.0
#
# Unless required by applicable law or agreed to in writing, software
# distributed under the License is distributed on an "AS IS" BASIS,
# WITHOUT WARRANTIES OR CONDITIONS OF ANY KIND, either express or implied.
# See the License for the specific language governing permissions and
# limitations under the License.
import gc
import tempfile
import unittest
from transformers import AutoConfig, AutoModelForCausalLM, AutoTokenizer, AwqConfig, OPTForCausalLM
from transformers.testing_utils import (
require_accelerate,
require_auto_awq,
require_torch_gpu,
require_torch_multi_gpu,
slow,
torch_device,
)
from transformers.utils import is_accelerate_available, is_torch_available
if is_torch_available():
import torch
if is_accelerate_available():
from accelerate import init_empty_weights
@require_torch_gpu
class AwqConfigTest(unittest.TestCase):
def test_wrong_backend(self):
"""
Simple test that checks if a user passes a wrong backend an error is raised
"""
# This should work fine
_ = AwqConfig(bits=4)
with self.assertRaises(ValueError):
AwqConfig(bits=4, backend="")
# These should work fine
_ = AwqConfig(bits=4, version="GEMM")
_ = AwqConfig(bits=4, version="gemm")
with self.assertRaises(ValueError):
AwqConfig(bits=4, backend="unexisting-backend")
compute_capability = torch.cuda.get_device_capability()
major, minor = compute_capability
if major < 8:
# LLMAWQ does not work on a T4
with self.assertRaises(ValueError):
AwqConfig(bits=4, backend="llm-awq")
else:
# LLMAWQ should work on an A100
AwqConfig(bits=4, backend="llm-awq")
def test_to_dict(self):
"""
Simple test that checks if one uses a config and converts it to a dict, the dict is the same as the config object
"""
quantization_config = AwqConfig(bits=4)
config_to_dict = quantization_config.to_dict()
for key in config_to_dict:
self.assertEqual(getattr(quantization_config, key), config_to_dict[key])
def test_from_dict(self):
"""
Simple test that checks if one uses a dict and converts it to a config object, the config object is the same as the dict
"""
dict = {"bits": 2, "zero_point": False, "backend": "autoawq"}
quantization_config = AwqConfig.from_dict(dict)
self.assertEqual(dict["bits"], quantization_config.bits)
self.assertEqual(dict["zero_point"], quantization_config.zero_point)
self.assertEqual(dict["backend"], quantization_config.backend)
@slow
@require_torch_gpu
@require_auto_awq
@require_accelerate
class AwqTest(unittest.TestCase):
model_name = "TheBloke/Mistral-7B-v0.1-AWQ"
dummy_transformers_model_name = "bigscience/bloom-560m"
model_with_no_k_proj_quantized = "hf-internal-testing/opt-125m-awq-no-k-proj"
input_text = "Hello my name is"
EXPECTED_OUTPUT = "Hello my name is Katie and I am a 20 year old student at the University of North Carolina at Chapel Hill. I am a junior and I am majoring in Journalism and minoring in Spanish"
EXPECTED_OUTPUT_BF16 = "Hello my name is Katie and I am a 20 year old student at the University of North Carolina at Chapel Hill. I am a junior and I am majoring in Exercise and Sport Science with a"
device_map = "cuda"
# called only once for all test in this class
@classmethod
def setUpClass(cls):
"""
Setup quantized model
"""
cls.tokenizer = AutoTokenizer.from_pretrained(cls.model_name)
cls.quantized_model = AutoModelForCausalLM.from_pretrained(
cls.model_name,
device_map=cls.device_map,
)
def tearDown(self):
gc.collect()
torch.cuda.empty_cache()
gc.collect()
def test_quantized_model_conversion(self):
"""
Simple test that checks if the quantized model has been converted properly
"""
from awq.modules.linear import WQLinear_GEMM, WQLinear_GEMV
from transformers.integrations.awq import replace_with_awq_linear
model_id = "facebook/opt-350m"
config = AutoConfig.from_pretrained(model_id, revision="cb32f77e905cccbca1d970436fb0f5e6b58ee3c5")
quantization_config = AwqConfig(bits=4)
with init_empty_weights():
model = OPTForCausalLM(config)
nb_linears = 0
for module in model.modules():
if isinstance(module, torch.nn.Linear):
nb_linears += 1
model, _ = replace_with_awq_linear(model, quantization_config=quantization_config)
nb_awq_linear = 0
for module in model.modules():
if isinstance(module, (WQLinear_GEMM, WQLinear_GEMV)):
nb_awq_linear += 1
self.assertEqual(nb_linears, nb_awq_linear)
# Try with `modules_not_to_convert`
with init_empty_weights():
model = OPTForCausalLM(config)
model, _ = replace_with_awq_linear(
model, quantization_config=quantization_config, modules_to_not_convert=["lm_head"]
)
nb_awq_linear = 0
for module in model.modules():
if isinstance(module, (WQLinear_GEMM, WQLinear_GEMV)):
nb_awq_linear += 1
self.assertEqual(nb_linears - 1, nb_awq_linear)
def test_quantized_model(self):
"""
Simple test that checks if the quantized model is working properly
"""
input_ids = self.tokenizer(self.input_text, return_tensors="pt").to(torch_device)
output = self.quantized_model.generate(**input_ids, max_new_tokens=40)
self.assertEqual(self.tokenizer.decode(output[0], skip_special_tokens=True), self.EXPECTED_OUTPUT)
def test_raise_if_non_quantized(self):
model_id = "facebook/opt-125m"
quantization_config = AwqConfig(bits=4)
with self.assertRaises(ValueError):
_ = AutoModelForCausalLM.from_pretrained(model_id, quantization_config=quantization_config)
def test_quantized_model_bf16(self):
"""
Simple test that checks if the quantized model is working properly with bf16
"""
input_ids = self.tokenizer(self.input_text, return_tensors="pt").to(torch_device)
quantized_model = AutoModelForCausalLM.from_pretrained(self.model_name, torch_dtype=torch.bfloat16).to(
torch_device
)
output = quantized_model.generate(**input_ids, max_new_tokens=40)
self.assertEqual(self.tokenizer.decode(output[0], skip_special_tokens=True), self.EXPECTED_OUTPUT_BF16)
def test_quantized_model_no_device_map(self):
"""
Simple test that checks if the quantized model is working properly
"""
input_ids = self.tokenizer(self.input_text, return_tensors="pt").to(torch_device)
quantized_model = AutoModelForCausalLM.from_pretrained(self.model_name).to(torch_device)
output = quantized_model.generate(**input_ids, max_new_tokens=40)
self.assertEqual(self.tokenizer.decode(output[0], skip_special_tokens=True), self.EXPECTED_OUTPUT)
def test_save_pretrained(self):
"""
Simple test that checks if the quantized model is working properly after being saved and loaded
"""
with tempfile.TemporaryDirectory() as tmpdirname:
self.quantized_model.save_pretrained(tmpdirname)
model = AutoModelForCausalLM.from_pretrained(tmpdirname, device_map=self.device_map)
input_ids = self.tokenizer(self.input_text, return_tensors="pt").to(torch_device)
output = model.generate(**input_ids, max_new_tokens=40)
self.assertEqual(self.tokenizer.decode(output[0], skip_special_tokens=True), self.EXPECTED_OUTPUT)
@require_torch_multi_gpu
def test_quantized_model_multi_gpu(self):
"""
Simple test that checks if the quantized model is working properly with multiple GPUs
"""
input_ids = self.tokenizer(self.input_text, return_tensors="pt").to(torch_device)
quantized_model = AutoModelForCausalLM.from_pretrained(self.model_name, device_map="auto")
self.assertTrue(set(quantized_model.hf_device_map.values()) == {0, 1, 2, 3})
output = quantized_model.generate(**input_ids, max_new_tokens=40)
self.assertEqual(self.tokenizer.decode(output[0], skip_special_tokens=True), self.EXPECTED_OUTPUT)
def test_quantized_model_no_k_proj_quantized(self):
"""
Simple test that checks if the quantized model is working properly with multiple GPUs
"""
dummy_input = torch.LongTensor([[0, 1, 0]]).to(torch_device)
quantized_model = AutoModelForCausalLM.from_pretrained(self.model_with_no_k_proj_quantized).to(torch_device)
self.assertTrue(isinstance(quantized_model.model.decoder.layers[0].self_attn.k_proj, torch.nn.Linear))
self.assertFalse(isinstance(quantized_model.model.decoder.layers[0].self_attn.v_proj, torch.nn.Linear))
EXPECTED_OUTPUT = torch.LongTensor([[0, 1, 0, 50118, 50118, 133, 248, 12, 134, 16, 10, 372, 2031]]).to(
torch_device
)
output = quantized_model.generate(dummy_input, max_new_tokens=10)
self.assertTrue((EXPECTED_OUTPUT == output).all())
@slow
@require_torch_gpu
@require_auto_awq
@require_accelerate
class AwqFusedTest(unittest.TestCase):
model_name = "TheBloke/Mistral-7B-OpenOrca-AWQ"
model_revision = "7048b2af77d0dd1c81b000b19d73f9cc8950b510"
custom_mapping_model_id = "TheBloke/Yi-34B-AWQ"
custom_model_revision = "f1b2cd1b7459ceecfdc1fac5bb8725f13707c589"
mixtral_model_name = "casperhansen/mixtral-instruct-awq"
mixtral_model_revision = "87dd4ec502dde74fb3a624835c776b000d190c3b"
multi_modal_model_name = "ybelkada/llava-1.5-7b-hf-awq"
multi_modal_model_code_revision = "ad108a50f5b9e681bdd7378409f57b7fa59a7442"
prompt = (
"You're standing on the surface of the Earth. "
"You walk one mile south, one mile west and one mile north. "
"You end up exactly where you started. Where are you?"
)
EXPECTED_GENERATION = prompt + "\n\nThis is a classic puzzle that has been around for"
EXPECTED_GENERATION_CUSTOM_MODEL = "HelloWorld.java:11)\r\n\tat org"
EXPECTED_GENERATION_MIXTRAL = prompt + " You're on the North Pole.\n\nThe"
def tearDown(self):
gc.collect()
torch.cuda.empty_cache()
gc.collect()
def _check_fused_modules(self, model):
has_fused_modules = False
fused_modules_name = ["QuantAttentionFused", "QuantFusedMLP", "FasterTransformerRMSNorm"]
for _, module in model.named_modules():
if module.__class__.__name__ in fused_modules_name:
has_fused_modules = True
break
self.assertTrue(has_fused_modules, "Modules fusing not performed correctly!")
def test_raise_save_pretrained(self):
"""
Test that `save_pretrained` is effectively blocked for fused models
"""
quantization_config = AwqConfig(bits=4, fuse_max_seq_len=128, do_fuse=True)
model = AutoModelForCausalLM.from_pretrained(
self.model_name,
quantization_config=quantization_config,
low_cpu_mem_usage=True,
revision=self.model_revision,
).to(torch_device)
self._check_fused_modules(model)
with self.assertRaises(ValueError), tempfile.TemporaryDirectory() as tmpdirname:
model.save_pretrained(tmpdirname)
def test_fused_modules_to_not_convert(self):
"""
Test if fused + modules to_not_covnert work as expected
"""
model_id = "hf-internal-testing/Mixtral-tiny-AWQ"
quantization_config = AwqConfig(bits=4, fuse_max_seq_len=128, do_fuse=True)
model = AutoModelForCausalLM.from_pretrained(
model_id,
quantization_config=quantization_config,
low_cpu_mem_usage=True,
).to(torch_device)
# Check if model has been correctly fused
self._check_fused_modules(model)
# Checks if the modules_to_not_convert (here gate layer) is a Linear
self.assertTrue(isinstance(model.model.layers[0].block_sparse_moe.gate, torch.nn.Linear))
def test_generation_fused(self):
"""
Test generation quality for fused models - single batch case
"""
quantization_config = AwqConfig(bits=4, fuse_max_seq_len=128, do_fuse=True)
model = AutoModelForCausalLM.from_pretrained(
self.model_name,
quantization_config=quantization_config,
low_cpu_mem_usage=True,
revision=self.model_revision,
).to(torch_device)
self._check_fused_modules(model)
tokenizer = AutoTokenizer.from_pretrained(self.model_name, revision=self.model_revision)
inputs = tokenizer(self.prompt, return_tensors="pt").to(torch_device)
outputs = model.generate(**inputs, max_new_tokens=12)
self.assertEqual(tokenizer.decode(outputs[0], skip_special_tokens=True), self.EXPECTED_GENERATION)
def test_generation_fused_batched(self):
"""
Test generation quality for fused models - multi batch case
"""
quantization_config = AwqConfig(bits=4, fuse_max_seq_len=128, do_fuse=True)
model = AutoModelForCausalLM.from_pretrained(
self.model_name,
quantization_config=quantization_config,
low_cpu_mem_usage=True,
revision=self.model_revision,
).to(torch_device)
self._check_fused_modules(model)
tokenizer = AutoTokenizer.from_pretrained(self.model_name, revision=self.model_revision)
tokenizer.pad_token_id = tokenizer.eos_token_id
inputs = tokenizer([self.prompt, self.prompt], return_tensors="pt", padding=True).to(torch_device)
outputs = model.generate(**inputs, max_new_tokens=12)
self.assertEqual(tokenizer.decode(outputs[0], skip_special_tokens=True), self.EXPECTED_GENERATION)
def test_generation_llava_fused(self):
from transformers import pipeline
quantization_config = AwqConfig(do_fuse=True, fuse_max_seq_len=2048)
pipe = pipeline(
"image-to-text",
model=self.multi_modal_model_name,
device=0,
model_kwargs={
"quantization_config": quantization_config,
},
revision=self.multi_modal_model_code_revision,
)
url = "https://huggingface.co/datasets/huggingface/documentation-images/resolve/main/diffusers/compel-neg.png"
prompt = "USER: <image>\nCan you please describe this image?\nASSISTANT:"
outputs = pipe(url, prompt=prompt, generate_kwargs={"max_new_tokens": 100})
EXPECTED_OUTPUT = "USER: \nCan you please describe this image?\nASSISTANT: The image features a brown and white cat sitting on a green surface, possibly a carpet or a grassy area. The cat is holding a red ball in its paws, seemingly playing with it. The cat appears to be focused on the ball, possibly preparing to play or just enjoying the toy."
self.assertEqual(outputs[0]["generated_text"], EXPECTED_OUTPUT)
@require_torch_multi_gpu
def test_generation_custom_model(self):
"""
Test generation quality for fused models using custom fused map.
"""
quantization_config = AwqConfig(
bits=4,
fuse_max_seq_len=512,
modules_to_fuse={
"attention": ["q_proj", "k_proj", "v_proj", "o_proj"],
"layernorm": ["ln1", "ln2", "norm"],
"mlp": ["gate_proj", "up_proj", "down_proj"],
"use_alibi": False,
"num_attention_heads": 56,
"num_key_value_heads": 8,
"hidden_size": 7168,
},
)
model = AutoModelForCausalLM.from_pretrained(
self.custom_mapping_model_id,
quantization_config=quantization_config,
trust_remote_code=True,
device_map="balanced",
revision=self.custom_model_revision,
)
self._check_fused_modules(model)
tokenizer = AutoTokenizer.from_pretrained(
self.custom_mapping_model_id, revision=self.custom_model_revision, trust_remote_code=True
)
prompt = "Hello"
inputs = tokenizer(prompt, return_tensors="pt").to(torch_device)
outputs = model.generate(**inputs, max_new_tokens=12)
self.assertEqual(tokenizer.decode(outputs[0], skip_special_tokens=True), self.EXPECTED_GENERATION_CUSTOM_MODEL)
@require_torch_multi_gpu
def test_generation_mixtral_fused(self):
"""
Text generation test for Mixtral + AWQ + fused
"""
quantization_config = AwqConfig(bits=4, fuse_max_seq_len=1024, do_fuse=True)
model = AutoModelForCausalLM.from_pretrained(
self.mixtral_model_name,
quantization_config=quantization_config,
device_map="auto",
revision=self.mixtral_model_revision,
)
tokenizer = AutoTokenizer.from_pretrained(self.mixtral_model_name)
tokenizer.pad_token = tokenizer.eos_token
inputs = tokenizer([self.prompt, self.prompt], return_tensors="pt", padding=True).to(torch_device)
outputs = model.generate(**inputs, max_new_tokens=12)
self.assertEqual(tokenizer.decode(outputs[0], skip_special_tokens=True), self.EXPECTED_GENERATION_MIXTRAL)
| transformers/tests/quantization/autoawq/test_awq.py/0 | {
"file_path": "transformers/tests/quantization/autoawq/test_awq.py",
"repo_id": "transformers",
"token_count": 7755
} | 371 |
import json
import logging
import os
import subprocess
from argparse import ArgumentParser
logger = logging.getLogger(__name__)
def parse_args():
parser = ArgumentParser()
parsed, unknown = parser.parse_known_args()
for arg in unknown:
if arg.startswith(("-", "--")):
parser.add_argument(arg.split("=")[0])
return parser.parse_args()
def main():
args = parse_args()
port = 8888
num_gpus = int(os.environ["SM_NUM_GPUS"])
hosts = json.loads(os.environ["SM_HOSTS"])
num_nodes = len(hosts)
current_host = os.environ["SM_CURRENT_HOST"]
rank = hosts.index(current_host)
os.environ["NCCL_DEBUG"] = "INFO"
if num_nodes > 1:
cmd = f"""python -m torch.distributed.launch \
--nnodes={num_nodes} \
--node_rank={rank} \
--nproc_per_node={num_gpus} \
--master_addr={hosts[0]} \
--master_port={port} \
./run_glue.py \
{"".join([f" --{parameter} {value}" for parameter,value in args.__dict__.items()])}"""
else:
cmd = f"""python -m torch.distributed.launch \
--nproc_per_node={num_gpus} \
./run_glue.py \
{"".join([f" --{parameter} {value}" for parameter,value in args.__dict__.items()])}"""
try:
subprocess.run(cmd, shell=True)
except Exception as e:
logger.info(e)
if __name__ == "__main__":
main()
| transformers/tests/sagemaker/scripts/pytorch/run_ddp.py/0 | {
"file_path": "transformers/tests/sagemaker/scripts/pytorch/run_ddp.py",
"repo_id": "transformers",
"token_count": 694
} | 372 |
# coding=utf-8
# Copyright 2022 HuggingFace Inc.
#
# Licensed under the Apache License, Version 2.0 (the "License");
# you may not use this file except in compliance with the License.
# You may obtain a copy of the License at
#
# http://www.apache.org/licenses/LICENSE-2.0
#
# Unless required by applicable law or agreed to in writing, software
# distributed under the License is distributed on an "AS IS" BASIS,
# WITHOUT WARRANTIES OR CONDITIONS OF ANY KIND, either express or implied.
# See the License for the specific language governing permissions and
# limitations under the License.
import unittest
import numpy as np
from parameterized import parameterized
from transformers.testing_utils import require_flax, require_tf, require_torch, require_vision
from transformers.utils.import_utils import is_flax_available, is_tf_available, is_torch_available, is_vision_available
if is_torch_available():
import torch
if is_tf_available():
import tensorflow as tf
if is_flax_available():
import jax
if is_vision_available():
import PIL.Image
from transformers.image_transforms import (
center_crop,
center_to_corners_format,
convert_to_rgb,
corners_to_center_format,
flip_channel_order,
get_resize_output_image_size,
id_to_rgb,
normalize,
pad,
resize,
rgb_to_id,
to_channel_dimension_format,
to_pil_image,
)
def get_random_image(height, width, num_channels=3, channels_first=True):
shape = (num_channels, height, width) if channels_first else (height, width, num_channels)
random_array = np.random.randint(0, 256, shape, dtype=np.uint8)
return random_array
@require_vision
class ImageTransformsTester(unittest.TestCase):
@parameterized.expand(
[
("numpy_float_channels_first", (3, 4, 5), np.float32),
("numpy_float_channels_last", (4, 5, 3), np.float32),
("numpy_float_channels_first", (3, 4, 5), np.float64),
("numpy_float_channels_last", (4, 5, 3), np.float64),
("numpy_int_channels_first", (3, 4, 5), np.int32),
("numpy_uint_channels_first", (3, 4, 5), np.uint8),
]
)
@require_vision
def test_to_pil_image(self, name, image_shape, dtype):
image = np.random.randint(0, 256, image_shape).astype(dtype)
pil_image = to_pil_image(image)
self.assertIsInstance(pil_image, PIL.Image.Image)
self.assertEqual(pil_image.size, (5, 4))
# make sure image is correctly rescaled
self.assertTrue(np.abs(np.asarray(pil_image)).sum() > 0)
@parameterized.expand(
[
("numpy_float_channels_first", (3, 4, 5), np.float32),
("numpy_float_channels_first", (3, 4, 5), np.float64),
("numpy_float_channels_last", (4, 5, 3), np.float32),
("numpy_float_channels_last", (4, 5, 3), np.float64),
]
)
@require_vision
def test_to_pil_image_from_float(self, name, image_shape, dtype):
image = np.random.rand(*image_shape).astype(dtype)
pil_image = to_pil_image(image)
self.assertIsInstance(pil_image, PIL.Image.Image)
self.assertEqual(pil_image.size, (5, 4))
# make sure image is correctly rescaled
self.assertTrue(np.abs(np.asarray(pil_image)).sum() > 0)
# Make sure that an exception is raised if image is not in [0, 1]
image = np.random.randn(*image_shape).astype(dtype)
with self.assertRaises(ValueError):
to_pil_image(image)
@require_vision
def test_to_pil_image_from_mask(self):
# Make sure binary mask remains a binary mask
image = np.random.randint(0, 2, (3, 4, 5)).astype(np.uint8)
pil_image = to_pil_image(image)
self.assertIsInstance(pil_image, PIL.Image.Image)
self.assertEqual(pil_image.size, (5, 4))
np_img = np.asarray(pil_image)
self.assertTrue(np_img.min() == 0)
self.assertTrue(np_img.max() == 1)
image = np.random.randint(0, 2, (3, 4, 5)).astype(np.float32)
pil_image = to_pil_image(image)
self.assertIsInstance(pil_image, PIL.Image.Image)
self.assertEqual(pil_image.size, (5, 4))
np_img = np.asarray(pil_image)
self.assertTrue(np_img.min() == 0)
self.assertTrue(np_img.max() == 1)
@require_tf
def test_to_pil_image_from_tensorflow(self):
# channels_first
image = tf.random.uniform((3, 4, 5))
pil_image = to_pil_image(image)
self.assertIsInstance(pil_image, PIL.Image.Image)
self.assertEqual(pil_image.size, (5, 4))
# channels_last
image = tf.random.uniform((4, 5, 3))
pil_image = to_pil_image(image)
self.assertIsInstance(pil_image, PIL.Image.Image)
self.assertEqual(pil_image.size, (5, 4))
@require_torch
def test_to_pil_image_from_torch(self):
# channels first
image = torch.rand((3, 4, 5))
pil_image = to_pil_image(image)
self.assertIsInstance(pil_image, PIL.Image.Image)
self.assertEqual(pil_image.size, (5, 4))
# channels last
image = torch.rand((4, 5, 3))
pil_image = to_pil_image(image)
self.assertIsInstance(pil_image, PIL.Image.Image)
self.assertEqual(pil_image.size, (5, 4))
@require_flax
def test_to_pil_image_from_jax(self):
key = jax.random.PRNGKey(0)
# channel first
image = jax.random.uniform(key, (3, 4, 5))
pil_image = to_pil_image(image)
self.assertIsInstance(pil_image, PIL.Image.Image)
self.assertEqual(pil_image.size, (5, 4))
# channel last
image = jax.random.uniform(key, (4, 5, 3))
pil_image = to_pil_image(image)
self.assertIsInstance(pil_image, PIL.Image.Image)
self.assertEqual(pil_image.size, (5, 4))
def test_to_channel_dimension_format(self):
# Test that function doesn't reorder if channel dim matches the input.
image = np.random.rand(3, 4, 5)
image = to_channel_dimension_format(image, "channels_first")
self.assertEqual(image.shape, (3, 4, 5))
image = np.random.rand(4, 5, 3)
image = to_channel_dimension_format(image, "channels_last")
self.assertEqual(image.shape, (4, 5, 3))
# Test that function reorders if channel dim doesn't match the input.
image = np.random.rand(3, 4, 5)
image = to_channel_dimension_format(image, "channels_last")
self.assertEqual(image.shape, (4, 5, 3))
image = np.random.rand(4, 5, 3)
image = to_channel_dimension_format(image, "channels_first")
self.assertEqual(image.shape, (3, 4, 5))
# Can pass in input_data_format and works if data format is ambiguous or unknown.
image = np.random.rand(4, 5, 6)
image = to_channel_dimension_format(image, "channels_first", input_channel_dim="channels_last")
self.assertEqual(image.shape, (6, 4, 5))
def test_get_resize_output_image_size(self):
image = np.random.randint(0, 256, (3, 224, 224))
# Test the output size defaults to (x, x) if an int is given.
self.assertEqual(get_resize_output_image_size(image, 10), (10, 10))
self.assertEqual(get_resize_output_image_size(image, [10]), (10, 10))
self.assertEqual(get_resize_output_image_size(image, (10,)), (10, 10))
# Test the output size is the same as the input if a two element tuple/list is given.
self.assertEqual(get_resize_output_image_size(image, (10, 20)), (10, 20))
self.assertEqual(get_resize_output_image_size(image, [10, 20]), (10, 20))
self.assertEqual(get_resize_output_image_size(image, (10, 20), default_to_square=True), (10, 20))
# To match pytorch behaviour, max_size is only relevant if size is an int
self.assertEqual(get_resize_output_image_size(image, (10, 20), max_size=5), (10, 20))
# Test output size = (int(size * height / width), size) if size is an int and height > width
image = np.random.randint(0, 256, (3, 50, 40))
self.assertEqual(get_resize_output_image_size(image, 20, default_to_square=False), (25, 20))
# Test output size = (size, int(size * width / height)) if size is an int and width <= height
image = np.random.randint(0, 256, (3, 40, 50))
self.assertEqual(get_resize_output_image_size(image, 20, default_to_square=False), (20, 25))
# Test size is resized if longer size > max_size
image = np.random.randint(0, 256, (3, 50, 40))
self.assertEqual(get_resize_output_image_size(image, 20, default_to_square=False, max_size=22), (22, 17))
# Test output size = (int(size * height / width), size) if size is an int and height > width and
# input has 4 channels
image = np.random.randint(0, 256, (4, 50, 40))
self.assertEqual(
get_resize_output_image_size(image, 20, default_to_square=False, input_data_format="channels_first"),
(25, 20),
)
# Test correct channel dimension is returned if output size if height == 3
# Defaults to input format - channels first
image = np.random.randint(0, 256, (3, 18, 97))
resized_image = resize(image, (3, 20))
self.assertEqual(resized_image.shape, (3, 3, 20))
# Defaults to input format - channels last
image = np.random.randint(0, 256, (18, 97, 3))
resized_image = resize(image, (3, 20))
self.assertEqual(resized_image.shape, (3, 20, 3))
image = np.random.randint(0, 256, (3, 18, 97))
resized_image = resize(image, (3, 20), data_format="channels_last")
self.assertEqual(resized_image.shape, (3, 20, 3))
image = np.random.randint(0, 256, (18, 97, 3))
resized_image = resize(image, (3, 20), data_format="channels_first")
self.assertEqual(resized_image.shape, (3, 3, 20))
def test_resize(self):
image = np.random.randint(0, 256, (3, 224, 224))
# Check the channel order is the same by default
resized_image = resize(image, (30, 40))
self.assertIsInstance(resized_image, np.ndarray)
self.assertEqual(resized_image.shape, (3, 30, 40))
# Check channel order is changed if specified
resized_image = resize(image, (30, 40), data_format="channels_last")
self.assertIsInstance(resized_image, np.ndarray)
self.assertEqual(resized_image.shape, (30, 40, 3))
# Check PIL.Image.Image is returned if return_numpy=False
resized_image = resize(image, (30, 40), return_numpy=False)
self.assertIsInstance(resized_image, PIL.Image.Image)
# PIL size is in (width, height) order
self.assertEqual(resized_image.size, (40, 30))
# Check an image with float values between 0-1 is returned with values in this range
image = np.random.rand(3, 224, 224)
resized_image = resize(image, (30, 40))
self.assertIsInstance(resized_image, np.ndarray)
self.assertEqual(resized_image.shape, (3, 30, 40))
self.assertTrue(np.all(resized_image >= 0))
self.assertTrue(np.all(resized_image <= 1))
# Check that an image with 4 channels is resized correctly
image = np.random.randint(0, 256, (4, 224, 224))
resized_image = resize(image, (30, 40), input_data_format="channels_first")
self.assertIsInstance(resized_image, np.ndarray)
self.assertEqual(resized_image.shape, (4, 30, 40))
def test_normalize(self):
image = np.random.randint(0, 256, (224, 224, 3)) / 255
# Test that exception is raised if inputs are incorrect
# Not a numpy array image
with self.assertRaises(ValueError):
normalize(5, 5, 5)
# Number of mean values != number of channels
with self.assertRaises(ValueError):
normalize(image, mean=(0.5, 0.6), std=1)
# Number of std values != number of channels
with self.assertRaises(ValueError):
normalize(image, mean=1, std=(0.5, 0.6))
# Test result is correct - output data format is channels_first and normalization
# correctly computed
mean = (0.5, 0.6, 0.7)
std = (0.1, 0.2, 0.3)
expected_image = ((image - mean) / std).transpose((2, 0, 1))
normalized_image = normalize(image, mean=mean, std=std, data_format="channels_first")
self.assertIsInstance(normalized_image, np.ndarray)
self.assertEqual(normalized_image.shape, (3, 224, 224))
self.assertTrue(np.allclose(normalized_image, expected_image, atol=1e-6))
# Test image with 4 channels is normalized correctly
image = np.random.randint(0, 256, (224, 224, 4)) / 255
mean = (0.5, 0.6, 0.7, 0.8)
std = (0.1, 0.2, 0.3, 0.4)
expected_image = (image - mean) / std
self.assertTrue(
np.allclose(
normalize(image, mean=mean, std=std, input_data_format="channels_last"), expected_image, atol=1e-6
)
)
# Test float32 image input keeps float32 dtype
image = np.random.randint(0, 256, (224, 224, 3)).astype(np.float32) / 255
mean = (0.5, 0.6, 0.7)
std = (0.1, 0.2, 0.3)
expected_image = ((image - mean) / std).astype(np.float32)
normalized_image = normalize(image, mean=mean, std=std)
self.assertEqual(normalized_image.dtype, np.float32)
self.assertTrue(np.allclose(normalized_image, expected_image, atol=1e-6))
# Test float16 image input keeps float16 dtype
image = np.random.randint(0, 256, (224, 224, 3)).astype(np.float16) / 255
mean = (0.5, 0.6, 0.7)
std = (0.1, 0.2, 0.3)
# The mean and std are cast to match the dtype of the input image
cast_mean = np.array(mean, dtype=np.float16)
cast_std = np.array(std, dtype=np.float16)
expected_image = (image - cast_mean) / cast_std
normalized_image = normalize(image, mean=mean, std=std)
self.assertEqual(normalized_image.dtype, np.float16)
self.assertTrue(np.allclose(normalized_image, expected_image, atol=1e-6))
# Test int image input is converted to float32
image = np.random.randint(0, 2, (224, 224, 3), dtype=np.uint8)
mean = (0.5, 0.6, 0.7)
std = (0.1, 0.2, 0.3)
expected_image = (image.astype(np.float32) - mean) / std
normalized_image = normalize(image, mean=mean, std=std)
self.assertEqual(normalized_image.dtype, np.float32)
self.assertTrue(np.allclose(normalized_image, expected_image, atol=1e-6))
def test_center_crop(self):
image = np.random.randint(0, 256, (3, 224, 224))
# Test that exception is raised if inputs are incorrect
with self.assertRaises(ValueError):
center_crop(image, 10)
# Test result is correct - output data format is channels_first and center crop
# correctly computed
expected_image = image[:, 52:172, 82:142].transpose(1, 2, 0)
cropped_image = center_crop(image, (120, 60), data_format="channels_last")
self.assertIsInstance(cropped_image, np.ndarray)
self.assertEqual(cropped_image.shape, (120, 60, 3))
self.assertTrue(np.allclose(cropped_image, expected_image))
# Test that image is padded with zeros if crop size is larger than image size
expected_image = np.zeros((300, 260, 3))
expected_image[38:262, 18:242, :] = image.transpose((1, 2, 0))
cropped_image = center_crop(image, (300, 260), data_format="channels_last")
self.assertIsInstance(cropped_image, np.ndarray)
self.assertEqual(cropped_image.shape, (300, 260, 3))
self.assertTrue(np.allclose(cropped_image, expected_image))
# Test image with 4 channels is cropped correctly
image = np.random.randint(0, 256, (224, 224, 4))
expected_image = image[52:172, 82:142, :]
self.assertTrue(np.allclose(center_crop(image, (120, 60), input_data_format="channels_last"), expected_image))
def test_center_to_corners_format(self):
bbox_center = np.array([[10, 20, 4, 8], [15, 16, 3, 4]])
expected = np.array([[8, 16, 12, 24], [13.5, 14, 16.5, 18]])
self.assertTrue(np.allclose(center_to_corners_format(bbox_center), expected))
# Check that the function and inverse function are inverse of each other
self.assertTrue(np.allclose(corners_to_center_format(center_to_corners_format(bbox_center)), bbox_center))
def test_corners_to_center_format(self):
bbox_corners = np.array([[8, 16, 12, 24], [13.5, 14, 16.5, 18]])
expected = np.array([[10, 20, 4, 8], [15, 16, 3, 4]])
self.assertTrue(np.allclose(corners_to_center_format(bbox_corners), expected))
# Check that the function and inverse function are inverse of each other
self.assertTrue(np.allclose(center_to_corners_format(corners_to_center_format(bbox_corners)), bbox_corners))
def test_rgb_to_id(self):
# test list input
rgb = [125, 4, 255]
self.assertEqual(rgb_to_id(rgb), 16712829)
# test numpy array input
color = np.array(
[
[
[213, 54, 165],
[88, 207, 39],
[156, 108, 128],
],
[
[183, 194, 46],
[137, 58, 88],
[114, 131, 233],
],
]
)
expected = np.array([[10827477, 2608984, 8416412], [3064503, 5782153, 15303538]])
self.assertTrue(np.allclose(rgb_to_id(color), expected))
def test_id_to_rgb(self):
# test int input
self.assertEqual(id_to_rgb(16712829), [125, 4, 255])
# test array input
id_array = np.array([[10827477, 2608984, 8416412], [3064503, 5782153, 15303538]])
color = np.array(
[
[
[213, 54, 165],
[88, 207, 39],
[156, 108, 128],
],
[
[183, 194, 46],
[137, 58, 88],
[114, 131, 233],
],
]
)
self.assertTrue(np.allclose(id_to_rgb(id_array), color))
def test_pad(self):
# fmt: off
image = np.array([[
[0, 1],
[2, 3],
]])
# fmt: on
# Test that exception is raised if unknown padding mode is specified
with self.assertRaises(ValueError):
pad(image, 10, mode="unknown")
# Test that exception is raised if invalid padding is specified
with self.assertRaises(ValueError):
# Cannot pad on channel dimension
pad(image, (5, 10, 10))
# Test image is padded equally on all sides is padding is an int
# fmt: off
expected_image = np.array([
[[0, 0, 0, 0],
[0, 0, 1, 0],
[0, 2, 3, 0],
[0, 0, 0, 0]],
])
# fmt: on
self.assertTrue(np.allclose(expected_image, pad(image, 1)))
# Test the left and right of each axis is padded (pad_left, pad_right)
# fmt: off
expected_image = np.array(
[[0, 0, 0, 0, 0],
[0, 0, 0, 0, 0],
[0, 0, 0, 1, 0],
[0, 0, 2, 3, 0],
[0, 0, 0, 0, 0]])
# fmt: on
self.assertTrue(np.allclose(expected_image, pad(image, (2, 1))))
# Test only one axis is padded (pad_left, pad_right)
# fmt: off
expected_image = np.array([[
[9, 9],
[9, 9],
[0, 1],
[2, 3],
[9, 9]
]])
# fmt: on
self.assertTrue(np.allclose(expected_image, pad(image, ((2, 1), (0, 0)), constant_values=9)))
# Test padding with a constant value
# fmt: off
expected_image = np.array([[
[8, 8, 0, 1, 9],
[8, 8, 2, 3, 9],
[8, 8, 7, 7, 9],
[8, 8, 7, 7, 9]
]])
# fmt: on
self.assertTrue(np.allclose(expected_image, pad(image, ((0, 2), (2, 1)), constant_values=((6, 7), (8, 9)))))
# fmt: off
image = np.array([[
[0, 1, 2],
[3, 4, 5],
[6, 7, 8],
]])
# fmt: on
# Test padding with PaddingMode.REFLECT
# fmt: off
expected_image = np.array([[
[2, 1, 0, 1, 2, 1],
[5, 4, 3, 4, 5, 4],
[8, 7, 6, 7, 8, 7],
[5, 4, 3, 4, 5, 4],
[2, 1, 0, 1, 2, 1],
]])
# fmt: on
self.assertTrue(np.allclose(expected_image, pad(image, ((0, 2), (2, 1)), mode="reflect")))
# Test padding with PaddingMode.REPLICATE
# fmt: off
expected_image = np.array([[
[0, 0, 0, 1, 2, 2],
[3, 3, 3, 4, 5, 5],
[6, 6, 6, 7, 8, 8],
[6, 6, 6, 7, 8, 8],
[6, 6, 6, 7, 8, 8],
]])
# fmt: on
self.assertTrue(np.allclose(expected_image, pad(image, ((0, 2), (2, 1)), mode="replicate")))
# Test padding with PaddingMode.SYMMETRIC
# fmt: off
expected_image = np.array([[
[1, 0, 0, 1, 2, 2],
[4, 3, 3, 4, 5, 5],
[7, 6, 6, 7, 8, 8],
[7, 6, 6, 7, 8, 8],
[4, 3, 3, 4, 5, 5],
]])
# fmt: on
self.assertTrue(np.allclose(expected_image, pad(image, ((0, 2), (2, 1)), mode="symmetric")))
# Test we can specify the output data format
# Test padding with PaddingMode.REFLECT
# fmt: off
image = np.array([[
[0, 1],
[2, 3],
]])
expected_image = np.array([
[[0], [1], [0], [1], [0]],
[[2], [3], [2], [3], [2]],
[[0], [1], [0], [1], [0]],
[[2], [3], [2], [3], [2]]
])
# fmt: on
self.assertTrue(
np.allclose(expected_image, pad(image, ((0, 2), (2, 1)), mode="reflect", data_format="channels_last"))
)
# Test we can pad on an image with 2 channels
# fmt: off
image = np.array([
[[0, 1], [2, 3]],
])
expected_image = np.array([
[[0, 0], [0, 1], [2, 3]],
[[0, 0], [0, 0], [0, 0]],
])
# fmt: on
self.assertTrue(
np.allclose(
expected_image, pad(image, ((0, 1), (1, 0)), mode="constant", input_data_format="channels_last")
)
)
@require_vision
def test_convert_to_rgb(self):
# Test that an RGBA image is converted to RGB
image = np.array([[[1, 2, 3, 4], [5, 6, 7, 8]]], dtype=np.uint8)
pil_image = PIL.Image.fromarray(image)
self.assertEqual(pil_image.mode, "RGBA")
self.assertEqual(pil_image.size, (2, 1))
# For the moment, numpy images are returned as is
rgb_image = convert_to_rgb(image)
self.assertEqual(rgb_image.shape, (1, 2, 4))
self.assertTrue(np.allclose(rgb_image, image))
# And PIL images are converted
rgb_image = convert_to_rgb(pil_image)
self.assertEqual(rgb_image.mode, "RGB")
self.assertEqual(rgb_image.size, (2, 1))
self.assertTrue(np.allclose(np.array(rgb_image), np.array([[[1, 2, 3], [5, 6, 7]]], dtype=np.uint8)))
# Test that a grayscale image is converted to RGB
image = np.array([[0, 255]], dtype=np.uint8)
pil_image = PIL.Image.fromarray(image)
self.assertEqual(pil_image.mode, "L")
self.assertEqual(pil_image.size, (2, 1))
rgb_image = convert_to_rgb(pil_image)
self.assertEqual(rgb_image.mode, "RGB")
self.assertEqual(rgb_image.size, (2, 1))
self.assertTrue(np.allclose(np.array(rgb_image), np.array([[[0, 0, 0], [255, 255, 255]]], dtype=np.uint8)))
def test_flip_channel_order(self):
# fmt: off
img_channels_first = np.array([
[[ 0, 1, 2, 3],
[ 4, 5, 6, 7]],
[[ 8, 9, 10, 11],
[12, 13, 14, 15]],
[[16, 17, 18, 19],
[20, 21, 22, 23]],
])
# fmt: on
img_channels_last = np.moveaxis(img_channels_first, 0, -1)
# fmt: off
flipped_img_channels_first = np.array([
[[16, 17, 18, 19],
[20, 21, 22, 23]],
[[ 8, 9, 10, 11],
[12, 13, 14, 15]],
[[ 0, 1, 2, 3],
[ 4, 5, 6, 7]],
])
# fmt: on
flipped_img_channels_last = np.moveaxis(flipped_img_channels_first, 0, -1)
self.assertTrue(np.allclose(flip_channel_order(img_channels_first), flipped_img_channels_first))
self.assertTrue(
np.allclose(flip_channel_order(img_channels_first, "channels_last"), flipped_img_channels_last)
)
self.assertTrue(np.allclose(flip_channel_order(img_channels_last), flipped_img_channels_last))
self.assertTrue(
np.allclose(flip_channel_order(img_channels_last, "channels_first"), flipped_img_channels_first)
)
# Can flip when the image has 2 channels
# fmt: off
img_channels_first = np.array([
[[ 0, 1, 2, 3],
[ 4, 5, 6, 7]],
[[ 8, 9, 10, 11],
[12, 13, 14, 15]],
])
# fmt: on
flipped_img_channels_first = img_channels_first[::-1, :, :]
self.assertTrue(
np.allclose(
flip_channel_order(img_channels_first, input_data_format="channels_first"), flipped_img_channels_first
)
)
| transformers/tests/test_image_transforms.py/0 | {
"file_path": "transformers/tests/test_image_transforms.py",
"repo_id": "transformers",
"token_count": 12379
} | 373 |
# coding=utf-8
# Copyright 2023 HuggingFace Inc.
#
# Licensed under the Apache License, Version 2.0 (the "License");
# you may not use this file except in compliance with the License.
# You may obtain a copy of the License at
#
# http://www.apache.org/licenses/LICENSE-2.0
#
# Unless required by applicable law or agreed to in writing, software
# distributed under the License is distributed on an "AS IS" BASIS,
# WITHOUT WARRANTIES OR CONDITIONS OF ANY KIND, either express or implied.
# See the License for the specific language governing permissions and
# limitations under the License.
import os
import tempfile
import unittest
import uuid
from pathlib import Path
from transformers.testing_utils import get_tests_dir, require_soundfile, require_torch, require_vision
from transformers.tools.agent_types import AgentAudio, AgentImage, AgentText
from transformers.utils import is_soundfile_availble, is_torch_available, is_vision_available
if is_torch_available():
import torch
if is_soundfile_availble():
import soundfile as sf
if is_vision_available():
from PIL import Image
def get_new_path(suffix="") -> str:
directory = tempfile.mkdtemp()
return os.path.join(directory, str(uuid.uuid4()) + suffix)
@require_soundfile
@require_torch
class AgentAudioTests(unittest.TestCase):
def test_from_tensor(self):
tensor = torch.rand(12, dtype=torch.float64) - 0.5
agent_type = AgentAudio(tensor)
path = str(agent_type.to_string())
# Ensure that the tensor and the agent_type's tensor are the same
self.assertTrue(torch.allclose(tensor, agent_type.to_raw(), atol=1e-4))
del agent_type
# Ensure the path remains even after the object deletion
self.assertTrue(os.path.exists(path))
# Ensure that the file contains the same value as the original tensor
new_tensor, _ = sf.read(path)
self.assertTrue(torch.allclose(tensor, torch.tensor(new_tensor), atol=1e-4))
def test_from_string(self):
tensor = torch.rand(12, dtype=torch.float64) - 0.5
path = get_new_path(suffix=".wav")
sf.write(path, tensor, 16000)
agent_type = AgentAudio(path)
self.assertTrue(torch.allclose(tensor, agent_type.to_raw(), atol=1e-4))
self.assertEqual(agent_type.to_string(), path)
@require_vision
@require_torch
class AgentImageTests(unittest.TestCase):
def test_from_tensor(self):
tensor = torch.randint(0, 256, (64, 64, 3))
agent_type = AgentImage(tensor)
path = str(agent_type.to_string())
# Ensure that the tensor and the agent_type's tensor are the same
self.assertTrue(torch.allclose(tensor, agent_type._tensor, atol=1e-4))
self.assertIsInstance(agent_type.to_raw(), Image.Image)
# Ensure the path remains even after the object deletion
del agent_type
self.assertTrue(os.path.exists(path))
def test_from_string(self):
path = Path(get_tests_dir("fixtures/tests_samples/COCO")) / "000000039769.png"
image = Image.open(path)
agent_type = AgentImage(path)
self.assertTrue(path.samefile(agent_type.to_string()))
self.assertTrue(image == agent_type.to_raw())
# Ensure the path remains even after the object deletion
del agent_type
self.assertTrue(os.path.exists(path))
def test_from_image(self):
path = Path(get_tests_dir("fixtures/tests_samples/COCO")) / "000000039769.png"
image = Image.open(path)
agent_type = AgentImage(image)
self.assertFalse(path.samefile(agent_type.to_string()))
self.assertTrue(image == agent_type.to_raw())
# Ensure the path remains even after the object deletion
del agent_type
self.assertTrue(os.path.exists(path))
class AgentTextTests(unittest.TestCase):
def test_from_string(self):
string = "Hey!"
agent_type = AgentText(string)
self.assertEqual(string, agent_type.to_string())
self.assertEqual(string, agent_type.to_raw())
self.assertEqual(string, agent_type)
| transformers/tests/tools/test_agent_types.py/0 | {
"file_path": "transformers/tests/tools/test_agent_types.py",
"repo_id": "transformers",
"token_count": 1583
} | 374 |
# Copyright 2020 The HuggingFace Team. All rights reserved.
#
# Licensed under the Apache License, Version 2.0 (the "License");
# you may not use this file except in compliance with the License.
# You may obtain a copy of the License at
#
# http://www.apache.org/licenses/LICENSE-2.0
#
# Unless required by applicable law or agreed to in writing, software
# distributed under the License is distributed on an "AS IS" BASIS,
# WITHOUT WARRANTIES OR CONDITIONS OF ANY KIND, either express or implied.
# See the License for the specific language governing permissions and
# limitations under the License.
import shutil
import tempfile
import unittest
from unittest.mock import patch
from transformers import (
DefaultFlowCallback,
IntervalStrategy,
PrinterCallback,
ProgressCallback,
Trainer,
TrainerCallback,
TrainingArguments,
is_torch_available,
)
from transformers.testing_utils import require_torch
if is_torch_available():
from transformers.trainer import DEFAULT_CALLBACKS
from .test_trainer import RegressionDataset, RegressionModelConfig, RegressionPreTrainedModel
class MyTestTrainerCallback(TrainerCallback):
"A callback that registers the events that goes through."
def __init__(self):
self.events = []
def on_init_end(self, args, state, control, **kwargs):
self.events.append("on_init_end")
def on_train_begin(self, args, state, control, **kwargs):
self.events.append("on_train_begin")
def on_train_end(self, args, state, control, **kwargs):
self.events.append("on_train_end")
def on_epoch_begin(self, args, state, control, **kwargs):
self.events.append("on_epoch_begin")
def on_epoch_end(self, args, state, control, **kwargs):
self.events.append("on_epoch_end")
def on_step_begin(self, args, state, control, **kwargs):
self.events.append("on_step_begin")
def on_step_end(self, args, state, control, **kwargs):
self.events.append("on_step_end")
def on_evaluate(self, args, state, control, **kwargs):
self.events.append("on_evaluate")
def on_predict(self, args, state, control, **kwargs):
self.events.append("on_predict")
def on_save(self, args, state, control, **kwargs):
self.events.append("on_save")
def on_log(self, args, state, control, **kwargs):
self.events.append("on_log")
def on_prediction_step(self, args, state, control, **kwargs):
self.events.append("on_prediction_step")
@require_torch
class TrainerCallbackTest(unittest.TestCase):
def setUp(self):
self.output_dir = tempfile.mkdtemp()
def tearDown(self):
shutil.rmtree(self.output_dir)
def get_trainer(self, a=0, b=0, train_len=64, eval_len=64, callbacks=None, disable_tqdm=False, **kwargs):
# disable_tqdm in TrainingArguments has a flaky default since it depends on the level of logging. We make sure
# its set to False since the tests later on depend on its value.
train_dataset = RegressionDataset(length=train_len)
eval_dataset = RegressionDataset(length=eval_len)
config = RegressionModelConfig(a=a, b=b)
model = RegressionPreTrainedModel(config)
args = TrainingArguments(self.output_dir, disable_tqdm=disable_tqdm, report_to=[], **kwargs)
return Trainer(
model,
args,
train_dataset=train_dataset,
eval_dataset=eval_dataset,
callbacks=callbacks,
)
def check_callbacks_equality(self, cbs1, cbs2):
self.assertEqual(len(cbs1), len(cbs2))
# Order doesn't matter
cbs1 = sorted(cbs1, key=lambda cb: cb.__name__ if isinstance(cb, type) else cb.__class__.__name__)
cbs2 = sorted(cbs2, key=lambda cb: cb.__name__ if isinstance(cb, type) else cb.__class__.__name__)
for cb1, cb2 in zip(cbs1, cbs2):
if isinstance(cb1, type) and isinstance(cb2, type):
self.assertEqual(cb1, cb2)
elif isinstance(cb1, type) and not isinstance(cb2, type):
self.assertEqual(cb1, cb2.__class__)
elif not isinstance(cb1, type) and isinstance(cb2, type):
self.assertEqual(cb1.__class__, cb2)
else:
self.assertEqual(cb1, cb2)
def get_expected_events(self, trainer):
expected_events = ["on_init_end", "on_train_begin"]
step = 0
train_dl_len = len(trainer.get_eval_dataloader())
evaluation_events = ["on_prediction_step"] * len(trainer.get_eval_dataloader()) + ["on_log", "on_evaluate"]
for _ in range(trainer.state.num_train_epochs):
expected_events.append("on_epoch_begin")
for _ in range(train_dl_len):
step += 1
expected_events += ["on_step_begin", "on_step_end"]
if step % trainer.args.logging_steps == 0:
expected_events.append("on_log")
if trainer.args.evaluation_strategy == IntervalStrategy.STEPS and step % trainer.args.eval_steps == 0:
expected_events += evaluation_events.copy()
if step % trainer.args.save_steps == 0:
expected_events.append("on_save")
expected_events.append("on_epoch_end")
if trainer.args.evaluation_strategy == IntervalStrategy.EPOCH:
expected_events += evaluation_events.copy()
expected_events += ["on_log", "on_train_end"]
return expected_events
def test_init_callback(self):
trainer = self.get_trainer()
expected_callbacks = DEFAULT_CALLBACKS.copy() + [ProgressCallback]
self.check_callbacks_equality(trainer.callback_handler.callbacks, expected_callbacks)
# Callbacks passed at init are added to the default callbacks
trainer = self.get_trainer(callbacks=[MyTestTrainerCallback])
expected_callbacks.append(MyTestTrainerCallback)
self.check_callbacks_equality(trainer.callback_handler.callbacks, expected_callbacks)
# TrainingArguments.disable_tqdm controls if use ProgressCallback or PrinterCallback
trainer = self.get_trainer(disable_tqdm=True)
expected_callbacks = DEFAULT_CALLBACKS.copy() + [PrinterCallback]
self.check_callbacks_equality(trainer.callback_handler.callbacks, expected_callbacks)
def test_add_remove_callback(self):
expected_callbacks = DEFAULT_CALLBACKS.copy() + [ProgressCallback]
trainer = self.get_trainer()
# We can add, pop, or remove by class name
trainer.remove_callback(DefaultFlowCallback)
expected_callbacks.remove(DefaultFlowCallback)
self.check_callbacks_equality(trainer.callback_handler.callbacks, expected_callbacks)
trainer = self.get_trainer()
cb = trainer.pop_callback(DefaultFlowCallback)
self.assertEqual(cb.__class__, DefaultFlowCallback)
self.check_callbacks_equality(trainer.callback_handler.callbacks, expected_callbacks)
trainer.add_callback(DefaultFlowCallback)
expected_callbacks.insert(0, DefaultFlowCallback)
self.check_callbacks_equality(trainer.callback_handler.callbacks, expected_callbacks)
# We can also add, pop, or remove by instance
trainer = self.get_trainer()
cb = trainer.callback_handler.callbacks[0]
trainer.remove_callback(cb)
expected_callbacks.remove(DefaultFlowCallback)
self.check_callbacks_equality(trainer.callback_handler.callbacks, expected_callbacks)
trainer = self.get_trainer()
cb1 = trainer.callback_handler.callbacks[0]
cb2 = trainer.pop_callback(cb1)
self.assertEqual(cb1, cb2)
self.check_callbacks_equality(trainer.callback_handler.callbacks, expected_callbacks)
trainer.add_callback(cb1)
expected_callbacks.insert(0, DefaultFlowCallback)
self.check_callbacks_equality(trainer.callback_handler.callbacks, expected_callbacks)
def test_event_flow(self):
import warnings
# XXX: for now ignore scatter_gather warnings in this test since it's not relevant to what's being tested
warnings.simplefilter(action="ignore", category=UserWarning)
trainer = self.get_trainer(callbacks=[MyTestTrainerCallback])
trainer.train()
events = trainer.callback_handler.callbacks[-2].events
self.assertEqual(events, self.get_expected_events(trainer))
# Independent log/save/eval
trainer = self.get_trainer(callbacks=[MyTestTrainerCallback], logging_steps=5)
trainer.train()
events = trainer.callback_handler.callbacks[-2].events
self.assertEqual(events, self.get_expected_events(trainer))
trainer = self.get_trainer(callbacks=[MyTestTrainerCallback], save_steps=5)
trainer.train()
events = trainer.callback_handler.callbacks[-2].events
self.assertEqual(events, self.get_expected_events(trainer))
trainer = self.get_trainer(callbacks=[MyTestTrainerCallback], eval_steps=5, evaluation_strategy="steps")
trainer.train()
events = trainer.callback_handler.callbacks[-2].events
self.assertEqual(events, self.get_expected_events(trainer))
trainer = self.get_trainer(callbacks=[MyTestTrainerCallback], evaluation_strategy="epoch")
trainer.train()
events = trainer.callback_handler.callbacks[-2].events
self.assertEqual(events, self.get_expected_events(trainer))
# A bit of everything
trainer = self.get_trainer(
callbacks=[MyTestTrainerCallback],
logging_steps=3,
save_steps=10,
eval_steps=5,
evaluation_strategy="steps",
)
trainer.train()
events = trainer.callback_handler.callbacks[-2].events
self.assertEqual(events, self.get_expected_events(trainer))
# warning should be emitted for duplicated callbacks
with patch("transformers.trainer_callback.logger.warning") as warn_mock:
trainer = self.get_trainer(
callbacks=[MyTestTrainerCallback, MyTestTrainerCallback],
)
assert str(MyTestTrainerCallback) in warn_mock.call_args[0][0]
| transformers/tests/trainer/test_trainer_callback.py/0 | {
"file_path": "transformers/tests/trainer/test_trainer_callback.py",
"repo_id": "transformers",
"token_count": 4143
} | 375 |
# coding=utf-8
# Copyright 2019-present, the HuggingFace Inc. team.
#
# Licensed under the Apache License, Version 2.0 (the "License");
# you may not use this file except in compliance with the License.
# You may obtain a copy of the License at
#
# http://www.apache.org/licenses/LICENSE-2.0
#
# Unless required by applicable law or agreed to in writing, software
# distributed under the License is distributed on an "AS IS" BASIS,
# WITHOUT WARRANTIES OR CONDITIONS OF ANY KIND, either express or implied.
# See the License for the specific language governing permissions and
# limitations under the License.
import unittest
import numpy as np
from transformers.testing_utils import require_flax, require_tf, require_torch
from transformers.utils import (
expand_dims,
flatten_dict,
is_flax_available,
is_tf_available,
is_torch_available,
reshape,
squeeze,
transpose,
)
if is_flax_available():
import jax.numpy as jnp
if is_tf_available():
import tensorflow as tf
if is_torch_available():
import torch
class GenericTester(unittest.TestCase):
def test_flatten_dict(self):
input_dict = {
"task_specific_params": {
"summarization": {"length_penalty": 1.0, "max_length": 128, "min_length": 12, "num_beams": 4},
"summarization_cnn": {"length_penalty": 2.0, "max_length": 142, "min_length": 56, "num_beams": 4},
"summarization_xsum": {"length_penalty": 1.0, "max_length": 62, "min_length": 11, "num_beams": 6},
}
}
expected_dict = {
"task_specific_params.summarization.length_penalty": 1.0,
"task_specific_params.summarization.max_length": 128,
"task_specific_params.summarization.min_length": 12,
"task_specific_params.summarization.num_beams": 4,
"task_specific_params.summarization_cnn.length_penalty": 2.0,
"task_specific_params.summarization_cnn.max_length": 142,
"task_specific_params.summarization_cnn.min_length": 56,
"task_specific_params.summarization_cnn.num_beams": 4,
"task_specific_params.summarization_xsum.length_penalty": 1.0,
"task_specific_params.summarization_xsum.max_length": 62,
"task_specific_params.summarization_xsum.min_length": 11,
"task_specific_params.summarization_xsum.num_beams": 6,
}
self.assertEqual(flatten_dict(input_dict), expected_dict)
def test_transpose_numpy(self):
x = np.random.randn(3, 4)
self.assertTrue(np.allclose(transpose(x), x.transpose()))
x = np.random.randn(3, 4, 5)
self.assertTrue(np.allclose(transpose(x, axes=(1, 2, 0)), x.transpose((1, 2, 0))))
@require_torch
def test_transpose_torch(self):
x = np.random.randn(3, 4)
t = torch.tensor(x)
self.assertTrue(np.allclose(transpose(x), transpose(t).numpy()))
x = np.random.randn(3, 4, 5)
t = torch.tensor(x)
self.assertTrue(np.allclose(transpose(x, axes=(1, 2, 0)), transpose(t, axes=(1, 2, 0)).numpy()))
@require_tf
def test_transpose_tf(self):
x = np.random.randn(3, 4)
t = tf.constant(x)
self.assertTrue(np.allclose(transpose(x), transpose(t).numpy()))
x = np.random.randn(3, 4, 5)
t = tf.constant(x)
self.assertTrue(np.allclose(transpose(x, axes=(1, 2, 0)), transpose(t, axes=(1, 2, 0)).numpy()))
@require_flax
def test_transpose_flax(self):
x = np.random.randn(3, 4)
t = jnp.array(x)
self.assertTrue(np.allclose(transpose(x), np.asarray(transpose(t))))
x = np.random.randn(3, 4, 5)
t = jnp.array(x)
self.assertTrue(np.allclose(transpose(x, axes=(1, 2, 0)), np.asarray(transpose(t, axes=(1, 2, 0)))))
def test_reshape_numpy(self):
x = np.random.randn(3, 4)
self.assertTrue(np.allclose(reshape(x, (4, 3)), np.reshape(x, (4, 3))))
x = np.random.randn(3, 4, 5)
self.assertTrue(np.allclose(reshape(x, (12, 5)), np.reshape(x, (12, 5))))
@require_torch
def test_reshape_torch(self):
x = np.random.randn(3, 4)
t = torch.tensor(x)
self.assertTrue(np.allclose(reshape(x, (4, 3)), reshape(t, (4, 3)).numpy()))
x = np.random.randn(3, 4, 5)
t = torch.tensor(x)
self.assertTrue(np.allclose(reshape(x, (12, 5)), reshape(t, (12, 5)).numpy()))
@require_tf
def test_reshape_tf(self):
x = np.random.randn(3, 4)
t = tf.constant(x)
self.assertTrue(np.allclose(reshape(x, (4, 3)), reshape(t, (4, 3)).numpy()))
x = np.random.randn(3, 4, 5)
t = tf.constant(x)
self.assertTrue(np.allclose(reshape(x, (12, 5)), reshape(t, (12, 5)).numpy()))
@require_flax
def test_reshape_flax(self):
x = np.random.randn(3, 4)
t = jnp.array(x)
self.assertTrue(np.allclose(reshape(x, (4, 3)), np.asarray(reshape(t, (4, 3)))))
x = np.random.randn(3, 4, 5)
t = jnp.array(x)
self.assertTrue(np.allclose(reshape(x, (12, 5)), np.asarray(reshape(t, (12, 5)))))
def test_squeeze_numpy(self):
x = np.random.randn(1, 3, 4)
self.assertTrue(np.allclose(squeeze(x), np.squeeze(x)))
x = np.random.randn(1, 4, 1, 5)
self.assertTrue(np.allclose(squeeze(x, axis=2), np.squeeze(x, axis=2)))
@require_torch
def test_squeeze_torch(self):
x = np.random.randn(1, 3, 4)
t = torch.tensor(x)
self.assertTrue(np.allclose(squeeze(x), squeeze(t).numpy()))
x = np.random.randn(1, 4, 1, 5)
t = torch.tensor(x)
self.assertTrue(np.allclose(squeeze(x, axis=2), squeeze(t, axis=2).numpy()))
@require_tf
def test_squeeze_tf(self):
x = np.random.randn(1, 3, 4)
t = tf.constant(x)
self.assertTrue(np.allclose(squeeze(x), squeeze(t).numpy()))
x = np.random.randn(1, 4, 1, 5)
t = tf.constant(x)
self.assertTrue(np.allclose(squeeze(x, axis=2), squeeze(t, axis=2).numpy()))
@require_flax
def test_squeeze_flax(self):
x = np.random.randn(1, 3, 4)
t = jnp.array(x)
self.assertTrue(np.allclose(squeeze(x), np.asarray(squeeze(t))))
x = np.random.randn(1, 4, 1, 5)
t = jnp.array(x)
self.assertTrue(np.allclose(squeeze(x, axis=2), np.asarray(squeeze(t, axis=2))))
def test_expand_dims_numpy(self):
x = np.random.randn(3, 4)
self.assertTrue(np.allclose(expand_dims(x, axis=1), np.expand_dims(x, axis=1)))
@require_torch
def test_expand_dims_torch(self):
x = np.random.randn(3, 4)
t = torch.tensor(x)
self.assertTrue(np.allclose(expand_dims(x, axis=1), expand_dims(t, axis=1).numpy()))
@require_tf
def test_expand_dims_tf(self):
x = np.random.randn(3, 4)
t = tf.constant(x)
self.assertTrue(np.allclose(expand_dims(x, axis=1), expand_dims(t, axis=1).numpy()))
@require_flax
def test_expand_dims_flax(self):
x = np.random.randn(3, 4)
t = jnp.array(x)
self.assertTrue(np.allclose(expand_dims(x, axis=1), np.asarray(expand_dims(t, axis=1))))
| transformers/tests/utils/test_generic.py/0 | {
"file_path": "transformers/tests/utils/test_generic.py",
"repo_id": "transformers",
"token_count": 3485
} | 376 |
# coding=utf-8
# Copyright 2022 The HuggingFace Inc. team.
#
# Licensed under the Apache License, Version 2.0 (the "License");
# you may not use this file except in compliance with the License.
# You may obtain a copy of the License at
#
# http://www.apache.org/licenses/LICENSE-2.0
#
# Unless required by applicable law or agreed to in writing, software
# distributed under the License is distributed on an "AS IS" BASIS,
# WITHOUT WARRANTIES OR CONDITIONS OF ANY KIND, either express or implied.
# See the License for the specific language governing permissions and
# limitations under the License.
import inspect
import re
from transformers.utils import direct_transformers_import
# All paths are set with the intent you should run this script from the root of the repo with the command
# python utils/check_config_docstrings.py
PATH_TO_TRANSFORMERS = "src/transformers"
# This is to make sure the transformers module imported is the one in the repo.
transformers = direct_transformers_import(PATH_TO_TRANSFORMERS)
CONFIG_MAPPING = transformers.models.auto.configuration_auto.CONFIG_MAPPING
# Regex pattern used to find the checkpoint mentioned in the docstring of `config_class`.
# For example, `[bert-base-uncased](https://huggingface.co/bert-base-uncased)`
_re_checkpoint = re.compile(r"\[(.+?)\]\((https://huggingface\.co/.+?)\)")
CONFIG_CLASSES_TO_IGNORE_FOR_DOCSTRING_CHECKPOINT_CHECK = {
"DecisionTransformerConfig",
"EncoderDecoderConfig",
"MusicgenConfig",
"RagConfig",
"SpeechEncoderDecoderConfig",
"TimmBackboneConfig",
"VisionEncoderDecoderConfig",
"VisionTextDualEncoderConfig",
"LlamaConfig",
}
def get_checkpoint_from_config_class(config_class):
checkpoint = None
# source code of `config_class`
config_source = inspect.getsource(config_class)
checkpoints = _re_checkpoint.findall(config_source)
# Each `checkpoint` is a tuple of a checkpoint name and a checkpoint link.
# For example, `('bert-base-uncased', 'https://huggingface.co/bert-base-uncased')`
for ckpt_name, ckpt_link in checkpoints:
# allow the link to end with `/`
if ckpt_link.endswith("/"):
ckpt_link = ckpt_link[:-1]
# verify the checkpoint name corresponds to the checkpoint link
ckpt_link_from_name = f"https://huggingface.co/{ckpt_name}"
if ckpt_link == ckpt_link_from_name:
checkpoint = ckpt_name
break
return checkpoint
def check_config_docstrings_have_checkpoints():
configs_without_checkpoint = []
for config_class in list(CONFIG_MAPPING.values()):
# Skip deprecated models
if "models.deprecated" in config_class.__module__:
continue
checkpoint = get_checkpoint_from_config_class(config_class)
name = config_class.__name__
if checkpoint is None and name not in CONFIG_CLASSES_TO_IGNORE_FOR_DOCSTRING_CHECKPOINT_CHECK:
configs_without_checkpoint.append(name)
if len(configs_without_checkpoint) > 0:
message = "\n".join(sorted(configs_without_checkpoint))
raise ValueError(
f"The following configurations don't contain any valid checkpoint:\n{message}\n\n"
"The requirement is to include a link pointing to one of the models of this architecture in the "
"docstring of the config classes listed above. The link should have be a markdown format like "
"[myorg/mymodel](https://huggingface.co/myorg/mymodel)."
)
if __name__ == "__main__":
check_config_docstrings_have_checkpoints()
| transformers/utils/check_config_docstrings.py/0 | {
"file_path": "transformers/utils/check_config_docstrings.py",
"repo_id": "transformers",
"token_count": 1277
} | 377 |
""" Script for downloading all GLUE data.
Original source: https://gist.github.com/W4ngatang/60c2bdb54d156a41194446737ce03e2e
Note: for legal reasons, we are unable to host MRPC.
You can either use the version hosted by the SentEval team, which is already tokenized,
or you can download the original data from (https://download.microsoft.com/download/D/4/6/D46FF87A-F6B9-4252-AA8B-3604ED519838/MSRParaphraseCorpus.msi) and extract the data from it manually.
For Windows users, you can run the .msi file. For Mac and Linux users, consider an external library such as 'cabextract' (see below for an example).
You should then rename and place specific files in a folder (see below for an example).
mkdir MRPC
cabextract MSRParaphraseCorpus.msi -d MRPC
cat MRPC/_2DEC3DBE877E4DB192D17C0256E90F1D | tr -d $'\r' > MRPC/msr_paraphrase_train.txt
cat MRPC/_D7B391F9EAFF4B1B8BCE8F21B20B1B61 | tr -d $'\r' > MRPC/msr_paraphrase_test.txt
rm MRPC/_*
rm MSRParaphraseCorpus.msi
1/30/19: It looks like SentEval is no longer hosting their extracted and tokenized MRPC data, so you'll need to download the data from the original source for now.
2/11/19: It looks like SentEval actually *is* hosting the extracted data. Hooray!
"""
import argparse
import os
import sys
import urllib.request
import zipfile
TASKS = ["CoLA", "SST", "MRPC", "QQP", "STS", "MNLI", "SNLI", "QNLI", "RTE", "WNLI", "diagnostic"]
TASK2PATH = {
"CoLA": "https://firebasestorage.googleapis.com/v0/b/mtl-sentence-representations.appspot.com/o/data%2FCoLA.zip?alt=media&token=46d5e637-3411-4188-bc44-5809b5bfb5f4",
"SST": "https://firebasestorage.googleapis.com/v0/b/mtl-sentence-representations.appspot.com/o/data%2FSST-2.zip?alt=media&token=aabc5f6b-e466-44a2-b9b4-cf6337f84ac8",
"MRPC": "https://firebasestorage.googleapis.com/v0/b/mtl-sentence-representations.appspot.com/o/data%2Fmrpc_dev_ids.tsv?alt=media&token=ec5c0836-31d5-48f4-b431-7480817f1adc",
"QQP": "https://firebasestorage.googleapis.com/v0/b/mtl-sentence-representations.appspot.com/o/data%2FQQP.zip?alt=media&token=700c6acf-160d-4d89-81d1-de4191d02cb5",
"STS": "https://firebasestorage.googleapis.com/v0/b/mtl-sentence-representations.appspot.com/o/data%2FSTS-B.zip?alt=media&token=bddb94a7-8706-4e0d-a694-1109e12273b5",
"MNLI": "https://firebasestorage.googleapis.com/v0/b/mtl-sentence-representations.appspot.com/o/data%2FMNLI.zip?alt=media&token=50329ea1-e339-40e2-809c-10c40afff3ce",
"SNLI": "https://firebasestorage.googleapis.com/v0/b/mtl-sentence-representations.appspot.com/o/data%2FSNLI.zip?alt=media&token=4afcfbb2-ff0c-4b2d-a09a-dbf07926f4df",
"QNLI": "https://firebasestorage.googleapis.com/v0/b/mtl-sentence-representations.appspot.com/o/data%2FQNLIv2.zip?alt=media&token=6fdcf570-0fc5-4631-8456-9505272d1601",
"RTE": "https://firebasestorage.googleapis.com/v0/b/mtl-sentence-representations.appspot.com/o/data%2FRTE.zip?alt=media&token=5efa7e85-a0bb-4f19-8ea2-9e1840f077fb",
"WNLI": "https://firebasestorage.googleapis.com/v0/b/mtl-sentence-representations.appspot.com/o/data%2FWNLI.zip?alt=media&token=068ad0a0-ded7-4bd7-99a5-5e00222e0faf",
"diagnostic": "https://storage.googleapis.com/mtl-sentence-representations.appspot.com/tsvsWithoutLabels%2FAX.tsv?GoogleAccessId=firebase-adminsdk-0khhl@mtl-sentence-representations.iam.gserviceaccount.com&Expires=2498860800&Signature=DuQ2CSPt2Yfre0C%2BiISrVYrIFaZH1Lc7hBVZDD4ZyR7fZYOMNOUGpi8QxBmTNOrNPjR3z1cggo7WXFfrgECP6FBJSsURv8Ybrue8Ypt%2FTPxbuJ0Xc2FhDi%2BarnecCBFO77RSbfuz%2Bs95hRrYhTnByqu3U%2FYZPaj3tZt5QdfpH2IUROY8LiBXoXS46LE%2FgOQc%2FKN%2BA9SoscRDYsnxHfG0IjXGwHN%2Bf88q6hOmAxeNPx6moDulUF6XMUAaXCSFU%2BnRO2RDL9CapWxj%2BDl7syNyHhB7987hZ80B%2FwFkQ3MEs8auvt5XW1%2Bd4aCU7ytgM69r8JDCwibfhZxpaa4gd50QXQ%3D%3D",
}
MRPC_TRAIN = "https://dl.fbaipublicfiles.com/senteval/senteval_data/msr_paraphrase_train.txt"
MRPC_TEST = "https://dl.fbaipublicfiles.com/senteval/senteval_data/msr_paraphrase_test.txt"
def download_and_extract(task, data_dir):
print(f"Downloading and extracting {task}...")
data_file = f"{task}.zip"
urllib.request.urlretrieve(TASK2PATH[task], data_file)
with zipfile.ZipFile(data_file) as zip_ref:
zip_ref.extractall(data_dir)
os.remove(data_file)
print("\tCompleted!")
def format_mrpc(data_dir, path_to_data):
print("Processing MRPC...")
mrpc_dir = os.path.join(data_dir, "MRPC")
if not os.path.isdir(mrpc_dir):
os.mkdir(mrpc_dir)
if path_to_data:
mrpc_train_file = os.path.join(path_to_data, "msr_paraphrase_train.txt")
mrpc_test_file = os.path.join(path_to_data, "msr_paraphrase_test.txt")
else:
print("Local MRPC data not specified, downloading data from %s" % MRPC_TRAIN)
mrpc_train_file = os.path.join(mrpc_dir, "msr_paraphrase_train.txt")
mrpc_test_file = os.path.join(mrpc_dir, "msr_paraphrase_test.txt")
urllib.request.urlretrieve(MRPC_TRAIN, mrpc_train_file)
urllib.request.urlretrieve(MRPC_TEST, mrpc_test_file)
if not os.path.isfile(mrpc_train_file):
raise ValueError(f"Train data not found at {mrpc_train_file}")
if not os.path.isfile(mrpc_test_file):
raise ValueError(f"Test data not found at {mrpc_test_file}")
urllib.request.urlretrieve(TASK2PATH["MRPC"], os.path.join(mrpc_dir, "dev_ids.tsv"))
dev_ids = []
with open(os.path.join(mrpc_dir, "dev_ids.tsv"), encoding="utf8") as ids_fh:
for row in ids_fh:
dev_ids.append(row.strip().split("\t"))
with open(mrpc_train_file, encoding="utf8") as data_fh, open(
os.path.join(mrpc_dir, "train.tsv"), "w", encoding="utf8"
) as train_fh, open(os.path.join(mrpc_dir, "dev.tsv"), "w", encoding="utf8") as dev_fh:
header = data_fh.readline()
train_fh.write(header)
dev_fh.write(header)
for row in data_fh:
label, id1, id2, s1, s2 = row.strip().split("\t")
if [id1, id2] in dev_ids:
dev_fh.write("%s\t%s\t%s\t%s\t%s\n" % (label, id1, id2, s1, s2))
else:
train_fh.write("%s\t%s\t%s\t%s\t%s\n" % (label, id1, id2, s1, s2))
with open(mrpc_test_file, encoding="utf8") as data_fh, open(
os.path.join(mrpc_dir, "test.tsv"), "w", encoding="utf8"
) as test_fh:
header = data_fh.readline()
test_fh.write("index\t#1 ID\t#2 ID\t#1 String\t#2 String\n")
for idx, row in enumerate(data_fh):
label, id1, id2, s1, s2 = row.strip().split("\t")
test_fh.write("%d\t%s\t%s\t%s\t%s\n" % (idx, id1, id2, s1, s2))
print("\tCompleted!")
def download_diagnostic(data_dir):
print("Downloading and extracting diagnostic...")
if not os.path.isdir(os.path.join(data_dir, "diagnostic")):
os.mkdir(os.path.join(data_dir, "diagnostic"))
data_file = os.path.join(data_dir, "diagnostic", "diagnostic.tsv")
urllib.request.urlretrieve(TASK2PATH["diagnostic"], data_file)
print("\tCompleted!")
return
def get_tasks(task_names):
task_names = task_names.split(",")
if "all" in task_names:
tasks = TASKS
else:
tasks = []
for task_name in task_names:
if task_name not in TASKS:
raise ValueError(f"Task {task_name} not found!")
tasks.append(task_name)
return tasks
def main(arguments):
parser = argparse.ArgumentParser()
parser.add_argument("--data_dir", help="directory to save data to", type=str, default="glue_data")
parser.add_argument(
"--tasks", help="tasks to download data for as a comma separated string", type=str, default="all"
)
parser.add_argument(
"--path_to_mrpc",
help="path to directory containing extracted MRPC data, msr_paraphrase_train.txt and msr_paraphrase_text.txt",
type=str,
default="",
)
args = parser.parse_args(arguments)
if not os.path.isdir(args.data_dir):
os.mkdir(args.data_dir)
tasks = get_tasks(args.tasks)
for task in tasks:
if task == "MRPC":
format_mrpc(args.data_dir, args.path_to_mrpc)
elif task == "diagnostic":
download_diagnostic(args.data_dir)
else:
download_and_extract(task, args.data_dir)
if __name__ == "__main__":
sys.exit(main(sys.argv[1:]))
| transformers/utils/download_glue_data.py/0 | {
"file_path": "transformers/utils/download_glue_data.py",
"repo_id": "transformers",
"token_count": 3917
} | 378 |
repos:
- repo: https://github.com/PyCQA/isort
rev: 5.12.0
hooks:
- id: isort
args:
- --profile=black
- --skip-glob=wandb/**/*
- --thirdparty=wandb
- repo: https://github.com/myint/autoflake
rev: v1.4
hooks:
- id: autoflake
args:
- -r
- --exclude=wandb,__init__.py
- --in-place
- --remove-unused-variables
- --remove-all-unused-imports
- repo: https://github.com/python/black
rev: 22.3.0
hooks:
- id: black
args:
- --line-length=119
- --target-version=py38
- --exclude=wandb
- repo: https://github.com/pycqa/flake8
rev: 6.0.0
hooks:
- id: flake8
args:
- --ignore=E203,E501,W503,E128
- --max-line-length=119
# - repo: https://github.com/codespell-project/codespell
# rev: v2.1.0
# hooks:
# - id: codespell
# args:
# - --ignore-words-list=nd,reacher,thist,ths,magent,ba
# - --skip=docs/css/termynal.css,docs/js/termynal.js
| trl/.pre-commit-config.yaml/0 | {
"file_path": "trl/.pre-commit-config.yaml",
"repo_id": "trl",
"token_count": 597
} | 379 |
#!/bin/bash
#SBATCH --job-name=trl
#SBATCH --partition=hopper-cpu
#SBATCH --ntasks=1
#SBATCH --output=slurm/logs/%x_%j.out
sleep 2m
bash $BENCHMARK_PLOT_SCRIPT
srun python benchmark/post_github_comment.py
| trl/benchmark/post_github_comment.sbatch/0 | {
"file_path": "trl/benchmark/post_github_comment.sbatch",
"repo_id": "trl",
"token_count": 90
} | 380 |
<div style="text-align: center">
<img src="https://huggingface.co/datasets/trl-internal-testing/example-images/resolve/main/images/trl_banner_dark.png">
</div>
# TRL - Transformer Reinforcement Learning
TRL is a full stack library where we provide a set of tools to train transformer language models with Reinforcement Learning, from the Supervised Fine-tuning step (SFT), Reward Modeling step (RM) to the Proximal Policy Optimization (PPO) step.
The library is integrated with 🤗 [transformers](https://github.com/huggingface/transformers).
<div style="text-align: center">
<img src="https://huggingface.co/datasets/trl-internal-testing/example-images/resolve/main/images/TRL-readme.png">
</div>
Check the appropriate sections of the documentation depending on your needs:
## API documentation
- [Model Classes](models): *A brief overview of what each public model class does.*
- [`SFTTrainer`](sft_trainer): *Supervise Fine-tune your model easily with `SFTTrainer`*
- [`RewardTrainer`](reward_trainer): *Train easily your reward model using `RewardTrainer`.*
- [`PPOTrainer`](ppo_trainer): *Further fine-tune the supervised fine-tuned model using PPO algorithm*
- [Best-of-N Sampling](best-of-n): *Use best of n sampling as an alternative way to sample predictions from your active model*
- [`DPOTrainer`](dpo_trainer): *Direct Preference Optimization training using `DPOTrainer`.*
- [`TextEnvironment`](text_environment): *Text environment to train your model using tools with RL.*
## Examples
- [Sentiment Tuning](sentiment_tuning): *Fine tune your model to generate positive movie contents*
- [Training with PEFT](lora_tuning_peft): *Memory efficient RLHF training using adapters with PEFT*
- [Detoxifying LLMs](detoxifying_a_lm): *Detoxify your language model through RLHF*
- [StackLlama](using_llama_models): *End-to-end RLHF training of a Llama model on Stack exchange dataset*
- [Learning with Tools](learning_tools): *Walkthrough of using `TextEnvironments`*
- [Multi-Adapter Training](multi_adapter_rl): *Use a single base model and multiple adapters for memory efficient end-to-end training*
## Blog posts
<div class="mt-10">
<div class="w-full flex flex-col space-y-4 md:space-y-0 md:grid md:grid-cols-2 md:gap-y-4 md:gap-x-5">
<a class="!no-underline border dark:border-gray-700 p-5 rounded-lg shadow hover:shadow-lg" href="https://huggingface.co/blog/rlhf">
<img src="https://raw.githubusercontent.com/huggingface/blog/main/assets/120_rlhf/thumbnail.png" alt="thumbnail">
<p class="text-gray-700">Illustrating Reinforcement Learning from Human Feedback</p>
</a>
<a class="!no-underline border dark:border-gray-700 p-5 rounded-lg shadow hover:shadow-lg" href="https://huggingface.co/blog/trl-peft">
<img src="https://github.com/huggingface/blog/blob/main/assets/133_trl_peft/thumbnail.png?raw=true" alt="thumbnail">
<p class="text-gray-700">Fine-tuning 20B LLMs with RLHF on a 24GB consumer GPU</p>
</a>
<a class="!no-underline border dark:border-gray-700 p-5 rounded-lg shadow hover:shadow-lg" href="https://huggingface.co/blog/stackllama">
<img src="https://github.com/huggingface/blog/blob/main/assets/138_stackllama/thumbnail.png?raw=true" alt="thumbnail">
<p class="text-gray-700">StackLLaMA: A hands-on guide to train LLaMA with RLHF</p>
</a>
<a class="!no-underline border dark:border-gray-700 p-5 rounded-lg shadow hover:shadow-lg" href="https://huggingface.co/blog/dpo-trl">
<img src="https://github.com/huggingface/blog/blob/main/assets/157_dpo_trl/dpo_thumbnail.png?raw=true" alt="thumbnail">
<p class="text-gray-700">Fine-tune Llama 2 with DPO</p>
</a>
<a class="!no-underline border dark:border-gray-700 p-5 rounded-lg shadow hover:shadow-lg" href="https://huggingface.co/blog/trl-ddpo">
<img src="https://github.com/huggingface/blog/blob/main/assets/166_trl_ddpo/thumbnail.png?raw=true" alt="thumbnail">
<p class="text-gray-700">Finetune Stable Diffusion Models with DDPO via TRL</p>
</a>
</div>
</div>
| trl/docs/source/index.mdx/0 | {
"file_path": "trl/docs/source/index.mdx",
"repo_id": "trl",
"token_count": 1414
} | 381 |
# Using LLaMA models with TRL
We've begun rolling out examples to use Meta's LLaMA models in `trl` (see [Meta's LLaMA release](https://ai.facebook.com/blog/large-language-model-llama-meta-ai/) for the original LLaMA model).
## Efficient training strategies
Even training the smallest LLaMA model requires an enormous amount of memory. Some quick math: in bf16, every parameter uses 2 bytes (in fp32 4 bytes) in addition to 8 bytes used, e.g., in the Adam optimizer (see the [performance docs](https://huggingface.co/docs/transformers/perf_train_gpu_one#optimizer) in Transformers for more info). So a 7B parameter model would use `(2+8)*7B=70GB` just to fit in memory and would likely need more when you compute intermediate values such as attention scores. So you couldn’t train the model even on a single 80GB A100 like that. You can use some tricks, like more efficient optimizers of half-precision training, to squeeze a bit more into memory, but you’ll run out sooner or later.
Another option is to use Parameter-Efficient Fine-Tuning (PEFT) techniques, such as the [`peft`](https://github.com/huggingface/peft) library, which can perform low-rank adaptation (LoRA) on a model loaded in 8-bit.
For more on `peft` + `trl`, see the [docs](https://huggingface.co/docs/trl/sentiment_tuning_peft).
Loading the model in 8bit reduces the memory footprint drastically since you only need one byte per parameter for the weights (e.g. 7B LlaMa is 7GB in memory).
Instead of training the original weights directly, LoRA adds small adapter layers on top of some specific layers (usually the attention layers); thus, the number of trainable parameters is drastically reduced.
In this scenario, a rule of thumb is to allocate ~1.2-1.4GB per billion parameters (depending on the batch size and sequence length) to fit the entire fine-tuning setup.
This enables fine-tuning larger models (up to 50-60B scale models on a NVIDIA A100 80GB) at low cost.
Now we can fit very large models into a single GPU, but the training might still be very slow.
The simplest strategy in this scenario is data parallelism: we replicate the same training setup into separate GPUs and pass different batches to each GPU.
With this, you can parallelize the forward/backward passes of the model and scale with the number of GPUs.
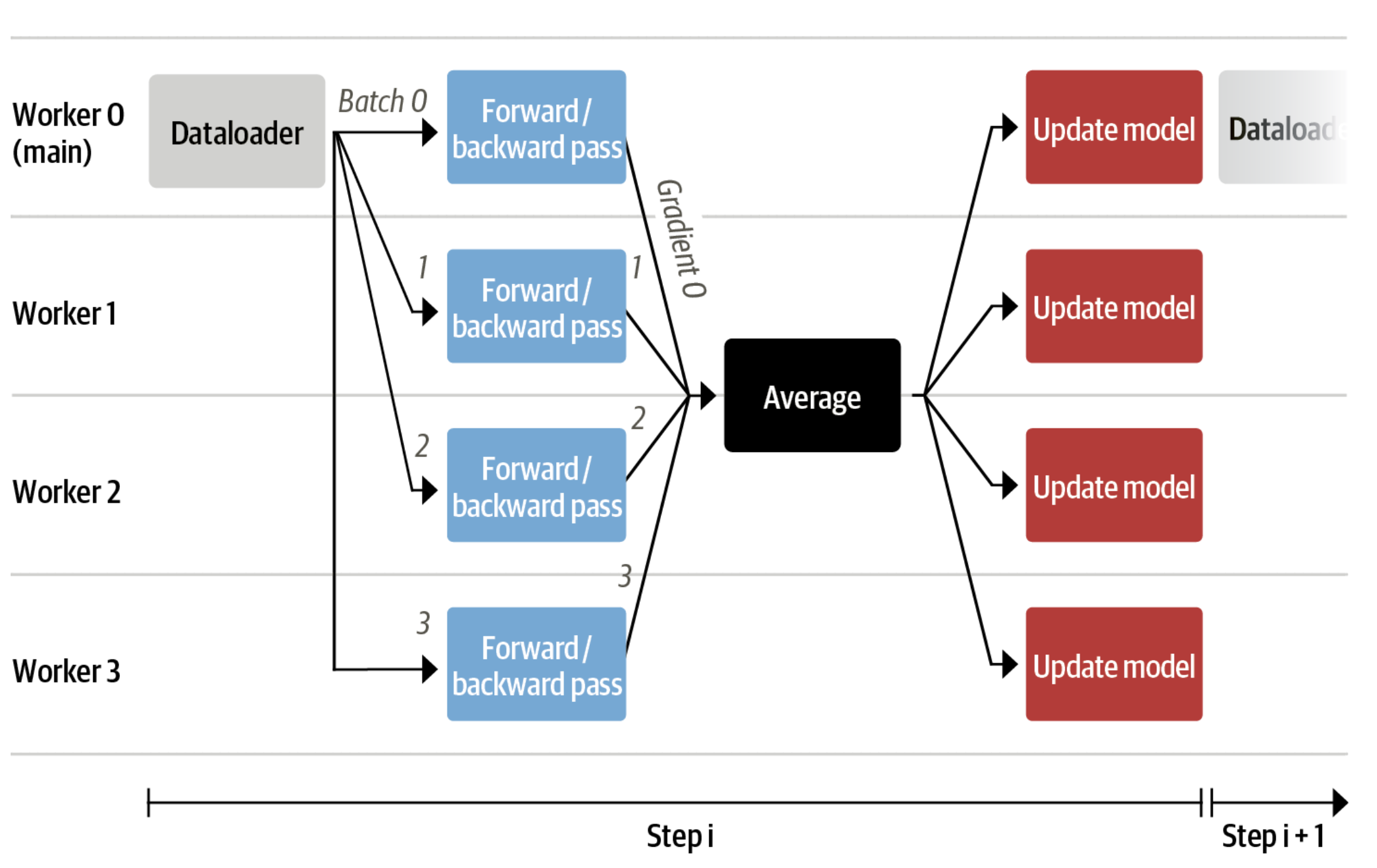
We use either the `transformers.Trainer` or `accelerate`, which both support data parallelism without any code changes, by simply passing arguments when calling the scripts with `torchrun` or `accelerate launch`. The following runs a training script with 8 GPUs on a single machine with `accelerate` and `torchrun`, respectively.
```bash
accelerate launch --multi_gpu --num_machines 1 --num_processes 8 my_accelerate_script.py
torchrun --nnodes 1 --nproc_per_node 8 my_torch_script.py
```
## Supervised fine-tuning
Before we start training reward models and tuning our model with RL, it helps if the model is already good in the domain we are interested in.
In our case, we want it to answer questions, while for other use cases, we might want it to follow instructions, in which case instruction tuning is a great idea.
The easiest way to achieve this is by continuing to train the language model with the language modeling objective on texts from the domain or task.
The [StackExchange dataset](https://huggingface.co/datasets/HuggingFaceH4/stack-exchange-preferences) is enormous (over 10 million instructions), so we can easily train the language model on a subset of it.
There is nothing special about fine-tuning the model before doing RLHF - it’s just the causal language modeling objective from pretraining that we apply here.
To use the data efficiently, we use a technique called packing: instead of having one text per sample in the batch and then padding to either the longest text or the maximal context of the model, we concatenate a lot of texts with a EOS token in between and cut chunks of the context size to fill the batch without any padding.
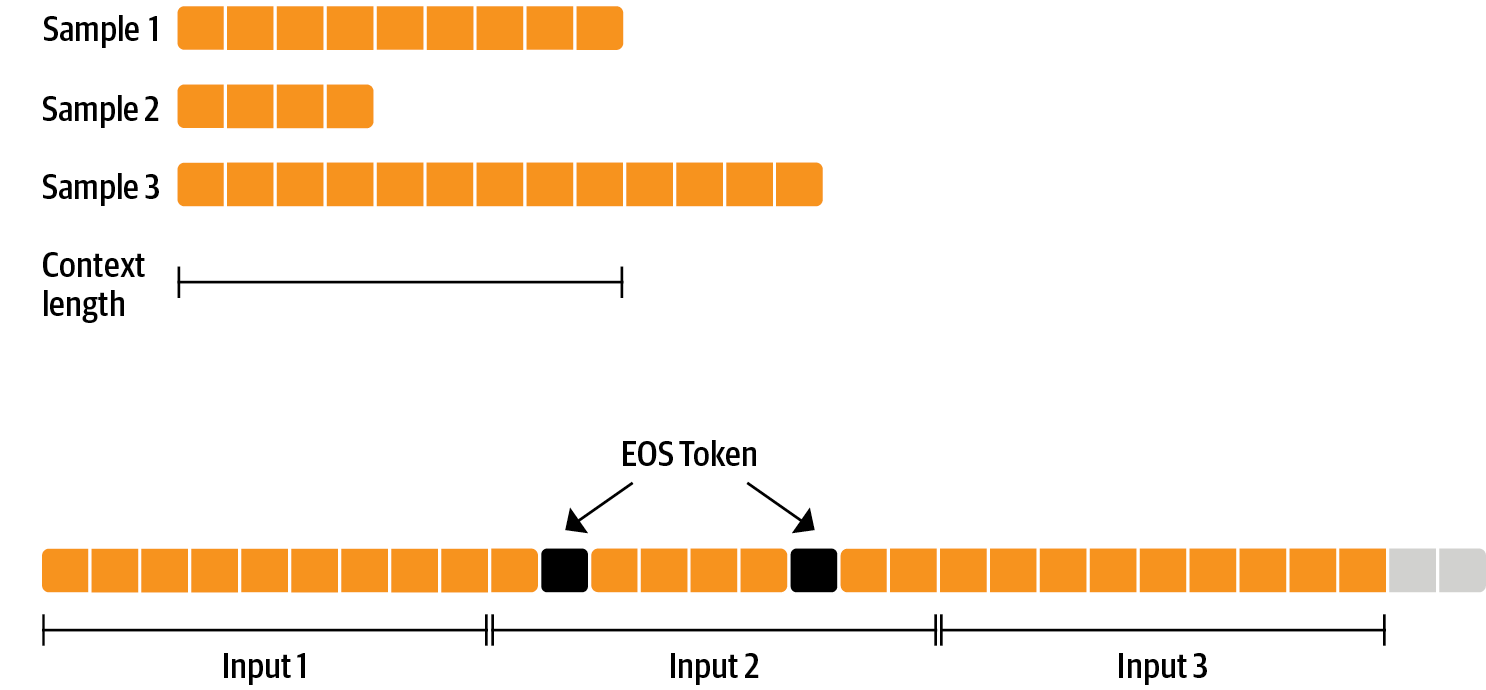
With this approach the training is much more efficient as each token that is passed through the model is also trained in contrast to padding tokens which are usually masked from the loss.
If you don't have much data and are more concerned about occasionally cutting off some tokens that are overflowing the context you can also use a classical data loader.
The packing is handled by the `ConstantLengthDataset` and we can then use the `Trainer` after loading the model with `peft`. First, we load the model in int8, prepare it for training, and then add the LoRA adapters.
```python
# load model in 8bit
model = AutoModelForCausalLM.from_pretrained(
args.model_path,
load_in_8bit=True,
device_map={"": Accelerator().local_process_index}
)
model = prepare_model_for_kbit_training(model)
# add LoRA to model
lora_config = LoraConfig(
r=16,
lora_alpha=32,
lora_dropout=0.05,
bias="none",
task_type="CAUSAL_LM",
)
model = get_peft_model(model, config)
```
We train the model for a few thousand steps with the causal language modeling objective and save the model.
Since we will tune the model again with different objectives, we merge the adapter weights with the original model weights.
**Disclaimer:** due to LLaMA's license, we release only the adapter weights for this and the model checkpoints in the following sections.
You can apply for access to the base model's weights by filling out Meta AI's [form](https://docs.google.com/forms/d/e/1FAIpQLSfqNECQnMkycAp2jP4Z9TFX0cGR4uf7b_fBxjY_OjhJILlKGA/viewform) and then converting them to the 🤗 Transformers format by running this [script](https://github.com/huggingface/transformers/blob/main/src/transformers/models/llama/convert_llama_weights_to_hf.py).
Note that you'll also need to install 🤗 Transformers from source until the `v4.28` is released.
Now that we have fine-tuned the model for the task, we are ready to train a reward model.
## Reward modeling and human preferences
In principle, we could fine-tune the model using RLHF directly with the human annotations.
However, this would require us to send some samples to humans for rating after each optimization iteration.
This is expensive and slow due to the number of training samples needed for convergence and the inherent latency of human reading and annotator speed.
A trick that works well instead of direct feedback is training a reward model on human annotations collected before the RL loop.
The goal of the reward model is to imitate how a human would rate a text. There are several possible strategies to build a reward model: the most straightforward way would be to predict the annotation (e.g. a rating score or a binary value for “good”/”bad”).
In practice, what works better is to predict the ranking of two examples, where the reward model is presented with two candidates `(y_k, y_j)` for a given prompt `x` and has to predict which one would be rated higher by a human annotator.
With the StackExchange dataset, we can infer which of the two answers was preferred by the users based on the score.
With that information and the loss defined above, we can then modify the `transformers.Trainer` by adding a custom loss function.
```python
class RewardTrainer(Trainer):
def compute_loss(self, model, inputs, return_outputs=False):
rewards_j = model(input_ids=inputs["input_ids_j"], attention_mask=inputs["attention_mask_j"])[0]
rewards_k = model(input_ids=inputs["input_ids_k"], attention_mask=inputs["attention_mask_k"])[0]
loss = -nn.functional.logsigmoid(rewards_j - rewards_k).mean()
if return_outputs:
return loss, {"rewards_j": rewards_j, "rewards_k": rewards_k}
return loss
```
We utilize a subset of a 100,000 pair of candidates and evaluate on a held-out set of 50,000. With a modest training batch size of 4, we train the Llama model using the LoRA `peft` adapter for a single epoch using the Adam optimizer with BF16 precision. Our LoRA configuration is:
```python
peft_config = LoraConfig(
task_type=TaskType.SEQ_CLS,
inference_mode=False,
r=8,
lora_alpha=32,
lora_dropout=0.1,
)
```
As detailed in the next section, the resulting adapter can be merged into the frozen model and saved for further downstream use.
## Reinforcement Learning from Human Feedback
With the fine-tuned language model and the reward model at hand, we are now ready to run the RL loop. It follows roughly three steps:
1. Generate responses from prompts,
2. Rate the responses with the reward model,
3. Run a reinforcement learning policy-optimization step with the ratings.
The Query and Response prompts are templated as follows before being tokenized and passed to the model:
```bash
Question: <Query>
Answer: <Response>
```
The same template was used for SFT, RM and RLHF stages.
Once more, we utilize `peft` for memory-efficient training, which offers an extra advantage in the RLHF context.
Here, the reference model and policy share the same base, the SFT model, which we load in 8-bit and freeze during training.
We exclusively optimize the policy's LoRA weights using PPO while sharing the base model's weights.
```python
for epoch, batch in tqdm(enumerate(ppo_trainer.dataloader)):
question_tensors = batch["input_ids"]
# sample from the policy and to generate responses
response_tensors = ppo_trainer.generate(
question_tensors,
return_prompt=False,
length_sampler=output_length_sampler,
**generation_kwargs,
)
batch["response"] = tokenizer.batch_decode(response_tensors, skip_special_tokens=True)
# Compute sentiment score
texts = [q + r for q, r in zip(batch["query"], batch["response"])]
pipe_outputs = sentiment_pipe(texts, **sent_kwargs)
rewards = [torch.tensor(output[0]["score"] - script_args.reward_baseline) for output in pipe_outputs]
# Run PPO step
stats = ppo_trainer.step(question_tensors, response_tensors, rewards)
# Log stats to Wandb
ppo_trainer.log_stats(stats, batch, rewards)
```
For the rest of the details and evaluation, please refer to our [blog post on StackLLaMA](https://huggingface.co/blog/stackllama).
| trl/docs/source/using_llama_models.mdx/0 | {
"file_path": "trl/docs/source/using_llama_models.mdx",
"repo_id": "trl",
"token_count": 2999
} | 382 |
# coding=utf-8
# Copyright 2022 The HuggingFace Inc. team. All rights reserved.
#
# Licensed under the Apache License, Version 2.0 (the "License");
# you may not use this file except in compliance with the License.
# You may obtain a copy of the License at
#
# http://www.apache.org/licenses/LICENSE-2.0
#
# Unless required by applicable law or agreed to in writing, software
# distributed under the License is distributed on an "AS IS" BASIS,
# WITHOUT WARRANTIES OR CONDITIONS OF ANY KIND, either express or implied.
# See the License for the specific language governing permissions and
# limitations under the License.
from dataclasses import dataclass, field
from typing import Optional
import torch
from accelerate import Accelerator
from datasets import load_dataset
from peft import LoraConfig
from tqdm import tqdm
from transformers import Adafactor, AutoTokenizer, HfArgumentParser, pipeline
from trl import AutoModelForCausalLMWithValueHead, PPOConfig, PPOTrainer, set_seed
from trl.core import LengthSampler
tqdm.pandas()
@dataclass
class ScriptArguments:
"""
The name of the Casual LM model we wish to fine-tune with PPO
"""
# NOTE: gpt2 models use Conv1D instead of Linear layers which are not yet supported in 8 bit mode
# models like gpt-neo* models are more suitable.
model_name: Optional[str] = field(default="", metadata={"help": "the model name"})
tokenizer_name: Optional[str] = field(default="", metadata={"help": "the tokenizer name"})
reward_model_name: Optional[str] = field(default="", metadata={"help": "the reward model name"})
log_with: Optional[str] = field(default=None, metadata={"help": "use 'wandb' to log with wandb"})
learning_rate: Optional[float] = field(default=1.41e-5, metadata={"help": "the learning rate"})
output_max_length: Optional[int] = field(default=128, metadata={"help": "maximum length for generation"})
mini_batch_size: Optional[int] = field(default=1, metadata={"help": "the PPO minibatch size"})
batch_size: Optional[int] = field(default=32, metadata={"help": "the batch size"})
ppo_epochs: Optional[int] = field(default=4, metadata={"help": "the number of ppo epochs"})
gradient_accumulation_steps: Optional[int] = field(
default=4, metadata={"help": "the number of gradient accumulation steps"}
)
adafactor: Optional[bool] = field(default=False, metadata={"help": "whether to use the adafactor optimizer"})
early_stopping: Optional[bool] = field(default=False, metadata={"help": "whether to early stop"})
target_kl: Optional[float] = field(default=0.1, metadata={"help": "kl target for early stopping"})
reward_baseline: Optional[float] = field(
default=0.0,
metadata={"help": "a baseline value that is subtracted from the reward"},
)
batched_gen: Optional[bool] = field(default=False, metadata={"help": "whether to use the batched text gen"})
save_freq: Optional[int] = field(default=None, metadata={"help": "n steps to save the model"})
output_dir: Optional[str] = field(default="runs/", metadata={"help": "n steps to save the model"})
seed: Optional[int] = field(default=0, metadata={"help": "the seed"})
steps: Optional[int] = field(default=20000, metadata={"help": "number of epochs"})
init_kl_coef: Optional[float] = field(
default=0.2,
metadata={"help": "Initial KL penalty coefficient (used for adaptive and linear control)"},
)
adap_kl_ctrl: Optional[bool] = field(default=True, metadata={"help": "Use adaptive KL control, otherwise linear"})
parser = HfArgumentParser(ScriptArguments)
script_args: ScriptArguments = parser.parse_args_into_dataclasses()[0]
reward_model_name = script_args.reward_model_name
dataset_name = "lvwerra/stack-exchange-paired"
config = PPOConfig(
steps=script_args.steps,
model_name=script_args.model_name,
learning_rate=script_args.learning_rate,
log_with=script_args.log_with,
batch_size=script_args.batch_size,
mini_batch_size=script_args.mini_batch_size,
gradient_accumulation_steps=script_args.gradient_accumulation_steps,
optimize_cuda_cache=True,
early_stopping=script_args.early_stopping,
target_kl=script_args.target_kl,
ppo_epochs=script_args.ppo_epochs,
seed=script_args.seed,
init_kl_coef=script_args.init_kl_coef,
adap_kl_ctrl=script_args.adap_kl_ctrl,
)
train_dataset = load_dataset("lvwerra/stack-exchange-paired", data_dir="data/rl", split="train")
train_dataset = train_dataset.select(range(100000))
original_columns = train_dataset.column_names
# We then define the arguments to pass to the sentiment analysis pipeline.
# We set `return_all_scores` to True to get the sentiment score for each token.
sent_kwargs = {
"return_all_scores": True,
"function_to_apply": "none",
"batch_size": 16,
"truncation": True,
}
tokenizer = AutoTokenizer.from_pretrained(script_args.tokenizer_name)
# GPT-2 tokenizer has a pad token, but it is not eos_token by default. We need to set it to eos_token.
# only for this model.
if getattr(tokenizer, "pad_token", None) is None:
tokenizer.pad_token = tokenizer.eos_token
# Below is an example function to build the dataset. In our case, we use the IMDB dataset
# from the `datasets` library. One should customize this function to train the model on
# its own dataset.
def build_dataset(
tokenizer,
dataset_name="lvwerra/stack-exchange-paired",
):
"""
Build dataset for training. This builds the dataset from `load_dataset`, one should
customize this function to train the model on its own dataset.
Args:
dataset_name (`str`):
The name of the dataset to be loaded.
Returns:
dataloader (`torch.utils.data.DataLoader`):
The dataloader for the dataset.
"""
num_proc = 24
def preprocess_function(examples):
new_examples = {
"query": [],
"input_ids": [],
}
for question in examples["question"]:
query = "Question: " + question + "\n\nAnswer: "
tokenized_question = tokenizer(query, truncation=True)
new_examples["query"].append(query)
new_examples["input_ids"].append(tokenized_question["input_ids"])
return new_examples
ds = train_dataset.map(
preprocess_function,
batched=True,
num_proc=num_proc,
remove_columns=original_columns,
)
ds = ds.filter(lambda x: len(x["input_ids"]) < 512, batched=False)
ds.set_format(type="torch")
return ds
# We retrieve the dataloader by calling the `build_dataset` function.
dataset = build_dataset(tokenizer)
def collator(data):
return dict((key, [d[key] for d in data]) for key in data[0])
# set seed before initializing value head for deterministic eval
set_seed(config.seed)
# Now let's build the model, the reference model, and the tokenizer.
current_device = Accelerator().local_process_index
lora_config = LoraConfig(
r=16,
lora_alpha=32,
lora_dropout=0.05,
bias="none",
task_type="CAUSAL_LM",
)
model = AutoModelForCausalLMWithValueHead.from_pretrained(
config.model_name,
load_in_8bit=True,
device_map={"": current_device},
peft_config=lora_config,
)
optimizer = None
if script_args.adafactor:
optimizer = Adafactor(
filter(lambda p: p.requires_grad, model.parameters()),
scale_parameter=False,
relative_step=False,
warmup_init=False,
lr=config.learning_rate,
)
# We then build the PPOTrainer, passing the model, the reference model, the tokenizer
ppo_trainer = PPOTrainer(
config,
model,
ref_model=None,
tokenizer=tokenizer,
dataset=dataset,
data_collator=collator,
optimizer=optimizer,
)
# We then build the sentiment analysis pipeline using our reward model, passing the
# model name and the sentiment analysis pipeline arguments. Let's also make sure to
# set the device to the same device as the PPOTrainer.
device = ppo_trainer.accelerator.device
if ppo_trainer.accelerator.num_processes == 1:
device = 0 if torch.cuda.is_available() else "cpu" # to avoid a ` pipeline` bug
sentiment_pipe = pipeline(
"sentiment-analysis",
model=reward_model_name,
device_map={"": current_device},
model_kwargs={"load_in_8bit": True},
tokenizer=tokenizer,
return_token_type_ids=False,
)
# We then define the arguments to pass to the `generate` function. These arguments
# are passed to the `generate` function of the PPOTrainer, which is a wrapper around
# the `generate` function of the trained model.
generation_kwargs = {
# "min_length": -1,
"top_k": 0.0,
"top_p": 1.0,
"do_sample": True,
"pad_token_id": tokenizer.pad_token_id,
"eos_token_id": 100_000,
}
output_min_length = 32
output_max_length = script_args.output_max_length
output_length_sampler = LengthSampler(output_min_length, output_max_length)
for epoch, batch in tqdm(enumerate(ppo_trainer.dataloader)):
if epoch >= config.total_ppo_epochs:
break
question_tensors = batch["input_ids"]
response_tensors = ppo_trainer.generate(
question_tensors,
return_prompt=False,
length_sampler=output_length_sampler,
**generation_kwargs,
)
batch["response"] = tokenizer.batch_decode(response_tensors, skip_special_tokens=True)
# Compute reward score (using the sentiment analysis pipeline)
texts = [q + r for q, r in zip(batch["query"], batch["response"])]
pipe_outputs = sentiment_pipe(texts, **sent_kwargs)
rewards = [torch.tensor(output[0]["score"] - script_args.reward_baseline) for output in pipe_outputs]
# Run PPO step
stats = ppo_trainer.step(question_tensors, response_tensors, rewards)
ppo_trainer.log_stats(stats, batch, rewards)
if script_args.save_freq and epoch and epoch % script_args.save_freq == 0:
ppo_trainer.save_pretrained(script_args.output_dir + f"step_{epoch}")
| trl/examples/research_projects/stack_llama/scripts/rl_training.py/0 | {
"file_path": "trl/examples/research_projects/stack_llama/scripts/rl_training.py",
"repo_id": "trl",
"token_count": 3635
} | 383 |
# coding=utf-8
# Copyright 2023 The HuggingFace Inc. team. All rights reserved.
#
# Licensed under the Apache License, Version 2.0 (the "License");
# you may not use this file except in compliance with the License.
# You may obtain a copy of the License at
#
# http://www.apache.org/licenses/LICENSE-2.0
#
# Unless required by applicable law or agreed to in writing, software
# distributed under the License is distributed on an "AS IS" BASIS,
# WITHOUT WARRANTIES OR CONDITIONS OF ANY KIND, either express or implied.
# See the License for the specific language governing permissions and
# limitations under the License.
"""
python examples/scripts/reward_modeling.py \
--model_name_or_path=facebook/opt-350m \
--output_dir="reward_modeling_anthropic_hh" \
--per_device_train_batch_size=64 \
--num_train_epochs=1 \
--gradient_accumulation_steps=16 \
--gradient_checkpointing=True \
--learning_rate=1.41e-5 \
--report_to="wandb" \
--remove_unused_columns=False \
--optim="adamw_torch" \
--logging_steps=10 \
--evaluation_strategy="steps" \
--max_length=512 \
"""
import torch
from datasets import load_dataset
from tqdm import tqdm
from transformers import AutoModelForSequenceClassification, AutoTokenizer, HfArgumentParser
from trl import ModelConfig, RewardConfig, RewardTrainer, get_kbit_device_map, get_peft_config, get_quantization_config
tqdm.pandas()
if __name__ == "__main__":
parser = HfArgumentParser((RewardConfig, ModelConfig))
reward_config, model_config = parser.parse_args_into_dataclasses()
reward_config.gradient_checkpointing_kwargs = dict(use_reentrant=False)
################
# Model & Tokenizer
################
torch_dtype = (
model_config.torch_dtype
if model_config.torch_dtype in ["auto", None]
else getattr(torch, model_config.torch_dtype)
)
quantization_config = get_quantization_config(model_config)
model_kwargs = dict(
revision=model_config.model_revision,
trust_remote_code=model_config.trust_remote_code,
device_map=get_kbit_device_map() if quantization_config is not None else None,
quantization_config=quantization_config,
)
tokenizer = AutoTokenizer.from_pretrained(model_config.model_name_or_path, use_fast=True)
model = AutoModelForSequenceClassification.from_pretrained(
model_config.model_name_or_path, num_labels=1, **model_kwargs
)
################
# Dataset
################
raw_datasets = load_dataset("Anthropic/hh-rlhf")
# Tokenize chosen/rejected pairs of inputs
# Adapt this section to your needs for custom datasets
def preprocess_function(examples):
new_examples = {
"input_ids_chosen": [],
"attention_mask_chosen": [],
"input_ids_rejected": [],
"attention_mask_rejected": [],
}
for chosen, rejected in zip(examples["chosen"], examples["rejected"]):
tokenized_chosen = tokenizer(chosen)
tokenized_rejected = tokenizer(rejected)
new_examples["input_ids_chosen"].append(tokenized_chosen["input_ids"])
new_examples["attention_mask_chosen"].append(tokenized_chosen["attention_mask"])
new_examples["input_ids_rejected"].append(tokenized_rejected["input_ids"])
new_examples["attention_mask_rejected"].append(tokenized_rejected["attention_mask"])
return new_examples
# Preprocess the dataset and filter out examples that are longer than args.max_length
raw_datasets = raw_datasets.map(
preprocess_function,
batched=True,
num_proc=4,
)
raw_datasets = raw_datasets.filter(
lambda x: len(x["input_ids_chosen"]) <= reward_config.max_length
and len(x["input_ids_rejected"]) <= reward_config.max_length
)
train_dataset = raw_datasets["train"]
eval_dataset = raw_datasets["test"]
################
# Training
################
trainer = RewardTrainer(
model=model,
tokenizer=tokenizer,
args=reward_config,
train_dataset=train_dataset,
eval_dataset=eval_dataset,
peft_config=get_peft_config(model_config),
)
trainer.train()
trainer.save_model(reward_config.output_dir)
| trl/examples/scripts/reward_modeling.py/0 | {
"file_path": "trl/examples/scripts/reward_modeling.py",
"repo_id": "trl",
"token_count": 1701
} | 384 |
# Copyright 2023 The HuggingFace Team. All rights reserved.
#
# Licensed under the Apache License, Version 2.0 (the "License");
# you may not use this file except in compliance with the License.
# You may obtain a copy of the License at
#
# http://www.apache.org/licenses/LICENSE-2.0
#
# Unless required by applicable law or agreed to in writing, software
# distributed under the License is distributed on an "AS IS" BASIS,
# WITHOUT WARRANTIES OR CONDITIONS OF ANY KIND, either express or implied.
# See the License for the specific language governing permissions and
# limitations under the License.
import unittest
import torch
from transformers import AutoTokenizer
from trl import DataCollatorForCompletionOnlyLM
class DataCollatorForCompletionOnlyLMTester(unittest.TestCase):
def test_data_collator_finds_response_template_llama2_tokenizer(self):
# this should ideally be tested with meta-llama/Llama-2-7b-hf
self.tokenizer = AutoTokenizer.from_pretrained("trl-internal-testing/dummy-GPT2-correct-vocab")
self.instruction = """### System: You are a helpful assistant.
### User: How much is 2+2?
### Assistant: 2+2 equals 4"""
self.instruction_template = "\n### User:"
self.response_template = "\n### Assistant:"
# GPT2Tokenizer: [198, 21017, 11787, 25] -> [21017, 11787, 25]
# Llama2Tokenizer: [29871, 13, 2277, 29937, 4911, 29901] -> [2277, 29937, 4911, 29901]
# Note: If this test is ever switched to Llama2Tokenizer, this should be double checked,
# and possibly switched back to [2:] instead of [1:].
# With GPT2Tokenizer, [1:] is correct - we want the 21017 token included, which is ###.
self.tokenized_instruction_w_context = self.tokenizer.encode(
self.instruction_template, add_special_tokens=False
)[1:]
# GPT2Tokenizer: [198, 21017, 15286, 25] -> [15286, 25]
# Llama2Tokenizer: [29871, 13, 2277, 29937, 4007, 22137, 29901] -> [2277, 29937, 4007, 22137, 29901]
self.tokenized_response_w_context = self.tokenizer.encode(self.response_template, add_special_tokens=False)[2:]
# Plain check on string
assert self.response_template in self.instruction
self.tokenized_instruction = self.tokenizer.encode(self.instruction, add_special_tokens=False)
# Test the fix for #598
# Pass already tokenized (w context) and truncated response_template so token_ids are like in the instruction + response
self.collator = DataCollatorForCompletionOnlyLM(self.tokenized_response_w_context, tokenizer=self.tokenizer)
self.collator.torch_call([self.tokenized_instruction])
# Test for PR #749
# Pass already tokenized (w context) instruction and response both so token_ids are like in the instruction + response
self.collator = DataCollatorForCompletionOnlyLM(
self.tokenized_response_w_context, self.tokenized_instruction_w_context, tokenizer=self.tokenizer
)
self.collator.torch_call([self.tokenized_instruction])
# Test for PR #1185
# We pass in a string where the first user template is different than the rest.
# Usually this would happen due to context-sensitive tokenization, but here we
# explicitly change the template to test the fix.
self.instruction = """## User: First instruction
### Assistant: First response
### User: Second instruction
### Assistant: Second response"""
self.tokenized_instruction = self.tokenizer.encode(self.instruction, add_special_tokens=False)
self.collator = DataCollatorForCompletionOnlyLM(
self.tokenized_response_w_context, self.tokenized_instruction_w_context, tokenizer=self.tokenizer
)
collator_output = self.collator.torch_call([self.tokenized_instruction])
collator_text = self.tokenizer.decode(
collator_output["labels"][torch.where(collator_output["labels"] != -100)]
)
expected_text = " First response\n\n Second response" ""
assert collator_text == expected_text
def test_data_collator_handling_of_long_sequences(self):
self.tokenizer = AutoTokenizer.from_pretrained("trl-internal-testing/dummy-GPT2-correct-vocab")
self.instruction = """### System: You are a helpful assistant.
### User: How much is 2+2? I'm asking because I'm not sure. And I'm not sure because I'm not good at math.
"""
self.response_template = "\n### Assistant:"
# check DataCollatorForCompletionOnlyLM using response template only
self.tokenized_instruction = self.tokenizer.encode(self.instruction, add_special_tokens=False)
self.collator = DataCollatorForCompletionOnlyLM(self.response_template, tokenizer=self.tokenizer)
encoded_instance = self.collator.torch_call([self.tokenized_instruction])
result = torch.all(encoded_instance["labels"] == -100)
assert result, "Not all values in the tensor are -100."
# check DataCollatorForCompletionOnlyLM using response template and instruction template
self.instruction_template = "\n### User:"
self.collator = DataCollatorForCompletionOnlyLM(
self.response_template, self.instruction_template, tokenizer=self.tokenizer
)
encoded_instance = self.collator.torch_call([self.tokenized_instruction])
result = torch.all(encoded_instance["labels"] == -100)
assert result, "Not all values in the tensor are -100."
| trl/tests/test_data_collator_completion_only.py/0 | {
"file_path": "trl/tests/test_data_collator_completion_only.py",
"repo_id": "trl",
"token_count": 1973
} | 385 |
# Copyright 2022 The HuggingFace Team. All rights reserved.
#
# Licensed under the Apache License, Version 2.0 (the "License");
# you may not use this file except in compliance with the License.
# You may obtain a copy of the License at
#
# http://www.apache.org/licenses/LICENSE-2.0
#
# Unless required by applicable law or agreed to in writing, software
# distributed under the License is distributed on an "AS IS" BASIS,
# WITHOUT WARRANTIES OR CONDITIONS OF ANY KIND, either express or implied.
# See the License for the specific language governing permissions and
# limitations under the License.
import gc
import random
import warnings
from contextlib import contextmanager
from typing import Dict, List, Optional, Tuple, Union
import numpy as np
import torch
import torch.nn as nn
import torch.nn.functional as F
from torch.nn.utils.rnn import pad_sequence
from transformers import top_k_top_p_filtering
from .import_utils import is_npu_available, is_xpu_available
try:
from collections.abc import Mapping
except ImportError:
from collections import Mapping
WANDB_PADDING = -1
def flatten_dict(nested: Dict, sep: str = "/") -> Dict:
"""Flatten dictionary and concatenate nested keys with separator."""
def recurse(nest: Dict, prefix: str, into: Dict) -> None:
for k, v in nest.items():
if sep in k:
raise ValueError(f"separator '{sep}' not allowed to be in key '{k}'")
if isinstance(v, Mapping):
recurse(v, prefix + k + sep, into)
else:
into[prefix + k] = v
flat = {}
recurse(nested, "", flat)
return flat
def convert_to_scalar(stats: Dict) -> Dict:
"""
Converts the stats from a flattened dict to single scalar dicts
"""
tensorboard_stats = {}
for k, v in stats.items():
# for tensorboard compatibility - arrays and tensors are ignored with tensorboard
# therefore we convert single element tensors to scalars
if (isinstance(v, torch.Tensor) or isinstance(v, np.ndarray)) and (
len(v.shape) == 0 or (len(v.shape) == 1 and v.shape[0] == 1)
):
v = v.item()
tensorboard_stats[k] = v
return tensorboard_stats
def stack_dicts(stats_dicts: List[Dict]) -> Dict:
"""Stack the values of a dict."""
results = dict()
for k in stats_dicts[0]:
stats_list = [torch.flatten(d[k]) for d in stats_dicts]
results[k] = pad_sequence(stats_list, batch_first=True, padding_value=WANDB_PADDING)
return results
def add_suffix(input_dict: Dict, suffix: str) -> Dict:
"""Add suffix to dict keys."""
return dict((k + suffix, v) for k, v in input_dict.items())
def pad_to_size(tensor: torch.Tensor, size: int, dim: int = 1, padding: int = 50256) -> torch.Tensor:
"""Pad tensor to size."""
t_size = tensor.size()[dim]
if t_size == size:
return tensor
else:
return torch.nn.functional.pad(tensor, (0, size - t_size), "constant", padding)
def logprobs_from_logits(logits: torch.Tensor, labels: torch.Tensor, gather: bool = True) -> torch.Tensor:
"""
See: https://github.com/pytorch/pytorch/issues/563#issuecomment-330103591
"""
logp = F.log_softmax(logits, dim=2)
if not gather:
return logp
logpy = torch.gather(logp, 2, labels.unsqueeze(2)).squeeze(-1)
return logpy
def whiten(values: torch.Tensor, shift_mean: bool = True) -> torch.Tensor:
"""Whiten values."""
mean, var = torch.mean(values), torch.var(values)
whitened = (values - mean) * torch.rsqrt(var + 1e-8)
if not shift_mean:
whitened += mean
return whitened
def masked_mean(values: torch.Tensor, mask: torch.Tensor, axis: Optional[bool] = None) -> torch.Tensor:
"""Compute mean of tensor with a masked values."""
if axis is not None:
return (values * mask).sum(axis=axis) / mask.sum(axis=axis)
else:
return (values * mask).sum() / mask.sum()
def masked_var(values: torch.Tensor, mask: torch.Tensor, unbiased: bool = True) -> torch.Tensor:
"""Compute variance of tensor with masked values."""
mean = masked_mean(values, mask)
centered_values = values - mean
variance = masked_mean(centered_values**2, mask)
if unbiased:
mask_sum = mask.sum()
if mask_sum == 0:
raise ValueError(
"The sum of the mask is zero, which can happen when `mini_batch_size=1`;"
"try increase the `mini_batch_size` or `gradient_accumulation_steps`"
)
# note that if mask_sum == 1, then there is a division by zero issue
# to avoid it you just need to use a larger minibatch_size
bessel_correction = mask_sum / (mask_sum - 1)
variance = variance * bessel_correction
return variance
def masked_whiten(values: torch.Tensor, mask: torch.Tensor, shift_mean: bool = True) -> torch.Tensor:
"""Whiten values with masked values."""
mean, var = masked_mean(values, mask), masked_var(values, mask)
whitened = (values - mean) * torch.rsqrt(var + 1e-8)
if not shift_mean:
whitened += mean
return whitened
def clip_by_value(x: torch.Tensor, tensor_min: float, tensor_max: float) -> torch.Tensor:
"""
Tensor extension to torch.clamp
https://github.com/pytorch/pytorch/issues/2793#issuecomment-428784713
"""
clipped = torch.max(torch.min(x, tensor_max), tensor_min)
return clipped
def entropy_from_logits(logits: torch.Tensor) -> torch.Tensor:
"""Calculate entropy from logits."""
pd = torch.nn.functional.softmax(logits, dim=-1)
entropy = torch.logsumexp(logits, axis=-1) - torch.sum(pd * logits, axis=-1)
return entropy
def average_torch_dicts(list_of_dicts: List[Dict]) -> Dict:
"""Average values of a list of dicts with torch tensors."""
average_dict = dict()
for key in list_of_dicts[0].keys():
average_dict[key] = torch.mean(torch.stack([d[key] for d in list_of_dicts]), axis=0)
return average_dict
def stats_to_np(stats_dict: Dict) -> Dict:
"""Cast all torch.tensors in dict to numpy arrays."""
new_dict = dict()
for k, v in stats_dict.items():
if isinstance(v, torch.Tensor):
new_dict[k] = v.detach().cpu()
if new_dict[k].dtype == torch.bfloat16:
new_dict[k] = new_dict[k].float()
new_dict[k] = new_dict[k].numpy()
else:
new_dict[k] = v
if np.isscalar(new_dict[k]):
new_dict[k] = float(new_dict[k])
return new_dict
def respond_to_batch(
model: nn.Module, queries: List[torch.LongTensor], txt_len: int = 20, top_k: int = 0, top_p: float = 1.0
) -> torch.LongTensor:
"""Sample text from language model."""
input_ids = queries
for i in range(txt_len):
# Get Logits
outputs = model(input_ids)
next_token_logits = outputs[0][:, -1, :]
next_token_logits = top_k_top_p_filtering(next_token_logits, top_k=top_k, top_p=top_p)
# Sample
probs = F.softmax(next_token_logits, dim=-1)
next_token = torch.multinomial(probs, num_samples=1).squeeze(1)
input_ids = torch.cat([input_ids, next_token.unsqueeze(-1)], dim=-1)
return input_ids[:, -txt_len:]
def set_seed(seed: int) -> None:
"""
Helper function for reproducible behavior to set the seed in `random`, `numpy`, and `torch`.
Args:
seed (`int`): The seed to set.
"""
random.seed(seed)
np.random.seed(seed)
torch.manual_seed(seed)
if is_xpu_available():
torch.xpu.manual_seed_all(seed)
elif is_npu_available():
torch.npu.manual_seed_all(seed)
else:
torch.cuda.manual_seed_all(seed)
class LengthSampler:
"""
Samples a length
"""
def __init__(self, min_value: int, max_value: int):
self.values = list(range(min_value, max_value))
def __call__(self) -> int:
return np.random.choice(self.values)
class PPODecorators(object):
optimize_device_cache = False
@classmethod
@contextmanager
def empty_device_cache(cls):
yield
if cls.optimize_device_cache:
if is_xpu_available():
gc.collect()
torch.xpu.empty_cache()
gc.collect()
elif is_npu_available():
gc.collect()
torch.npu.empty_cache()
gc.collect()
elif torch.cuda.is_available():
gc.collect()
torch.cuda.empty_cache()
gc.collect()
def randn_tensor(
shape: Union[Tuple, List],
generator: Optional[Union[List[torch.Generator], torch.Generator]] = None,
device: Optional[torch.device] = None,
dtype: Optional[torch.dtype] = None,
layout: Optional[torch.layout] = None,
) -> torch.Tensor:
"""A helper function to create random tensors on the desired `device` with the desired `dtype`. When
passing a list of generators, you can seed each batch size individually. If CPU generators are passed, the tensor
is always created on the CPU.
"""
# device on which tensor is created defaults to device
rand_device = device
batch_size = shape[0]
layout = layout or torch.strided
device = device or torch.device("cpu")
if generator is not None:
gen_device_type = generator.device.type if not isinstance(generator, list) else generator[0].device.type
if gen_device_type != device.type and gen_device_type == "cpu":
rand_device = "cpu"
if device != "mps":
warnings.warn(
f"The passed generator was created on 'cpu' even though a tensor on {device} was expected."
f" Tensors will be created on 'cpu' and then moved to {device}. Note that one can probably"
f" slighly speed up this function by passing a generator that was created on the {device} device."
)
elif gen_device_type != device.type and gen_device_type == "cuda":
raise ValueError(f"Cannot generate a {device} tensor from a generator of type {gen_device_type}.")
# make sure generator list of length 1 is treated like a non-list
if isinstance(generator, list) and len(generator) == 1:
generator = generator[0]
if isinstance(generator, list):
shape = (1,) + shape[1:]
latents = [
torch.randn(shape, generator=generator[i], device=rand_device, dtype=dtype, layout=layout)
for i in range(batch_size)
]
latents = torch.cat(latents, dim=0).to(device)
else:
latents = torch.randn(shape, generator=generator, device=rand_device, dtype=dtype, layout=layout).to(device)
return latents
| trl/trl/core.py/0 | {
"file_path": "trl/trl/core.py",
"repo_id": "trl",
"token_count": 4437
} | 386 |
# DPO Authors: Rafael Rafailov, Archit Sharma, Eric Mitchell, Stefano Ermon, Christopher D. Manning, and Chelsea Finn 2023
# Copyright 2023 The HuggingFace Team. All rights reserved.
#
# Licensed under the Apache License, Version 2.0 (the "License");
# you may not use this file except in compliance with the License.
# You may obtain a copy of the License at
#
# http://www.apache.org/licenses/LICENSE-2.0
#
# Unless required by applicable law or agreed to in writing, software
# distributed under the License is distributed on an "AS IS" BASIS,
# WITHOUT WARRANTIES OR CONDITIONS OF ANY KIND, either express or implied.
# See the License for the specific language governing permissions and
# limitations under the License.
import inspect
import random
import warnings
from collections import defaultdict
from contextlib import contextmanager, nullcontext
from copy import deepcopy
from functools import wraps
from typing import Any, Callable, Dict, List, Literal, Optional, Tuple, Union
import numpy as np
import torch
import torch.nn as nn
import torch.nn.functional as F
from accelerate import PartialState
from accelerate.utils import is_deepspeed_available, tqdm
from datasets import Dataset
from torch.utils.data import DataLoader
from transformers import (
AutoModelForCausalLM,
DataCollator,
PreTrainedModel,
PreTrainedTokenizerBase,
Trainer,
TrainingArguments,
)
from transformers.trainer_callback import TrainerCallback
from transformers.trainer_utils import EvalLoopOutput
from ..import_utils import is_peft_available, is_wandb_available
from ..models import PreTrainedModelWrapper, create_reference_model
from .utils import (
DPODataCollatorWithPadding,
disable_dropout_in_model,
pad_to_length,
peft_module_casting_to_bf16,
trl_sanitze_kwargs_for_tagging,
)
if is_peft_available():
from peft import PeftModel, get_peft_model, prepare_model_for_kbit_training
if is_wandb_available():
import wandb
if is_deepspeed_available():
import deepspeed
class DPOTrainer(Trainer):
r"""
Initialize DPOTrainer.
Args:
model (`transformers.PreTrainedModel`):
The model to train, preferably an `AutoModelForSequenceClassification`.
ref_model (`PreTrainedModelWrapper`):
Hugging Face transformer model with a casual language modelling head. Used for implicit reward computation and loss. If no
reference model is provided, the trainer will create a reference model with the same architecture as the model to be optimized.
beta (`float`, defaults to 0.1):
The beta factor in DPO loss. Higher beta means less divergence from the initial policy. For the IPO loss, beta is the regularization parameter denoted by tau in the paper.
label_smoothing (`float`, defaults to 0):
The robust DPO label smoothing parameter from the [cDPO](https://ericmitchell.ai/cdpo.pdf) report that should be between 0 and 0.5.
loss_type (`str`, defaults to `"sigmoid"`):
The type of DPO loss to use. Either `"sigmoid"` the default DPO loss,`"hinge"` loss from [SLiC](https://arxiv.org/abs/2305.10425) paper, `"ipo"` from [IPO](https://arxiv.org/abs/2310.12036) paper, or `"kto"` from the HALOs [report](https://github.com/ContextualAI/HALOs/blob/main/assets/report.pdf).
args (`transformers.TrainingArguments`):
The arguments to use for training.
data_collator (`transformers.DataCollator`):
The data collator to use for training. If None is specified, the default data collator (`DPODataCollatorWithPadding`) will be used
which will pad the sequences to the maximum length of the sequences in the batch, given a dataset of paired sequences.
label_pad_token_id (`int`, defaults to `-100`):
The label pad token id. This argument is required if you want to use the default data collator.
padding_value (`int`, defaults to `0`):
The padding value if it is different to the tokenizer's pad_token_id.
truncation_mode (`str`, defaults to `keep_end`):
The truncation mode to use, either `keep_end` or `keep_start`. This argument is required if you want to use the default data collator.
train_dataset (`datasets.Dataset`):
The dataset to use for training.
eval_dataset (`datasets.Dataset`):
The dataset to use for evaluation.
tokenizer (`transformers.PreTrainedTokenizerBase`):
The tokenizer to use for training. This argument is required if you want to use the default data collator.
model_init (`Callable[[], transformers.PreTrainedModel]`):
The model initializer to use for training. If None is specified, the default model initializer will be used.
callbacks (`List[transformers.TrainerCallback]`):
The callbacks to use for training.
optimizers (`Tuple[torch.optim.Optimizer, torch.optim.lr_scheduler.LambdaLR]`):
The optimizer and scheduler to use for training.
preprocess_logits_for_metrics (`Callable[[torch.Tensor, torch.Tensor], torch.Tensor]`):
The function to use to preprocess the logits before computing the metrics.
max_length (`int`, defaults to `None`):
The maximum length of the sequences in the batch. This argument is required if you want to use the default data collator.
max_prompt_length (`int`, defaults to `None`):
The maximum length of the prompt. This argument is required if you want to use the default data collator.
max_target_length (`int`, defaults to `None`):
The maximum length of the target. This argument is required if you want to use the default data collator and your model is an encoder-decoder.
peft_config (`Dict`, defaults to `None`):
The PEFT configuration to use for training. If you pass a PEFT configuration, the model will be wrapped in a PEFT model.
is_encoder_decoder (`Optional[bool]`, `optional`, defaults to `None`):
If no model is provided, we need to know if the model_init returns an encoder-decoder.
disable_dropout (`bool`, defaults to `True`):
Whether or not to disable dropouts in `model` and `ref_model`.
generate_during_eval (`bool`, defaults to `False`):
Whether to sample and log generations during evaluation step.
compute_metrics (`Callable[[EvalPrediction], Dict]`, *optional*):
The function to use to compute the metrics. Must take a `EvalPrediction` and return
a dictionary string to metric values.
precompute_ref_log_probs (`bool`, defaults to `False`):
Flag to precompute reference model log probabilities and evaluation datasets. This is useful if you want to train
without the reference model and reduce the total GPU memory needed.
dataset_num_proc (`Optional[int]`, *optional*):
The number of workers to use to tokenize the data. Defaults to None.
model_init_kwargs (`Optional[Dict]`, *optional*):
Dict of Optional kwargs to pass when instantiating the model from a string
ref_model_init_kwargs (`Optional[Dict]`, *optional*):
Dict of Optional kwargs to pass when instantiating the ref model from a string
model_adapter_name (`str`, defaults to `None`):
Name of the train target PEFT adapter, when using LoRA with multiple adapters.
ref_adapter_name (`str`, defaults to `None`):
Name of the reference PEFT adapter, when using LoRA with multiple adapters.
reference_free (`bool`):
If True, we ignore the _provided_ reference model and implicitly use a reference model that assigns equal probability to all responses.
"""
_tag_names = ["trl", "dpo"]
def __init__(
self,
model: Optional[Union[PreTrainedModel, nn.Module, str]] = None,
ref_model: Optional[Union[PreTrainedModel, nn.Module, str]] = None,
beta: float = 0.1,
label_smoothing: float = 0,
loss_type: Literal["sigmoid", "hinge", "ipo", "kto_pair"] = "sigmoid",
args: Optional[TrainingArguments] = None,
data_collator: Optional[DataCollator] = None,
label_pad_token_id: int = -100,
padding_value: Optional[int] = None,
truncation_mode: str = "keep_end",
train_dataset: Optional[Dataset] = None,
eval_dataset: Optional[Union[Dataset, Dict[str, Dataset]]] = None,
tokenizer: Optional[PreTrainedTokenizerBase] = None,
model_init: Optional[Callable[[], PreTrainedModel]] = None,
callbacks: Optional[List[TrainerCallback]] = None,
optimizers: Tuple[torch.optim.Optimizer, torch.optim.lr_scheduler.LambdaLR] = (None, None),
preprocess_logits_for_metrics: Optional[Callable[[torch.Tensor, torch.Tensor], torch.Tensor]] = None,
max_length: Optional[int] = None,
max_prompt_length: Optional[int] = None,
max_target_length: Optional[int] = None,
peft_config: Optional[Dict] = None,
is_encoder_decoder: Optional[bool] = None,
disable_dropout: bool = True,
generate_during_eval: bool = False,
compute_metrics: Optional[Callable[[EvalLoopOutput], Dict]] = None,
precompute_ref_log_probs: bool = False,
dataset_num_proc: Optional[int] = None,
model_init_kwargs: Optional[Dict] = None,
ref_model_init_kwargs: Optional[Dict] = None,
model_adapter_name: Optional[str] = None,
ref_adapter_name: Optional[str] = None,
reference_free: bool = False,
):
if model_init_kwargs is None:
model_init_kwargs = {}
elif not isinstance(model, str):
raise ValueError("You passed model_kwargs to the DPOTrainer. But your model is already instantiated.")
if ref_model_init_kwargs is None:
ref_model_init_kwargs = {}
elif not isinstance(ref_model, str):
raise ValueError(
"You passed ref_model_kwargs to the DPOTrainer. But your ref_model is already instantiated."
)
if isinstance(model, str):
warnings.warn(
"You passed a model_id to the DPOTrainer. This will automatically create an "
"`AutoModelForCausalLM` or a `PeftModel` (if you passed a `peft_config`) for you."
)
model = AutoModelForCausalLM.from_pretrained(model, **model_init_kwargs)
if isinstance(ref_model, str):
warnings.warn(
"You passed a ref model_id to the DPOTrainer. This will automatically create an "
"`AutoModelForCausalLM`"
)
ref_model = AutoModelForCausalLM.from_pretrained(ref_model, **ref_model_init_kwargs)
# Initialize this variable to False. This helps tracking the case when `peft_module_casting_to_bf16`
# has been called in order to properly call autocast if needed.
self._peft_has_been_casted_to_bf16 = False
if not is_peft_available() and peft_config is not None:
raise ValueError(
"PEFT is not installed and you passed a `peft_config` in the trainer's kwargs, please install it to use the PEFT models"
)
elif is_peft_available() and peft_config is not None:
# if model is a peft model and we have a peft_config, we merge and unload it first
if isinstance(model, PeftModel):
model = model.merge_and_unload()
if ref_model is not None:
raise ValueError(
"You passed both a ref_model and a peft_config. For training PEFT adapters with DPO there is no need to pass a reference"
" model. Please pass `ref_model=None` in case you want to train PEFT adapters."
)
if getattr(model, "is_loaded_in_8bit", False) or getattr(model, "is_loaded_in_4bit", False):
_support_gc_kwargs = hasattr(
args, "gradient_checkpointing_kwargs"
) and "gradient_checkpointing_kwargs" in list(
inspect.signature(prepare_model_for_kbit_training).parameters
)
preprare_model_kwargs = {"use_gradient_checkpointing": args.gradient_checkpointing}
if _support_gc_kwargs:
preprare_model_kwargs["gradient_checkpointing_kwargs"] = args.gradient_checkpointing_kwargs
model = prepare_model_for_kbit_training(model, **preprare_model_kwargs)
elif getattr(args, "gradient_checkpointing", False):
# For backward compatibility with older versions of transformers
if hasattr(model, "enable_input_require_grads"):
model.enable_input_require_grads()
else:
def make_inputs_require_grad(module, input, output):
output.requires_grad_(True)
model.get_input_embeddings().register_forward_hook(make_inputs_require_grad)
# get peft model with the given config
model = get_peft_model(model, peft_config)
if args.bf16 and getattr(model, "is_loaded_in_4bit", False):
peft_module_casting_to_bf16(model)
# If args.bf16 we need to explicitly call `generate` with torch amp autocast context manager
self._peft_has_been_casted_to_bf16 = True
# For models that use gradient_checkpoiting, we need to attach a hook that enables input
# to explicitly have `requires_grad=True`, otherwise training will either silently
# fail or completely fail.
elif getattr(args, "gradient_checkpointing", False):
# For backward compatibility with older versions of transformers
if hasattr(model, "enable_input_require_grads"):
model.enable_input_require_grads()
else:
def make_inputs_require_grad(module, input, output):
output.requires_grad_(True)
model.get_input_embeddings().register_forward_hook(make_inputs_require_grad)
if generate_during_eval and not is_wandb_available():
raise ValueError(
"`generate_during_eval=True` requires Weights and Biases to be installed."
" Please install `wandb` to resolve."
)
if model is not None:
self.is_encoder_decoder = model.config.is_encoder_decoder
elif is_encoder_decoder is None:
raise ValueError("When no model is provided, you need to pass the parameter is_encoder_decoder.")
else:
self.is_encoder_decoder = is_encoder_decoder
self.is_peft_model = is_peft_available() and isinstance(model, PeftModel)
self.model_adapter_name = model_adapter_name
self.ref_adapter_name = ref_adapter_name
self.reference_free = reference_free
if ref_model:
self.ref_model = ref_model
elif self.is_peft_model or precompute_ref_log_probs:
# The `model` with adapters turned off will be used as the reference model
self.ref_model = None
else:
self.ref_model = create_reference_model(model)
if tokenizer is None:
raise ValueError("tokenizer must be specified to tokenize a DPO dataset.")
if max_length is None:
warnings.warn(
"`max_length` is not set in the DPOTrainer's init"
" it will default to `512` by default, but you should do it yourself in the future.",
UserWarning,
)
max_length = 512
if max_prompt_length is None:
warnings.warn(
"`max_prompt_length` is not set in the DPOTrainer's init"
" it will default to `128` by default, but you should do it yourself in the future.",
UserWarning,
)
max_prompt_length = 128
if max_target_length is None and self.is_encoder_decoder:
warnings.warn(
"When using an encoder decoder architecture, you should set `max_target_length` in the DPOTrainer's init"
" it will default to `128` by default, but you should do it yourself in the future.",
UserWarning,
)
max_target_length = 128
if data_collator is None:
data_collator = DPODataCollatorWithPadding(
pad_token_id=tokenizer.pad_token_id,
label_pad_token_id=label_pad_token_id,
is_encoder_decoder=self.is_encoder_decoder,
)
if args.remove_unused_columns:
args.remove_unused_columns = False
# warn users
warnings.warn(
"When using DPODataCollatorWithPadding, you should set `remove_unused_columns=False` in your TrainingArguments"
" we have set it for you, but you should do it yourself in the future.",
UserWarning,
)
self.use_dpo_data_collator = True
else:
self.use_dpo_data_collator = False
if disable_dropout:
disable_dropout_in_model(model)
if self.ref_model is not None:
disable_dropout_in_model(self.ref_model)
self.max_length = max_length
self.generate_during_eval = generate_during_eval
self.label_pad_token_id = label_pad_token_id
self.padding_value = padding_value if padding_value is not None else tokenizer.pad_token_id
self.max_prompt_length = max_prompt_length
self.truncation_mode = truncation_mode
self.max_target_length = max_target_length
self.tokenizer = tokenizer
self.precompute_ref_log_probs = precompute_ref_log_probs
# Since ref_logs are precomputed on the first call to get_train/eval_dataloader
# keep track of first called to avoid computation of future calls
self._precomputed_train_ref_log_probs = False
self._precomputed_eval_ref_log_probs = False
if loss_type in ["hinge", "ipo", "kto_pair"] and label_smoothing > 0:
warnings.warn(
"You are using a loss type that does not support label smoothing. Ignoring label_smoothing parameter."
)
self.beta = beta
self.label_smoothing = label_smoothing
self.loss_type = loss_type
self._stored_metrics = defaultdict(lambda: defaultdict(list))
self.dataset_num_proc = dataset_num_proc
# Compute that only on the main process for faster data processing.
# see: https://github.com/huggingface/trl/pull/1255
with PartialState().local_main_process_first():
# tokenize the dataset
train_dataset = train_dataset.map(self.tokenize_row, num_proc=self.dataset_num_proc)
if eval_dataset is not None:
eval_dataset = eval_dataset.map(self.tokenize_row, num_proc=self.dataset_num_proc)
super().__init__(
model=model,
args=args,
data_collator=data_collator,
train_dataset=train_dataset,
eval_dataset=eval_dataset,
tokenizer=tokenizer,
model_init=model_init,
compute_metrics=compute_metrics,
callbacks=callbacks,
optimizers=optimizers,
preprocess_logits_for_metrics=preprocess_logits_for_metrics,
)
if not hasattr(self, "accelerator"):
raise AttributeError(
"Your `Trainer` does not have an `accelerator` object. Consider upgrading `transformers`."
)
# Deepspeed Zero-3 does not support precompute_ref_log_probs
if self.is_deepspeed_enabled:
if self.accelerator.state.deepspeed_plugin.zero_stage == 3 and self.precompute_ref_log_probs:
raise ValueError(
"You cannot use `precompute_ref_log_probs=True` with Deepspeed ZeRO-3. Please set `precompute_ref_log_probs=False`."
)
if self.ref_model is None:
if not (self.is_peft_model or self.precompute_ref_log_probs):
raise ValueError(
"No reference model and model is not a Peft model. Try setting `precompute_ref_log_probs=True`"
)
else:
if self.is_deepspeed_enabled:
self.ref_model = self._prepare_deepspeed(self.ref_model)
else:
self.ref_model = self.accelerator.prepare_model(self.ref_model, evaluation_mode=True)
def _prepare_deepspeed(self, model: PreTrainedModelWrapper):
# Adapted from accelerate: https://github.com/huggingface/accelerate/blob/739b135f8367becb67ffaada12fe76e3aa60fefd/src/accelerate/accelerator.py#L1473
deepspeed_plugin = self.accelerator.state.deepspeed_plugin
config_kwargs = deepcopy(deepspeed_plugin.deepspeed_config)
if model is not None:
if hasattr(model, "config"):
hidden_size = (
max(model.config.hidden_sizes)
if getattr(model.config, "hidden_sizes", None)
else getattr(model.config, "hidden_size", None)
)
if hidden_size is not None and config_kwargs["zero_optimization"]["stage"] == 3:
# Note that `stage3_prefetch_bucket_size` can produce DeepSpeed messages like: `Invalidate trace cache @ step 0: expected module 1, but got module 0`
# This is expected and is not an error, see: https://github.com/microsoft/DeepSpeed/discussions/4081
config_kwargs.update(
{
"zero_optimization.reduce_bucket_size": hidden_size * hidden_size,
"zero_optimization.stage3_param_persistence_threshold": 10 * hidden_size,
"zero_optimization.stage3_prefetch_bucket_size": 0.9 * hidden_size * hidden_size,
}
)
# If ZeRO-3 is used, we shard both the active and reference model.
# Otherwise, we assume the reference model fits in memory and is initialized on each device with ZeRO disabled (stage 0)
if config_kwargs["zero_optimization"]["stage"] != 3:
config_kwargs["zero_optimization"]["stage"] = 0
model, *_ = deepspeed.initialize(model=model, config=config_kwargs)
model.eval()
return model
def get_train_dataloader(self) -> DataLoader:
"""
Returns the training [`~torch.utils.data.DataLoader`].
Subclass of transformers.src.transformers.trainer.get_train_dataloader to precompute `ref_log_probs`.
"""
if self.precompute_ref_log_probs and not self._precomputed_train_ref_log_probs:
dataloader_params = {
"batch_size": self.args.per_device_train_batch_size,
"collate_fn": self.data_collator,
"num_workers": self.args.dataloader_num_workers,
"pin_memory": self.args.dataloader_pin_memory,
"shuffle": False,
}
# prepare dataloader
data_loader = self.accelerator.prepare(DataLoader(self.train_dataset, **dataloader_params))
reference_chosen_logps = []
reference_rejected_logps = []
for padded_batch in tqdm(iterable=data_loader, desc="Train dataset reference log probs"):
reference_chosen_logp, reference_rejected_logp = self.compute_reference_log_probs(padded_batch)
reference_chosen_logp, reference_rejected_logp = self.accelerator.gather_for_metrics(
(reference_chosen_logp, reference_rejected_logp)
)
reference_chosen_logps.append(reference_chosen_logp.cpu())
reference_rejected_logps.append(reference_rejected_logp.cpu())
all_reference_chosen_logps = torch.cat(reference_chosen_logps).float().numpy()
all_reference_rejected_logps = torch.cat(reference_rejected_logps).float().numpy()
self.train_dataset = self.train_dataset.add_column(
name="reference_chosen_logps", column=all_reference_chosen_logps
)
self.train_dataset = self.train_dataset.add_column(
name="reference_rejected_logps", column=all_reference_rejected_logps
)
self._precomputed_train_ref_log_probs = True
return super().get_train_dataloader()
def get_eval_dataloader(self, eval_dataset: Optional[Dataset] = None) -> DataLoader:
"""
Returns the evaluation [`~torch.utils.data.DataLoader`].
Subclass of transformers.src.transformers.trainer.get_eval_dataloader to precompute `ref_log_probs`.
Args:
eval_dataset (`torch.utils.data.Dataset`, *optional*):
If provided, will override `self.eval_dataset`. If it is a [`~datasets.Dataset`], columns not accepted
by the `model.forward()` method are automatically removed. It must implement `__len__`.
"""
if eval_dataset is None and self.eval_dataset is None:
raise ValueError("Trainer: evaluation requires an eval_dataset.")
eval_dataset = eval_dataset if eval_dataset is not None else self.eval_dataset
if self.precompute_ref_log_probs and not self._precomputed_eval_ref_log_probs:
dataloader_params = {
"batch_size": self.args.per_device_eval_batch_size,
"collate_fn": self.data_collator,
"num_workers": self.args.dataloader_num_workers,
"pin_memory": self.args.dataloader_pin_memory,
"shuffle": False,
}
# prepare dataloader
data_loader = self.accelerator.prepare(DataLoader(eval_dataset, **dataloader_params))
reference_chosen_logps = []
reference_rejected_logps = []
for padded_batch in tqdm(iterable=data_loader, desc="Eval dataset reference log probs"):
reference_chosen_logp, reference_rejected_logp = self.compute_reference_log_probs(padded_batch)
reference_chosen_logp, reference_rejected_logp = self.accelerator.gather_for_metrics(
(reference_chosen_logp, reference_rejected_logp)
)
reference_chosen_logps.append(reference_chosen_logp.cpu())
reference_rejected_logps.append(reference_rejected_logp.cpu())
all_reference_chosen_logps = torch.cat(reference_chosen_logps).float().numpy()
all_reference_rejected_logps = torch.cat(reference_rejected_logps).float().numpy()
eval_dataset = eval_dataset.add_column(name="reference_chosen_logps", column=all_reference_chosen_logps)
eval_dataset = eval_dataset.add_column(
name="reference_rejected_logps", column=all_reference_rejected_logps
)
# Save calculated reference_chosen_logps and reference_rejected_logps to the eval_dataset for subsequent runs
if self.eval_dataset is not None:
self.eval_dataset = eval_dataset
self._precomputed_eval_ref_log_probs = True
return super().get_eval_dataloader(eval_dataset=eval_dataset)
def build_tokenized_answer(self, prompt, answer):
"""
Llama tokenizer does satisfy `enc(a + b) = enc(a) + enc(b)`.
It does ensure `enc(a + b) = enc(a) + enc(a + b)[len(enc(a)):]`.
Reference:
https://github.com/EleutherAI/lm-evaluation-harness/pull/531#issuecomment-1595586257
"""
full_tokenized = self.tokenizer(prompt + answer, add_special_tokens=False)
prompt_input_ids = self.tokenizer(prompt, add_special_tokens=False)["input_ids"]
answer_input_ids = full_tokenized["input_ids"][len(prompt_input_ids) :]
answer_attention_mask = full_tokenized["attention_mask"][len(prompt_input_ids) :]
# Concat tokens to form `enc(a) + enc(a + b)[len(enc(a)):]`
full_concat_input_ids = np.concatenate([prompt_input_ids, answer_input_ids])
# Prepare input tokens for token by token comparison
full_input_ids = np.array(full_tokenized["input_ids"])
if len(full_input_ids) != len(full_concat_input_ids):
raise ValueError("Prompt input ids and answer input ids should have the same length.")
# On some tokenizers, like Llama-2 tokenizer, there are occasions where tokens
# can be merged together when tokenizing prompt+answer. This could result
# on the last token from the prompt being different when tokenized on its own
# vs when done as prompt+answer.
response_token_ids_start_idx = len(prompt_input_ids)
# If tokenized prompt is different than both prompt+answer, then it means the
# last token has changed due to merging.
if prompt_input_ids != full_tokenized["input_ids"][:response_token_ids_start_idx]:
response_token_ids_start_idx -= 1
prompt_input_ids = full_tokenized["input_ids"][:response_token_ids_start_idx]
prompt_attention_mask = full_tokenized["attention_mask"][:response_token_ids_start_idx]
if len(prompt_input_ids) != len(prompt_attention_mask):
raise ValueError("Prompt input ids and attention mask should have the same length.")
answer_input_ids = full_tokenized["input_ids"][response_token_ids_start_idx:]
answer_attention_mask = full_tokenized["attention_mask"][response_token_ids_start_idx:]
return dict(
prompt_input_ids=prompt_input_ids,
prompt_attention_mask=prompt_attention_mask,
input_ids=answer_input_ids,
attention_mask=answer_attention_mask,
)
def tokenize_row(self, feature, model: Optional[Union[PreTrainedModel, nn.Module]] = None) -> Dict:
"""Tokenize a single row from a DPO specific dataset.
At this stage, we don't convert to PyTorch tensors yet; we just handle the truncation
in case the prompt + chosen or prompt + rejected responses is/are too long. First
we truncate the prompt; if we're still too long, we truncate the chosen/rejected.
We also create the labels for the chosen/rejected responses, which are of length equal to
the sum of the length of the prompt and the chosen/rejected response, with
label_pad_token_id for the prompt tokens.
"""
batch = {}
prompt = feature["prompt"]
chosen = feature["chosen"]
rejected = feature["rejected"]
if not self.is_encoder_decoder:
# Check issues below for more details
# 1. https://github.com/huggingface/trl/issues/907
# 2. https://github.com/EleutherAI/lm-evaluation-harness/pull/531#issuecomment-1595586257
# 3. https://github.com/LianjiaTech/BELLE/issues/337
if not isinstance(prompt, str):
raise ValueError(f"prompt should be an str but got {type(prompt)}")
prompt_tokens = self.tokenizer(prompt, add_special_tokens=False)
prompt_tokens = {f"prompt_{k}": v for k, v in prompt_tokens.items()}
if not isinstance(chosen, str):
raise ValueError(f"chosen should be an str but got {type(chosen)}")
chosen_tokens = self.build_tokenized_answer(prompt, chosen)
if not isinstance(rejected, str):
raise ValueError(f"rejected should be an str but got {type(rejected)}")
rejected_tokens = self.build_tokenized_answer(prompt, rejected)
# Last prompt token might get merged by tokenizer and
# it should not be included for generation if that happens
prompt_len_input_ids = len(prompt_tokens["prompt_input_ids"])
chosen_prompt_len_input_ids = len(chosen_tokens["prompt_input_ids"])
rejected_prompt_len_input_ids = len(rejected_tokens["prompt_input_ids"])
prompt_len_input_ids = min(chosen_prompt_len_input_ids, rejected_prompt_len_input_ids)
for k, v in prompt_tokens.items():
prompt_tokens[k] = v[:prompt_len_input_ids]
# Make sure prompts only have one different token at most an
# and length only differs by 1 at most
num_diff_tokens = sum(
[a != b for a, b in zip(chosen_tokens["prompt_input_ids"], rejected_tokens["prompt_input_ids"])]
)
num_diff_len = abs(chosen_prompt_len_input_ids - rejected_prompt_len_input_ids)
if num_diff_tokens > 1 or num_diff_len > 1:
raise ValueError(
"Chosen and rejected prompt_input_ids might only differ on the "
"last token due to tokenizer merge ops."
)
# add BOS token to head of prompt
prompt_tokens["prompt_input_ids"] = [self.tokenizer.bos_token_id] + prompt_tokens["prompt_input_ids"]
chosen_tokens["prompt_input_ids"] = [self.tokenizer.bos_token_id] + chosen_tokens["prompt_input_ids"]
rejected_tokens["prompt_input_ids"] = [self.tokenizer.bos_token_id] + rejected_tokens["prompt_input_ids"]
prompt_tokens["prompt_attention_mask"] = [1] + prompt_tokens["prompt_attention_mask"]
chosen_tokens["prompt_attention_mask"] = [1] + chosen_tokens["prompt_attention_mask"]
rejected_tokens["prompt_attention_mask"] = [1] + rejected_tokens["prompt_attention_mask"]
# add EOS token to end of answer
chosen_tokens["input_ids"].append(self.tokenizer.eos_token_id)
chosen_tokens["attention_mask"].append(1)
rejected_tokens["input_ids"].append(self.tokenizer.eos_token_id)
rejected_tokens["attention_mask"].append(1)
longer_response_length = max(len(chosen_tokens["input_ids"]), len(rejected_tokens["input_ids"]))
# if combined sequence is too long, truncate the prompt
for answer_tokens in [chosen_tokens, rejected_tokens, prompt_tokens]:
if len(answer_tokens["prompt_input_ids"]) + longer_response_length > self.max_length:
if self.truncation_mode == "keep_start":
for k in ["prompt_input_ids", "prompt_attention_mask"]:
answer_tokens[k] = answer_tokens[k][: self.max_prompt_length]
elif self.truncation_mode == "keep_end":
for k in ["prompt_input_ids", "prompt_attention_mask"]:
answer_tokens[k] = answer_tokens[k][-self.max_prompt_length :]
else:
raise ValueError(f"Unknown truncation mode: {self.truncation_mode}")
# if that's still too long, truncate the response
for answer_tokens in [chosen_tokens, rejected_tokens]:
if len(answer_tokens["prompt_input_ids"]) + longer_response_length > self.max_length:
for k in ["input_ids", "attention_mask"]:
answer_tokens[k] = answer_tokens[k][: self.max_length - self.max_prompt_length]
# Create labels
chosen_sequence_tokens = {
k: chosen_tokens[f"prompt_{k}"] + chosen_tokens[k] for k in ["input_ids", "attention_mask"]
}
rejected_sequence_tokens = {
k: rejected_tokens[f"prompt_{k}"] + rejected_tokens[k] for k in ["input_ids", "attention_mask"]
}
chosen_sequence_tokens["labels"] = chosen_sequence_tokens["input_ids"][:]
chosen_sequence_tokens["labels"][: len(chosen_tokens["prompt_input_ids"])] = [
self.label_pad_token_id
] * len(chosen_tokens["prompt_input_ids"])
rejected_sequence_tokens["labels"] = rejected_sequence_tokens["input_ids"][:]
rejected_sequence_tokens["labels"][: len(rejected_tokens["prompt_input_ids"])] = [
self.label_pad_token_id
] * len(rejected_tokens["prompt_input_ids"])
for k, toks in {
"chosen_": chosen_sequence_tokens,
"rejected_": rejected_sequence_tokens,
"": prompt_tokens,
}.items():
for type_key, tokens in toks.items():
if type_key == "token_type_ids":
continue
batch[f"{k}{type_key}"] = tokens
else:
chosen_tokens = self.tokenizer(
chosen, truncation=True, max_length=self.max_target_length, add_special_tokens=True
)
rejected_tokens = self.tokenizer(
rejected, truncation=True, max_length=self.max_target_length, add_special_tokens=True
)
prompt_tokens = self.tokenizer(
prompt, truncation=True, max_length=self.max_prompt_length, add_special_tokens=True
)
batch["chosen_labels"] = chosen_tokens["input_ids"]
batch["rejected_labels"] = rejected_tokens["input_ids"]
batch["prompt_input_ids"] = prompt_tokens["input_ids"]
batch["prompt_attention_mask"] = prompt_tokens["attention_mask"]
if model is not None and hasattr(model, "prepare_decoder_input_ids_from_labels"):
batch["rejected_decoder_input_ids"] = model.prepare_decoder_input_ids_from_labels(
labels=batch["rejected_labels"]
)
batch["chosen_decoder_input_ids"] = model.prepare_decoder_input_ids_from_labels(
labels=batch["chosen_labels"]
)
return batch
@contextmanager
def null_ref_context(self):
"""Context manager for handling null reference model (that is, peft adapter manipulation)."""
with self.accelerator.unwrap_model(
self.model
).disable_adapter() if self.is_peft_model and not self.ref_adapter_name else nullcontext():
if self.ref_adapter_name:
self.model.set_adapter(self.ref_adapter_name)
yield
if self.ref_adapter_name:
self.model.set_adapter(self.model_adapter_name or "default")
def compute_reference_log_probs(self, padded_batch: Dict) -> Dict:
"""Computes log probabilities of the reference model for a single padded batch of a DPO specific dataset."""
compte_ref_context_manager = torch.cuda.amp.autocast if self._peft_has_been_casted_to_bf16 else nullcontext
# compute reference logps
with torch.no_grad(), compte_ref_context_manager():
if self.ref_model is None:
with self.null_ref_context():
(
reference_chosen_logps,
reference_rejected_logps,
_,
_,
) = self.concatenated_forward(self.model, padded_batch)
else:
(
reference_chosen_logps,
reference_rejected_logps,
_,
_,
) = self.concatenated_forward(self.ref_model, padded_batch)
return reference_chosen_logps, reference_rejected_logps
@staticmethod
def concatenated_inputs(
batch: Dict[str, Union[List, torch.LongTensor]],
is_encoder_decoder: bool = False,
label_pad_token_id: int = -100,
padding_value: int = 0,
device: Optional[torch.device] = None,
) -> Dict[str, torch.LongTensor]:
"""Concatenate the chosen and rejected inputs into a single tensor.
Args:
batch: A batch of data. Must contain the keys 'chosen_input_ids' and 'rejected_input_ids', which are tensors of shape (batch_size, sequence_length).
is_encoder_decoder: Whether the model is an encoder-decoder model.
label_pad_token_id: The label pad token id.
padding_value: The padding value to use for the concatenated inputs_ids.
device: The device for the concatenated inputs.
Returns:
A dictionary containing the concatenated inputs under the key 'concatenated_input_ids'.
"""
concatenated_batch = {}
if is_encoder_decoder:
max_length = max(batch["chosen_labels"].shape[1], batch["rejected_labels"].shape[1])
else:
max_length = max(batch["chosen_input_ids"].shape[1], batch["rejected_input_ids"].shape[1])
for k in batch:
if k.startswith("chosen") and isinstance(batch[k], torch.Tensor):
if "labels" in k or is_encoder_decoder:
pad_value = label_pad_token_id
elif k.endswith("_input_ids"):
pad_value = padding_value
elif k.endswith("_attention_mask"):
pad_value = 0
concatenated_key = k.replace("chosen", "concatenated")
concatenated_batch[concatenated_key] = pad_to_length(batch[k], max_length, pad_value=pad_value)
for k in batch:
if k.startswith("rejected") and isinstance(batch[k], torch.Tensor):
if "labels" in k or is_encoder_decoder:
pad_value = label_pad_token_id
elif k.endswith("_input_ids"):
pad_value = padding_value
elif k.endswith("_attention_mask"):
pad_value = 0
concatenated_key = k.replace("rejected", "concatenated")
concatenated_batch[concatenated_key] = torch.cat(
(
concatenated_batch[concatenated_key],
pad_to_length(batch[k], max_length, pad_value=pad_value),
),
dim=0,
).to(device=device)
if is_encoder_decoder:
concatenated_batch["concatenated_input_ids"] = batch["prompt_input_ids"].repeat(2, 1).to(device=device)
concatenated_batch["concatenated_attention_mask"] = (
batch["prompt_attention_mask"].repeat(2, 1).to(device=device)
)
return concatenated_batch
def dpo_loss(
self,
policy_chosen_logps: torch.FloatTensor,
policy_rejected_logps: torch.FloatTensor,
reference_chosen_logps: torch.FloatTensor,
reference_rejected_logps: torch.FloatTensor,
) -> Tuple[torch.FloatTensor, torch.FloatTensor, torch.FloatTensor]:
"""Compute the DPO loss for a batch of policy and reference model log probabilities.
Args:
policy_chosen_logps: Log probabilities of the policy model for the chosen responses. Shape: (batch_size,)
policy_rejected_logps: Log probabilities of the policy model for the rejected responses. Shape: (batch_size,)
reference_chosen_logps: Log probabilities of the reference model for the chosen responses. Shape: (batch_size,)
reference_rejected_logps: Log probabilities of the reference model for the rejected responses. Shape: (batch_size,)
Returns:
A tuple of three tensors: (losses, chosen_rewards, rejected_rewards).
The losses tensor contains the DPO loss for each example in the batch.
The chosen_rewards and rejected_rewards tensors contain the rewards for the chosen and rejected responses, respectively.
"""
pi_logratios = policy_chosen_logps - policy_rejected_logps
if self.reference_free:
ref_logratios = torch.tensor([0], dtype=pi_logratios.dtype, device=pi_logratios.device)
else:
ref_logratios = reference_chosen_logps - reference_rejected_logps
pi_logratios = pi_logratios.to(self.accelerator.device)
ref_logratios = ref_logratios.to(self.accelerator.device)
logits = pi_logratios - ref_logratios
# The beta is a temperature parameter for the DPO loss, typically something in the range of 0.1 to 0.5.
# We ignore the reference model as beta -> 0. The label_smoothing parameter encodes our uncertainty about the labels and
# calculates a conservative DPO loss.
if self.loss_type == "sigmoid":
losses = (
-F.logsigmoid(self.beta * logits) * (1 - self.label_smoothing)
- F.logsigmoid(-self.beta * logits) * self.label_smoothing
)
elif self.loss_type == "hinge":
losses = torch.relu(1 - self.beta * logits)
elif self.loss_type == "ipo":
# eqn (17) of the paper where beta is the regularization parameter for the IPO loss, denoted by tau in the paper.
losses = (logits - 1 / (2 * self.beta)) ** 2
elif self.loss_type == "kto_pair":
# eqn (7) of the HALOs paper
chosen_KL = (policy_chosen_logps - reference_chosen_logps).mean().clamp(min=0)
rejected_KL = (policy_rejected_logps - reference_rejected_logps).mean().clamp(min=0)
chosen_logratios = policy_chosen_logps - reference_chosen_logps
rejected_logratios = policy_rejected_logps - reference_rejected_logps
# As described in the KTO report, the KL term for chosen (rejected) is estimated using the rejected (chosen) half.
losses = torch.cat(
(
1 - F.sigmoid(self.beta * (chosen_logratios - rejected_KL)),
1 - F.sigmoid(self.beta * (chosen_KL - rejected_logratios)),
),
0,
)
else:
raise ValueError(
f"Unknown loss type: {self.loss_type}. Should be one of ['sigmoid', 'hinge', 'ipo', 'kto_pair']"
)
chosen_rewards = (
self.beta
* (
policy_chosen_logps.to(self.accelerator.device) - reference_chosen_logps.to(self.accelerator.device)
).detach()
)
rejected_rewards = (
self.beta
* (
policy_rejected_logps.to(self.accelerator.device)
- reference_rejected_logps.to(self.accelerator.device)
).detach()
)
return losses, chosen_rewards, rejected_rewards
@staticmethod
def get_batch_logps(
logits: torch.FloatTensor,
labels: torch.LongTensor,
average_log_prob: bool = False,
label_pad_token_id: int = -100,
is_encoder_decoder: bool = False,
) -> torch.FloatTensor:
"""Compute the log probabilities of the given labels under the given logits.
Args:
logits: Logits of the model (unnormalized). Shape: (batch_size, sequence_length, vocab_size)
labels: Labels for which to compute the log probabilities. Label tokens with a value of label_pad_token_id are ignored. Shape: (batch_size, sequence_length)
average_log_prob: If True, return the average log probability per (non-masked) token. Otherwise, return the sum of the log probabilities of the (non-masked) tokens.
label_pad_token_id: The label pad token id.
is_encoder_decoder: Whether the model is an encoder-decoder model.
Returns:
A tensor of shape (batch_size,) containing the average/sum log probabilities of the given labels under the given logits.
"""
if logits.shape[:-1] != labels.shape:
raise ValueError("Logits (batch and sequence length dim) and labels must have the same shape.")
if not is_encoder_decoder:
labels = labels[:, 1:].clone()
logits = logits[:, :-1, :]
loss_mask = labels != label_pad_token_id
# dummy token; we'll ignore the losses on these tokens later
labels[labels == label_pad_token_id] = 0
per_token_logps = torch.gather(logits.log_softmax(-1), dim=2, index=labels.unsqueeze(2)).squeeze(2)
if average_log_prob:
return (per_token_logps * loss_mask).sum(-1) / loss_mask.sum(-1)
else:
return (per_token_logps * loss_mask).sum(-1)
def concatenated_forward(
self, model: nn.Module, batch: Dict[str, Union[List, torch.LongTensor]]
) -> Tuple[torch.FloatTensor, torch.FloatTensor, torch.FloatTensor, torch.FloatTensor]:
"""Run the given model on the given batch of inputs, concatenating the chosen and rejected inputs together.
We do this to avoid doing two forward passes, because it's faster for FSDP.
"""
concatenated_batch = self.concatenated_inputs(
batch,
is_encoder_decoder=self.is_encoder_decoder,
label_pad_token_id=self.label_pad_token_id,
padding_value=self.padding_value,
device=self.accelerator.device,
)
len_chosen = batch["chosen_labels"].shape[0]
model_kwargs = (
{
"labels": concatenated_batch["concatenated_labels"],
"decoder_input_ids": concatenated_batch.pop("concatenated_decoder_input_ids", None),
}
if self.is_encoder_decoder
else {}
)
all_logits = model(
concatenated_batch["concatenated_input_ids"],
attention_mask=concatenated_batch["concatenated_attention_mask"],
use_cache=False,
**model_kwargs,
).logits
all_logps = self.get_batch_logps(
all_logits,
concatenated_batch["concatenated_labels"],
average_log_prob=self.loss_type == "ipo",
is_encoder_decoder=self.is_encoder_decoder,
label_pad_token_id=self.label_pad_token_id,
)
chosen_logps = all_logps[:len_chosen]
rejected_logps = all_logps[len_chosen:]
chosen_logits = all_logits[:len_chosen]
rejected_logits = all_logits[len_chosen:]
return (chosen_logps, rejected_logps, chosen_logits, rejected_logits)
def get_batch_loss_metrics(
self,
model,
batch: Dict[str, Union[List, torch.LongTensor]],
train_eval: Literal["train", "eval"] = "train",
):
"""Compute the DPO loss and other metrics for the given batch of inputs for train or test."""
metrics = {}
(
policy_chosen_logps,
policy_rejected_logps,
policy_chosen_logits,
policy_rejected_logits,
) = self.concatenated_forward(model, batch)
# if reference_chosen_logps and reference_rejected_logps in batch use them, otherwise use the reference model
if "reference_chosen_logps" in batch and "reference_rejected_logps" in batch:
reference_chosen_logps = batch["reference_chosen_logps"]
reference_rejected_logps = batch["reference_rejected_logps"]
else:
with torch.no_grad():
if self.ref_model is None:
with self.null_ref_context():
(
reference_chosen_logps,
reference_rejected_logps,
_,
_,
) = self.concatenated_forward(self.model, batch)
else:
(
reference_chosen_logps,
reference_rejected_logps,
_,
_,
) = self.concatenated_forward(self.ref_model, batch)
losses, chosen_rewards, rejected_rewards = self.dpo_loss(
policy_chosen_logps,
policy_rejected_logps,
reference_chosen_logps,
reference_rejected_logps,
)
reward_accuracies = (chosen_rewards > rejected_rewards).float()
prefix = "eval_" if train_eval == "eval" else ""
metrics[f"{prefix}rewards/chosen"] = chosen_rewards.mean().cpu()
metrics[f"{prefix}rewards/rejected"] = rejected_rewards.mean().cpu()
metrics[f"{prefix}rewards/accuracies"] = reward_accuracies.mean().cpu()
metrics[f"{prefix}rewards/margins"] = (chosen_rewards - rejected_rewards).mean().cpu()
metrics[f"{prefix}logps/rejected"] = policy_rejected_logps.detach().mean().cpu()
metrics[f"{prefix}logps/chosen"] = policy_chosen_logps.detach().mean().cpu()
metrics[f"{prefix}logits/rejected"] = policy_rejected_logits.detach().mean().cpu()
metrics[f"{prefix}logits/chosen"] = policy_chosen_logits.detach().mean().cpu()
return losses.mean(), metrics
def compute_loss(
self,
model: Union[PreTrainedModel, nn.Module],
inputs: Dict[str, Union[torch.Tensor, Any]],
return_outputs=False,
) -> Union[torch.Tensor, Tuple[torch.Tensor, Dict[str, torch.Tensor]]]:
if not self.use_dpo_data_collator:
warnings.warn(
"compute_loss is only implemented for DPODataCollatorWithPadding, and you passed a datacollator that is different than "
"DPODataCollatorWithPadding - you might see unexpected behavior. Alternatively, you can implement your own prediction_step method if you are using a custom data collator"
)
compute_loss_context_manager = torch.cuda.amp.autocast if self._peft_has_been_casted_to_bf16 else nullcontext
with compute_loss_context_manager():
loss, metrics = self.get_batch_loss_metrics(model, inputs, train_eval="train")
# force log the metrics
self.store_metrics(metrics, train_eval="train")
if return_outputs:
return (loss, metrics)
return loss
def get_batch_samples(self, model, batch: Dict[str, torch.LongTensor]) -> Tuple[str, str]:
"""Generate samples from the model and reference model for the given batch of inputs."""
# If one uses `generate_during_eval` with peft + bf16, we need to explictly call generate with
# the torch cuda amp context manager as some hidden states are silently casted to full precision.
generate_context_manager = nullcontext if not self._peft_has_been_casted_to_bf16 else torch.cuda.amp.autocast
with generate_context_manager():
policy_output = model.generate(
input_ids=batch["prompt_input_ids"],
attention_mask=batch["prompt_attention_mask"],
max_length=self.max_length,
do_sample=True,
pad_token_id=self.tokenizer.pad_token_id,
)
# if reference_output in batch use that otherwise use the reference model
if "reference_output" in batch:
reference_output = batch["reference_output"]
else:
if self.ref_model is None:
with self.null_ref_context():
reference_output = self.model.generate(
input_ids=batch["prompt_input_ids"],
attention_mask=batch["prompt_attention_mask"],
max_length=self.max_length,
do_sample=True,
pad_token_id=self.tokenizer.pad_token_id,
)
else:
reference_output = self.ref_model.generate(
input_ids=batch["prompt_input_ids"],
attention_mask=batch["prompt_attention_mask"],
max_length=self.max_length,
do_sample=True,
pad_token_id=self.tokenizer.pad_token_id,
)
policy_output = pad_to_length(policy_output, self.max_length, self.tokenizer.pad_token_id)
policy_output_decoded = self.tokenizer.batch_decode(policy_output, skip_special_tokens=True)
reference_output = pad_to_length(reference_output, self.max_length, self.tokenizer.pad_token_id)
reference_output_decoded = self.tokenizer.batch_decode(reference_output, skip_special_tokens=True)
return policy_output_decoded, reference_output_decoded
def prediction_step(
self,
model: Union[PreTrainedModel, nn.Module],
inputs: Dict[str, Union[torch.Tensor, Any]],
prediction_loss_only: bool,
ignore_keys: Optional[List[str]] = None,
):
if not self.use_dpo_data_collator:
warnings.warn(
"prediction_step is only implemented for DPODataCollatorWithPadding, and you passed a datacollator that is different than "
"DPODataCollatorWithPadding - you might see unexpected behavior. Alternatively, you can implement your own prediction_step method if you are using a custom data collator"
)
if ignore_keys is None:
if hasattr(model, "config"):
ignore_keys = getattr(model.config, "keys_to_ignore_at_inference", [])
else:
ignore_keys = []
prediction_context_manager = torch.cuda.amp.autocast if self._peft_has_been_casted_to_bf16 else nullcontext
with torch.no_grad(), prediction_context_manager():
loss, metrics = self.get_batch_loss_metrics(model, inputs, train_eval="eval")
# force log the metrics
self.store_metrics(metrics, train_eval="eval")
if prediction_loss_only:
return (loss.detach(), None, None)
# logits for the chosen and rejected samples from model
logits_dict = {
"eval_logits/chosen": metrics["eval_logits/chosen"],
"eval_logits/rejected": metrics["eval_logits/rejected"],
}
logits = tuple(v.unsqueeze(dim=0) for k, v in logits_dict.items() if k not in ignore_keys)
logits = torch.stack(logits).mean(axis=1).to(self.accelerator.device)
labels = torch.zeros(logits.shape[0], device=self.accelerator.device)
return (loss.detach(), logits, labels)
def store_metrics(self, metrics: Dict[str, float], train_eval: Literal["train", "eval"] = "train") -> None:
for key, value in metrics.items():
self._stored_metrics[train_eval][key].append(value)
def evaluation_loop(
self,
dataloader: DataLoader,
description: str,
prediction_loss_only: Optional[bool] = None,
ignore_keys: Optional[List[str]] = None,
metric_key_prefix: str = "eval",
) -> EvalLoopOutput:
"""
Overriding built-in evaluation loop to store metrics for each batch.
Prediction/evaluation loop, shared by `Trainer.evaluate()` and `Trainer.predict()`.
Works both with or without labels.
"""
# Sample and save to game log if requested (for one batch to save time)
if self.generate_during_eval:
# Generate random indices within the range of the total number of samples
num_samples = len(dataloader.dataset)
random_indices = random.sample(range(num_samples), k=self.args.eval_batch_size)
# Use dataloader.dataset.select to get the random batch without iterating over the DataLoader
random_batch_dataset = dataloader.dataset.select(random_indices)
random_batch = self.data_collator(random_batch_dataset)
random_batch = self._prepare_inputs(random_batch)
policy_output_decoded, ref_output_decoded = self.get_batch_samples(self.model, random_batch)
self.log(
{
"game_log": wandb.Table(
columns=["Prompt", "Policy", "Ref Model"],
rows=[
[prompt, pol[len(prompt) :], ref[len(prompt) :]]
for prompt, pol, ref in zip(
random_batch["prompt"], policy_output_decoded, ref_output_decoded
)
],
)
}
)
self.state.log_history.pop()
# Base evaluation
initial_output = super().evaluation_loop(
dataloader, description, prediction_loss_only, ignore_keys, metric_key_prefix
)
return initial_output
def log(self, logs: Dict[str, float]) -> None:
"""
Log `logs` on the various objects watching training, including stored metrics.
Args:
logs (`Dict[str, float]`):
The values to log.
"""
# logs either has 'loss' or 'eval_loss'
train_eval = "train" if "loss" in logs else "eval"
# Add averaged stored metrics to logs
for key, metrics in self._stored_metrics[train_eval].items():
logs[key] = torch.tensor(metrics).mean().item()
del self._stored_metrics[train_eval]
return super().log(logs)
@wraps(Trainer.push_to_hub)
def push_to_hub(self, commit_message: Optional[str] = "End of training", blocking: bool = True, **kwargs) -> str:
"""
Overwrite the `push_to_hub` method in order to force-add the tag "sft" when pushing the
model on the Hub. Please refer to `~transformers.Trainer.push_to_hub` for more details.
"""
kwargs = trl_sanitze_kwargs_for_tagging(model=self.model, tag_names=self._tag_names, kwargs=kwargs)
return super().push_to_hub(commit_message=commit_message, blocking=blocking, **kwargs)
| trl/trl/trainer/dpo_trainer.py/0 | {
"file_path": "trl/trl/trainer/dpo_trainer.py",
"repo_id": "trl",
"token_count": 27655
} | 387 |
<!--Copyright 2022 The HuggingFace Team. All rights reserved.
Licensed under the Apache License, Version 2.0 (the "License"); you may not use this file except in compliance with
the License. You may obtain a copy of the License at
http://www.apache.org/licenses/LICENSE-2.0
Unless required by applicable law or agreed to in writing, software distributed under the License is distributed on
an "AS IS" BASIS, WITHOUT WARRANTIES OR CONDITIONS OF ANY KIND, either express or implied. See the License for the
specific language governing permissions and limitations under the License.
⚠️ Note that this file is in Markdown but contain specific syntax for our doc-builder (similar to MDX) that may not be
rendered properly in your Markdown viewer.
-->
# Migrating your code to 🤗 Accelerate
This tutorial will detail how to easily convert existing PyTorch code to use 🤗 Accelerate!
You'll see that by just changing a few lines of code, 🤗 Accelerate can perform its magic and get you on
your way toward running your code on distributed systems with ease!
## The base training loop
To begin, write out a very basic PyTorch training loop.
<Tip>
We are under the presumption that `training_dataloader`, `model`, `optimizer`, `scheduler`, and `loss_function` have been defined beforehand.
</Tip>
```python
device = "cuda"
model.to(device)
for batch in training_dataloader:
optimizer.zero_grad()
inputs, targets = batch
inputs = inputs.to(device)
targets = targets.to(device)
outputs = model(inputs)
loss = loss_function(outputs, targets)
loss.backward()
optimizer.step()
scheduler.step()
```
## Add in 🤗 Accelerate
To start using 🤗 Accelerate, first import and create an [`Accelerator`] instance:
```python
from accelerate import Accelerator
accelerator = Accelerator()
```
[`Accelerator`] is the main force behind utilizing all the possible options for distributed training!
### Setting the right device
The [`Accelerator`] class knows the right device to move any PyTorch object to at any time, so you should
change the definition of `device` to come from [`Accelerator`]:
```diff
- device = 'cuda'
+ device = accelerator.device
model.to(device)
```
### Preparing your objects
Next, you need to pass all of the important objects related to training into [`~Accelerator.prepare`]. 🤗 Accelerate will
make sure everything is setup in the current environment for you to start training:
```
model, optimizer, training_dataloader, scheduler = accelerator.prepare(
model, optimizer, training_dataloader, scheduler
)
```
These objects are returned in the same order they were sent in. By default when using `device_placement=True`, all of the objects that can be sent to the right device will be.
If you need to work with data that isn't passed to [~Accelerator.prepare] but should be on the active device, you should pass in the `device` you made earlier.
<Tip warning={true}>
Accelerate will only prepare objects that inherit from their respective PyTorch classes (such as `torch.optim.Optimizer`).
</Tip>
### Modifying the training loop
Finally, three lines of code need to be changed in the training loop. 🤗 Accelerate's DataLoader classes will automatically handle the device placement by default,
and [`~Accelerator.backward`] should be used for performing the backward pass:
```diff
- inputs = inputs.to(device)
- targets = targets.to(device)
outputs = model(inputs)
loss = loss_function(outputs, targets)
- loss.backward()
+ accelerator.backward(loss)
```
With that, your training loop is now ready to use 🤗 Accelerate!
## The finished code
Below is the final version of the converted code:
```python
from accelerate import Accelerator
accelerator = Accelerator()
model, optimizer, training_dataloader, scheduler = accelerator.prepare(
model, optimizer, training_dataloader, scheduler
)
for batch in training_dataloader:
optimizer.zero_grad()
inputs, targets = batch
outputs = model(inputs)
loss = loss_function(outputs, targets)
accelerator.backward(loss)
optimizer.step()
scheduler.step()
```
## More Resources
To check out more ways on how to migrate to 🤗 Accelerate, check out our [interactive migration tutorial](https://huggingface.co/docs/accelerate/usage_guides/explore) which showcases other items that need to be watched for when using Accelerate and how to do so quickly. | accelerate/docs/source/basic_tutorials/migration.md/0 | {
"file_path": "accelerate/docs/source/basic_tutorials/migration.md",
"repo_id": "accelerate",
"token_count": 1276
} | 0 |
<!--Copyright 2021 The HuggingFace Team. All rights reserved.
Licensed under the Apache License, Version 2.0 (the "License"); you may not use this file except in compliance with
the License. You may obtain a copy of the License at
http://www.apache.org/licenses/LICENSE-2.0
Unless required by applicable law or agreed to in writing, software distributed under the License is distributed on
an "AS IS" BASIS, WITHOUT WARRANTIES OR CONDITIONS OF ANY KIND, either express or implied. See the License for the
specific language governing permissions and limitations under the License.
⚠️ Note that this file is in Markdown but contain specific syntax for our doc-builder (similar to MDX) that may not be
rendered properly in your Markdown viewer.
-->
# The Command Line
Below is a list of all the available commands 🤗 Accelerate with their parameters
## accelerate config
**Command**:
`accelerate config` or `accelerate-config`
Launches a series of prompts to create and save a `default_config.yml` configuration file for your training system. Should
always be ran first on your machine.
**Usage**:
```bash
accelerate config [arguments]
```
**Optional Arguments**:
* `--config_file CONFIG_FILE` (`str`) -- The path to use to store the config file. Will default to a file named default_config.yaml in the cache location, which is the content
of the environment `HF_HOME` suffixed with 'accelerate', or if you don't have such an environment variable, your cache directory
(`~/.cache` or the content of `XDG_CACHE_HOME`) suffixed with `huggingface`.
* `-h`, `--help` (`bool`) -- Show a help message and exit
## accelerate config default
**Command**:
`accelerate config default` or `accelerate-config default`
Create a default config file for Accelerate with only a few flags set.
**Usage**:
```bash
accelerate config default [arguments]
```
**Optional Arguments**:
* `--config_file CONFIG_FILE` (`str`) -- The path to use to store the config file. Will default to a file named default_config.yaml in the cache location, which is the content
of the environment `HF_HOME` suffixed with 'accelerate', or if you don't have such an environment variable, your cache directory
(`~/.cache` or the content of `XDG_CACHE_HOME`) suffixed with `huggingface`.
* `-h`, `--help` (`bool`) -- Show a help message and exit
* `--mixed_precision {no,fp16,bf16}` (`str`) -- Whether or not to use mixed precision training. Choose between FP16 and BF16 (bfloat16) training. BF16 training is only supported on Nvidia Ampere GPUs and PyTorch 1.10 or later.
## accelerate config update
**Command**:
`accelerate config update` or `accelerate-config update`
Update an existing config file with the latest defaults while maintaining the old configuration.
**Usage**:
```bash
accelerate config update [arguments]
```
**Optional Arguments**:
* `--config_file CONFIG_FILE` (`str`) -- The path to the config file to update. Will default to a file named default_config.yaml in the cache location, which is the content
of the environment `HF_HOME` suffixed with 'accelerate', or if you don't have such an environment variable, your cache directory
(`~/.cache` or the content of `XDG_CACHE_HOME`) suffixed with `huggingface`.
* `-h`, `--help` (`bool`) -- Show a help message and exit
## accelerate env
**Command**:
`accelerate env` or `accelerate-env` or `python -m accelerate.commands.env`
Lists the contents of the passed 🤗 Accelerate configuration file. Should always be used when opening an issue on the [GitHub repository](https://github.com/huggingface/accelerate).
**Usage**:
```bash
accelerate env [arguments]
```
**Optional Arguments**:
* `--config_file CONFIG_FILE` (`str`) -- The path to use to store the config file. Will default to a file named default_config.yaml in the cache location, which is the content
of the environment `HF_HOME` suffixed with 'accelerate', or if you don't have such an environment variable, your cache directory
(`~/.cache` or the content of `XDG_CACHE_HOME`) suffixed with `huggingface`.
* `-h`, `--help` (`bool`) -- Show a help message and exit
## accelerate launch
**Command**:
`accelerate launch` or `accelerate-launch` or `python -m accelerate.commands.launch`
Launches a specified script on a distributed system with the right parameters.
**Usage**:
```bash
accelerate launch [arguments] {training_script} --{training_script-argument-1} --{training_script-argument-2} ...
```
**Positional Arguments**:
- `{training_script}` -- The full path to the script to be launched in parallel
- `--{training_script-argument-1}` -- Arguments of the training script
**Optional Arguments**:
* `-h`, `--help` (`bool`) -- Show a help message and exit
* `--config_file CONFIG_FILE` (`str`)-- The config file to use for the default values in the launching script.
* `-m`, `--module` (`bool`) -- Change each process to interpret the launch script as a Python module, executing with the same behavior as 'python -m'.
* `--no_python` (`bool`) -- Skip prepending the training script with 'python' - just execute it directly. Useful when the script is not a Python script.
* `--debug` (`bool`) -- Whether to print out the torch.distributed stack trace when something fails.
* `-q`, `--quiet` (`bool`) -- Silence subprocess errors from the launch stack trace to only show the relevant tracebacks. (Only applicable to DeepSpeed and single-process configurations).
The rest of these arguments are configured through `accelerate config` and are read in from the specified `--config_file` (or default configuration) for their
values. They can also be passed in manually.
**Hardware Selection Arguments**:
* `--cpu` (`bool`) -- Whether or not to force the training on the CPU.
* `--multi_gpu` (`bool`) -- Whether or not this should launch a distributed GPU training.
* `--tpu` (`bool`) -- Whether or not this should launch a TPU training.
* `--ipex` (`bool`) -- Whether or not this should launch an Intel Pytorch Extension (IPEX) training.
**Resource Selection Arguments**:
The following arguments are useful for fine-tuning how available hardware should be used
* `--mixed_precision {no,fp16,bf16}` (`str`) -- Whether or not to use mixed precision training. Choose between FP16 and BF16 (bfloat16) training. BF16 training is only supported on Nvidia Ampere GPUs and PyTorch 1.10 or later.
* `--num_processes NUM_PROCESSES` (`int`) -- The total number of processes to be launched in parallel.
* `--num_machines NUM_MACHINES` (`int`) -- The total number of machines used in this training.
* `--num_cpu_threads_per_process NUM_CPU_THREADS_PER_PROCESS` (`int`) -- The number of CPU threads per process. Can be tuned for optimal performance.
**Training Paradigm Arguments**:
The following arguments are useful for selecting which training paradigm to use.
* `--use_deepspeed` (`bool`) -- Whether or not to use DeepSpeed for training.
* `--use_fsdp` (`bool`) -- Whether or not to use FullyShardedDataParallel for training.
* `--use_megatron_lm` (`bool`) -- Whether or not to use Megatron-LM for training.
* `--use_xpu` (`bool`) -- Whether to use IPEX plugin to speed up training on XPU specifically.
**Distributed GPU Arguments**:
The following arguments are only useful when `multi_gpu` is passed or multi-gpu training is configured through `accelerate config`:
* `--gpu_ids` (`str`) -- What GPUs (by id) should be used for training on this machine as a comma-seperated list
* `--same_network` (`bool`) -- Whether all machines used for multinode training exist on the same local network.
* `--machine_rank MACHINE_RANK` (`int`) -- The rank of the machine on which this script is launched.
* `--main_process_ip MAIN_PROCESS_IP` (`str`) -- The IP address of the machine of rank 0.
* `--main_process_port MAIN_PROCESS_PORT` (`int`) -- The port to use to communicate with the machine of rank 0.
* `--rdzv_backend` (`str`) -- The rendezvous method to use, such as "static" or "c10d"
* `--rdzv_conf` (`str`) -- Additional rendezvous configuration (<key1>=<value1>,<key2>=<value2>,...).
* `--max_restarts` (`int`) -- Maximum number of worker group restarts before failing.
* `--monitor_interval` (`float`) -- Interval, in seconds, to monitor the state of workers.
**TPU Arguments**:
The following arguments are only useful when `tpu` is passed or TPU training is configured through `accelerate config`:
* `--main_training_function MAIN_TRAINING_FUNCTION` (`str`) -- The name of the main function to be executed in your script.
* `--downcast_bf16` (`bool`) -- Whether when using bf16 precision on TPUs if both float and double tensors are cast to bfloat16 or if double tensors remain as float32.
**DeepSpeed Arguments**:
The following arguments are only useful when `use_deepspeed` is passed or `deepspeed` is configured through `accelerate config`:
* `--deepspeed_config_file` (`str`) -- DeepSpeed config file.
* `--zero_stage` (`int`) -- DeepSpeed's ZeRO optimization stage.
* `--offload_optimizer_device` (`str`) -- Decides where (none|cpu|nvme) to offload optimizer states.
* `--offload_param_device` (`str`) -- Decides where (none|cpu|nvme) to offload parameters.
* `--gradient_accumulation_steps` (`int`) -- No of gradient_accumulation_steps used in your training script.
* `--gradient_clipping` (`float`) -- Gradient clipping value used in your training script.
* `--zero3_init_flag` (`str`) -- Decides Whether (true|false) to enable `deepspeed.zero.Init` for constructing massive models. Only applicable with DeepSpeed ZeRO Stage-3.
* `--zero3_save_16bit_model` (`str`) -- Decides Whether (true|false) to save 16-bit model weights when using ZeRO Stage-3. Only applicable with DeepSpeed ZeRO Stage-3.
* `--deepspeed_hostfile` (`str`) -- DeepSpeed hostfile for configuring multi-node compute resources.
* `--deepspeed_exclusion_filter` (`str`) -- DeepSpeed exclusion filter string when using mutli-node setup.
* `--deepspeed_inclusion_filter` (`str`) -- DeepSpeed inclusion filter string when using mutli-node setup.
* `--deepspeed_multinode_launcher` (`str`) -- DeepSpeed multi-node launcher to use.
**Fully Sharded Data Parallelism Arguments**:
The following arguments are only useful when `use_fsdp` is passed or Fully Sharded Data Parallelism is configured through `accelerate config`:
* `--fsdp_offload_params` (`str`) -- Decides Whether (true|false) to offload parameters and gradients to CPU.
* `--fsdp_min_num_params` (`int`) -- FSDP's minimum number of parameters for Default Auto Wrapping.
* `--fsdp_sharding_strategy` (`int`) -- FSDP's Sharding Strategy.
* `--fsdp_auto_wrap_policy` (`str`) -- FSDP's auto wrap policy.
* `--fsdp_transformer_layer_cls_to_wrap` (`str`) -- Transformer layer class name (case-sensitive) to wrap, e.g, `BertLayer`, `GPTJBlock`, `T5Block` ...
* `--fsdp_backward_prefetch_policy` (`str`) -- FSDP's backward prefetch policy.
* `--fsdp_state_dict_type` (`str`) -- FSDP's state dict type.
**Megatron-LM Arguments**:
The following arguments are only useful when `use_megatron_lm` is passed or Megatron-LM is configured through `accelerate config`:
* `--megatron_lm_tp_degree` (``) -- Megatron-LM's Tensor Parallelism (TP) degree.
* `--megatron_lm_pp_degree` (``) -- Megatron-LM's Pipeline Parallelism (PP) degree.
* `--megatron_lm_num_micro_batches` (``) -- Megatron-LM's number of micro batches when PP degree > 1.
* `--megatron_lm_sequence_parallelism` (``) -- Decides Whether (true|false) to enable Sequence Parallelism when TP degree > 1.
* `--megatron_lm_recompute_activations` (``) -- Decides Whether (true|false) to enable Selective Activation Recomputation.
* `--megatron_lm_use_distributed_optimizer` (``) -- Decides Whether (true|false) to use distributed optimizer which shards optimizer state and gradients across Data Parallel (DP) ranks.
* `--megatron_lm_gradient_clipping` (``) -- Megatron-LM's gradient clipping value based on global L2 Norm (0 to disable).
**AWS SageMaker Arguments**:
The following arguments are only useful when training in SageMaker
* `--aws_access_key_id AWS_ACCESS_KEY_ID` (`str`) -- The AWS_ACCESS_KEY_ID used to launch the Amazon SageMaker training job
* `--aws_secret_access_key AWS_SECRET_ACCESS_KEY` (`str`) -- The AWS_SECRET_ACCESS_KEY used to launch the Amazon SageMaker training job
## accelerate estimate-memory
**Command**:
`accelerate estimate-memory` or `accelerate-estimate-memory` or `python -m accelerate.commands.estimate`
Estimates the total vRAM a particular model hosted on the Hub needs to be loaded in with an estimate for training. Requires that `huggingface_hub` be installed.
<Tip>
When performing inference, typically add ≤20% to the result as overall allocation [as referenced here](https://blog.eleuther.ai/transformer-math/). We will have more extensive estimations in the future that will automatically be included in the calculation.
</Tip>
**Usage**:
```bash
accelerate estimate-memory {MODEL_NAME} --library_name {LIBRARY_NAME} --dtypes {dtype_1} {dtype_2} ...
```
**Required Arguments**:
* `MODEL_NAME` (`str`)-- The model name on the Hugging Face Hub
**Optional Arguments**:
* `--library_name {timm,transformers}` (`str`) -- The library the model has an integration with, such as `transformers`, needed only if this information is not stored on the Hub
* `--dtypes {float32,float16,int8,int4}` (`[{float32,float16,int8,int4} ...]`) -- The dtypes to use for the model, must be one (or many) of `float32`, `float16`, `int8`, and `int4`
* `--trust_remote_code` (`bool`) -- Whether or not to allow for custom models defined on the Hub in their own modeling files. This option should only be passed for repositories you trust and in which you have read the code, as it will execute code present on the Hub on your local machine.
## accelerate tpu-config
`accelerate tpu-config`
**Usage**:
```bash
accelerate tpu-config [arguments]
```
**Optional Arguments**:
* `-h`, `--help` (`bool`) -- Show a help message and exit
**Config Arguments**:
Arguments that can be configured through `accelerate config`.
* `--config_file` (`str`) -- Path to the config file to use for accelerate.
* `--tpu_name` (`str`) -- The name of the TPU to use. If not specified, will use the TPU specified in the config file.
* `--tpu_zone` (`str`) -- The zone of the TPU to use. If not specified, will use the zone specified in the config file.
**TPU Arguments**:
Arguments for options ran inside the TPU.
* `--command_file` (`str`) -- The path to the file containing the commands to run on the pod on startup.
* `--command` (`str`) -- A command to run on the pod. Can be passed multiple times.
* `--install_accelerate` (`bool`) -- Whether to install accelerate on the pod. Defaults to False.
* `--accelerate_version` (`str`) -- The version of accelerate to install on the pod. If not specified, will use the latest pypi version. Specify 'dev' to install from GitHub.
* `--debug` (`bool`) -- If set, will print the command that would be run instead of running it.
## accelerate test
`accelerate test` or `accelerate-test`
Runs `accelerate/test_utils/test_script.py` to verify that 🤗 Accelerate has been properly configured on your system and runs.
**Usage**:
```bash
accelerate test [arguments]
```
**Optional Arguments**:
* `--config_file CONFIG_FILE` (`str`) -- The path to use to store the config file. Will default to a file named default_config.yaml in the cache location, which is the content
of the environment `HF_HOME` suffixed with 'accelerate', or if you don't have such an environment variable, your cache directory
(`~/.cache` or the content of `XDG_CACHE_HOME`) suffixed with `huggingface`.
* `-h`, `--help` (`bool`) -- Show a help message and exit
| accelerate/docs/source/package_reference/cli.md/0 | {
"file_path": "accelerate/docs/source/package_reference/cli.md",
"repo_id": "accelerate",
"token_count": 4969
} | 1 |
<!--Copyright 2022 The HuggingFace Team. All rights reserved.
Licensed under the Apache License, Version 2.0 (the "License"); you may not use this file except in compliance with
the License. You may obtain a copy of the License at
http://www.apache.org/licenses/LICENSE-2.0
Unless required by applicable law or agreed to in writing, software distributed under the License is distributed on
an "AS IS" BASIS, WITHOUT WARRANTIES OR CONDITIONS OF ANY KIND, either express or implied. See the License for the
specific language governing permissions and limitations under the License.
⚠️ Note that this file is in Markdown but contain specific syntax for our doc-builder (similar to MDX) that may not be
rendered properly in your Markdown viewer.
-->
# Learning how to incorporate 🤗 Accelerate features quickly!
Please use the interactive tool below to help you get started with learning about a particular
feature of 🤗 Accelerate and how to utilize it! It will provide you with a code diff, an explanation
towards what is going on, as well as provide you with some useful links to explore more within
the documentation!
Most code examples start from the following python code before integrating 🤗 Accelerate in some way:
```python
for batch in dataloader:
optimizer.zero_grad()
inputs, targets = batch
inputs = inputs.to(device)
targets = targets.to(device)
outputs = model(inputs)
loss = loss_function(outputs, targets)
loss.backward()
optimizer.step()
scheduler.step()
```
<div class="block dark:hidden">
<iframe
src="https://hf-accelerate-accelerate-examples.hf.space?__theme=light"
width="850"
height="1600"
></iframe>
</div>
<div class="hidden dark:block">
<iframe
src="https://hf-accelerate-accelerate-examples.hf.space?__theme=dark"
width="850"
height="1600"
></iframe>
</div>
| accelerate/docs/source/usage_guides/explore.md/0 | {
"file_path": "accelerate/docs/source/usage_guides/explore.md",
"repo_id": "accelerate",
"token_count": 581
} | 2 |
# coding=utf-8
# Copyright 2021 The HuggingFace Inc. team. All rights reserved.
#
# Licensed under the Apache License, Version 2.0 (the "License");
# you may not use this file except in compliance with the License.
# You may obtain a copy of the License at
#
# http://www.apache.org/licenses/LICENSE-2.0
#
# Unless required by applicable law or agreed to in writing, software
# distributed under the License is distributed on an "AS IS" BASIS,
# WITHOUT WARRANTIES OR CONDITIONS OF ANY KIND, either express or implied.
# See the License for the specific language governing permissions and
# limitations under the License.
import argparse
import os
import evaluate
import torch
from datasets import load_dataset
from torch.optim import AdamW
from torch.utils.data import DataLoader
from transformers import AutoModelForSequenceClassification, AutoTokenizer, get_linear_schedule_with_warmup, set_seed
from accelerate import Accelerator, DistributedType
########################################################################
# This is a fully working simple example to use Accelerate,
# specifically showcasing the checkpointing capability,
# and builds off the `nlp_example.py` script.
#
# This example trains a Bert base model on GLUE MRPC
# in any of the following settings (with the same script):
# - single CPU or single GPU
# - multi GPUS (using PyTorch distributed mode)
# - (multi) TPUs
# - fp16 (mixed-precision) or fp32 (normal precision)
#
# To help focus on the differences in the code, building `DataLoaders`
# was refactored into its own function.
# New additions from the base script can be found quickly by
# looking for the # New Code # tags
#
# To run it in each of these various modes, follow the instructions
# in the readme for examples:
# https://github.com/huggingface/accelerate/tree/main/examples
#
########################################################################
MAX_GPU_BATCH_SIZE = 16
EVAL_BATCH_SIZE = 32
def get_dataloaders(accelerator: Accelerator, batch_size: int = 16):
"""
Creates a set of `DataLoader`s for the `glue` dataset,
using "bert-base-cased" as the tokenizer.
Args:
accelerator (`Accelerator`):
An `Accelerator` object
batch_size (`int`, *optional*):
The batch size for the train and validation DataLoaders.
"""
tokenizer = AutoTokenizer.from_pretrained("bert-base-cased")
datasets = load_dataset("glue", "mrpc")
def tokenize_function(examples):
# max_length=None => use the model max length (it's actually the default)
outputs = tokenizer(examples["sentence1"], examples["sentence2"], truncation=True, max_length=None)
return outputs
# Apply the method we just defined to all the examples in all the splits of the dataset
# starting with the main process first:
with accelerator.main_process_first():
tokenized_datasets = datasets.map(
tokenize_function,
batched=True,
remove_columns=["idx", "sentence1", "sentence2"],
)
# We also rename the 'label' column to 'labels' which is the expected name for labels by the models of the
# transformers library
tokenized_datasets = tokenized_datasets.rename_column("label", "labels")
def collate_fn(examples):
# On TPU it's best to pad everything to the same length or training will be very slow.
max_length = 128 if accelerator.distributed_type == DistributedType.TPU else None
# When using mixed precision we want round multiples of 8/16
if accelerator.mixed_precision == "fp8":
pad_to_multiple_of = 16
elif accelerator.mixed_precision != "no":
pad_to_multiple_of = 8
else:
pad_to_multiple_of = None
return tokenizer.pad(
examples,
padding="longest",
max_length=max_length,
pad_to_multiple_of=pad_to_multiple_of,
return_tensors="pt",
)
# Instantiate dataloaders.
train_dataloader = DataLoader(
tokenized_datasets["train"], shuffle=True, collate_fn=collate_fn, batch_size=batch_size
)
eval_dataloader = DataLoader(
tokenized_datasets["validation"], shuffle=False, collate_fn=collate_fn, batch_size=EVAL_BATCH_SIZE
)
return train_dataloader, eval_dataloader
# For testing only
if os.environ.get("TESTING_MOCKED_DATALOADERS", None) == "1":
from accelerate.test_utils.training import mocked_dataloaders
get_dataloaders = mocked_dataloaders # noqa: F811
def training_function(config, args):
# For testing only
if os.environ.get("TESTING_MOCKED_DATALOADERS", None) == "1":
config["num_epochs"] = 2
# Initialize accelerator
accelerator = Accelerator(cpu=args.cpu, mixed_precision=args.mixed_precision)
# Sample hyper-parameters for learning rate, batch size, seed and a few other HPs
lr = config["lr"]
num_epochs = int(config["num_epochs"])
seed = int(config["seed"])
batch_size = int(config["batch_size"])
# New Code #
# Parse out whether we are saving every epoch or after a certain number of batches
if hasattr(args.checkpointing_steps, "isdigit"):
if args.checkpointing_steps == "epoch":
checkpointing_steps = args.checkpointing_steps
elif args.checkpointing_steps.isdigit():
checkpointing_steps = int(args.checkpointing_steps)
else:
raise ValueError(
f"Argument `checkpointing_steps` must be either a number or `epoch`. `{args.checkpointing_steps}` passed."
)
else:
checkpointing_steps = None
set_seed(seed)
train_dataloader, eval_dataloader = get_dataloaders(accelerator, batch_size)
metric = evaluate.load("glue", "mrpc")
# If the batch size is too big we use gradient accumulation
gradient_accumulation_steps = 1
if batch_size > MAX_GPU_BATCH_SIZE and accelerator.distributed_type != DistributedType.TPU:
gradient_accumulation_steps = batch_size // MAX_GPU_BATCH_SIZE
batch_size = MAX_GPU_BATCH_SIZE
# Instantiate the model (we build the model here so that the seed also control new weights initialization)
model = AutoModelForSequenceClassification.from_pretrained("bert-base-cased", return_dict=True)
# We could avoid this line since the accelerator is set with `device_placement=True` (default value).
# Note that if you are placing tensors on devices manually, this line absolutely needs to be before the optimizer
# creation otherwise training will not work on TPU (`accelerate` will kindly throw an error to make us aware of that).
model = model.to(accelerator.device)
# Instantiate optimizer
optimizer = AdamW(params=model.parameters(), lr=lr)
# Instantiate scheduler
lr_scheduler = get_linear_schedule_with_warmup(
optimizer=optimizer,
num_warmup_steps=100,
num_training_steps=(len(train_dataloader) * num_epochs) // gradient_accumulation_steps,
)
# Prepare everything
# There is no specific order to remember, we just need to unpack the objects in the same order we gave them to the
# prepare method.
model, optimizer, train_dataloader, eval_dataloader, lr_scheduler = accelerator.prepare(
model, optimizer, train_dataloader, eval_dataloader, lr_scheduler
)
# New Code #
# We need to keep track of how many total steps we have iterated over
overall_step = 0
# We also need to keep track of the stating epoch so files are named properly
starting_epoch = 0
# We need to load the checkpoint back in before training here with `load_state`
# The total number of epochs is adjusted based on where the state is being loaded from,
# as we assume continuation of the same training script
if args.resume_from_checkpoint:
if args.resume_from_checkpoint is not None or args.resume_from_checkpoint != "":
accelerator.print(f"Resumed from checkpoint: {args.resume_from_checkpoint}")
accelerator.load_state(args.resume_from_checkpoint)
path = os.path.basename(args.resume_from_checkpoint)
else:
# Get the most recent checkpoint
dirs = [f.name for f in os.scandir(os.getcwd()) if f.is_dir()]
dirs.sort(key=os.path.getctime)
path = dirs[-1] # Sorts folders by date modified, most recent checkpoint is the last
# Extract `epoch_{i}` or `step_{i}`
training_difference = os.path.splitext(path)[0]
if "epoch" in training_difference:
starting_epoch = int(training_difference.replace("epoch_", "")) + 1
resume_step = None
else:
resume_step = int(training_difference.replace("step_", ""))
starting_epoch = resume_step // len(train_dataloader)
resume_step -= starting_epoch * len(train_dataloader)
# Now we train the model
for epoch in range(starting_epoch, num_epochs):
model.train()
# New Code #
if args.resume_from_checkpoint and epoch == starting_epoch and resume_step is not None:
# We need to skip steps until we reach the resumed step
active_dataloader = accelerator.skip_first_batches(train_dataloader, resume_step)
overall_step += resume_step
else:
# After the first iteration though, we need to go back to the original dataloader
active_dataloader = train_dataloader
for step, batch in enumerate(active_dataloader):
# We could avoid this line since we set the accelerator with `device_placement=True`.
batch.to(accelerator.device)
outputs = model(**batch)
loss = outputs.loss
loss = loss / gradient_accumulation_steps
accelerator.backward(loss)
if step % gradient_accumulation_steps == 0:
optimizer.step()
lr_scheduler.step()
optimizer.zero_grad()
# New Code #
overall_step += 1
# New Code #
# We save the model, optimizer, lr_scheduler, and seed states by calling `save_state`
# These are saved to folders named `step_{overall_step}`
# Will contain files: "pytorch_model.bin", "optimizer.bin", "scheduler.bin", and "random_states.pkl"
# If mixed precision was used, will also save a "scalar.bin" file
if isinstance(checkpointing_steps, int):
output_dir = f"step_{overall_step}"
if overall_step % checkpointing_steps == 0:
if args.output_dir is not None:
output_dir = os.path.join(args.output_dir, output_dir)
accelerator.save_state(output_dir)
model.eval()
for step, batch in enumerate(eval_dataloader):
# We could avoid this line since we set the accelerator with `device_placement=True` (the default).
batch.to(accelerator.device)
with torch.no_grad():
outputs = model(**batch)
predictions = outputs.logits.argmax(dim=-1)
predictions, references = accelerator.gather_for_metrics((predictions, batch["labels"]))
metric.add_batch(
predictions=predictions,
references=references,
)
eval_metric = metric.compute()
# Use accelerator.print to print only on the main process.
accelerator.print(f"epoch {epoch}:", eval_metric)
# New Code #
# We save the model, optimizer, lr_scheduler, and seed states by calling `save_state`
# These are saved to folders named `epoch_{epoch}`
# Will contain files: "pytorch_model.bin", "optimizer.bin", "scheduler.bin", and "random_states.pkl"
# If mixed precision was used, will also save a "scalar.bin" file
if checkpointing_steps == "epoch":
output_dir = f"epoch_{epoch}"
if args.output_dir is not None:
output_dir = os.path.join(args.output_dir, output_dir)
accelerator.save_state(output_dir)
def main():
parser = argparse.ArgumentParser(description="Simple example of training script.")
parser.add_argument(
"--mixed_precision",
type=str,
default=None,
choices=["no", "fp16", "bf16", "fp8"],
help="Whether to use mixed precision. Choose"
"between fp16 and bf16 (bfloat16). Bf16 requires PyTorch >= 1.10."
"and an Nvidia Ampere GPU.",
)
parser.add_argument("--cpu", action="store_true", help="If passed, will train on the CPU.")
parser.add_argument(
"--checkpointing_steps",
type=str,
default=None,
help="Whether the various states should be saved at the end of every n steps, or 'epoch' for each epoch.",
)
parser.add_argument(
"--output_dir",
type=str,
default=".",
help="Optional save directory where all checkpoint folders will be stored. Default is the current working directory.",
)
parser.add_argument(
"--resume_from_checkpoint",
type=str,
default=None,
help="If the training should continue from a checkpoint folder.",
)
args = parser.parse_args()
config = {"lr": 2e-5, "num_epochs": 3, "seed": 42, "batch_size": 16}
training_function(config, args)
if __name__ == "__main__":
main()
| accelerate/examples/by_feature/checkpointing.py/0 | {
"file_path": "accelerate/examples/by_feature/checkpointing.py",
"repo_id": "accelerate",
"token_count": 5205
} | 3 |
__version__ = "0.27.0.dev0"
from .accelerator import Accelerator
from .big_modeling import (
cpu_offload,
cpu_offload_with_hook,
disk_offload,
dispatch_model,
init_empty_weights,
init_on_device,
load_checkpoint_and_dispatch,
)
from .data_loader import skip_first_batches
from .launchers import debug_launcher, notebook_launcher
from .state import PartialState
from .utils import (
AutocastKwargs,
DeepSpeedPlugin,
DistributedDataParallelKwargs,
DistributedType,
FullyShardedDataParallelPlugin,
GradScalerKwargs,
InitProcessGroupKwargs,
find_executable_batch_size,
infer_auto_device_map,
is_rich_available,
load_checkpoint_in_model,
synchronize_rng_states,
)
if is_rich_available():
from .utils import rich
| accelerate/src/accelerate/__init__.py/0 | {
"file_path": "accelerate/src/accelerate/__init__.py",
"repo_id": "accelerate",
"token_count": 304
} | 4 |
#!/usr/bin/env python
# Copyright 2021 The HuggingFace Team. All rights reserved.
#
# Licensed under the Apache License, Version 2.0 (the "License");
# you may not use this file except in compliance with the License.
# You may obtain a copy of the License at
#
# http://www.apache.org/licenses/LICENSE-2.0
#
# Unless required by applicable law or agreed to in writing, software
# distributed under the License is distributed on an "AS IS" BASIS,
# WITHOUT WARRANTIES OR CONDITIONS OF ANY KIND, either express or implied.
# See the License for the specific language governing permissions and
# limitations under the License.
import argparse
import importlib
import logging
import os
import subprocess
import sys
from pathlib import Path
import psutil
import torch
from accelerate.commands.config import default_config_file, load_config_from_file
from accelerate.commands.config.config_args import SageMakerConfig
from accelerate.commands.config.config_utils import DYNAMO_BACKENDS
from accelerate.state import get_int_from_env
from accelerate.utils import (
ComputeEnvironment,
DistributedType,
PrepareForLaunch,
_filter_args,
check_cuda_p2p_ib_support,
is_bf16_available,
is_deepspeed_available,
is_npu_available,
is_rich_available,
is_sagemaker_available,
is_torch_version,
is_tpu_available,
is_xpu_available,
patch_environment,
prepare_deepspeed_cmd_env,
prepare_multi_gpu_env,
prepare_sagemager_args_inputs,
prepare_simple_launcher_cmd_env,
prepare_tpu,
)
from accelerate.utils.constants import DEEPSPEED_MULTINODE_LAUNCHERS, TORCH_DYNAMO_MODES
if is_rich_available():
from rich import get_console
from rich.logging import RichHandler
FORMAT = "%(message)s"
logging.basicConfig(format=FORMAT, datefmt="[%X]", handlers=[RichHandler()])
logger = logging.getLogger(__name__)
options_to_group = {
"--multi-gpu": "Distributed GPUs",
"--tpu": "TPU",
"--use_deepspeed": "DeepSpeed Arguments",
"--use_fsdp": "FSDP Arguments",
"--use_megatron_lm": "Megatron-LM Arguments",
}
def clean_option(option):
"Finds all cases of - after the first two characters and changes them to _"
if option.startswith("--"):
return option[:3] + option[3:].replace("-", "_")
class _CustomHelpAction(argparse._HelpAction):
"""
This is a custom help action that will hide all arguments that are not used in the command line when the help is
called. This is useful for the case where the user is using a specific platform and only wants to see the arguments
for that platform.
"""
def __call__(self, parser, namespace, values, option_string=None):
if "accelerate" in sys.argv[0] and "launch" in sys.argv[1:]:
args = sys.argv[2:]
else:
args = sys.argv[1:]
opts = parser._actions
titles = [
"Hardware Selection Arguments",
"Resource Selection Arguments",
"Training Paradigm Arguments",
"positional arguments",
"optional arguments",
]
if len(args) > 1:
used_platforms = [arg for arg in args if arg in options_to_group.keys()]
args = list(map(clean_option, args))
used_titles = [options_to_group[o] for o in used_platforms]
for i, arg in enumerate(opts):
# If the argument's container is outside of the used titles, hide it
if arg.container.title not in titles + used_titles:
setattr(opts[i], "help", argparse.SUPPRESS)
# If the argument is hardware selection, but not being passed, hide it
elif arg.container.title == "Hardware Selection Arguments":
if set(arg.option_strings).isdisjoint(set(args)):
setattr(opts[i], "help", argparse.SUPPRESS)
else:
setattr(opts[i], "help", arg.help + " (currently selected)")
# If the argument is a training paradigm, but not being passed, hide it
elif arg.container.title == "Training Paradigm Arguments":
if set(arg.option_strings).isdisjoint(set(used_platforms)):
setattr(opts[i], "help", argparse.SUPPRESS)
else:
setattr(opts[i], "help", arg.help + " (currently selected)")
for i, group in enumerate(list(parser._action_groups)):
# If all arguments in the group are hidden, hide the group
if all([arg.help == argparse.SUPPRESS for arg in group._group_actions]):
parser._action_groups.remove(group)
super().__call__(parser, namespace, values, option_string)
def launch_command_parser(subparsers=None):
if subparsers is not None:
parser = subparsers.add_parser("launch", add_help=False, allow_abbrev=False)
else:
parser = argparse.ArgumentParser("Accelerate launch command", add_help=False, allow_abbrev=False)
parser.register("action", "help", _CustomHelpAction)
parser.add_argument("-h", "--help", action="help", help="Show this help message and exit.")
parser.add_argument(
"--config_file", default=None, help="The config file to use for the default values in the launching script."
)
parser.add_argument(
"--quiet",
"-q",
action="store_true",
help="Silence subprocess errors from the launch stack trace and only show the relevant tracebacks. (Only applicable to DeepSpeed and single-process configurations)",
)
# Hardware selection arguments
hardware_args = parser.add_argument_group(
"Hardware Selection Arguments", "Arguments for selecting the hardware to be used."
)
hardware_args.add_argument(
"--cpu", default=False, action="store_true", help="Whether or not to force the training on the CPU."
)
hardware_args.add_argument(
"--multi_gpu",
default=False,
action="store_true",
help="Whether or not this should launch a distributed GPU training.",
)
hardware_args.add_argument(
"--tpu", default=False, action="store_true", help="Whether or not this should launch a TPU training."
)
hardware_args.add_argument(
"--ipex",
default=False,
action="store_true",
help="Whether or not this should launch a Intel PyTorch Extension (IPEX) training.",
)
# Resource selection arguments
resource_args = parser.add_argument_group(
"Resource Selection Arguments", "Arguments for fine-tuning how available hardware should be used."
)
resource_args.add_argument(
"--mixed_precision",
type=str,
choices=["no", "fp16", "bf16", "fp8"],
help="Whether or not to use mixed precision training. "
"Choose between FP16 and BF16 (bfloat16) training. "
"BF16 training is only supported on Nvidia Ampere GPUs and PyTorch 1.10 or later.",
)
resource_args.add_argument(
"--num_processes", type=int, default=None, help="The total number of processes to be launched in parallel."
)
resource_args.add_argument(
"--num_machines", type=int, default=None, help="The total number of machines used in this training."
)
resource_args.add_argument(
"--num_cpu_threads_per_process",
type=int,
default=None,
help="The number of CPU threads per process. Can be tuned for optimal performance.",
)
# Dynamo arguments
resource_args.add_argument(
"--dynamo_backend",
type=str,
choices=["no"] + [b.lower() for b in DYNAMO_BACKENDS],
help="Choose a backend to optimize your training with dynamo, see more at "
"https://github.com/pytorch/torchdynamo.",
)
resource_args.add_argument(
"--dynamo_mode",
type=str,
default="default",
choices=TORCH_DYNAMO_MODES,
help="Choose a mode to optimize your training with dynamo.",
)
resource_args.add_argument(
"--dynamo_use_fullgraph",
default=False,
action="store_true",
help="Whether to use full graph mode for dynamo or it is ok to break model into several subgraphs",
)
resource_args.add_argument(
"--dynamo_use_dynamic",
default=False,
action="store_true",
help="Whether to enable dynamic shape tracing.",
)
# Training Paradigm arguments
paradigm_args = parser.add_argument_group(
"Training Paradigm Arguments", "Arguments for selecting which training paradigm to be used."
)
paradigm_args.add_argument(
"--use_deepspeed",
default=False,
action="store_true",
help="Whether to use deepspeed.",
)
paradigm_args.add_argument(
"--use_fsdp",
default=False,
action="store_true",
help="Whether to use fsdp.",
)
paradigm_args.add_argument(
"--use_megatron_lm",
default=False,
action="store_true",
help="Whether to use Megatron-LM.",
)
paradigm_args.add_argument(
"--use_xpu",
default=False,
action="store_true",
help="Whether to use IPEX plugin to speed up training on XPU specifically.",
)
# distributed GPU training arguments
distributed_args = parser.add_argument_group("Distributed GPUs", "Arguments related to distributed GPU training.")
distributed_args.add_argument(
"--gpu_ids",
default=None,
help="What GPUs (by id) should be used for training on this machine as a comma-seperated list",
)
distributed_args.add_argument(
"--same_network",
default=False,
action="store_true",
help="Whether all machines used for multinode training exist on the same local network.",
)
distributed_args.add_argument(
"--machine_rank", type=int, default=None, help="The rank of the machine on which this script is launched."
)
distributed_args.add_argument(
"--main_process_ip", type=str, default=None, help="The IP address of the machine of rank 0."
)
distributed_args.add_argument(
"--main_process_port",
type=int,
default=None,
help="The port to use to communicate with the machine of rank 0.",
)
distributed_args.add_argument(
"-t",
"--tee",
default="0",
type=str,
help="Tee std streams into a log file and also to console.",
)
distributed_args.add_argument(
"--role",
type=str,
default="default",
help="User-defined role for the workers.",
)
# Rendezvous related arguments
distributed_args.add_argument(
"--rdzv_backend",
type=str,
default="static",
help="The rendezvous method to use, such as 'static' (the default) or 'c10d'",
)
distributed_args.add_argument(
"--rdzv_conf",
type=str,
default="",
help="Additional rendezvous configuration (<key1>=<value1>,<key2>=<value2>,...).",
)
distributed_args.add_argument(
"--max_restarts",
type=int,
default=0,
help="Maximum number of worker group restarts before failing.",
)
distributed_args.add_argument(
"--monitor_interval",
type=float,
default=5,
help="Interval, in seconds, to monitor the state of workers.",
)
parser.add_argument(
"-m",
"--module",
action="store_true",
help="Change each process to interpret the launch script as a Python module, executing with the same behavior as 'python -m'.",
)
parser.add_argument(
"--no_python",
action="store_true",
help="Skip prepending the training script with 'python' - just execute it directly. Useful when the script is not a Python script.",
)
# TPU arguments
tpu_args = parser.add_argument_group("TPU", "Arguments related to TPU.")
tpu_args.add_argument(
"--tpu_cluster",
action="store_true",
dest="tpu_use_cluster",
help="Whether to use a GCP TPU pod for training.",
)
tpu_args.add_argument(
"--no_tpu_cluster",
action="store_false",
dest="tpu_use_cluster",
help="Should not be passed explicitly, this is for internal use only.",
)
tpu_args.add_argument(
"--tpu_use_sudo",
action="store_true",
help="Whether to use `sudo` when running the TPU training script in each pod.",
)
tpu_args.add_argument(
"--vm",
type=str,
action="append",
help=(
"List of single Compute VM instance names. "
"If not provided we assume usage of instance groups. For TPU pods."
),
)
tpu_args.add_argument(
"--env",
type=str,
action="append",
help="List of environment variables to set on the Compute VM instances. For TPU pods.",
)
tpu_args.add_argument(
"--main_training_function",
type=str,
default=None,
help="The name of the main function to be executed in your script (only for TPU training).",
)
tpu_args.add_argument(
"--downcast_bf16",
action="store_true",
help="Whether when using bf16 precision on TPUs if both float and double tensors are cast to bfloat16 or if double tensors remain as float32.",
)
# DeepSpeed arguments
deepspeed_args = parser.add_argument_group("DeepSpeed Arguments", "Arguments related to DeepSpeed.")
deepspeed_args.add_argument(
"--deepspeed_config_file",
default=None,
type=str,
help="DeepSpeed config file.",
)
deepspeed_args.add_argument(
"--zero_stage",
default=None,
type=int,
help="DeepSpeed's ZeRO optimization stage (useful only when `use_deepspeed` flag is passed). "
"If unspecified, will default to `2`.",
)
deepspeed_args.add_argument(
"--offload_optimizer_device",
default=None,
type=str,
help="Decides where (none|cpu|nvme) to offload optimizer states (useful only when `use_deepspeed` flag is passed). "
"If unspecified, will default to 'none'.",
)
deepspeed_args.add_argument(
"--offload_param_device",
default=None,
type=str,
help="Decides where (none|cpu|nvme) to offload parameters (useful only when `use_deepspeed` flag is passed). "
"If unspecified, will default to 'none'.",
)
deepspeed_args.add_argument(
"--offload_optimizer_nvme_path",
default=None,
type=str,
help="Decides Nvme Path to offload optimizer states (useful only when `use_deepspeed` flag is passed). "
"If unspecified, will default to 'none'.",
)
deepspeed_args.add_argument(
"--offload_param_nvme_path",
default=None,
type=str,
help="Decides Nvme Path to offload parameters (useful only when `use_deepspeed` flag is passed). "
"If unspecified, will default to 'none'.",
)
deepspeed_args.add_argument(
"--gradient_accumulation_steps",
default=None,
type=int,
help="No of gradient_accumulation_steps used in your training script (useful only when `use_deepspeed` flag is passed). "
"If unspecified, will default to `1`.",
)
deepspeed_args.add_argument(
"--gradient_clipping",
default=None,
type=float,
help="gradient clipping value used in your training script (useful only when `use_deepspeed` flag is passed). "
"If unspecified, will default to `1.0`.",
)
deepspeed_args.add_argument(
"--zero3_init_flag",
default=None,
type=str,
help="Decides Whether (true|false) to enable `deepspeed.zero.Init` for constructing massive models. "
"Only applicable with DeepSpeed ZeRO Stage-3. If unspecified, will default to `true`.",
)
deepspeed_args.add_argument(
"--zero3_save_16bit_model",
default=None,
type=str,
help="Decides Whether (true|false) to save 16-bit model weights when using ZeRO Stage-3. "
"Only applicable with DeepSpeed ZeRO Stage-3. If unspecified, will default to `false`.",
)
deepspeed_args.add_argument(
"--deepspeed_hostfile",
default=None,
type=str,
help="DeepSpeed hostfile for configuring multi-node compute resources.",
)
deepspeed_args.add_argument(
"--deepspeed_exclusion_filter",
default=None,
type=str,
help="DeepSpeed exclusion filter string when using mutli-node setup.",
)
deepspeed_args.add_argument(
"--deepspeed_inclusion_filter",
default=None,
type=str,
help="DeepSpeed inclusion filter string when using mutli-node setup.",
)
deepspeed_args.add_argument(
"--deepspeed_multinode_launcher",
default=None,
type=str,
help="DeepSpeed multi-node launcher to use. If unspecified, will default to `pdsh`.",
)
# fsdp arguments
fsdp_args = parser.add_argument_group("FSDP Arguments", "Arguments related to Fully Shared Data Parallelism.")
fsdp_args.add_argument(
"--fsdp_offload_params",
default="false",
type=str,
help="Decides Whether (true|false) to offload parameters and gradients to CPU. (useful only when `use_fsdp` flag is passed).",
)
fsdp_args.add_argument(
"--fsdp_min_num_params",
type=int,
default=1e8,
help="FSDP's minimum number of parameters for Default Auto Wrapping. (useful only when `use_fsdp` flag is passed).",
)
fsdp_args.add_argument(
"--fsdp_sharding_strategy",
type=str,
default="FULL_SHARD",
help="FSDP's Sharding Strategy. (useful only when `use_fsdp` flag is passed).",
)
fsdp_args.add_argument(
"--fsdp_auto_wrap_policy",
type=str,
default=None,
help="FSDP's auto wrap policy. (useful only when `use_fsdp` flag is passed).",
)
fsdp_args.add_argument(
"--fsdp_transformer_layer_cls_to_wrap",
default=None,
type=str,
help="Transformer layer class name (case-sensitive) to wrap ,e.g, `BertLayer`, `GPTJBlock`, `T5Block` .... "
"(useful only when `use_fsdp` flag is passed).",
)
fsdp_args.add_argument(
"--fsdp_backward_prefetch_policy",
default=None,
type=str,
help="This argument is deprecated and will be removed in version 0.27.0 of 🤗 Accelerate. Use `fsdp_backward_prefetch` instead.",
)
fsdp_args.add_argument(
"--fsdp_backward_prefetch",
default=None,
type=str,
help="FSDP's backward prefetch policy. (useful only when `use_fsdp` flag is passed).",
)
fsdp_args.add_argument(
"--fsdp_state_dict_type",
default=None,
type=str,
help="FSDP's state dict type. (useful only when `use_fsdp` flag is passed).",
)
fsdp_args.add_argument(
"--fsdp_forward_prefetch",
default="false",
type=str,
help="If True, then FSDP explicitly prefetches the next upcoming "
"all-gather while executing in the forward pass (useful only when `use_fsdp` flag is passed).",
)
fsdp_args.add_argument(
"--fsdp_use_orig_params",
default="true",
type=str,
help="If True, allows non-uniform `requires_grad` during init, which means support for interspersed frozen and trainable paramteres."
" (useful only when `use_fsdp` flag is passed).",
)
fsdp_args.add_argument(
"--fsdp_cpu_ram_efficient_loading",
default="true",
type=str,
help="If True, only the first process loads the pretrained model checkoint while all other processes have empty weights. "
"Only applicable for 🤗 Transformers. When using this, `--fsdp_sync_module_states` needs to True. "
"(useful only when `use_fsdp` flag is passed).",
)
fsdp_args.add_argument(
"--fsdp_sync_module_states",
default="true",
type=str,
help="If True, each individually wrapped FSDP unit will broadcast module parameters from rank 0."
" (useful only when `use_fsdp` flag is passed).",
)
# megatron_lm args
megatron_lm_args = parser.add_argument_group("Megatron-LM Arguments", "Arguments related to Megatron-LM.")
megatron_lm_args.add_argument(
"--megatron_lm_tp_degree",
type=int,
default=1,
help="Megatron-LM's Tensor Parallelism (TP) degree. (useful only when `use_megatron_lm` flag is passed).",
)
megatron_lm_args.add_argument(
"--megatron_lm_pp_degree",
type=int,
default=1,
help="Megatron-LM's Pipeline Parallelism (PP) degree. (useful only when `use_megatron_lm` flag is passed).",
)
megatron_lm_args.add_argument(
"--megatron_lm_num_micro_batches",
type=int,
default=None,
help="Megatron-LM's number of micro batches when PP degree > 1. (useful only when `use_megatron_lm` flag is passed).",
)
megatron_lm_args.add_argument(
"--megatron_lm_sequence_parallelism",
default=None,
type=str,
help="Decides Whether (true|false) to enable Sequence Parallelism when TP degree > 1. "
"(useful only when `use_megatron_lm` flag is passed).",
)
megatron_lm_args.add_argument(
"--megatron_lm_recompute_activations",
default=None,
type=str,
help="Decides Whether (true|false) to enable Selective Activation Recomputation. "
"(useful only when `use_megatron_lm` flag is passed).",
)
megatron_lm_args.add_argument(
"--megatron_lm_use_distributed_optimizer",
default=None,
type=str,
help="Decides Whether (true|false) to use distributed optimizer "
"which shards optimizer state and gradients across Data Pralellel (DP) ranks. "
"(useful only when `use_megatron_lm` flag is passed).",
)
megatron_lm_args.add_argument(
"--megatron_lm_gradient_clipping",
default=1.0,
type=float,
help="Megatron-LM's gradient clipping value based on global L2 Norm (0 to disable). "
"(useful only when `use_megatron_lm` flag is passed).",
)
# AWS arguments
aws_args = parser.add_argument_group("AWS Arguments", "Arguments related to AWS.")
aws_args.add_argument(
"--aws_access_key_id",
type=str,
default=None,
help="The AWS_ACCESS_KEY_ID used to launch the Amazon SageMaker training job",
)
aws_args.add_argument(
"--aws_secret_access_key",
type=str,
default=None,
help="The AWS_SECRET_ACCESS_KEY used to launch the Amazon SageMaker training job.",
)
parser.add_argument(
"--debug",
action="store_true",
help="Whether to print out the torch.distributed stack trace when something fails.",
)
parser.add_argument(
"training_script",
type=str,
help=(
"The full path to the script to be launched in parallel, followed by all the arguments for the training "
"script."
),
)
# Other arguments of the training scripts
parser.add_argument("training_script_args", nargs=argparse.REMAINDER, help="Arguments of the training script.")
if subparsers is not None:
parser.set_defaults(func=launch_command)
return parser
def simple_launcher(args):
cmd, current_env = prepare_simple_launcher_cmd_env(args)
process = subprocess.Popen(cmd, env=current_env)
process.wait()
if process.returncode != 0:
if not args.quiet:
raise subprocess.CalledProcessError(returncode=process.returncode, cmd=cmd)
else:
sys.exit(1)
def multi_gpu_launcher(args):
import torch.distributed.run as distrib_run
current_env = prepare_multi_gpu_env(args)
if not check_cuda_p2p_ib_support():
message = "Using RTX 4000 series which doesn't support faster communication speedups. Ensuring P2P and IB communications are disabled."
warn = False
if "NCCL_P2P_DISABLE" not in current_env:
current_env["NCCL_P2P_DISABLE"] = "1"
warn = True
if "NCCL_IB_DISABLE" not in current_env:
current_env["NCCL_IB_DISABLE"] = "1"
warn = True
if warn:
logger.warning(message)
debug = getattr(args, "debug", False)
args = _filter_args(
args,
distrib_run.get_args_parser(),
["--training_script", args.training_script, "--training_script_args", args.training_script_args],
)
with patch_environment(**current_env):
try:
distrib_run.run(args)
except Exception:
if is_rich_available() and debug:
console = get_console()
console.print("\n[bold red]Using --debug, `torch.distributed` Stack Trace:[/bold red]")
console.print_exception(suppress=[__file__], show_locals=False)
else:
raise
def deepspeed_launcher(args):
import torch.distributed.run as distrib_run
if not is_deepspeed_available():
raise ImportError("DeepSpeed is not installed => run `pip3 install deepspeed` or build it from source.")
cmd, current_env = prepare_deepspeed_cmd_env(args)
if not check_cuda_p2p_ib_support():
message = "Using RTX 4000 series which doesn't support faster communication speedups. Ensuring P2P and IB communications are disabled."
warn = False
if "NCCL_P2P_DISABLE" not in current_env:
current_env["NCCL_P2P_DISABLE"] = "1"
warn = True
if "NCCL_IB_DISABLE" not in current_env:
current_env["NCCL_IB_DISABLE"] = "1"
warn = True
if warn:
logger.warning(message)
if args.num_machines > 1 and args.deepspeed_multinode_launcher != DEEPSPEED_MULTINODE_LAUNCHERS[1]:
with open(".deepspeed_env", "a") as f:
for key, value in current_env.items():
if ";" in value or " " in value:
continue
f.write(f"{key}={value}\n")
process = subprocess.Popen(cmd, env=current_env)
process.wait()
if process.returncode != 0:
if not args.quiet:
raise subprocess.CalledProcessError(returncode=process.returncode, cmd=cmd)
else:
sys.exit(1)
else:
debug = getattr(args, "debug", False)
args = _filter_args(
args,
distrib_run.get_args_parser(),
["--training_script", args.training_script, "--training_script_args", args.training_script_args],
)
with patch_environment(**current_env):
try:
distrib_run.run(args)
except Exception:
if is_rich_available() and debug:
console = get_console()
console.print("\n[bold red]Using --debug, `torch.distributed` Stack Trace:[/bold red]")
console.print_exception(suppress=[__file__], show_locals=False)
else:
raise
def tpu_launcher(args):
import torch_xla.distributed.xla_multiprocessing as xmp
if args.no_python:
raise ValueError("--no_python cannot be used with TPU launcher")
args, current_env = prepare_tpu(args, {})
if args.module:
mod_name = args.training_script
else:
# Import training_script as a module
script_path = Path(args.training_script)
sys.path.append(str(script_path.parent.resolve()))
mod_name = script_path.stem
mod = importlib.import_module(mod_name)
if not hasattr(mod, args.main_training_function):
raise ValueError(
f"Your training script should have a function named {args.main_training_function}, or you should pass a "
"different value to `--main_training_function`."
)
# Patch sys.argv
sys.argv = [mod.__file__] + args.training_script_args
main_function = getattr(mod, args.main_training_function)
with patch_environment(**current_env):
xmp.spawn(PrepareForLaunch(main_function), args=(), nprocs=args.num_processes)
def tpu_pod_launcher(args):
from torch_xla.distributed import xla_dist
current_env = {}
args, current_env = prepare_tpu(args, current_env, True)
debug = getattr(args, "debug", False)
training_script = args.training_script
training_script_args = args.training_script_args
new_args = _filter_args(
args, xla_dist.get_args_parser(), ["--tpu", args.tpu_name, "--positional", "", "--restart-tpuvm-pod-server"]
)
if args.tpu_use_sudo:
new_cmd = ["sudo"]
else:
new_cmd = []
new_cmd += [
"accelerate-launch",
"--tpu",
"--no_tpu_cluster",
"--num_machines",
"1",
"--mixed_precision",
"no",
"--dynamo_backend",
"no",
"--num_processes",
str(args.num_processes),
"--main_training_function",
str(args.main_training_function),
training_script,
] + training_script_args
new_args.positional = new_cmd
bad_flags = ""
for arg in vars(new_args):
if arg.startswith("docker_"):
value = getattr(new_args, arg)
if value != "" and value is not None:
bad_flags += f'{arg}="{value}"\n'
if bad_flags != "":
raise ValueError(
f"Docker containers are not supported for TPU pod launcher currently, please remove the following flags:\n{bad_flags}"
)
new_args.env = [f"{k}={v}" for k, v in current_env.items()]
new_args.env.append("ACCELERATE_IN_TPU_POD=1")
try:
xla_dist.resolve_and_execute(new_args)
except Exception:
if is_rich_available() and debug:
console = get_console()
console.print("\n[bold red]Using --debug, `torch_xla.xla_dist` Stack Trace:[/bold red]")
console.print_exception(suppress=[__file__], show_locals=False)
else:
raise
def sagemaker_launcher(sagemaker_config: SageMakerConfig, args):
if not is_sagemaker_available():
raise ImportError(
"Please install sagemaker to be able to launch training on Amazon SageMaker with `pip install accelerate[sagemaker]`"
)
if args.module or args.no_python:
raise ValueError(
"SageMaker requires a python training script file and cannot be used with --module or --no_python"
)
from sagemaker.huggingface import HuggingFace
args, sagemaker_inputs = prepare_sagemager_args_inputs(sagemaker_config, args)
huggingface_estimator = HuggingFace(**args)
huggingface_estimator.fit(inputs=sagemaker_inputs)
print(f"You can find your model data at: {huggingface_estimator.model_data}")
def _validate_launch_command(args):
# Sanity checks
if sum([args.multi_gpu, args.cpu, args.tpu, args.use_deepspeed, args.use_fsdp]) > 1:
raise ValueError(
"You can only use one of `--cpu`, `--multi_gpu`, `--tpu`, `--use_deepspeed`, `--use_fsdp` at a time."
)
if args.multi_gpu and (args.num_processes is not None) and (args.num_processes < 2):
raise ValueError("You need to use at least 2 processes to use `--multi_gpu`.")
defaults = None
warned = []
mp_from_config_flag = False
# Get the default from the config file.
if args.config_file is not None or os.path.isfile(default_config_file) and not args.cpu:
defaults = load_config_from_file(args.config_file)
if (
not args.multi_gpu
and not args.tpu
and not args.tpu_use_cluster
and not args.use_deepspeed
and not args.use_fsdp
and not args.use_megatron_lm
):
args.use_deepspeed = defaults.distributed_type == DistributedType.DEEPSPEED
args.multi_gpu = (
True
if defaults.distributed_type
in (DistributedType.MULTI_GPU, DistributedType.MULTI_NPU, DistributedType.MULTI_XPU)
else False
)
args.tpu = defaults.distributed_type == DistributedType.TPU
args.use_fsdp = defaults.distributed_type == DistributedType.FSDP
args.use_megatron_lm = defaults.distributed_type == DistributedType.MEGATRON_LM
args.tpu_use_cluster = defaults.tpu_use_cluster if args.tpu else False
if args.gpu_ids is None:
if defaults.gpu_ids is not None:
args.gpu_ids = defaults.gpu_ids
else:
args.gpu_ids = "all"
if args.multi_gpu and args.num_machines is None:
args.num_machines = defaults.num_machines
if len(args.gpu_ids.split(",")) < 2 and (args.gpu_ids != "all") and args.multi_gpu and args.num_machines <= 1:
raise ValueError(
"Less than two GPU ids were configured and tried to run on on multiple GPUs. "
"Please ensure at least two are specified for `--gpu_ids`, or use `--gpu_ids='all'`."
)
if defaults.compute_environment == ComputeEnvironment.LOCAL_MACHINE:
# Update args with the defaults
for name, attr in defaults.__dict__.items():
if isinstance(attr, dict):
for k in defaults.deepspeed_config:
setattr(args, k, defaults.deepspeed_config[k])
for k in defaults.fsdp_config:
arg_to_set = k
if "fsdp" not in arg_to_set:
arg_to_set = "fsdp_" + arg_to_set
setattr(args, arg_to_set, defaults.fsdp_config[k])
for k in defaults.megatron_lm_config:
setattr(args, k, defaults.megatron_lm_config[k])
for k in defaults.dynamo_config:
setattr(args, k, defaults.dynamo_config[k])
for k in defaults.ipex_config:
setattr(args, k, defaults.ipex_config[k])
continue
# Those args are handled separately
if (
name not in ["compute_environment", "mixed_precision", "distributed_type"]
and getattr(args, name, None) is None
):
setattr(args, name, attr)
if not args.debug:
args.debug = defaults.debug
if not args.mixed_precision:
if defaults.mixed_precision is None:
args.mixed_precision = "no"
else:
args.mixed_precision = defaults.mixed_precision
mp_from_config_flag = True
else:
native_amp = False
err = "{mode} mixed precision requires {requirement}"
if args.use_cpu or (args.use_xpu and torch.xpu.is_available()):
native_amp = is_torch_version(">=", "1.10")
else:
native_amp = is_bf16_available(True)
if args.mixed_precision == "bf16" and not native_amp and not (args.tpu and is_tpu_available()):
raise ValueError(err.format(mode="bf16", requirement="PyTorch >= 1.10 and a supported device."))
# Silently set the default here
if args.dynamo_backend is None:
args.dynamo_backend = "no"
else:
if args.num_processes is None:
if args.use_xpu and is_xpu_available():
args.num_processes = torch.xpu.device_count()
elif is_npu_available():
args.num_processes = torch.npu.device_count()
else:
args.num_processes = torch.cuda.device_count()
warned.append(f"\t`--num_processes` was set to a value of `{args.num_processes}`")
if args.debug is None:
args.debug = False
if not args.multi_gpu and (
(args.use_xpu and is_xpu_available() and torch.xpu.device_count() > 1)
or (is_npu_available() and torch.npu.device_count() > 1)
or (torch.cuda.device_count() > 1)
):
warned.append(
"\t\tMore than one GPU was found, enabling multi-GPU training.\n"
"\t\tIf this was unintended please pass in `--num_processes=1`."
)
args.multi_gpu = True
if args.num_machines is None:
warned.append("\t`--num_machines` was set to a value of `1`")
args.num_machines = 1
if args.mixed_precision is None:
warned.append("\t`--mixed_precision` was set to a value of `'no'`")
args.mixed_precision = "no"
if not hasattr(args, "use_cpu"):
args.use_cpu = args.cpu
if args.dynamo_backend is None:
warned.append("\t`--dynamo_backend` was set to a value of `'no'`")
args.dynamo_backend = "no"
if args.debug:
logger.debug("Running script in debug mode, expect distributed operations to be slightly slower.")
is_aws_env_disabled = defaults is None or (
defaults is not None and defaults.compute_environment != ComputeEnvironment.AMAZON_SAGEMAKER
)
if is_aws_env_disabled and args.num_cpu_threads_per_process is None:
args.num_cpu_threads_per_process = 1
if args.use_cpu and args.num_processes >= 1:
local_size = get_int_from_env(
["MPI_LOCALNRANKS", "OMPI_COMM_WORLD_LOCAL_SIZE", "MV2_COMM_WORLD_LOCAL_SIZE"], 1
)
threads_per_process = int(psutil.cpu_count(logical=False) / local_size)
if threads_per_process > 1:
args.num_cpu_threads_per_process = threads_per_process
warned.append(
f"\t`--num_cpu_threads_per_process` was set to `{args.num_cpu_threads_per_process}` to improve out-of-box performance when training on CPUs"
)
if any(warned):
message = "The following values were not passed to `accelerate launch` and had defaults used instead:\n"
message += "\n".join(warned)
message += (
"\nTo avoid this warning pass in values for each of the problematic parameters or run `accelerate config`."
)
logger.warning(message)
return args, defaults, mp_from_config_flag
def launch_command(args):
args, defaults, mp_from_config_flag = _validate_launch_command(args)
# Use the proper launcher
if args.use_deepspeed and not args.cpu:
args.deepspeed_fields_from_accelerate_config = list(defaults.deepspeed_config.keys()) if defaults else []
if mp_from_config_flag:
args.deepspeed_fields_from_accelerate_config.append("mixed_precision")
args.deepspeed_fields_from_accelerate_config = ",".join(args.deepspeed_fields_from_accelerate_config)
deepspeed_launcher(args)
elif args.use_fsdp and not args.cpu:
multi_gpu_launcher(args)
elif args.use_megatron_lm and not args.cpu:
multi_gpu_launcher(args)
elif args.multi_gpu and not args.cpu:
multi_gpu_launcher(args)
elif args.tpu and not args.cpu:
if args.tpu_use_cluster:
tpu_pod_launcher(args)
else:
tpu_launcher(args)
elif defaults is not None and defaults.compute_environment == ComputeEnvironment.AMAZON_SAGEMAKER:
sagemaker_launcher(defaults, args)
else:
simple_launcher(args)
def main():
parser = launch_command_parser()
args = parser.parse_args()
launch_command(args)
if __name__ == "__main__":
main()
| accelerate/src/accelerate/commands/launch.py/0 | {
"file_path": "accelerate/src/accelerate/commands/launch.py",
"repo_id": "accelerate",
"token_count": 17301
} | 5 |
# Copyright 2022 The HuggingFace Team. All rights reserved.
#
# Licensed under the Apache License, Version 2.0 (the "License");
# you may not use this file except in compliance with the License.
# You may obtain a copy of the License at
#
# http://www.apache.org/licenses/LICENSE-2.0
#
# Unless required by applicable law or agreed to in writing, software
# distributed under the License is distributed on an "AS IS" BASIS,
# WITHOUT WARRANTIES OR CONDITIONS OF ANY KIND, either express or implied.
# See the License for the specific language governing permissions and
# limitations under the License.
# We ignore warnings about stepping the scheduler since we step it ourselves during gradient accumulation
import warnings
from .state import AcceleratorState, GradientState
warnings.filterwarnings("ignore", category=UserWarning, module="torch.optim.lr_scheduler")
class AcceleratedScheduler:
"""
A wrapper around a learning rate scheduler that will only step when the optimizer(s) have a training step. Useful
to avoid making a scheduler step too fast when gradients went overflow and there was no training step (in mixed
precision training)
When performing gradient accumulation scheduler lengths should not be changed accordingly, Accelerate will always
step the scheduler to account for it.
Args:
scheduler (`torch.optim.lr_scheduler._LRScheduler`):
The scheduler to wrap.
optimizers (one or a list of `torch.optim.Optimizer`):
The optimizers used.
step_with_optimizer (`bool`, *optional*, defaults to `True`):
Whether or not the scheduler should be stepped at each optimizer step.
split_batches (`bool`, *optional*, defaults to `False`):
Whether or not the dataloaders split one batch across the different processes (so batch size is the same
regardless of the number of processes) or create batches on each process (so batch size is the original
batch size multiplied by the number of processes).
"""
def __init__(self, scheduler, optimizers, step_with_optimizer: bool = True, split_batches: bool = False):
self.scheduler = scheduler
self.optimizers = optimizers if isinstance(optimizers, (list, tuple)) else [optimizers]
self.split_batches = split_batches
self.step_with_optimizer = step_with_optimizer
self.gradient_state = GradientState()
def step(self, *args, **kwargs):
if not self.step_with_optimizer:
# No link between scheduler and optimizer -> just step
self.scheduler.step(*args, **kwargs)
return
# Otherwise, first make sure the optimizer was stepped.
if not self.gradient_state.sync_gradients:
if self.gradient_state.adjust_scheduler:
self.scheduler._step_count += 1
return
for opt in self.optimizers:
if opt.step_was_skipped:
return
if self.split_batches:
# Split batches -> the training dataloader batch size is not changed so one step per training step
self.scheduler.step(*args, **kwargs)
else:
# Otherwise the training dataloader batch size was multiplied by `num_processes`, so we need to do
# num_processes steps per training step
num_processes = AcceleratorState().num_processes
for _ in range(num_processes):
# Special case when using OneCycle and `drop_last` was not used
if hasattr(self.scheduler, "total_steps"):
if self.scheduler._step_count <= self.scheduler.total_steps:
self.scheduler.step(*args, **kwargs)
else:
self.scheduler.step(*args, **kwargs)
# Passthroughs
def get_last_lr(self):
return self.scheduler.get_last_lr()
def state_dict(self):
return self.scheduler.state_dict()
def load_state_dict(self, state_dict):
self.scheduler.load_state_dict(state_dict)
def get_lr(self):
return self.scheduler.get_lr()
def print_lr(self, *args, **kwargs):
return self.scheduler.print_lr(*args, **kwargs)
| accelerate/src/accelerate/scheduler.py/0 | {
"file_path": "accelerate/src/accelerate/scheduler.py",
"repo_id": "accelerate",
"token_count": 1577
} | 6 |
# Copyright 2021 The HuggingFace Team. All rights reserved.
#
# Licensed under the Apache License, Version 2.0 (the "License");
# you may not use this file except in compliance with the License.
# You may obtain a copy of the License at
#
# http://www.apache.org/licenses/LICENSE-2.0
#
# Unless required by applicable law or agreed to in writing, software
# distributed under the License is distributed on an "AS IS" BASIS,
# WITHOUT WARRANTIES OR CONDITIONS OF ANY KIND, either express or implied.
# See the License for the specific language governing permissions and
# limitations under the License.
import asyncio
import os
import shutil
import subprocess
import sys
import tempfile
import unittest
from contextlib import contextmanager
from functools import partial
from pathlib import Path
from typing import List, Union
from unittest import mock
import torch
from ..state import AcceleratorState, PartialState
from ..utils import (
gather,
is_bnb_available,
is_clearml_available,
is_comet_ml_available,
is_cuda_available,
is_datasets_available,
is_deepspeed_available,
is_dvclive_available,
is_mps_available,
is_npu_available,
is_pandas_available,
is_tensorboard_available,
is_timm_available,
is_torch_version,
is_tpu_available,
is_transformers_available,
is_wandb_available,
is_xpu_available,
str_to_bool,
)
def get_backend():
if is_cuda_available():
return "cuda", torch.cuda.device_count(), torch.cuda.memory_allocated
elif is_mps_available():
return "mps", 1, torch.mps.current_allocated_memory()
elif is_npu_available():
return "npu", torch.npu.device_count(), torch.npu.memory_allocated
elif is_xpu_available():
return "xpu", torch.xpu.device_count(), torch.xpu.memory_allocated
else:
return "cpu", 1, 0
torch_device, device_count, memory_allocated_func = get_backend()
def parse_flag_from_env(key, default=False):
try:
value = os.environ[key]
except KeyError:
# KEY isn't set, default to `default`.
_value = default
else:
# KEY is set, convert it to True or False.
try:
_value = str_to_bool(value)
except ValueError:
# More values are supported, but let's keep the message simple.
raise ValueError(f"If set, {key} must be yes or no.")
return _value
_run_slow_tests = parse_flag_from_env("RUN_SLOW", default=False)
def skip(test_case):
"Decorator that skips a test unconditionally"
return unittest.skip("Test was skipped")(test_case)
def slow(test_case):
"""
Decorator marking a test as slow. Slow tests are skipped by default. Set the RUN_SLOW environment variable to a
truthy value to run them.
"""
return unittest.skipUnless(_run_slow_tests, "test is slow")(test_case)
def require_cpu(test_case):
"""
Decorator marking a test that must be only ran on the CPU. These tests are skipped when a GPU is available.
"""
return unittest.skipUnless(torch_device == "cpu", "test requires only a CPU")(test_case)
def require_non_cpu(test_case):
"""
Decorator marking a test that requires a hardware accelerator backend. These tests are skipped when there are no
hardware accelerator available.
"""
return unittest.skipUnless(torch_device != "cpu", "test requires a GPU")(test_case)
def require_cuda(test_case):
"""
Decorator marking a test that requires CUDA. These tests are skipped when there are no GPU available.
"""
return unittest.skipUnless(is_cuda_available(), "test requires a GPU")(test_case)
def require_xpu(test_case):
"""
Decorator marking a test that requires XPU. These tests are skipped when there are no XPU available.
"""
return unittest.skipUnless(is_xpu_available(), "test requires a XPU")(test_case)
def require_mps(test_case):
"""
Decorator marking a test that requires MPS backend. These tests are skipped when torch doesn't support `mps`
backend.
"""
return unittest.skipUnless(is_mps_available(), "test requires a `mps` backend support in `torch`")(test_case)
def require_huggingface_suite(test_case):
"""
Decorator marking a test that requires transformers and datasets. These tests are skipped when they are not.
"""
return unittest.skipUnless(
is_transformers_available() and is_datasets_available(), "test requires the Hugging Face suite"
)(test_case)
def require_transformers(test_case):
"""
Decorator marking a test that requires transformers. These tests are skipped when they are not.
"""
return unittest.skipUnless(is_transformers_available(), "test requires the transformers library")(test_case)
def require_timm(test_case):
"""
Decorator marking a test that requires transformers. These tests are skipped when they are not.
"""
return unittest.skipUnless(is_timm_available(), "test requires the timm library")(test_case)
def require_bnb(test_case):
"""
Decorator marking a test that requires bitsandbytes. These tests are skipped when they are not.
"""
return unittest.skipUnless(is_bnb_available(), "test requires the bitsandbytes library")(test_case)
def require_tpu(test_case):
"""
Decorator marking a test that requires TPUs. These tests are skipped when there are no TPUs available.
"""
return unittest.skipUnless(is_tpu_available(), "test requires TPU")(test_case)
def require_single_device(test_case):
"""
Decorator marking a test that requires a single device. These tests are skipped when there is no hardware
accelerator available or number of devices is more than one.
"""
return unittest.skipUnless(torch_device != "cpu" and device_count == 1, "test requires a hardware accelerator")(
test_case
)
def require_single_gpu(test_case):
"""
Decorator marking a test that requires CUDA on a single GPU. These tests are skipped when there are no GPU
available or number of GPUs is more than one.
"""
return unittest.skipUnless(torch.cuda.device_count() == 1, "test requires a GPU")(test_case)
def require_single_xpu(test_case):
"""
Decorator marking a test that requires CUDA on a single XPU. These tests are skipped when there are no XPU
available or number of xPUs is more than one.
"""
return unittest.skipUnless(torch.xpu.device_count() == 1, "test requires a XPU")(test_case)
def require_multi_device(test_case):
"""
Decorator marking a test that requires a multi-device setup. These tests are skipped on a machine without multiple
devices.
"""
return unittest.skipUnless(device_count > 1, "test requires multiple hardware accelerators")(test_case)
def require_multi_gpu(test_case):
"""
Decorator marking a test that requires a multi-GPU setup. These tests are skipped on a machine without multiple
GPUs.
"""
return unittest.skipUnless(torch.cuda.device_count() > 1, "test requires multiple GPUs")(test_case)
def require_multi_xpu(test_case):
"""
Decorator marking a test that requires a multi-XPU setup. These tests are skipped on a machine without multiple
XPUs.
"""
return unittest.skipUnless(torch.xpu.device_count() > 1, "test requires multiple XPUs")(test_case)
def require_deepspeed(test_case):
"""
Decorator marking a test that requires DeepSpeed installed. These tests are skipped when DeepSpeed isn't installed
"""
return unittest.skipUnless(is_deepspeed_available(), "test requires DeepSpeed")(test_case)
def require_fsdp(test_case):
"""
Decorator marking a test that requires FSDP installed. These tests are skipped when FSDP isn't installed
"""
return unittest.skipUnless(is_torch_version(">=", "1.12.0"), "test requires torch version >= 1.12.0")(test_case)
def require_torch_min_version(test_case=None, version=None):
"""
Decorator marking that a test requires a particular torch version to be tested. These tests are skipped when an
installed torch version is less than the required one.
"""
if test_case is None:
return partial(require_torch_min_version, version=version)
return unittest.skipUnless(is_torch_version(">=", version), f"test requires torch version >= {version}")(test_case)
def require_tensorboard(test_case):
"""
Decorator marking a test that requires tensorboard installed. These tests are skipped when tensorboard isn't
installed
"""
return unittest.skipUnless(is_tensorboard_available(), "test requires Tensorboard")(test_case)
def require_wandb(test_case):
"""
Decorator marking a test that requires wandb installed. These tests are skipped when wandb isn't installed
"""
return unittest.skipUnless(is_wandb_available(), "test requires wandb")(test_case)
def require_comet_ml(test_case):
"""
Decorator marking a test that requires comet_ml installed. These tests are skipped when comet_ml isn't installed
"""
return unittest.skipUnless(is_comet_ml_available(), "test requires comet_ml")(test_case)
def require_clearml(test_case):
"""
Decorator marking a test that requires clearml installed. These tests are skipped when clearml isn't installed
"""
return unittest.skipUnless(is_clearml_available(), "test requires clearml")(test_case)
def require_dvclive(test_case):
"""
Decorator marking a test that requires dvclive installed. These tests are skipped when dvclive isn't installed
"""
return unittest.skipUnless(is_dvclive_available(), "test requires dvclive")(test_case)
def require_pandas(test_case):
"""
Decorator marking a test that requires pandas installed. These tests are skipped when pandas isn't installed
"""
return unittest.skipUnless(is_pandas_available(), "test requires pandas")(test_case)
_atleast_one_tracker_available = (
any([is_wandb_available(), is_tensorboard_available()]) and not is_comet_ml_available()
)
def require_trackers(test_case):
"""
Decorator marking that a test requires at least one tracking library installed. These tests are skipped when none
are installed
"""
return unittest.skipUnless(
_atleast_one_tracker_available,
"test requires at least one tracker to be available and for `comet_ml` to not be installed",
)(test_case)
class TempDirTestCase(unittest.TestCase):
"""
A TestCase class that keeps a single `tempfile.TemporaryDirectory` open for the duration of the class, wipes its
data at the start of a test, and then destroyes it at the end of the TestCase.
Useful for when a class or API requires a single constant folder throughout it's use, such as Weights and Biases
The temporary directory location will be stored in `self.tmpdir`
"""
clear_on_setup = True
@classmethod
def setUpClass(cls):
"Creates a `tempfile.TemporaryDirectory` and stores it in `cls.tmpdir`"
cls.tmpdir = tempfile.mkdtemp()
@classmethod
def tearDownClass(cls):
"Remove `cls.tmpdir` after test suite has finished"
if os.path.exists(cls.tmpdir):
shutil.rmtree(cls.tmpdir)
def setUp(self):
"Destroy all contents in `self.tmpdir`, but not `self.tmpdir`"
if self.clear_on_setup:
for path in Path(self.tmpdir).glob("**/*"):
if path.is_file():
path.unlink()
elif path.is_dir():
shutil.rmtree(path)
class AccelerateTestCase(unittest.TestCase):
"""
A TestCase class that will reset the accelerator state at the end of every test. Every test that checks or utilizes
the `AcceleratorState` class should inherit from this to avoid silent failures due to state being shared between
tests.
"""
def tearDown(self):
super().tearDown()
# Reset the state of the AcceleratorState singleton.
AcceleratorState._reset_state()
PartialState._reset_state()
class MockingTestCase(unittest.TestCase):
"""
A TestCase class designed to dynamically add various mockers that should be used in every test, mimicking the
behavior of a class-wide mock when defining one normally will not do.
Useful when a mock requires specific information available only initialized after `TestCase.setUpClass`, such as
setting an environment variable with that information.
The `add_mocks` function should be ran at the end of a `TestCase`'s `setUp` function, after a call to
`super().setUp()` such as:
```python
def setUp(self):
super().setUp()
mocks = mock.patch.dict(os.environ, {"SOME_ENV_VAR", "SOME_VALUE"})
self.add_mocks(mocks)
```
"""
def add_mocks(self, mocks: Union[mock.Mock, List[mock.Mock]]):
"""
Add custom mocks for tests that should be repeated on each test. Should be called during
`MockingTestCase.setUp`, after `super().setUp()`.
Args:
mocks (`mock.Mock` or list of `mock.Mock`):
Mocks that should be added to the `TestCase` after `TestCase.setUpClass` has been run
"""
self.mocks = mocks if isinstance(mocks, (tuple, list)) else [mocks]
for m in self.mocks:
m.start()
self.addCleanup(m.stop)
def are_the_same_tensors(tensor):
state = AcceleratorState()
tensor = tensor[None].clone().to(state.device)
tensors = gather(tensor).cpu()
tensor = tensor[0].cpu()
for i in range(tensors.shape[0]):
if not torch.equal(tensors[i], tensor):
return False
return True
class _RunOutput:
def __init__(self, returncode, stdout, stderr):
self.returncode = returncode
self.stdout = stdout
self.stderr = stderr
async def _read_stream(stream, callback):
while True:
line = await stream.readline()
if line:
callback(line)
else:
break
async def _stream_subprocess(cmd, env=None, stdin=None, timeout=None, quiet=False, echo=False) -> _RunOutput:
if echo:
print("\nRunning: ", " ".join(cmd))
p = await asyncio.create_subprocess_exec(
cmd[0],
*cmd[1:],
stdin=stdin,
stdout=asyncio.subprocess.PIPE,
stderr=asyncio.subprocess.PIPE,
env=env,
)
# note: there is a warning for a possible deadlock when using `wait` with huge amounts of data in the pipe
# https://docs.python.org/3/library/asyncio-subprocess.html#asyncio.asyncio.subprocess.Process.wait
#
# If it starts hanging, will need to switch to the following code. The problem is that no data
# will be seen until it's done and if it hangs for example there will be no debug info.
# out, err = await p.communicate()
# return _RunOutput(p.returncode, out, err)
out = []
err = []
def tee(line, sink, pipe, label=""):
line = line.decode("utf-8").rstrip()
sink.append(line)
if not quiet:
print(label, line, file=pipe)
# XXX: the timeout doesn't seem to make any difference here
await asyncio.wait(
[
asyncio.create_task(_read_stream(p.stdout, lambda l: tee(l, out, sys.stdout, label="stdout:"))),
asyncio.create_task(_read_stream(p.stderr, lambda l: tee(l, err, sys.stderr, label="stderr:"))),
],
timeout=timeout,
)
return _RunOutput(await p.wait(), out, err)
def execute_subprocess_async(cmd, env=None, stdin=None, timeout=180, quiet=False, echo=True) -> _RunOutput:
loop = asyncio.get_event_loop()
result = loop.run_until_complete(
_stream_subprocess(cmd, env=env, stdin=stdin, timeout=timeout, quiet=quiet, echo=echo)
)
cmd_str = " ".join(cmd)
if result.returncode > 0:
stderr = "\n".join(result.stderr)
raise RuntimeError(
f"'{cmd_str}' failed with returncode {result.returncode}\n\n"
f"The combined stderr from workers follows:\n{stderr}"
)
return result
class SubprocessCallException(Exception):
pass
def run_command(command: List[str], return_stdout=False, env=None):
"""
Runs `command` with `subprocess.check_output` and will potentially return the `stdout`. Will also properly capture
if an error occured while running `command`
"""
if env is None:
env = os.environ.copy()
try:
output = subprocess.check_output(command, stderr=subprocess.STDOUT, env=env)
if return_stdout:
if hasattr(output, "decode"):
output = output.decode("utf-8")
return output
except subprocess.CalledProcessError as e:
raise SubprocessCallException(
f"Command `{' '.join(command)}` failed with the following error:\n\n{e.output.decode()}"
) from e
@contextmanager
def assert_exception(exception_class: Exception, msg: str = None) -> bool:
"""
Context manager to assert that the right `Exception` class was raised.
If `msg` is provided, will check that the message is contained in the raised exception.
"""
was_ran = False
try:
yield
was_ran = True
except Exception as e:
assert isinstance(e, exception_class), f"Expected exception of type {exception_class} but got {type(e)}"
if msg is not None:
assert msg in str(e), f"Expected message '{msg}' to be in exception but got '{str(e)}'"
if was_ran:
raise AssertionError(f"Expected exception of type {exception_class} but ran without issue.")
| accelerate/src/accelerate/test_utils/testing.py/0 | {
"file_path": "accelerate/src/accelerate/test_utils/testing.py",
"repo_id": "accelerate",
"token_count": 6406
} | 7 |
# Copyright 2022 The HuggingFace Team. All rights reserved.
#
# Licensed under the Apache License, Version 2.0 (the "License");
# you may not use this file except in compliance with the License.
# You may obtain a copy of the License at
#
# http://www.apache.org/licenses/LICENSE-2.0
#
# Unless required by applicable law or agreed to in writing, software
# distributed under the License is distributed on an "AS IS" BASIS,
# WITHOUT WARRANTIES OR CONDITIONS OF ANY KIND, either express or implied.
# See the License for the specific language governing permissions and
# limitations under the License.
"""
A set of basic tensor ops compatible with tpu, gpu, and multigpu
"""
import pickle
import warnings
from functools import update_wrapper, wraps
from typing import Any, Mapping
import torch
from ..state import PartialState
from .constants import TORCH_DISTRIBUTED_OPERATION_TYPES
from .dataclasses import DistributedType, TensorInformation
from .imports import (
is_npu_available,
is_torch_distributed_available,
is_torch_version,
is_tpu_available,
is_xpu_available,
)
if is_tpu_available(check_device=False):
import torch_xla.core.xla_model as xm
if is_torch_distributed_available():
from torch.distributed import ReduceOp
def is_torch_tensor(tensor):
return isinstance(tensor, torch.Tensor)
def is_torch_xpu_tensor(tensor):
return isinstance(
tensor,
torch.xpu.FloatTensor,
torch.xpu.ByteTensor,
torch.xpu.IntTensor,
torch.xpu.LongTensor,
torch.xpu.HalfTensor,
torch.xpu.DoubleTensor,
torch.xpu.BFloat16Tensor,
)
def is_tensor_information(tensor_info):
return isinstance(tensor_info, TensorInformation)
def is_namedtuple(data):
"""
Checks if `x` is a `namedtuple` or not. Can have false positives, but only if a user is trying to mimic a
`namedtuple` perfectly.
"""
data_type = type(data)
bases = data_type.__bases__
if len(bases) != 1 or bases[0] != tuple:
return False
fields = getattr(data_type, "_fields", None)
if not isinstance(fields, tuple):
return False
return all(isinstance(member, str) for member in fields)
def honor_type(obj, generator):
"""
Cast a generator to the same type as obj (list, tuple, or namedtuple)
"""
# Some objects may not be able to instantiate from a generator directly
if is_namedtuple(obj):
return type(obj)(*list(generator))
else:
return type(obj)(generator)
def recursively_apply(func, data, *args, test_type=is_torch_tensor, error_on_other_type=False, **kwargs):
"""
Recursively apply a function on a data structure that is a nested list/tuple/dictionary of a given base type.
Args:
func (`callable`):
The function to recursively apply.
data (nested list/tuple/dictionary of `main_type`):
The data on which to apply `func`
*args:
Positional arguments that will be passed to `func` when applied on the unpacked data.
main_type (`type`, *optional*, defaults to `torch.Tensor`):
The base type of the objects to which apply `func`.
error_on_other_type (`bool`, *optional*, defaults to `False`):
Whether to return an error or not if after unpacking `data`, we get on an object that is not of type
`main_type`. If `False`, the function will leave objects of types different than `main_type` unchanged.
**kwargs:
Keyword arguments that will be passed to `func` when applied on the unpacked data.
Returns:
The same data structure as `data` with `func` applied to every object of type `main_type`.
"""
if isinstance(data, (tuple, list)):
return honor_type(
data,
(
recursively_apply(
func, o, *args, test_type=test_type, error_on_other_type=error_on_other_type, **kwargs
)
for o in data
),
)
elif isinstance(data, Mapping):
return type(data)(
{
k: recursively_apply(
func, v, *args, test_type=test_type, error_on_other_type=error_on_other_type, **kwargs
)
for k, v in data.items()
}
)
elif test_type(data):
return func(data, *args, **kwargs)
elif error_on_other_type:
raise TypeError(
f"Unsupported types ({type(data)}) passed to `{func.__name__}`. Only nested list/tuple/dicts of "
f"objects that are valid for `{test_type.__name__}` should be passed."
)
return data
def send_to_device(tensor, device, non_blocking=False, skip_keys=None):
"""
Recursively sends the elements in a nested list/tuple/dictionary of tensors to a given device.
Args:
tensor (nested list/tuple/dictionary of `torch.Tensor`):
The data to send to a given device.
device (`torch.device`):
The device to send the data to.
Returns:
The same data structure as `tensor` with all tensors sent to the proper device.
"""
if isinstance(tensor, (tuple, list)):
return honor_type(
tensor, (send_to_device(t, device, non_blocking=non_blocking, skip_keys=skip_keys) for t in tensor)
)
elif isinstance(tensor, Mapping):
if isinstance(skip_keys, str):
skip_keys = [skip_keys]
elif skip_keys is None:
skip_keys = []
return type(tensor)(
{
k: t if k in skip_keys else send_to_device(t, device, non_blocking=non_blocking, skip_keys=skip_keys)
for k, t in tensor.items()
}
)
elif hasattr(tensor, "to"):
if is_npu_available():
# `torch.Tensor.to(<int num>)` is not supported by `torch_npu` (see this [issue](https://github.com/Ascend/pytorch/issues/16)).
if isinstance(device, int):
device = f"npu:{device}"
# `torch.Tensor.to("npu")` could not find context when called for the first time (see this [issue](https://gitee.com/ascend/pytorch/issues/I8KECW?from=project-issue)).
elif device == torch.device("npu"):
device = "npu:0"
elif is_xpu_available():
if isinstance(device, int):
device = f"xpu:{device}"
elif device == torch.device("xpu"):
device = "xpu:0"
try:
return tensor.to(device, non_blocking=non_blocking)
except TypeError: # .to() doesn't accept non_blocking as kwarg
return tensor.to(device)
else:
return tensor
def get_data_structure(data):
"""
Recursively gathers the information needed to rebuild a nested list/tuple/dictionary of tensors.
Args:
data (nested list/tuple/dictionary of `torch.Tensor`):
The data to send to analyze.
Returns:
The same data structure as `data` with [`~utils.TensorInformation`] instead of tensors.
"""
def _get_data_structure(tensor):
return TensorInformation(shape=tensor.shape, dtype=tensor.dtype)
return recursively_apply(_get_data_structure, data)
def get_shape(data):
"""
Recursively gathers the shape of a nested list/tuple/dictionary of tensors as a list.
Args:
data (nested list/tuple/dictionary of `torch.Tensor`):
The data to send to analyze.
Returns:
The same data structure as `data` with lists of tensor shapes instead of tensors.
"""
def _get_shape(tensor):
return list(tensor.shape)
return recursively_apply(_get_shape, data)
def initialize_tensors(data_structure):
"""
Recursively initializes tensors from a nested list/tuple/dictionary of [`~utils.TensorInformation`].
Returns:
The same data structure as `data` with tensors instead of [`~utils.TensorInformation`].
"""
def _initialize_tensor(tensor_info):
return torch.empty(*tensor_info.shape, dtype=tensor_info.dtype)
return recursively_apply(_initialize_tensor, data_structure, test_type=is_tensor_information)
def find_batch_size(data):
"""
Recursively finds the batch size in a nested list/tuple/dictionary of lists of tensors.
Args:
data (nested list/tuple/dictionary of `torch.Tensor`): The data from which to find the batch size.
Returns:
`int`: The batch size.
"""
if isinstance(data, (tuple, list, Mapping)) and (len(data) == 0):
raise ValueError(f"Cannot find the batch size from empty {type(data)}.")
if isinstance(data, (tuple, list)):
return find_batch_size(data[0])
elif isinstance(data, Mapping):
for k in data.keys():
return find_batch_size(data[k])
elif not isinstance(data, torch.Tensor):
raise TypeError(f"Can only find the batch size of tensors but got {type(data)}.")
return data.shape[0]
def listify(data):
"""
Recursively finds tensors in a nested list/tuple/dictionary and converts them to a list of numbers.
Args:
data (nested list/tuple/dictionary of `torch.Tensor`): The data from which to convert to regular numbers.
Returns:
The same data structure as `data` with lists of numbers instead of `torch.Tensor`.
"""
def _convert_to_list(tensor):
tensor = tensor.detach().cpu()
if tensor.dtype == torch.bfloat16:
# As of Numpy 1.21.4, NumPy does not support bfloat16 (see
# https://github.com/numpy/numpy/blob/a47ecdea856986cd60eabbd53265c2ca5916ad5d/doc/source/user/basics.types.rst ).
# Until Numpy adds bfloat16, we must convert float32.
tensor = tensor.to(torch.float32)
return tensor.tolist()
return recursively_apply(_convert_to_list, data)
def _tpu_gather(tensor):
def _tpu_gather_one(tensor):
if tensor.ndim == 0:
tensor = tensor.clone()[None]
# Can only gather contiguous tensors
if not tensor.is_contiguous():
tensor = tensor.contiguous()
return xm.all_gather(tensor)
res = recursively_apply(_tpu_gather_one, tensor, error_on_other_type=True)
xm.mark_step()
return res
def _gpu_gather(tensor):
state = PartialState()
if is_torch_version(">=", "1.13"):
gather_op = torch.distributed.all_gather_into_tensor
else:
gather_op = torch.distributed._all_gather_base
def _gpu_gather_one(tensor):
if tensor.ndim == 0:
tensor = tensor.clone()[None]
# Can only gather contiguous tensors
if not tensor.is_contiguous():
tensor = tensor.contiguous()
if state.backend is not None and state.backend != "gloo":
# We use `empty` as `all_gather_into_tensor` slightly
# differs from `all_gather` for better efficiency,
# and we rely on the number of items in the tensor
# rather than its direct shape
output_tensors = torch.empty(
state.num_processes * tensor.numel(),
dtype=tensor.dtype,
device=state.device,
)
gather_op(output_tensors, tensor)
return output_tensors.view(-1, *tensor.size()[1:])
else:
# a backend of `None` is always CPU
# also gloo does not support `all_gather_into_tensor`,
# which will result in a larger memory overhead for the op
output_tensors = [torch.empty_like(tensor) for _ in range(state.num_processes)]
torch.distributed.all_gather(output_tensors, tensor)
return torch.cat(output_tensors, dim=0)
return recursively_apply(_gpu_gather_one, tensor, error_on_other_type=True)
class DistributedOperationException(Exception):
"""
An exception class for distributed operations. Raised if the operation cannot be performed due to the shape of the
tensors.
"""
pass
def verify_operation(function):
"""
Verifies that `tensor` is the same shape across all processes. Only ran if `PartialState().debug` is `True`.
"""
@wraps(function)
def wrapper(*args, **kwargs):
if PartialState().distributed_type == DistributedType.NO or not PartialState().debug:
return function(*args, **kwargs)
operation = f"{function.__module__}.{function.__name__}"
if "tensor" in kwargs:
tensor = kwargs["tensor"]
else:
tensor = args[0]
if PartialState().device.type != find_device(tensor).type:
raise DistributedOperationException(
f"One or more of the tensors passed to {operation} were not on the {tensor.device.type} while the `Accelerator` is configured for {PartialState().device.type}. "
f"Please move it to the {PartialState().device.type} before calling {operation}."
)
shapes = get_shape(tensor)
output = gather_object([shapes])
if output[0] is not None:
are_same = output.count(output[0]) == len(output)
if not are_same:
process_shape_str = "\n - ".join([f"Process {i}: {shape}" for i, shape in enumerate(output)])
raise DistributedOperationException(
f"Cannot apply desired operation due to shape mismatches. "
"All shapes across devices must be valid."
f"\n\nOperation: `{operation}`\nInput shapes:\n - {process_shape_str}"
)
return function(*args, **kwargs)
return wrapper
def chained_operation(function):
"""
Checks that `verify_operation` failed and if so reports a more helpful error chaining the existing
`DistributedOperationException`.
"""
@wraps(function)
def wrapper(*args, **kwargs):
try:
return function(*args, **kwargs)
except DistributedOperationException as e:
operation = f"{function.__module__}.{function.__name__}"
raise DistributedOperationException(
f"Error found while calling `{operation}`. Please see the earlier error for more details."
) from e
return wrapper
@verify_operation
def gather(tensor):
"""
Recursively gather tensor in a nested list/tuple/dictionary of tensors from all devices.
Args:
tensor (nested list/tuple/dictionary of `torch.Tensor`):
The data to gather.
Returns:
The same data structure as `tensor` with all tensors sent to the proper device.
"""
if PartialState().distributed_type == DistributedType.TPU:
return _tpu_gather(tensor)
elif PartialState().distributed_type in TORCH_DISTRIBUTED_OPERATION_TYPES:
return _gpu_gather(tensor)
else:
return tensor
def _gpu_gather_object(object: Any):
output_objects = [None for _ in range(PartialState().num_processes)]
torch.distributed.all_gather_object(output_objects, object)
# all_gather_object returns a list of lists, so we need to flatten it
return [x for y in output_objects for x in y]
def gather_object(object: Any):
"""
Recursively gather object in a nested list/tuple/dictionary of objects from all devices.
Args:
object (nested list/tuple/dictionary of picklable object):
The data to gather.
Returns:
The same data structure as `object` with all the objects sent to every device.
"""
if PartialState().distributed_type == DistributedType.TPU:
raise NotImplementedError("gather objects in TPU is not supported")
elif PartialState().distributed_type in TORCH_DISTRIBUTED_OPERATION_TYPES:
return _gpu_gather_object(object)
else:
return object
def _gpu_broadcast(data, src=0):
def _gpu_broadcast_one(tensor, src=0):
torch.distributed.broadcast(tensor, src=src)
return tensor
return recursively_apply(_gpu_broadcast_one, data, error_on_other_type=True, src=src)
def _tpu_broadcast(tensor, src=0, name="broadcast tensor"):
if isinstance(tensor, (list, tuple)):
return honor_type(tensor, (_tpu_broadcast(t, name=f"{name}_{i}") for i, t in enumerate(tensor)))
elif isinstance(tensor, Mapping):
return type(tensor)({k: _tpu_broadcast(v, name=f"{name}_{k}") for k, v in tensor.items()})
return xm.mesh_reduce(name, tensor, lambda x: x[src])
@verify_operation
def broadcast(tensor, from_process: int = 0):
"""
Recursively broadcast tensor in a nested list/tuple/dictionary of tensors to all devices.
Args:
tensor (nested list/tuple/dictionary of `torch.Tensor`):
The data to gather.
from_process (`int`, *optional*, defaults to 0):
The process from which to send the data
Returns:
The same data structure as `tensor` with all tensors broadcasted to the proper device.
"""
if PartialState().distributed_type == DistributedType.TPU:
return _tpu_broadcast(tensor, src=from_process, name="accelerate.utils.broadcast")
elif PartialState().distributed_type in TORCH_DISTRIBUTED_OPERATION_TYPES:
return _gpu_broadcast(tensor, src=from_process)
else:
return tensor
def broadcast_object_list(object_list, from_process: int = 0):
"""
Broadcast a list of picklable objects form one process to the others.
Args:
object_list (list of picklable objects):
The list of objects to broadcast. This list will be modified inplace.
from_process (`int`, *optional*, defaults to 0):
The process from which to send the data.
Returns:
The same list containing the objects from process 0.
"""
if PartialState().distributed_type == DistributedType.TPU:
for i, obj in enumerate(object_list):
object_list[i] = xm.mesh_reduce("accelerate.utils.broadcast_object_list", obj, lambda x: x[from_process])
elif PartialState().distributed_type in TORCH_DISTRIBUTED_OPERATION_TYPES:
torch.distributed.broadcast_object_list(object_list, src=from_process)
return object_list
def slice_tensors(data, tensor_slice, process_index=None, num_processes=None):
"""
Recursively takes a slice in a nested list/tuple/dictionary of tensors.
Args:
data (nested list/tuple/dictionary of `torch.Tensor`):
The data to slice.
tensor_slice (`slice`):
The slice to take.
Returns:
The same data structure as `data` with all the tensors slices.
"""
def _slice_tensor(tensor, tensor_slice):
return tensor[tensor_slice]
return recursively_apply(_slice_tensor, data, tensor_slice)
def concatenate(data, dim=0):
"""
Recursively concatenate the tensors in a nested list/tuple/dictionary of lists of tensors with the same shape.
Args:
data (nested list/tuple/dictionary of lists of tensors `torch.Tensor`):
The data to concatenate.
dim (`int`, *optional*, defaults to 0):
The dimension on which to concatenate.
Returns:
The same data structure as `data` with all the tensors concatenated.
"""
if isinstance(data[0], (tuple, list)):
return honor_type(data[0], (concatenate([d[i] for d in data], dim=dim) for i in range(len(data[0]))))
elif isinstance(data[0], Mapping):
return type(data[0])({k: concatenate([d[k] for d in data], dim=dim) for k in data[0].keys()})
elif not isinstance(data[0], torch.Tensor):
raise TypeError(f"Can only concatenate tensors but got {type(data[0])}")
return torch.cat(data, dim=dim)
class CannotPadNestedTensorWarning(UserWarning):
pass
@chained_operation
def pad_across_processes(tensor, dim=0, pad_index=0, pad_first=False):
"""
Recursively pad the tensors in a nested list/tuple/dictionary of tensors from all devices to the same size so they
can safely be gathered.
Args:
tensor (nested list/tuple/dictionary of `torch.Tensor`):
The data to gather.
dim (`int`, *optional*, defaults to 0):
The dimension on which to pad.
pad_index (`int`, *optional*, defaults to 0):
The value with which to pad.
pad_first (`bool`, *optional*, defaults to `False`):
Whether to pad at the beginning or the end.
"""
def _pad_across_processes(tensor, dim=0, pad_index=0, pad_first=False):
if getattr(tensor, "is_nested", False):
warnings.warn(
"Cannot pad nested tensors without more information. Leaving unprocessed.",
CannotPadNestedTensorWarning,
)
return tensor
if dim >= len(tensor.shape):
return tensor
# Gather all sizes
size = torch.tensor(tensor.shape, device=tensor.device)[None]
sizes = gather(size).cpu()
# Then pad to the maximum size
max_size = max(s[dim] for s in sizes)
if max_size == tensor.shape[dim]:
return tensor
old_size = tensor.shape
new_size = list(old_size)
new_size[dim] = max_size
new_tensor = tensor.new_zeros(tuple(new_size)) + pad_index
if pad_first:
indices = tuple(
slice(max_size - old_size[dim], max_size) if i == dim else slice(None) for i in range(len(new_size))
)
else:
indices = tuple(slice(0, old_size[dim]) if i == dim else slice(None) for i in range(len(new_size)))
new_tensor[indices] = tensor
return new_tensor
return recursively_apply(
_pad_across_processes, tensor, error_on_other_type=True, dim=dim, pad_index=pad_index, pad_first=pad_first
)
@verify_operation
def reduce(tensor, reduction="mean", scale=1.0):
"""
Recursively reduce the tensors in a nested list/tuple/dictionary of lists of tensors across all processes by the
mean of a given operation.
Args:
tensor (nested list/tuple/dictionary of `torch.Tensor`):
The data to reduce.
reduction (`str`, *optional*, defaults to `"mean"`):
A reduction method. Can be of "mean", "sum", or "none"
scale (`float`, *optional*):
A default scaling value to be applied after the reduce, only valied on XLA.
Returns:
The same data structure as `data` with all the tensors reduced.
"""
def _reduce_across_processes(tensor, reduction="mean", scale=1.0):
state = PartialState()
cloned_tensor = tensor.clone()
if state.distributed_type == DistributedType.NO:
return cloned_tensor
if state.distributed_type == DistributedType.TPU:
# Some processes may have different HLO graphs than other
# processes, for example in the breakpoint API
# accelerator.set_trigger(). Use mark_step to make HLOs
# the same on all processes.
xm.mark_step()
xm.all_reduce(xm.REDUCE_SUM, [cloned_tensor], scale)
xm.mark_step()
elif state.distributed_type.value in TORCH_DISTRIBUTED_OPERATION_TYPES:
torch.distributed.all_reduce(cloned_tensor, ReduceOp.SUM)
if reduction == "mean":
cloned_tensor /= state.num_processes
return cloned_tensor
return recursively_apply(
_reduce_across_processes, tensor, error_on_other_type=True, reduction=reduction, scale=scale
)
def convert_to_fp32(tensor):
"""
Recursively converts the elements nested list/tuple/dictionary of tensors in FP16/BF16 precision to FP32.
Args:
tensor (nested list/tuple/dictionary of `torch.Tensor`):
The data to convert from FP16/BF16 to FP32.
Returns:
The same data structure as `tensor` with all tensors that were in FP16/BF16 precision converted to FP32.
"""
def _convert_to_fp32(tensor):
return tensor.float()
def _is_fp16_bf16_tensor(tensor):
return hasattr(tensor, "dtype") and tensor.dtype in (torch.float16, torch.bfloat16)
return recursively_apply(_convert_to_fp32, tensor, test_type=_is_fp16_bf16_tensor)
class ConvertOutputsToFp32:
"""
Decorator to apply to a function outputing tensors (like a model forward pass) that ensures the outputs in FP16
precision will be convert back to FP32.
Args:
model_forward (`Callable`):
The function which outputs we want to treat.
Returns:
The same function as `model_forward` but with converted outputs.
"""
def __init__(self, model_forward):
self.model_forward = model_forward
update_wrapper(self, model_forward)
def __call__(self, *args, **kwargs):
return convert_to_fp32(self.model_forward(*args, **kwargs))
def __getstate__(self):
raise pickle.PicklingError(
"Cannot pickle a prepared model with automatic mixed precision, please unwrap the model with `Accelerator.unwrap_model(model)` before pickling it."
)
def convert_outputs_to_fp32(model_forward):
model_forward = ConvertOutputsToFp32(model_forward)
def forward(*args, **kwargs):
return model_forward(*args, **kwargs)
# To act like a decorator so that it can be popped when doing `extract_model_from_parallel`
forward.__wrapped__ = model_forward
return forward
def find_device(data):
"""
Finds the device on which a nested dict/list/tuple of tensors lies (assuming they are all on the same device).
Args:
(nested list/tuple/dictionary of `torch.Tensor`): The data we want to know the device of.
"""
if isinstance(data, Mapping):
for obj in data.values():
device = find_device(obj)
if device is not None:
return device
elif isinstance(data, (tuple, list)):
for obj in data:
device = find_device(obj)
if device is not None:
return device
elif isinstance(data, torch.Tensor):
return data.device
| accelerate/src/accelerate/utils/operations.py/0 | {
"file_path": "accelerate/src/accelerate/utils/operations.py",
"repo_id": "accelerate",
"token_count": 10785
} | 8 |
compute_environment: LOCAL_MACHINE
deepspeed_config: {}
distributed_type: 'NO'
downcast_bf16: 'no'
fsdp_config: {}
machine_rank: 0
main_process_ip: null
main_process_port: null
main_training_function: main
mixed_precision: 'no'
num_machines: 1
num_processes: 1
use_cpu: false | accelerate/tests/test_configs/0_12_0.yaml/0 | {
"file_path": "accelerate/tests/test_configs/0_12_0.yaml",
"repo_id": "accelerate",
"token_count": 105
} | 9 |
# Copyright 2023 The HuggingFace Team. All rights reserved.
#
# Licensed under the Apache License, Version 2.0 (the "License");
# you may not use this file except in compliance with the License.
# You may obtain a copy of the License at
#
# http://www.apache.org/licenses/LICENSE-2.0
#
# Unless required by applicable law or agreed to in writing, software
# distributed under the License is distributed on an "AS IS" BASIS,
# WITHOUT WARRANTIES OR CONDITIONS OF ANY KIND, either express or implied.
# See the License for the specific language governing permissions and
# limitations under the License.
import gc
import tempfile
import unittest
import torch
import torch.nn as nn
from accelerate import Accelerator, init_empty_weights
from accelerate.test_utils import require_bnb, require_cuda, require_huggingface_suite, require_multi_gpu, slow
from accelerate.utils.bnb import load_and_quantize_model
from accelerate.utils.dataclasses import BnbQuantizationConfig
class BitsAndBytesConfigIntegration(unittest.TestCase):
def test_BnbQuantizationConfig(self):
with self.assertRaises(ValueError):
BnbQuantizationConfig(load_in_8bit=True, load_in_4bit=True)
@slow
@require_cuda
@require_bnb
@require_huggingface_suite
class MixedInt8EmptyModelTest(unittest.TestCase):
# We keep the constants inside the init function and model loading inside setUp function
# We need to test on relatively large models (aka >1b parameters otherwise the quantiztion may not work as expected)
# Therefore here we use only bloom-1b3 to test our module
model_name = "marcsun13/bloom-1b7_with_lm_head"
# Constant values
# This was obtained on a Quadro RTX 8000 so the number might slightly change
EXPECTED_RELATIVE_DIFFERENCE = 1.540025
input_text = "Hello my name is"
EXPECTED_OUTPUT = "Hello my name is John.\nI am a friend of the family.\n"
MAX_NEW_TOKENS = 10
def setUp(self):
"""
Setup quantized model from empty model
"""
from huggingface_hub import hf_hub_download
from transformers import AutoConfig, AutoModelForCausalLM, AutoTokenizer
# Models and tokenizer
self.model_fp16 = AutoModelForCausalLM.from_pretrained(
self.model_name, torch_dtype=torch.float16, device_map="auto"
)
# create model on meta device
with init_empty_weights():
self.model_8bit = AutoModelForCausalLM.from_config(AutoConfig.from_pretrained(self.model_name))
self.model_8bit.tie_weights()
self.weights_location = hf_hub_download(self.model_name, "pytorch_model.bin")
self.bnb_quantization_config = BnbQuantizationConfig(load_in_8bit=True)
self.model_8bit = load_and_quantize_model(
self.model_8bit,
self.bnb_quantization_config,
weights_location=self.weights_location,
device_map={"": 0},
no_split_module_classes=["BloomBlock"],
)
self.tokenizer = AutoTokenizer.from_pretrained("bigscience/bloom-1b7")
self.accelerate = Accelerator()
def tearDown(self):
r"""
TearDown function needs to be called at the end of each test to free the GPU memory and cache, also to
avoid unexpected behaviors. Please see: https://discuss.pytorch.org/t/how-can-we-release-gpu-memory-cache/14530/27
"""
del self.model_fp16
del self.model_8bit
gc.collect()
torch.cuda.empty_cache()
def test_memory_footprint(self):
r"""
A simple test to check if the model conversion has been done correctly by checking on the
memory footprint of the converted model and the class type of the linear layers of the converted models
"""
from bitsandbytes.nn import Int8Params
mem_fp16 = self.model_fp16.get_memory_footprint()
mem_8bit = self.model_8bit.get_memory_footprint()
self.assertAlmostEqual(mem_fp16 / mem_8bit, self.EXPECTED_RELATIVE_DIFFERENCE)
self.assertTrue(self.model_8bit.transformer.h[0].mlp.dense_4h_to_h.weight.__class__ == Int8Params)
def test_linear_are_8bit(self):
r"""
A simple test to check if the model conversion has been done correctly by checking on the
memory footprint of the converted model and the class type of the linear layers of the converted models
"""
self.model_fp16.get_memory_footprint()
self.model_8bit.get_memory_footprint()
for name, module in self.model_8bit.named_modules():
if isinstance(module, torch.nn.Linear):
modules_not_converted = (
self.bnb_quantization_config.keep_in_fp32_modules + self.bnb_quantization_config.skip_modules
)
if name not in modules_not_converted:
self.assertTrue(module.weight.dtype == torch.int8)
def test_llm_skip(self):
r"""
A simple test to check if `llm_int8_skip_modules` works as expected
"""
import bitsandbytes as bnb
from transformers import AutoConfig, AutoModelForCausalLM
bnb_quantization_config = BnbQuantizationConfig(
load_in_8bit=True, skip_modules=["lm_head", "transformer.word_embeddings"]
)
with init_empty_weights():
model = AutoModelForCausalLM.from_config(AutoConfig.from_pretrained(self.model_name))
model.tie_weights()
model = load_and_quantize_model(
model,
bnb_quantization_config,
weights_location=self.weights_location,
device_map="auto",
no_split_module_classes=["BloomBlock"],
)
self.assertTrue(model.transformer.h[1].mlp.dense_4h_to_h.weight.dtype == torch.int8)
self.assertTrue(isinstance(model.transformer.h[1].mlp.dense_4h_to_h, bnb.nn.Linear8bitLt))
self.assertTrue(isinstance(model.lm_head, nn.Linear))
self.assertTrue(model.lm_head.weight.dtype != torch.int8)
def check_inference_correctness(self, model):
r"""
Test the generation quality of the quantized model and see that we are matching the expected output.
Given that we are operating on small numbers + the testing model is relatively small, we might not get
the same output across GPUs. So we'll generate few tokens (5-10) and check their output.
"""
# Check that inference pass works on the model
encoded_input = self.tokenizer(self.input_text, return_tensors="pt")
# Check the exactness of the results
output_parallel = model.generate(input_ids=encoded_input["input_ids"].to(0), max_new_tokens=10)
# Get the generation
output_text = self.tokenizer.decode(output_parallel[0], skip_special_tokens=True)
self.assertEqual(output_text, self.EXPECTED_OUTPUT)
def test_generate_quality(self):
self.check_inference_correctness(self.model_8bit)
def test_fp32_8bit_conversion(self):
r"""
Test whether it is possible to mix both `8bit` and `fp32` weights when using `keep_in_fp32_modules` correctly.
"""
from transformers import AutoConfig, AutoModelForCausalLM
bnb_quantization_config = BnbQuantizationConfig(load_in_8bit=True, keep_in_fp32_modules=["lm_head"])
with init_empty_weights():
model = AutoModelForCausalLM.from_config(AutoConfig.from_pretrained(self.model_name))
model.tie_weights()
model = load_and_quantize_model(
model,
bnb_quantization_config,
weights_location=self.weights_location,
device_map="auto",
no_split_module_classes=["BloomBlock"],
)
self.assertTrue(model.lm_head.weight.dtype == torch.float32)
@require_multi_gpu
def test_cpu_gpu_loading_custom_device_map(self):
from bitsandbytes.nn import Int8Params
from transformers import AutoConfig, AutoModelForCausalLM
r"""
A test to check is dispatching a model on cpu & gpu works correctly using a custom `device_map`.
"""
device_map = {
"transformer.word_embeddings": "cpu",
"transformer.word_embeddings_layernorm": 0,
"lm_head": "cpu",
"transformer.h.0": "cpu",
"transformer.h.1": "cpu",
"transformer.h.2": "cpu",
"transformer.h.3": 0,
"transformer.h.4": 0,
"transformer.h.5": 0,
"transformer.h.6": 0,
"transformer.h.7": 0,
"transformer.h.8": 0,
"transformer.h.9": 1,
"transformer.h.10": 0,
"transformer.h.11": 1,
"transformer.h.12": 0,
"transformer.h.13": 0,
"transformer.h.14": 1,
"transformer.h.15": 0,
"transformer.h.16": 0,
"transformer.h.17": 1,
"transformer.h.18": 1,
"transformer.h.19": 0,
"transformer.h.20": 1,
"transformer.h.21": 1,
"transformer.h.22": 0,
"transformer.h.23": 0,
"transformer.ln_f": 1,
}
bnb_quantization_config = BnbQuantizationConfig(load_in_8bit=True)
with init_empty_weights():
model_8bit = AutoModelForCausalLM.from_config(AutoConfig.from_pretrained(self.model_name))
model_8bit.tie_weights()
model_8bit = load_and_quantize_model(
model_8bit,
bnb_quantization_config,
weights_location=self.weights_location,
device_map=device_map,
no_split_module_classes=["BloomBlock"],
)
self.assertTrue(model_8bit.transformer.h[0].mlp.dense_4h_to_h.weight.__class__ == Int8Params)
self.assertTrue(model_8bit.transformer.h[1].mlp.dense_4h_to_h.weight.__class__ == Int8Params)
self.check_inference_correctness(model_8bit)
@require_multi_gpu
def test_cpu_gpu_loading_custom_device_map_offload_state_dict(self):
from bitsandbytes.nn import Int8Params
from transformers import AutoConfig, AutoModelForCausalLM
r"""
A test to check is dispatching a model on cpu & gpu works correctly using a custom `device_map` and offload_state_dict=True.
"""
device_map = {
"transformer.word_embeddings": "cpu",
"transformer.word_embeddings_layernorm": 0,
"lm_head": "cpu",
"transformer.h.0": "cpu",
"transformer.h.1": "cpu",
"transformer.h.2": "cpu",
"transformer.h.3": 0,
"transformer.h.4": 0,
"transformer.h.5": 0,
"transformer.h.6": 0,
"transformer.h.7": 0,
"transformer.h.8": 0,
"transformer.h.9": 1,
"transformer.h.10": 0,
"transformer.h.11": 1,
"transformer.h.12": 0,
"transformer.h.13": 0,
"transformer.h.14": 1,
"transformer.h.15": 0,
"transformer.h.16": 0,
"transformer.h.17": 1,
"transformer.h.18": 1,
"transformer.h.19": 0,
"transformer.h.20": 1,
"transformer.h.21": 1,
"transformer.h.22": 0,
"transformer.h.23": 0,
"transformer.ln_f": 1,
}
bnb_quantization_config = BnbQuantizationConfig(load_in_8bit=True)
with init_empty_weights():
model_8bit = AutoModelForCausalLM.from_config(AutoConfig.from_pretrained(self.model_name))
model_8bit.tie_weights()
model_8bit = load_and_quantize_model(
model_8bit,
bnb_quantization_config,
weights_location=self.weights_location,
device_map=device_map,
no_split_module_classes=["BloomBlock"],
offload_state_dict=True,
)
self.assertTrue(model_8bit.transformer.h[0].mlp.dense_4h_to_h.weight.__class__ == Int8Params)
self.assertTrue(model_8bit.transformer.h[1].mlp.dense_4h_to_h.weight.__class__ == Int8Params)
self.check_inference_correctness(model_8bit)
@require_multi_gpu
def test_cpu_gpu_disk_loading_custom_device_map_kwargs(self):
from bitsandbytes.nn import Int8Params
from transformers import AutoConfig, AutoModelForCausalLM
r"""
A test to check is dispatching a model on cpu & gpu works correctly using a custom `device_map`.
This time we also add `disk` on the device_map - using the kwargs directly instead of the quantization config
"""
device_map = {
"transformer.word_embeddings": "cpu",
"transformer.word_embeddings_layernorm": 0,
"lm_head": "cpu",
"transformer.h.0": "cpu",
"transformer.h.1": "cpu",
"transformer.h.2": "cpu",
"transformer.h.3": "disk",
"transformer.h.4": "disk",
"transformer.h.5": "disk",
"transformer.h.6": 0,
"transformer.h.7": 0,
"transformer.h.8": 0,
"transformer.h.9": 1,
"transformer.h.10": 0,
"transformer.h.11": 1,
"transformer.h.12": 0,
"transformer.h.13": 0,
"transformer.h.14": 1,
"transformer.h.15": 0,
"transformer.h.16": 0,
"transformer.h.17": 1,
"transformer.h.18": 1,
"transformer.h.19": 0,
"transformer.h.20": 1,
"transformer.h.21": 1,
"transformer.h.22": 0,
"transformer.h.23": 0,
"transformer.ln_f": 1,
}
bnb_quantization_config = BnbQuantizationConfig(load_in_8bit=True)
with init_empty_weights():
model_8bit = AutoModelForCausalLM.from_config(AutoConfig.from_pretrained(self.model_name))
model_8bit.tie_weights()
with tempfile.TemporaryDirectory() as tmpdirname:
model_8bit = load_and_quantize_model(
model_8bit,
bnb_quantization_config,
weights_location=self.weights_location,
device_map=device_map,
no_split_module_classes=["BloomBlock"],
offload_folder=tmpdirname,
offload_state_dict=True,
)
self.assertTrue(model_8bit.transformer.h[4].mlp.dense_4h_to_h.weight.__class__ == Int8Params)
self.assertTrue(model_8bit.transformer.h[5].mlp.dense_4h_to_h.weight.__class__ == Int8Params)
self.check_inference_correctness(model_8bit)
def test_int8_serialization(self):
r"""
Test whether it is possible to serialize a model in 8-bit.
"""
from bitsandbytes.nn import Int8Params
from transformers import AutoConfig, AutoModelForCausalLM
with tempfile.TemporaryDirectory() as tmpdirname:
# saving state dict for now but will save config and other in the future
self.accelerate.save_model(self.model_8bit, tmpdirname)
with init_empty_weights():
# let's suppose that we can get the right config
model_8bit_from_saved = AutoModelForCausalLM.from_config(AutoConfig.from_pretrained(self.model_name))
model_8bit_from_saved.tie_weights()
bnb_quantization_config = BnbQuantizationConfig(load_in_8bit=True)
model_8bit_from_saved = load_and_quantize_model(
model_8bit_from_saved,
bnb_quantization_config,
weights_location=tmpdirname,
device_map="auto",
no_split_module_classes=["BloomBlock"],
)
self.assertTrue(model_8bit_from_saved.transformer.h[0].mlp.dense_4h_to_h.weight.__class__ == Int8Params)
self.assertTrue(hasattr(model_8bit_from_saved.transformer.h[0].mlp.dense_4h_to_h.weight, "SCB"))
self.assertTrue(hasattr(model_8bit_from_saved.transformer.h[0].mlp.dense_4h_to_h.weight, "CB"))
self.check_inference_correctness(model_8bit_from_saved)
@require_multi_gpu
def test_int8_serialization_offload(self):
r"""
Test whether it is possible to serialize a model in 8-bit and offload weights to cpu/disk
"""
from bitsandbytes.nn import Int8Params
from transformers import AutoConfig, AutoModelForCausalLM
with tempfile.TemporaryDirectory() as tmpdirname:
# saving state dict for now but will save config and other in the future
self.accelerate.save_model(self.model_8bit, tmpdirname)
with init_empty_weights():
# let's suppose that we can get the right config
model_8bit_from_saved = AutoModelForCausalLM.from_config(AutoConfig.from_pretrained(self.model_name))
model_8bit_from_saved.tie_weights()
bnb_quantization_config = BnbQuantizationConfig(load_in_8bit=True)
device_map = {
"transformer.word_embeddings": "cpu",
"transformer.word_embeddings_layernorm": 0,
"lm_head": "cpu",
"transformer.h.0": "cpu",
"transformer.h.1": "cpu",
"transformer.h.2": "cpu",
"transformer.h.3": "disk",
"transformer.h.4": "disk",
"transformer.h.5": "disk",
"transformer.h.6": 0,
"transformer.h.7": 0,
"transformer.h.8": 0,
"transformer.h.9": 1,
"transformer.h.10": 0,
"transformer.h.11": 1,
"transformer.h.12": 0,
"transformer.h.13": 0,
"transformer.h.14": 1,
"transformer.h.15": 0,
"transformer.h.16": 0,
"transformer.h.17": 1,
"transformer.h.18": 1,
"transformer.h.19": 0,
"transformer.h.20": 1,
"transformer.h.21": 1,
"transformer.h.22": 0,
"transformer.h.23": 0,
"transformer.ln_f": 1,
}
model_8bit_from_saved = load_and_quantize_model(
model_8bit_from_saved,
bnb_quantization_config,
weights_location=tmpdirname,
device_map=device_map,
no_split_module_classes=["BloomBlock"],
offload_folder=tmpdirname + "/tmp",
offload_state_dict=True,
)
self.assertTrue(model_8bit_from_saved.transformer.h[4].mlp.dense_4h_to_h.weight.__class__ == Int8Params)
self.assertTrue(model_8bit_from_saved.transformer.h[5].mlp.dense_4h_to_h.weight.__class__ == Int8Params)
self.check_inference_correctness(model_8bit_from_saved)
def test_int8_serialization_shard(self):
r"""
Test whether it is possible to serialize a model in 8-bit.
"""
from bitsandbytes.nn import Int8Params
from transformers import AutoConfig, AutoModelForCausalLM
with tempfile.TemporaryDirectory() as tmpdirname:
# saving state dict for now but will save config and other in the future
self.accelerate.save_model(self.model_8bit, tmpdirname, max_shard_size="1GB")
with init_empty_weights():
# let's suppose that we can get the right config
model_8bit_from_saved = AutoModelForCausalLM.from_config(AutoConfig.from_pretrained(self.model_name))
model_8bit_from_saved.tie_weights()
bnb_quantization_config = BnbQuantizationConfig(load_in_8bit=True)
model_8bit_from_saved = load_and_quantize_model(
model_8bit_from_saved,
bnb_quantization_config,
weights_location=tmpdirname,
device_map="auto",
no_split_module_classes=["BloomBlock"],
)
self.assertTrue(model_8bit_from_saved.transformer.h[0].mlp.dense_4h_to_h.weight.__class__ == Int8Params)
self.assertTrue(hasattr(model_8bit_from_saved.transformer.h[0].mlp.dense_4h_to_h.weight, "SCB"))
self.assertTrue(hasattr(model_8bit_from_saved.transformer.h[0].mlp.dense_4h_to_h.weight, "CB"))
self.check_inference_correctness(model_8bit_from_saved)
@slow
@require_cuda
@require_bnb
@require_huggingface_suite
class MixedInt8LoaddedModelTest(unittest.TestCase):
# We keep the constants inside the init function and model loading inside setUp function
# We need to test on relatively large models (aka >1b parameters otherwise the quantiztion may not work as expected)
# Therefore here we use only bloom-1b3 to test our module
model_name = "marcsun13/bloom-1b7_with_lm_head"
# Constant values
# This was obtained on a Quadro RTX 8000 so the number might slightly change
EXPECTED_RELATIVE_DIFFERENCE = 1.540025
input_text = "Hello my name is"
EXPECTED_OUTPUT = "Hello my name is John.\nI am a friend of the family.\n"
MAX_NEW_TOKENS = 10
def setUp(self):
"""
Setup quantized model from loaded model
"""
from transformers import AutoModelForCausalLM, AutoTokenizer
# Models and tokenizer
self.model_fp16 = AutoModelForCausalLM.from_pretrained(
self.model_name, torch_dtype=torch.float16, device_map="auto"
)
self.bnb_quantization_config = BnbQuantizationConfig(load_in_8bit=True)
self.model_8bit = AutoModelForCausalLM.from_pretrained(self.model_name, torch_dtype=torch.float16)
self.model_8bit = load_and_quantize_model(self.model_8bit, self.bnb_quantization_config)
self.tokenizer = AutoTokenizer.from_pretrained("bigscience/bloom-1b7")
def tearDown(self):
r"""
TearDown function needs to be called at the end of each test to free the GPU memory and cache, also to
avoid unexpected behaviors. Please see: https://discuss.pytorch.org/t/how-can-we-release-gpu-memory-cache/14530/27
"""
del self.model_fp16
del self.model_8bit
gc.collect()
torch.cuda.empty_cache()
def test_memory_footprint(self):
r"""
A simple test to check if the model conversion has been done correctly by checking on the
memory footprint of the converted model and the class type of the linear layers of the converted models
"""
from bitsandbytes.nn import Int8Params
mem_fp16 = self.model_fp16.get_memory_footprint()
mem_8bit = self.model_8bit.get_memory_footprint()
self.assertAlmostEqual(mem_fp16 / mem_8bit, self.EXPECTED_RELATIVE_DIFFERENCE)
self.assertTrue(self.model_8bit.transformer.h[0].mlp.dense_4h_to_h.weight.__class__ == Int8Params)
def test_linear_are_8bit(self):
r"""
A simple test to check if the model conversion has been done correctly by checking on the
memory footprint of the converted model and the class type of the linear layers of the converted models
"""
self.model_fp16.get_memory_footprint()
self.model_8bit.get_memory_footprint()
for name, module in self.model_8bit.named_modules():
if isinstance(module, torch.nn.Linear):
modules_not_converted = (
self.bnb_quantization_config.keep_in_fp32_modules + self.bnb_quantization_config.skip_modules
)
if name not in modules_not_converted:
self.assertTrue(module.weight.dtype == torch.int8)
def test_generate_quality(self):
r"""
Test the generation quality of the quantized model and see that we are matching the expected output.
Given that we are operating on small numbers + the testing model is relatively small, we might not get
the same output across GPUs. So we'll generate few tokens (5-10) and check their output.
"""
encoded_input = self.tokenizer(self.input_text, return_tensors="pt")
output_sequences = self.model_8bit.generate(
input_ids=encoded_input["input_ids"].to(self.model_8bit.device), max_new_tokens=10
)
self.assertEqual(self.tokenizer.decode(output_sequences[0], skip_special_tokens=True), self.EXPECTED_OUTPUT)
def test_fp32_8bit_conversion(self):
r"""
Test whether it is possible to mix both `8bit` and `fp32` weights when using `keep_in_fp32_modules` correctly.
"""
from transformers import AutoModelForCausalLM
bnb_quantization_config = BnbQuantizationConfig(load_in_8bit=True, keep_in_fp32_modules=["lm_head"])
model = AutoModelForCausalLM.from_pretrained(self.model_name, torch_dtype=torch.float16)
model = load_and_quantize_model(model, bnb_quantization_config)
self.assertTrue(model.lm_head.weight.dtype == torch.float32)
@slow
@require_cuda
@require_bnb
@require_huggingface_suite
class Bnb4BitEmptyModelTest(unittest.TestCase):
# We keep the constants inside the init function and model loading inside setUp function
# We need to test on relatively large models (aka >1b parameters otherwise the quantiztion may not work as expected)
# Therefore here we use only bloom-1b3 to test our module
model_name = "marcsun13/bloom-1b7_with_lm_head"
# Constant values
# This was obtained on a RTX Titan so the number might slightly change
EXPECTED_RELATIVE_DIFFERENCE = 2.109659552692574
input_text = "Hello my name is"
EXPECTED_OUTPUTS = set()
EXPECTED_OUTPUTS.add("Hello my name is John and I am a professional photographer. I")
EXPECTED_OUTPUTS.add("Hello my name is John.\nI am a friend of your father.\n")
MAX_NEW_TOKENS = 10
def setUp(self):
from huggingface_hub import hf_hub_download
from transformers import AutoConfig, AutoModelForCausalLM, AutoTokenizer
super().setUp()
# Models and tokenizer
self.model_fp16 = AutoModelForCausalLM.from_pretrained(
self.model_name, torch_dtype=torch.float16, device_map="auto"
)
# create model on meta device
with init_empty_weights():
self.model_4bit = AutoModelForCausalLM.from_config(AutoConfig.from_pretrained(self.model_name))
self.model_4bit.tie_weights()
self.weights_location = hf_hub_download(self.model_name, "pytorch_model.bin")
self.bnb_quantization_config = BnbQuantizationConfig(load_in_4bit=True)
self.model_4bit = load_and_quantize_model(
self.model_4bit,
self.bnb_quantization_config,
weights_location=self.weights_location,
device_map={"": 0},
no_split_module_classes=["BloomBlock"],
)
self.tokenizer = AutoTokenizer.from_pretrained("bigscience/bloom-1b7")
def tearDown(self):
"""
TearDown function needs to be called at the end of each test to free the GPU memory and cache, also to
avoid unexpected behaviors. Please see: https://discuss.pytorch.org/t/how-can-we-release-gpu-memory-cache/14530/27
"""
super().tearDown()
del self.model_fp16
del self.model_4bit
gc.collect()
torch.cuda.empty_cache()
def test_memory_footprint(self):
r"""
A simple test to check if the model conversion has been done correctly by checking on the
memory footprint of the converted model and the class type of the linear layers of the converted models
"""
from bitsandbytes.nn import Params4bit
mem_fp16 = self.model_fp16.get_memory_footprint()
mem_4bit = self.model_4bit.get_memory_footprint()
self.assertAlmostEqual(mem_fp16 / mem_4bit, self.EXPECTED_RELATIVE_DIFFERENCE)
self.assertTrue(self.model_4bit.transformer.h[0].mlp.dense_4h_to_h.weight.__class__ == Params4bit)
def check_inference_correctness(self, model):
r"""
Test the generation quality of the quantized model and see that we are matching the expected output.
Given that we are operating on small numbers + the testing model is relatively small, we might not get
the same output across GPUs. So we'll generate few tokens (5-10) and check their output.
"""
# Check that inference pass works on the model
encoded_input = self.tokenizer(self.input_text, return_tensors="pt")
# Check the exactness of the results
output_sequences = model.generate(input_ids=encoded_input["input_ids"].to(0), max_new_tokens=10)
self.assertIn(self.tokenizer.decode(output_sequences[0], skip_special_tokens=True), self.EXPECTED_OUTPUTS)
def test_generate_quality(self):
self.check_inference_correctness(self.model_4bit)
def test_linear_are_4bit(self):
r"""
A simple test to check if the model conversion has been done correctly by checking on the
memory footprint of the converted model and the class type of the linear layers of the converted models
"""
self.model_fp16.get_memory_footprint()
self.model_4bit.get_memory_footprint()
for name, module in self.model_4bit.named_modules():
if isinstance(module, torch.nn.Linear):
if (
name
not in self.bnb_quantization_config.keep_in_fp32_modules
+ self.bnb_quantization_config.skip_modules
):
# 4-bit parameters are packed in uint8 variables
self.assertTrue(module.weight.dtype == torch.uint8)
def test_fp32_4bit_conversion(self):
r"""
Test whether it is possible to mix both `4bit` and `fp32` weights when using `keep_in_fp32_modules` correctly.
"""
from transformers import AutoConfig, AutoModelForCausalLM
bnb_quantization_config = BnbQuantizationConfig(load_in_4bit=True, keep_in_fp32_modules=["lm_head"])
with init_empty_weights():
model = AutoModelForCausalLM.from_config(AutoConfig.from_pretrained(self.model_name))
model.tie_weights()
model = load_and_quantize_model(
model,
bnb_quantization_config,
weights_location=self.weights_location,
device_map="auto",
no_split_module_classes=["BloomBlock"],
)
self.assertTrue(model.lm_head.weight.dtype == torch.float32)
@require_multi_gpu
def test_cpu_gpu_loading_random_device_map(self):
from transformers import AutoConfig, AutoModelForCausalLM
r"""
A test to check is dispatching a model on cpu & gpu works correctly using a random `device_map`.
"""
device_map = {
"transformer.word_embeddings": "cpu",
"transformer.word_embeddings_layernorm": 0,
"lm_head": "cpu",
"transformer.h.0": 0,
"transformer.h.1": 0,
"transformer.h.2": 0,
"transformer.h.3": 0,
"transformer.h.4": 0,
"transformer.h.5": 0,
"transformer.h.6": 0,
"transformer.h.7": 0,
"transformer.h.8": 0,
"transformer.h.9": 1,
"transformer.h.10": 0,
"transformer.h.11": 1,
"transformer.h.12": 0,
"transformer.h.13": 0,
"transformer.h.14": 1,
"transformer.h.15": 0,
"transformer.h.16": 0,
"transformer.h.17": 1,
"transformer.h.18": 1,
"transformer.h.19": 0,
"transformer.h.20": 1,
"transformer.h.21": 1,
"transformer.h.22": 0,
"transformer.h.23": 0,
"transformer.ln_f": 1,
}
bnb_quantization_config = BnbQuantizationConfig(load_in_4bit=True)
with init_empty_weights():
model_4bit = AutoModelForCausalLM.from_config(AutoConfig.from_pretrained(self.model_name))
model_4bit.tie_weights()
model_4bit = load_and_quantize_model(
model_4bit,
bnb_quantization_config,
weights_location=self.weights_location,
device_map=device_map,
no_split_module_classes=["BloomBlock"],
)
self.check_inference_correctness(model_4bit)
@require_multi_gpu
def test_cpu_gpu_loading_custom_device_map(self):
from transformers import AutoConfig, AutoModelForCausalLM
r"""
A test to check is dispatching a model on cpu & gpu works correctly using a random `device_map`.
"""
device_map = {
"transformer.word_embeddings": "cpu",
"transformer.word_embeddings_layernorm": "cpu",
"lm_head": "cpu",
"transformer.h": 0,
"transformer.ln_f": 1,
}
bnb_quantization_config = BnbQuantizationConfig(load_in_4bit=True)
with init_empty_weights():
model_4bit = AutoModelForCausalLM.from_config(AutoConfig.from_pretrained(self.model_name))
model_4bit.tie_weights()
model_4bit = load_and_quantize_model(
model_4bit,
bnb_quantization_config,
weights_location=self.weights_location,
device_map=device_map,
no_split_module_classes=["BloomBlock"],
)
self.check_inference_correctness(model_4bit)
@require_multi_gpu
def test_cpu_gpu_disk_loading_custom_device_map_kwargs(self):
from transformers import AutoConfig, AutoModelForCausalLM
r"""
A test to check is dispatching a model on cpu & gpu works correctly using a custom `device_map`.
This time we also add `disk` on the device_map - using the kwargs directly instead of the quantization config
"""
device_map = {
"transformer.word_embeddings": 0,
"transformer.word_embeddings_layernorm": "disk",
"lm_head": 0,
"transformer.h": 1,
"transformer.ln_f": "cpu",
}
bnb_quantization_config = BnbQuantizationConfig(load_in_4bit=True)
with init_empty_weights():
model_4bit = AutoModelForCausalLM.from_config(AutoConfig.from_pretrained(self.model_name))
model_4bit.tie_weights()
with tempfile.TemporaryDirectory() as tmpdirname:
model_4bit = load_and_quantize_model(
model_4bit,
bnb_quantization_config,
weights_location=self.weights_location,
device_map=device_map,
no_split_module_classes=["BloomBlock"],
offload_folder=tmpdirname,
offload_state_dict=True,
)
self.check_inference_correctness(model_4bit)
@slow
@require_cuda
@require_bnb
@require_huggingface_suite
class Bnb4BitTestLoadedModel(unittest.TestCase):
# We keep the constants inside the init function and model loading inside setUp function
# We need to test on relatively large models (aka >1b parameters otherwise the quantiztion may not work as expected)
# Therefore here we use only bloom-1b3 to test our module
model_name = "marcsun13/bloom-1b7_with_lm_head"
# Constant values
# This was obtained on a RTX Titan so the number might slightly change
EXPECTED_RELATIVE_DIFFERENCE = 2.109659552692574
input_text = "Hello my name is"
EXPECTED_OUTPUTS = set()
EXPECTED_OUTPUTS.add("Hello my name is John and I am a professional photographer. I")
EXPECTED_OUTPUTS.add("Hello my name is John.\nI am a friend of your father.\n")
MAX_NEW_TOKENS = 10
def setUp(self):
"""
Setup quantized model from loaded model
"""
from transformers import AutoModelForCausalLM, AutoTokenizer
super().setUp()
# Models and tokenizer
self.model_fp16 = AutoModelForCausalLM.from_pretrained(
self.model_name, torch_dtype=torch.float16, device_map="auto"
)
self.bnb_quantization_config = BnbQuantizationConfig(load_in_4bit=True)
self.model_4bit = AutoModelForCausalLM.from_pretrained(self.model_name, torch_dtype=torch.float16)
self.model_4bit = load_and_quantize_model(self.model_4bit, self.bnb_quantization_config)
self.tokenizer = AutoTokenizer.from_pretrained("bigscience/bloom-1b7")
def tearDown(self):
"""
TearDown function needs to be called at the end of each test to free the GPU memory and cache, also to
avoid unexpected behaviors. Please see: https://discuss.pytorch.org/t/how-can-we-release-gpu-memory-cache/14530/27
"""
super().tearDown()
del self.model_fp16
del self.model_4bit
gc.collect()
torch.cuda.empty_cache()
def test_memory_footprint(self):
r"""
A simple test to check if the model conversion has been done correctly by checking on the
memory footprint of the converted model and the class type of the linear layers of the converted models
"""
from bitsandbytes.nn import Params4bit
mem_fp16 = self.model_fp16.get_memory_footprint()
mem_4bit = self.model_4bit.get_memory_footprint()
self.assertAlmostEqual(mem_fp16 / mem_4bit, self.EXPECTED_RELATIVE_DIFFERENCE)
self.assertTrue(self.model_4bit.transformer.h[0].mlp.dense_4h_to_h.weight.__class__ == Params4bit)
def test_linear_are_4bit(self):
r"""
A simple test to check if the model conversion has been done correctly by checking on the
memory footprint of the converted model and the class type of the linear layers of the converted models
"""
self.model_fp16.get_memory_footprint()
self.model_4bit.get_memory_footprint()
for name, module in self.model_4bit.named_modules():
if isinstance(module, torch.nn.Linear):
if (
name
not in self.bnb_quantization_config.keep_in_fp32_modules
+ self.bnb_quantization_config.skip_modules
):
# 4-bit parameters are packed in uint8 variables
self.assertTrue(module.weight.dtype == torch.uint8)
def test_generate_quality(self):
r"""
Test the generation quality of the quantized model and see that we are matching the expected output.
Given that we are operating on small numbers + the testing model is relatively small, we might not get
the same output across GPUs. So we'll generate few tokens (5-10) and check their output.
"""
encoded_input = self.tokenizer(self.input_text, return_tensors="pt")
output_sequences = self.model_4bit.generate(
input_ids=encoded_input["input_ids"].to(self.model_4bit.device), max_new_tokens=10
)
self.assertIn(self.tokenizer.decode(output_sequences[0], skip_special_tokens=True), self.EXPECTED_OUTPUTS)
def test_fp32_4bit_conversion(self):
r"""
Test whether it is possible to mix both `4bit` and `fp32` weights when using `keep_in_fp32_modules` correctly.
"""
from transformers import AutoModelForCausalLM
bnb_quantization_config = BnbQuantizationConfig(load_in_4bit=True, keep_in_fp32_modules=["lm_head"])
model = AutoModelForCausalLM.from_pretrained(self.model_name, torch_dtype=torch.float16)
model = load_and_quantize_model(model, bnb_quantization_config)
self.assertTrue(model.lm_head.weight.dtype == torch.float32)
| accelerate/tests/test_quantization.py/0 | {
"file_path": "accelerate/tests/test_quantization.py",
"repo_id": "accelerate",
"token_count": 17759
} | 10 |
- title: Unit 0. Welcome to the RLHF Handbook!
sections:
- local: chapter0/introduction
title: What is this about? | alignment-handbook/chapters/en/_toctree.yml/0 | {
"file_path": "alignment-handbook/chapters/en/_toctree.yml",
"repo_id": "alignment-handbook",
"token_count": 38
} | 11 |