TLCM: Training-efficient Latent Consistency Model for Image Generation with 2-8 Steps
๐ Paper โข ๐ค Checkpoints
we propose an innovative two-stage data-free consistency distillation (TDCD) approach to accelerate latent consistency model. The first stage improves consistency constraint by data-free sub-segment consistency distillation (DSCD). The second stage enforces the global consistency across inter-segments through data-free consistency distillation (DCD). Besides, we explore various techniques to promote TLCMโs performance in data-free manner, forming Training-efficient Latent Consistency Model (TLCM) with 2-8 step inference.
TLCM demonstrates a high level of flexibility by enabling adjustment of sampling steps within the range of 2 to 8 while still producing competitive outputs compared to full-step approaches.
Install Dependency
pip install diffusers
pip install transformers accelerate
or try
pip install prefetch_generator zhconv peft loguru transformers==4.39.1 accelerate==0.31.0
Example Use
We provide an example inference script in the directory of this repo. You should download the Lora path from here and use a base model, such as SDXL1.0 , as the recommended option. After that, you can activate the generation with the following code:
python inference.py --prompt {Your prompt} --output_dir {Your output directory} --lora_path {Lora_directory} --base_model_path {Base_model_directory} --infer-steps 4
More parameters are presented in paras.py. You can modify them according to your requirements.
๐ Update ๐
We integrate LCMScheduler in the diffuser pipeline for our workflow, so now you can now use a simpler version below with the base model SDXL 1.0, and we highly recommend it :
import torch,diffusers
from diffusers import LCMScheduler,AutoPipelineForText2Image
from peft import LoraConfig, get_peft_model
model_id = "stabilityai/stable-diffusion-xl-base-1.0"
lora_path = 'path/to/the/lora'
lora_config = LoraConfig(
r=64,
target_modules=[
"to_q",
"to_k",
"to_v",
"to_out.0",
"proj_in",
"proj_out",
"ff.net.0.proj",
"ff.net.2",
"conv1",
"conv2",
"conv_shortcut",
"downsamplers.0.conv",
"upsamplers.0.conv",
"time_emb_proj",
],
)
pipe = AutoPipelineForText2Image.from_pretrained(model_id,torch_dtype=torch.float16, variant="fp16")
pipe.scheduler = LCMScheduler.from_config(pipe.scheduler.config)
unet=pipe.unet
unet = get_peft_model(unet, lora_config)
unet.load_adapter(lora_path, adapter_name="default")
pipe.unet=unet
pipe.to('cuda')
eval_step=4 # the step can be changed within 2-8 steps
prompt = "An astronaut riding a horse in the jungle"
# disable guidance_scale by passing 0
image = pipe(prompt=prompt, num_inference_steps=eval_step, guidance_scale=0).images[0]
We also adapt our methods based on FLUX model. You can down load the corresponding LoRA model here and load it with the base model for faster sampling. The sampling script for faster FLUX sampling as below:
import os,torch
from diffusers import FluxPipeline
from scheduling_flow_match_tlcm import FlowMatchEulerTLCMScheduler
from peft import LoraConfig, get_peft_model
model_id = "black-forest-labs/FLUX.1-dev"
lora_path = "path/to/the/lora/folder"
lora_config = LoraConfig(
r=64,
target_modules=[
"to_k", "to_q", "to_v", "to_out.0",
"proj_in",
"proj_out",
"ff.net.0.proj",
"ff.net.2",
"context_embedder", "x_embedder",
"linear", "linear_1", "linear_2",
"proj_mlp",
"add_k_proj", "add_q_proj", "add_v_proj", "to_add_out",
"ff_context.net.0.proj", "ff_context.net.2"
],
)
pipe = FluxPipeline.from_pretrained(model_id, torch_dtype=torch.bfloat16)
pipe.scheduler = FlowMatchEulerTLCMScheduler.from_config(pipe.scheduler.config)
pipe.to('cuda:0')
transformer = pipe.transformer
transformer = get_peft_model(transformer, lora_config)
transformer.load_adapter(lora_path, adapter_name="default", is_trainable=False)
pipe.transformer=transformer
eval_step=4 # the step can be changed within 2-8 steps
prompt = "An astronaut riding a horse in the jungle"
image = pipe(prompt=prompt, num_inference_steps=eval_step, guidance_scale=7).images[0]
Art Gallery
Here we present some examples based on SDXL with different samping steps.
2-Steps Sampling

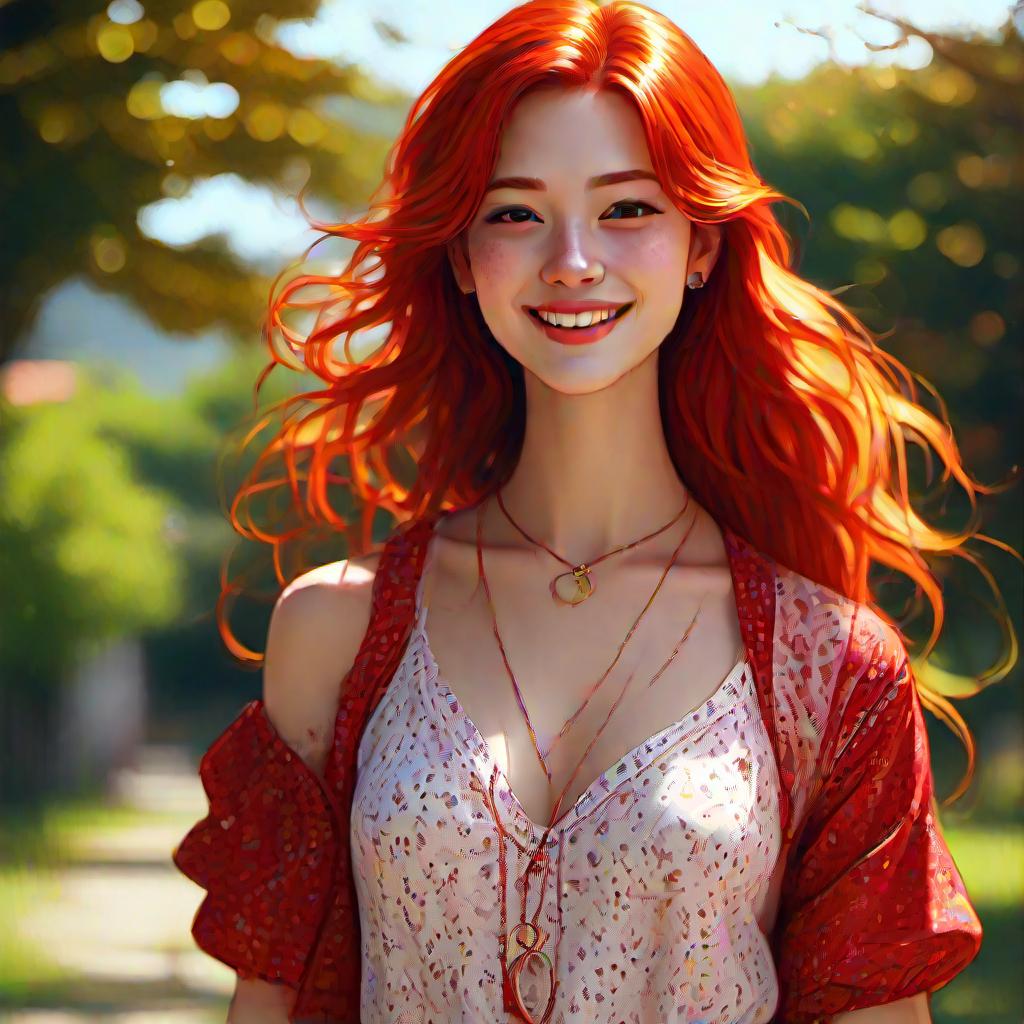


3-Steps Sampling

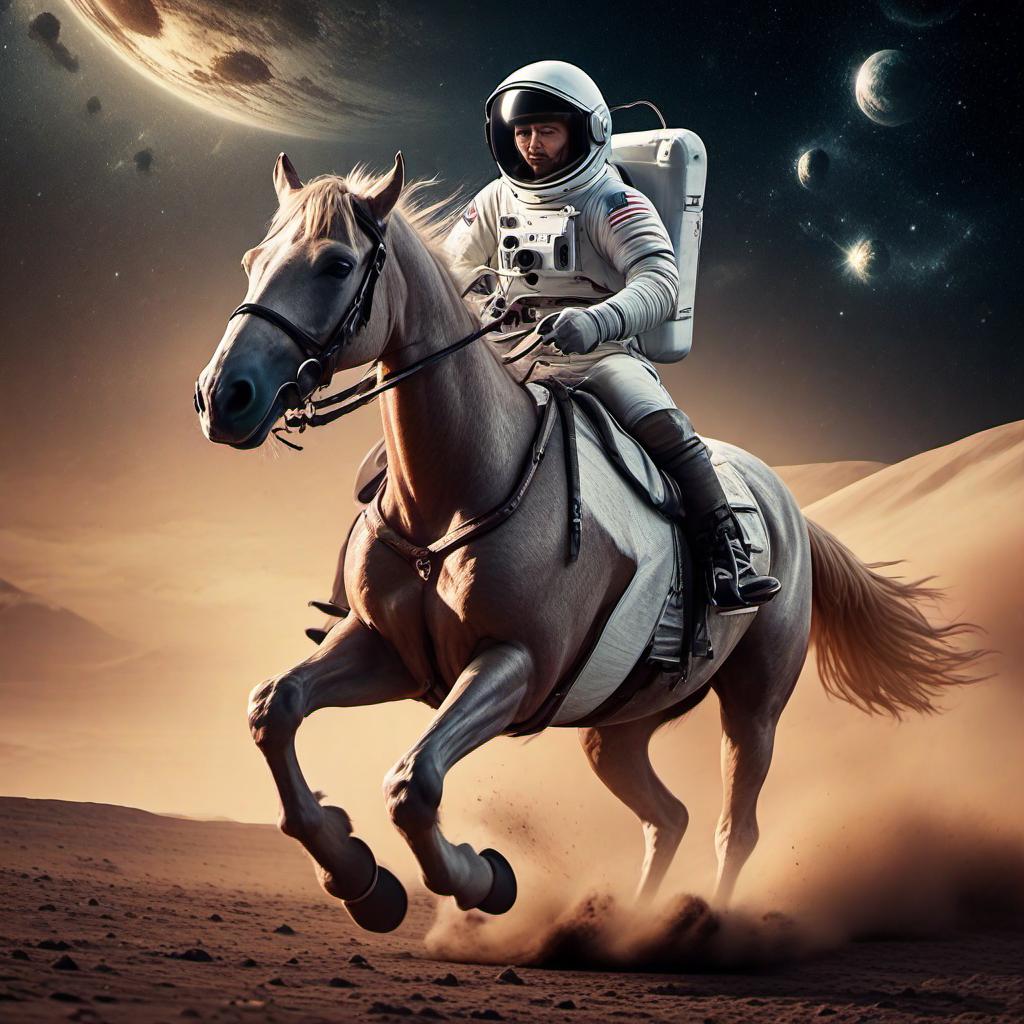


4-Steps Sampling
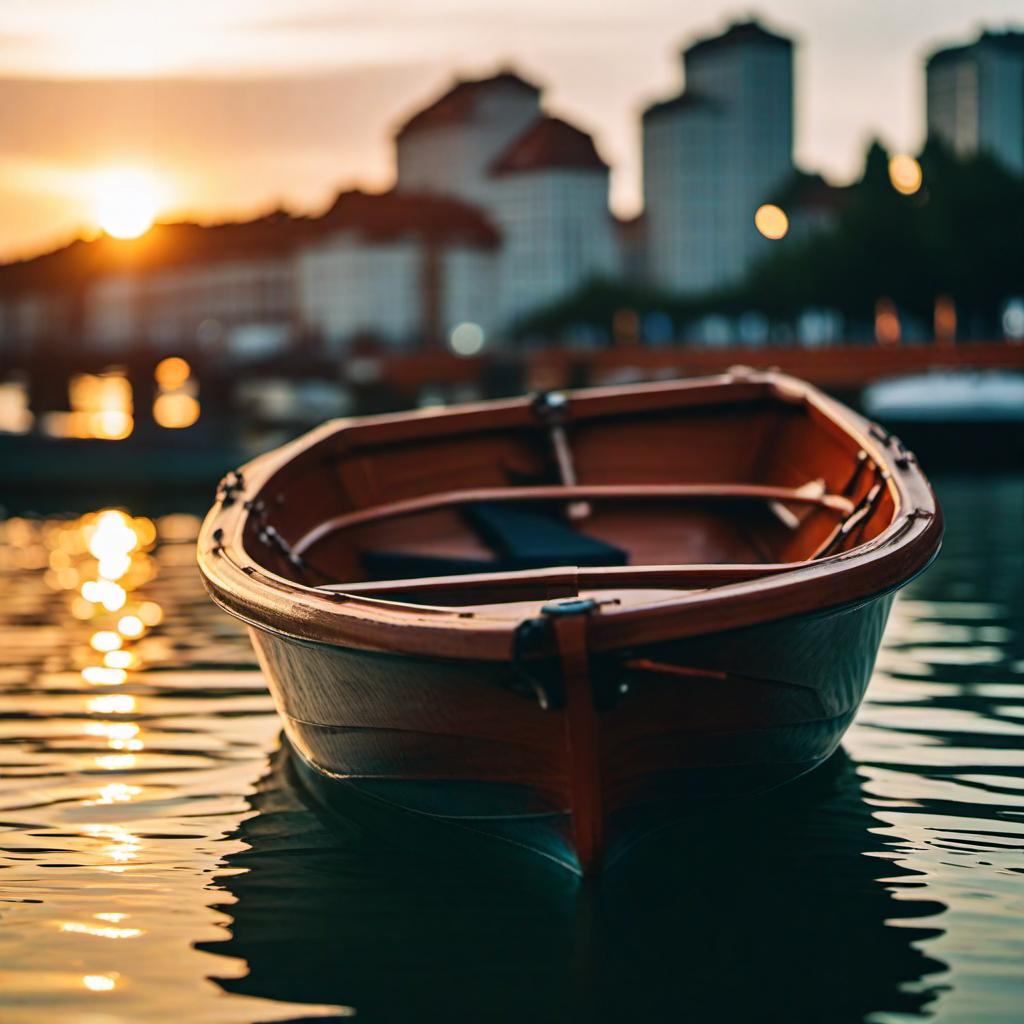



8-Steps Sampling



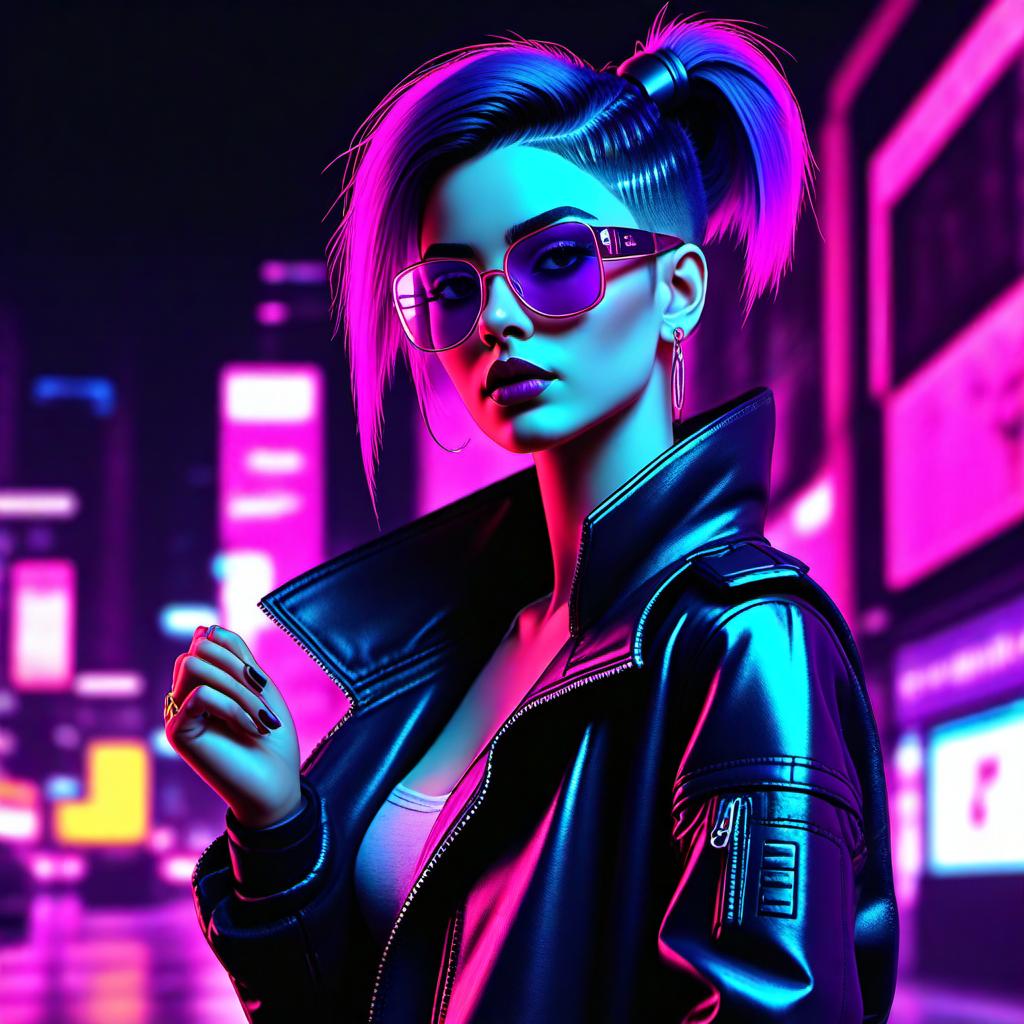
We also present some examples based on FLUX.
3-Steps Sampling

eyes behind glasses...

inside an opulent palace...

replace... with cityscape

blue eyes...
4-Steps Sampling
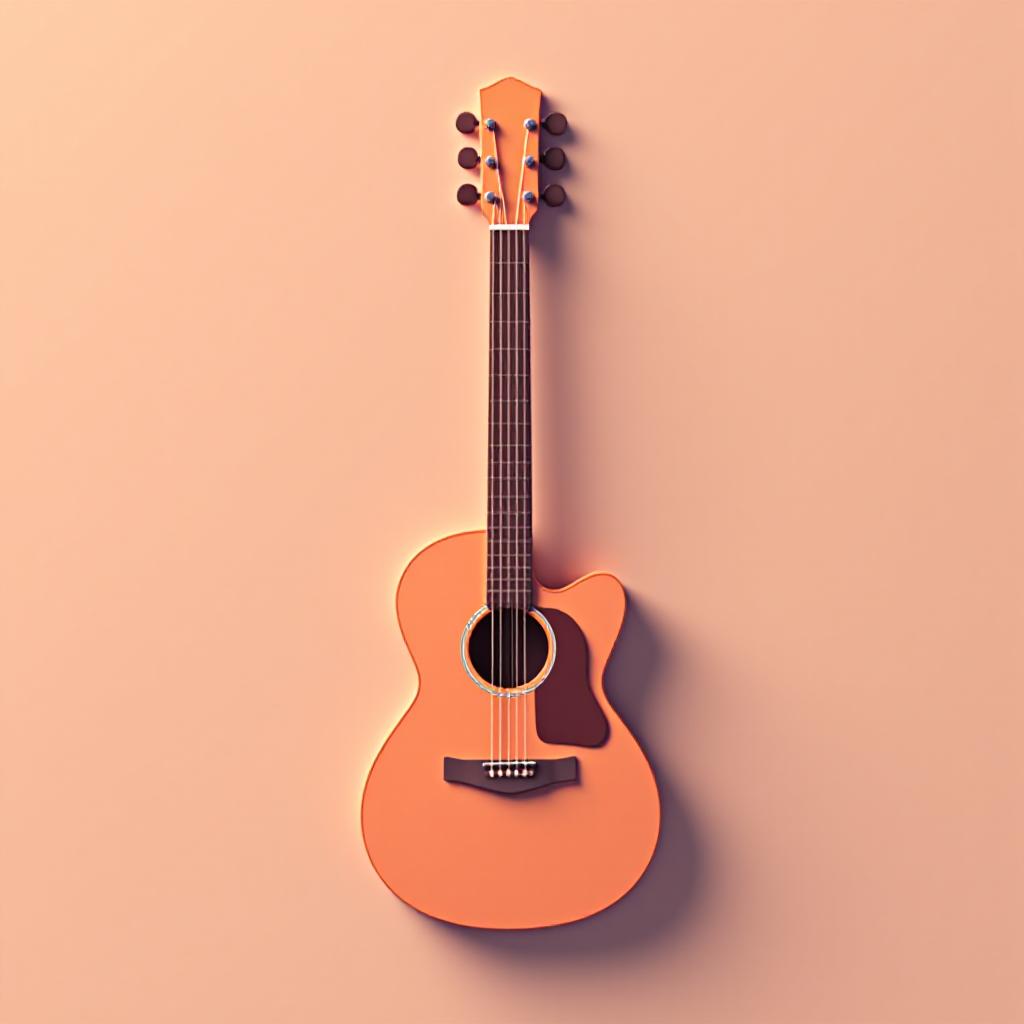
2d minimalistic icon...
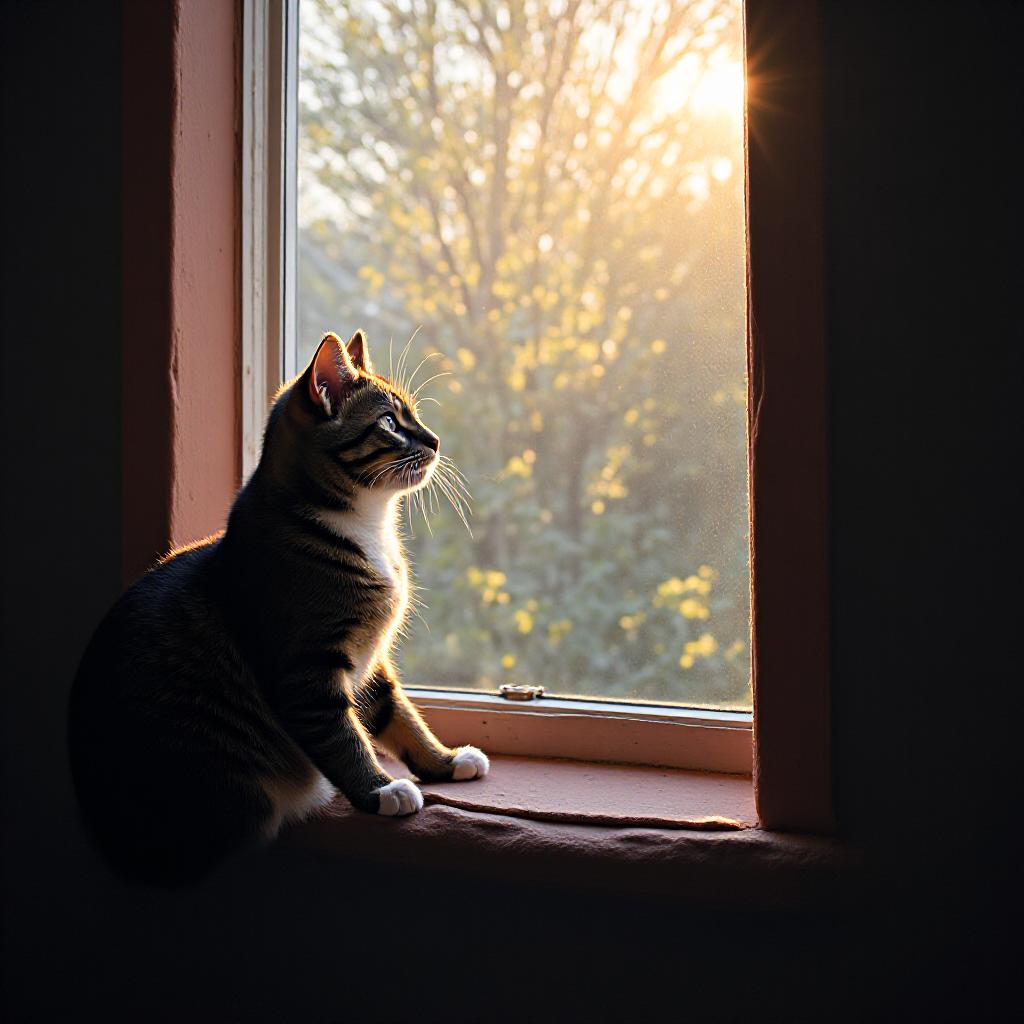
near the window...

forest in spring...

...a vibrant cherry blossom...
6-Steps Sampling

on the grass...

in glass kettle...

luxury product style...
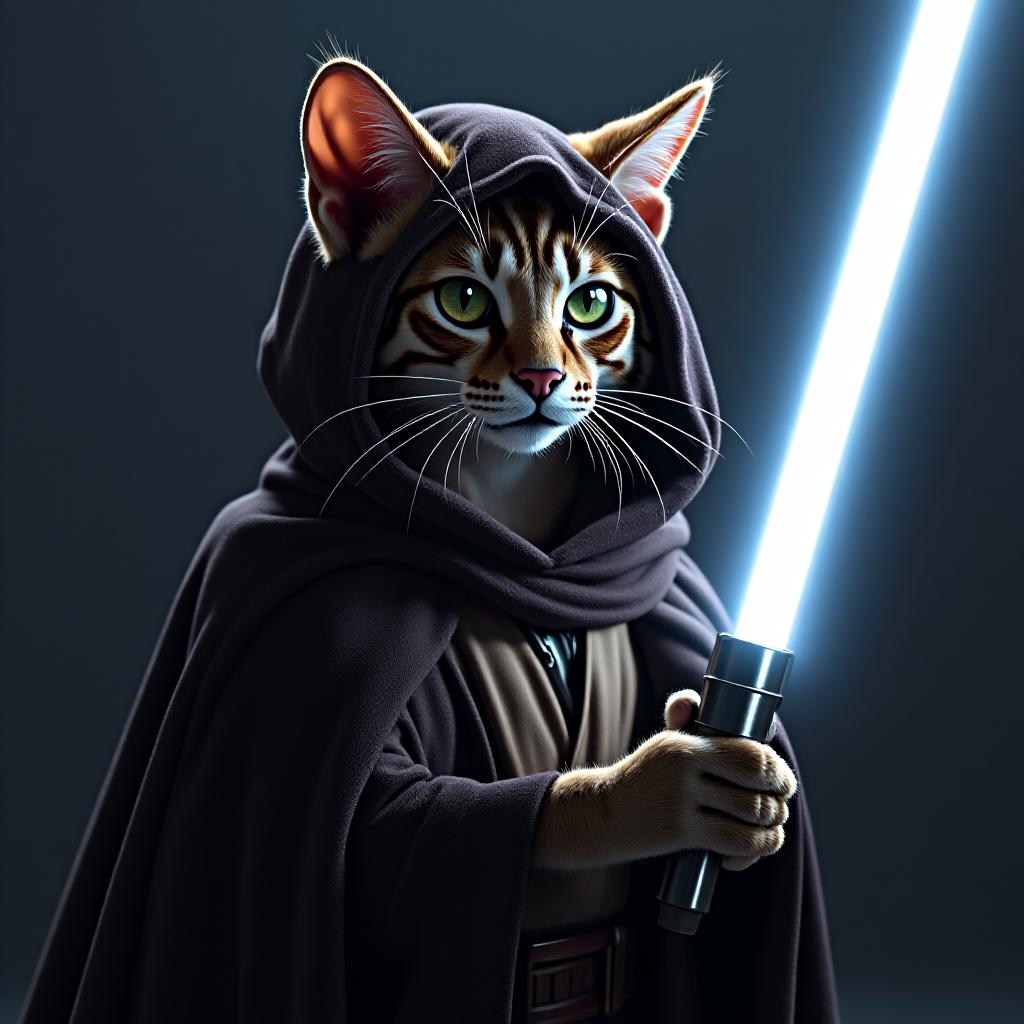
wearing a jedi cloak hood
8-Steps Sampling

low-poly game art...

blurred motion...
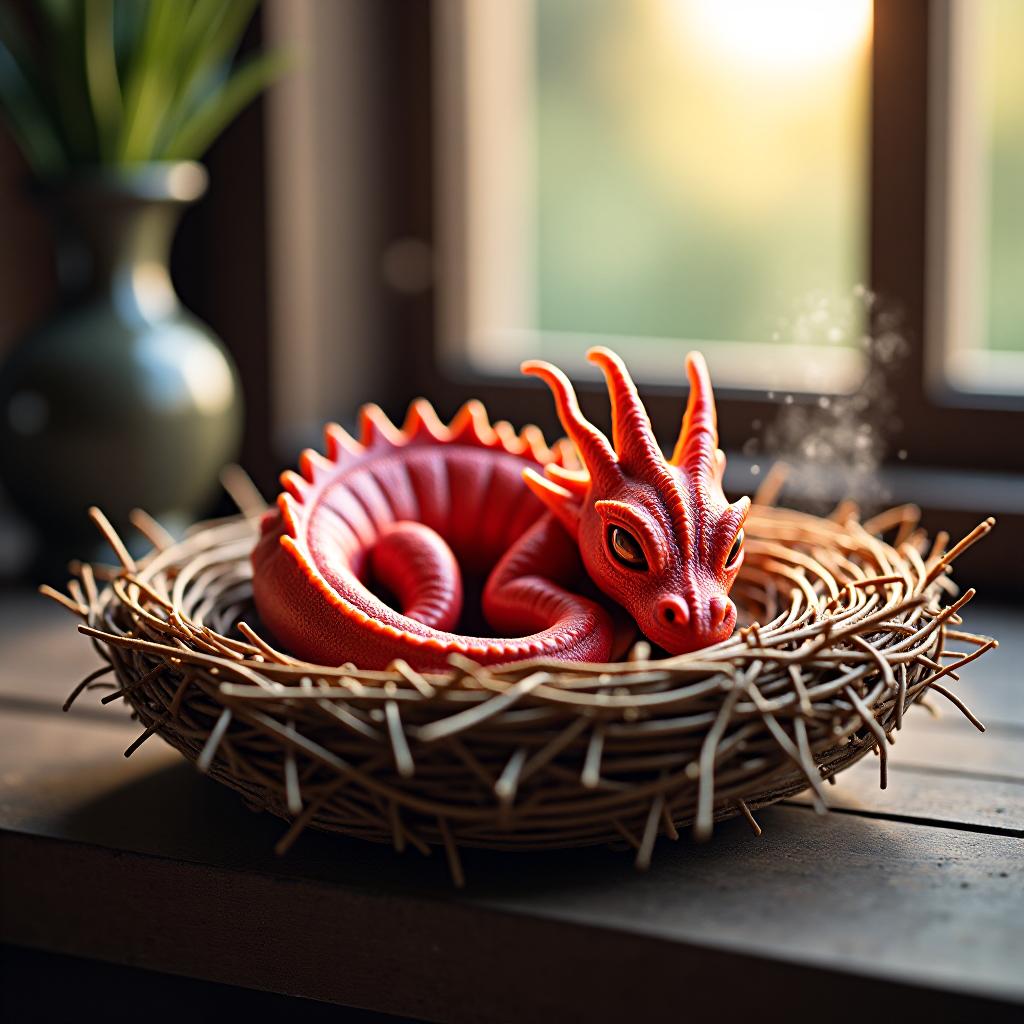
curled up in a nest...
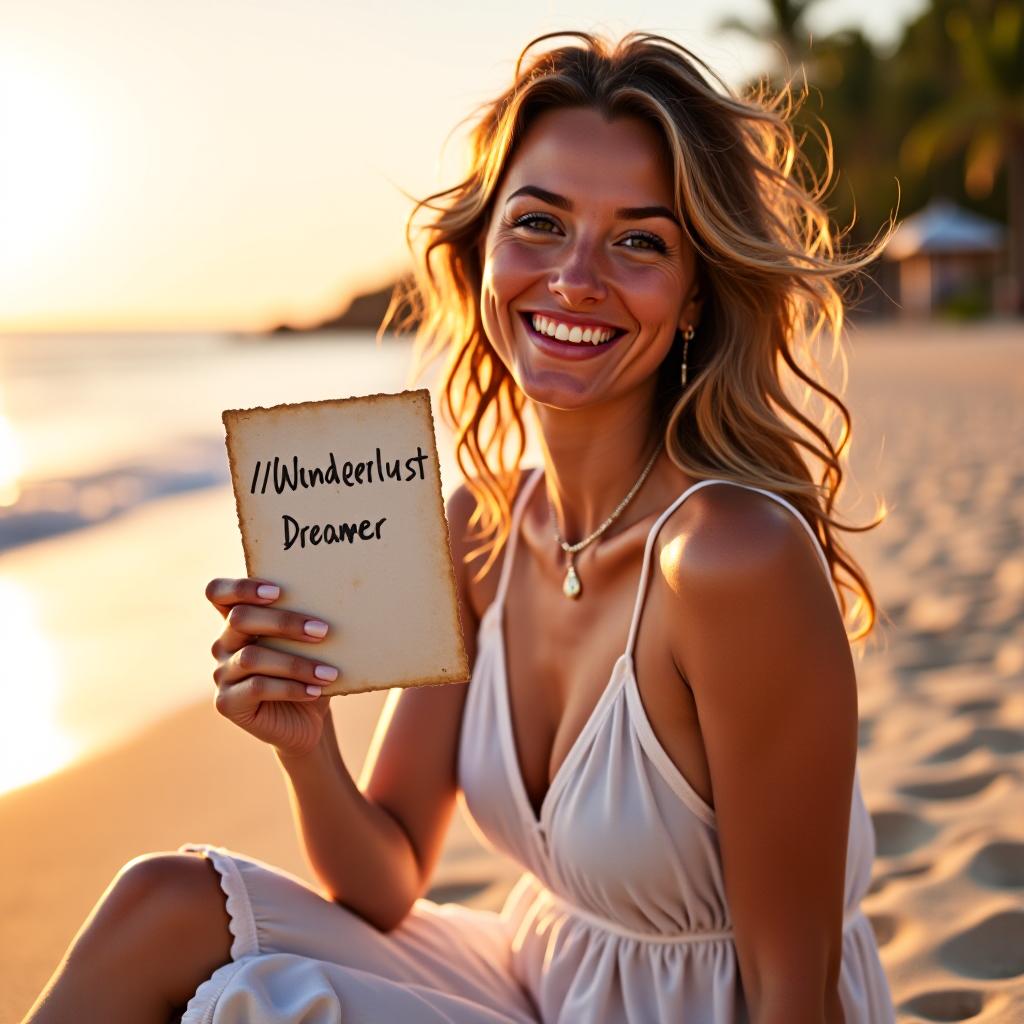
with "WanderlustDreamer"
Addition
We also provide the latent lpips model here. More details are presented in the paper.
Citation
@article{xie2024tlcm,
title={TLCM: Training-efficient Latent Consistency Model for Image Generation with 2-8 Steps},
author={Xie, Qingsong and Liao, Zhenyi and Deng, Zhijie and Lu, Haonan},
journal={arXiv preprint arXiv:2406.05768},
year={2024}
}
- Downloads last month
- 0
Model tree for OPPOer/TLCM
Base model
black-forest-labs/FLUX.1-dev