File size: 11,975 Bytes
0ad74ed |
1 2 3 4 5 6 7 8 9 10 11 12 13 14 15 16 17 18 19 20 21 22 23 24 25 26 27 28 29 30 31 32 33 34 35 36 37 38 39 40 41 42 43 44 45 46 47 48 49 50 51 52 53 54 55 56 57 58 59 60 61 62 63 64 65 66 67 68 69 70 71 72 73 74 75 76 77 78 79 80 81 82 83 84 85 86 87 88 89 90 91 92 93 94 95 96 97 98 99 100 101 102 103 104 105 106 107 108 109 110 111 112 113 114 115 116 117 118 119 120 121 122 123 124 125 126 127 128 129 130 131 132 133 134 135 136 137 138 139 140 141 142 143 144 145 146 147 148 149 150 151 152 153 154 155 156 157 158 159 160 161 162 163 164 165 166 167 168 169 170 171 172 173 174 175 176 177 178 179 180 181 182 183 184 185 186 187 188 189 190 191 192 193 194 195 196 197 198 199 200 201 202 203 204 205 206 207 208 209 210 211 212 213 214 215 216 217 218 219 220 221 222 223 224 225 226 227 228 229 230 231 232 233 234 235 236 237 238 239 240 241 242 243 244 245 246 247 248 249 250 251 252 253 254 255 256 257 258 259 260 261 262 263 264 265 266 267 268 269 270 271 272 273 274 275 276 277 278 279 280 281 282 283 284 285 286 287 288 289 290 291 292 293 294 295 296 297 298 299 300 301 302 303 304 305 306 307 308 309 310 311 312 313 314 315 316 317 318 319 320 321 322 323 324 325 326 327 328 329 330 331 332 333 334 335 336 337 338 339 340 341 342 343 344 345 346 347 348 349 350 351 352 353 354 355 356 357 358 |
# Build a Custom Multimodal Chatbot - Part 1
This is the first in a two part series where we build a custom Multimodal Chatbot component.
In part 1, we will modify the Gradio Chatbot component to display text and media files (video, audio, image) in the same message.
In part 2, we will build a custom Textbox component that will be able to send multimodal messages (text and media files) to the chatbot.
You can follow along with the author of this post as he implements the chatbot component in the following YouTube video!
<iframe width="560" height="315" src="https://www.youtube.com/embed/IVJkOHTBPn0?si=bs-sBv43X-RVA8ly" title="YouTube video player" frameborder="0" allow="accelerometer; autoplay; clipboard-write; encrypted-media; gyroscope; picture-in-picture; web-share" allowfullscreen></iframe>
Here's a preview of what our multimodal chatbot component will look like:
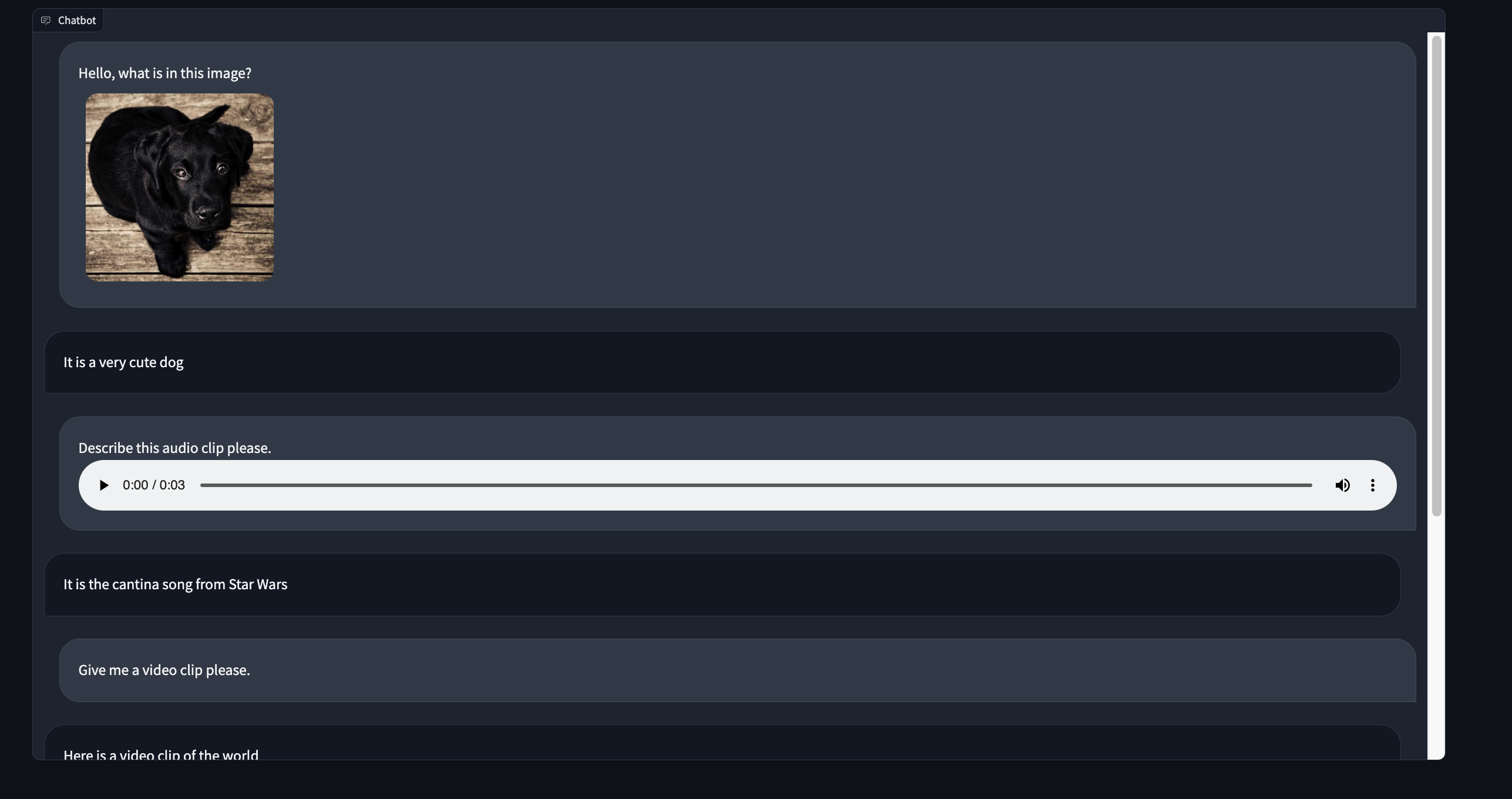
## Part 1 - Creating our project
For this demo we will be tweaking the existing Gradio `Chatbot` component to display text and media files in the same message.
Let's create a new custom component directory by templating off of the `Chatbot` component source code.
```bash
gradio cc create MultimodalChatbot --template Chatbot
```
And we're ready to go!
Tip: Make sure to modify the `Author` key in the `pyproject.toml` file.
## Part 2a - The backend data_model
Open up the `multimodalchatbot.py` file in your favorite code editor and let's get started modifying the backend of our component.
The first thing we will do is create the `data_model` of our component.
The `data_model` is the data format that your python component will receive and send to the javascript client running the UI.
You can read more about the `data_model` in the [backend guide](./backend).
For our component, each chatbot message will consist of two keys: a `text` key that displays the text message and an optional list of media files that can be displayed underneath the text.
Import the `FileData` and `GradioModel` classes from `gradio.data_classes` and modify the existing `ChatbotData` class to look like the following:
```python
class FileMessage(GradioModel):
file: FileData
alt_text: Optional[str] = None
class MultimodalMessage(GradioModel):
text: Optional[str] = None
files: Optional[List[FileMessage]] = None
class ChatbotData(GradioRootModel):
root: List[Tuple[Optional[MultimodalMessage], Optional[MultimodalMessage]]]
class MultimodalChatbot(Component):
...
data_model = ChatbotData
```
Tip: The `data_model`s are implemented using `Pydantic V2`. Read the documentation [here](https://docs.pydantic.dev/latest/).
We've done the hardest part already!
## Part 2b - The pre and postprocess methods
For the `preprocess` method, we will keep it simple and pass a list of `MultimodalMessage`s to the python functions that use this component as input.
This will let users of our component access the chatbot data with `.text` and `.files` attributes.
This is a design choice that you can modify in your implementation!
We can return the list of messages with the `root` property of the `ChatbotData` like so:
```python
def preprocess(
self,
payload: ChatbotData | None,
) -> List[MultimodalMessage] | None:
if payload is None:
return payload
return payload.root
```
Tip: Learn about the reasoning behind the `preprocess` and `postprocess` methods in the [key concepts guide](./key-component-concepts)
In the `postprocess` method we will coerce each message returned by the python function to be a `MultimodalMessage` class.
We will also clean up any indentation in the `text` field so that it can be properly displayed as markdown in the frontend.
We can leave the `postprocess` method as is and modify the `_postprocess_chat_messages`
```python
def _postprocess_chat_messages(
self, chat_message: MultimodalMessage | dict | None
) -> MultimodalMessage | None:
if chat_message is None:
return None
if isinstance(chat_message, dict):
chat_message = MultimodalMessage(**chat_message)
chat_message.text = inspect.cleandoc(chat_message.text or "")
for file_ in chat_message.files:
file_.file.mime_type = client_utils.get_mimetype(file_.file.path)
return chat_message
```
Before we wrap up with the backend code, let's modify the `example_value` and `example_payload` method to return a valid dictionary representation of the `ChatbotData`:
```python
def example_value(self) -> Any:
return [[{"text": "Hello!", "files": []}, None]]
def example_payload(self) -> Any:
return [[{"text": "Hello!", "files": []}, None]]
```
Congrats - the backend is complete!
## Part 3a - The Index.svelte file
The frontend for the `Chatbot` component is divided into two parts - the `Index.svelte` file and the `shared/Chatbot.svelte` file.
The `Index.svelte` file applies some processing to the data received from the server and then delegates the rendering of the conversation to the `shared/Chatbot.svelte` file.
First we will modify the `Index.svelte` file to apply processing to the new data type the backend will return.
Let's begin by porting our custom types from our python `data_model` to typescript.
Open `frontend/shared/utils.ts` and add the following type definitions at the top of the file:
```ts
export type FileMessage = {
file: FileData;
alt_text?: string;
};
export type MultimodalMessage = {
text: string;
files?: FileMessage[];
}
```
Now let's import them in `Index.svelte` and modify the type annotations for `value` and `_value`.
```ts
import type { FileMessage, MultimodalMessage } from "./shared/utils";
export let value: [
MultimodalMessage | null,
MultimodalMessage | null
][] = [];
let _value: [
MultimodalMessage | null,
MultimodalMessage | null
][];
```
We need to normalize each message to make sure each file has a proper URL to fetch its contents from.
We also need to format any embedded file links in the `text` key.
Let's add a `process_message` utility function and apply it whenever the `value` changes.
```ts
function process_message(msg: MultimodalMessage | null): MultimodalMessage | null {
if (msg === null) {
return msg;
}
msg.text = redirect_src_url(msg.text);
msg.files = msg.files.map(normalize_messages);
return msg;
}
$: _value = value
? value.map(([user_msg, bot_msg]) => [
process_message(user_msg),
process_message(bot_msg)
])
: [];
```
## Part 3b - the Chatbot.svelte file
Let's begin similarly to the `Index.svelte` file and let's first modify the type annotations.
Import `Mulimodal` message at the top of the `<script>` section and use it to type the `value` and `old_value` variables.
```ts
import type { MultimodalMessage } from "./utils";
export let value:
| [
MultimodalMessage | null,
MultimodalMessage | null
][]
| null;
let old_value:
| [
MultimodalMessage | null,
MultimodalMessage | null
][]
| null = null;
```
We also need to modify the `handle_select` and `handle_like` functions:
```ts
function handle_select(
i: number,
j: number,
message: MultimodalMessage | null
): void {
dispatch("select", {
index: [i, j],
value: message
});
}
function handle_like(
i: number,
j: number,
message: MultimodalMessage | null,
liked: boolean
): void {
dispatch("like", {
index: [i, j],
value: message,
liked: liked
});
}
```
Now for the fun part, actually rendering the text and files in the same message!
You should see some code like the following that determines whether a file or a markdown message should be displayed depending on the type of the message:
```svelte
{#if typeof message === "string"}
<Markdown
{message}
{latex_delimiters}
{sanitize_html}
{render_markdown}
{line_breaks}
on:load={scroll}
/>
{:else if message !== null && message.file?.mime_type?.includes("audio")}
<audio
data-testid="chatbot-audio"
controls
preload="metadata"
...
```
We will modify this code to always display the text message and then loop through the files and display all of them that are present:
```svelte
<Markdown
message={message.text}
{latex_delimiters}
{sanitize_html}
{render_markdown}
{line_breaks}
on:load={scroll}
/>
{#each message.files as file, k}
{#if file !== null && file.file.mime_type?.includes("audio")}
<audio
data-testid="chatbot-audio"
controls
preload="metadata"
src={file.file?.url}
title={file.alt_text}
on:play
on:pause
on:ended
/>
{:else if message !== null && file.file?.mime_type?.includes("video")}
<video
data-testid="chatbot-video"
controls
src={file.file?.url}
title={file.alt_text}
preload="auto"
on:play
on:pause
on:ended
>
<track kind="captions" />
</video>
{:else if message !== null && file.file?.mime_type?.includes("image")}
<img
data-testid="chatbot-image"
src={file.file?.url}
alt={file.alt_text}
/>
{:else if message !== null && file.file?.url !== null}
<a
data-testid="chatbot-file"
href={file.file?.url}
target="_blank"
download={window.__is_colab__
? null
: file.file?.orig_name || file.file?.path}
>
{file.file?.orig_name || file.file?.path}
</a>
{:else if pending_message && j === 1}
<Pending {layout} />
{/if}
{/each}
```
We did it! 🎉
## Part 4 - The demo
For this tutorial, let's keep the demo simple and just display a static conversation between a hypothetical user and a bot.
This demo will show how both the user and the bot can send files.
In part 2 of this tutorial series we will build a fully functional chatbot demo!
The demo code will look like the following:
```python
import gradio as gr
from gradio_multimodalchatbot import MultimodalChatbot
from gradio.data_classes import FileData
user_msg1 = {"text": "Hello, what is in this image?",
"files": [{"file": FileData(path="https://gradio-builds.s3.amazonaws.com/diffusion_image/cute_dog.jpg")}]
}
bot_msg1 = {"text": "It is a very cute dog",
"files": []}
user_msg2 = {"text": "Describe this audio clip please.",
"files": [{"file": FileData(path="cantina.wav")}]}
bot_msg2 = {"text": "It is the cantina song from Star Wars",
"files": []}
user_msg3 = {"text": "Give me a video clip please.",
"files": []}
bot_msg3 = {"text": "Here is a video clip of the world",
"files": [{"file": FileData(path="world.mp4")},
{"file": FileData(path="cantina.wav")}]}
conversation = [[user_msg1, bot_msg1], [user_msg2, bot_msg2], [user_msg3, bot_msg3]]
with gr.Blocks() as demo:
MultimodalChatbot(value=conversation, height=800)
demo.launch()
```
Tip: Change the filepaths so that they correspond to files on your machine. Also, if you are running in development mode, make sure the files are located in the top level of your custom component directory.
## Part 5 - Deploying and Conclusion
Let's build and deploy our demo with `gradio cc build` and `gradio cc deploy`!
You can check out our component deployed to [HuggingFace Spaces](https://huggingface.co/spaces/freddyaboulton/gradio_multimodalchatbot) and all of the source code is available [here](https://huggingface.co/spaces/freddyaboulton/gradio_multimodalchatbot/tree/main/src).
See you in the next installment of this series! |