|
import os |
|
import sys |
|
from dotenv import load_dotenv |
|
import requests |
|
import wave |
|
import zipfile |
|
now_dir = os.getcwd() |
|
sys.path.append(now_dir) |
|
load_dotenv() |
|
from infer.modules.vc.modules import VC |
|
from i18n.i18n import I18nAuto |
|
from configs.config import Config |
|
from sklearn.cluster import MiniBatchKMeans |
|
import torch |
|
import numpy as np |
|
import gradio as gr |
|
import faiss |
|
import fairseq |
|
import librosa |
|
import librosa.display |
|
import pathlib |
|
import json |
|
from pydub import AudioSegment |
|
from time import sleep |
|
from subprocess import Popen |
|
from random import shuffle |
|
import warnings |
|
import traceback |
|
import threading |
|
import shutil |
|
import logging |
|
import matplotlib.pyplot as plt |
|
import soundfile as sf |
|
from dotenv import load_dotenv |
|
|
|
|
|
import edge_tts, asyncio |
|
from infer.modules.vc.ilariatts import tts_order_voice |
|
language_dict = tts_order_voice |
|
ilariavoices = list(language_dict.keys()) |
|
|
|
now_dir = os.getcwd() |
|
sys.path.append(now_dir) |
|
load_dotenv() |
|
|
|
logging.getLogger("numba").setLevel(logging.WARNING) |
|
|
|
logger = logging.getLogger(__name__) |
|
|
|
tmp = os.path.join(now_dir, "TEMP") |
|
shutil.rmtree(tmp, ignore_errors=True) |
|
shutil.rmtree("%s/runtime/Lib/site-packages/infer_pack" % now_dir, ignore_errors=True) |
|
os.makedirs(tmp, exist_ok=True) |
|
os.makedirs(os.path.join(now_dir, "logs"), exist_ok=True) |
|
os.makedirs(os.path.join(now_dir, "models/pth"), exist_ok=True) |
|
os.environ["TEMP"] = tmp |
|
warnings.filterwarnings("ignore") |
|
torch.manual_seed(114514) |
|
|
|
config = Config() |
|
vc = VC(config) |
|
|
|
weight_root = os.getenv("weight_root") |
|
weight_uvr5_root = os.getenv("weight_uvr5_root") |
|
index_root = os.getenv("index_root") |
|
|
|
names = [] |
|
for name in os.listdir(weight_root): |
|
if name.endswith(".pth"): |
|
names.append(name) |
|
index_paths = [] |
|
for root, dirs, files in os.walk(index_root, topdown=False): |
|
for name in files: |
|
if name.endswith(".index") and "trained" not in name: |
|
index_paths.append("%s/%s" % (root, name)) |
|
|
|
|
|
if config.dml: |
|
def forward_dml(ctx, x, scale): |
|
ctx.scale = scale |
|
res = x.clone().detach() |
|
return res |
|
|
|
|
|
fairseq.modules.grad_multiply.GradMultiply.forward = forward_dml |
|
i18n = I18nAuto() |
|
logger.info(i18n) |
|
ngpu = torch.cuda.device_count() |
|
gpu_infos = [] |
|
mem = [] |
|
if_gpu_ok = False |
|
|
|
if torch.cuda.is_available() or ngpu != 0: |
|
for i in range(ngpu): |
|
gpu_name = torch.cuda.get_device_name(i) |
|
if any( |
|
value in gpu_name.upper() |
|
for value in [ |
|
"10", |
|
"16", |
|
"20", |
|
"30", |
|
"40", |
|
"A2", |
|
"A3", |
|
"A4", |
|
"P4", |
|
"A50", |
|
"500", |
|
"A60", |
|
"70", |
|
"80", |
|
"90", |
|
"M4", |
|
"T4", |
|
"TITAN", |
|
] |
|
): |
|
if_gpu_ok = True |
|
gpu_infos.append("%s\t%s" % (i, gpu_name)) |
|
mem.append( |
|
int( |
|
torch.cuda.get_device_properties(i).total_memory |
|
/ 1024 |
|
/ 1024 |
|
/ 1024 |
|
+ 0.4 |
|
) |
|
) |
|
if if_gpu_ok and len(gpu_infos) > 0: |
|
gpu_info = "\n".join(gpu_infos) |
|
default_batch_size = ((min(mem) // 2 + 1) // 2) * 2 |
|
else: |
|
gpu_info = i18n("Your GPU doesn't work for training") |
|
default_batch_size = 1 |
|
gpus = "-".join([i[0] for i in gpu_infos]) |
|
|
|
|
|
class ToolButton(gr.Button, gr.components.FormComponent): |
|
|
|
def __init__(self, **kwargs): |
|
super().__init__(variant="tool", **kwargs) |
|
|
|
def get_block_name(self): |
|
return "button" |
|
|
|
weight_root = os.getenv("weight_root") |
|
index_root = os.getenv("index_root") |
|
audio_root = "audios" |
|
sup_audioext = {'wav', 'mp3', 'flac', 'ogg', 'opus', |
|
'm4a', 'mp4', 'aac', 'alac', 'wma', |
|
'aiff', 'webm', 'ac3'} |
|
|
|
names = [os.path.join(root, file) |
|
for root, _, files in os.walk(weight_root) |
|
for file in files |
|
if file.endswith((".pth", ".onnx"))] |
|
|
|
indexes_list = [os.path.join(root, name) |
|
for root, _, files in os.walk(index_root, topdown=False) |
|
for name in files |
|
if name.endswith(".index") and "trained" not in name] |
|
audio_paths = [os.path.join(root, name) |
|
for root, _, files in os.walk(audio_root, topdown=False) |
|
for name in files |
|
if name.endswith(tuple(sup_audioext))] |
|
|
|
|
|
names = [] |
|
for name in os.listdir(weight_root): |
|
if name.endswith(".pth"): |
|
names.append(name) |
|
index_paths = [] |
|
for root, dirs, files in os.walk(index_root, topdown=False): |
|
for name in files: |
|
if name.endswith(".index") and "trained" not in name: |
|
index_paths.append("%s/%s" % (root, name)) |
|
|
|
def generate_spectrogram_and_get_info(audio_file): |
|
y, sr = librosa.load(audio_file, sr=None) |
|
|
|
S = librosa.feature.melspectrogram(y=y, sr=sr, n_mels=256) |
|
log_S = librosa.amplitude_to_db(S, ref=np.max, top_db=256) |
|
|
|
plt.figure(figsize=(12, 5.5)) |
|
librosa.display.specshow(log_S, sr=sr, x_axis='time') |
|
plt.colorbar(format='%+2.0f dB', pad=0.01) |
|
plt.tight_layout(pad=0.5) |
|
|
|
plt.savefig('spectrogram.png', dpi=500) |
|
|
|
audio_info = sf.info(audio_file) |
|
bit_depth = {'PCM_16': 16, 'FLOAT': 32}.get(audio_info.subtype, 0) |
|
minutes, seconds = divmod(audio_info.duration, 60) |
|
seconds, milliseconds = divmod(seconds, 1) |
|
milliseconds *= 1000 |
|
speed_in_kbps = audio_info.samplerate * bit_depth / 1000 |
|
filename_without_extension, _ = os.path.splitext(os.path.basename(audio_file)) |
|
|
|
info_table = f""" |
|
| Information | Value | |
|
| :---: | :---: | |
|
| File Name | {filename_without_extension} | |
|
| Duration | {int(minutes)} minutes - {int(seconds)} seconds - {int(milliseconds)} milliseconds | |
|
| Bitrate | {speed_in_kbps} kbp/s | |
|
| Audio Channels | {audio_info.channels} | |
|
| Samples per second | {audio_info.samplerate} Hz | |
|
| Bit per second | {audio_info.samplerate * audio_info.channels * bit_depth} bit/s | |
|
""" |
|
|
|
return info_table, "spectrogram.png" |
|
|
|
|
|
def change_choices(): |
|
names = [] |
|
for name in os.listdir(weight_root): |
|
if name.endswith(".pth"): |
|
names.append(name) |
|
index_paths = [] |
|
for root, dirs, files in os.walk(index_root, topdown=False): |
|
for name in files: |
|
if name.endswith(".index") and "trained" not in name: |
|
index_paths.append("%s/%s" % (root, name)) |
|
audios = [os.path.join(audio_root, file) for file in os.listdir(os.path.join(now_dir, "audios"))] |
|
|
|
return {"choices": sorted(names), "__type__": "update"}, {"choices": sorted(index_paths),"__type__": "update"},{ |
|
"choices": sorted(audios), "__type__": "update" |
|
} |
|
|
|
|
|
|
|
|
|
def tts_and_convert(ttsvoice, text, spk_item, vc_transform, f0_file, f0method, file_index1, file_index2, index_rate, filter_radius, resample_sr, rms_mix_rate, protect): |
|
|
|
|
|
vo=language_dict[ttsvoice] |
|
asyncio.run(edge_tts.Communicate(text, vo).save("./TEMP/temp_ilariatts.mp3")) |
|
aud_path = './TEMP/temp_ilariatts.mp3' |
|
|
|
|
|
vc_output1.update("Text converted successfully!") |
|
|
|
|
|
|
|
return vc.vc_single(spk_item , None,aud_path, vc_transform, f0_file, f0method, file_index1, file_index2, index_rate, filter_radius, resample_sr, rms_mix_rate, protect) |
|
|
|
|
|
def import_files(file): |
|
if file is not None: |
|
file_name = file.name |
|
if file_name.endswith('.zip'): |
|
with zipfile.ZipFile(file.name, 'r') as zip_ref: |
|
|
|
temp_dir = './TEMP' |
|
zip_ref.extractall(temp_dir) |
|
|
|
for root, dirs, files in os.walk(temp_dir): |
|
for file in files: |
|
if file.endswith('.pth'): |
|
destination = './models/pth/' + file |
|
if not os.path.exists(destination): |
|
shutil.move(os.path.join(root, file), destination) |
|
else: |
|
print(f"File {destination} already exists. Skipping.") |
|
elif file.endswith('.index'): |
|
destination = './models/index/' + file |
|
if not os.path.exists(destination): |
|
shutil.move(os.path.join(root, file), destination) |
|
else: |
|
print(f"File {destination} already exists. Skipping.") |
|
|
|
shutil.rmtree(temp_dir) |
|
return "Zip file has been successfully extracted." |
|
elif file_name.endswith('.pth'): |
|
destination = './models/pth/' + os.path.basename(file.name) |
|
if not os.path.exists(destination): |
|
os.rename(file.name, destination) |
|
else: |
|
print(f"File {destination} already exists. Skipping.") |
|
return "PTH file has been successfully imported." |
|
elif file_name.endswith('.index'): |
|
destination = './models/index/' + os.path.basename(file.name) |
|
if not os.path.exists(destination): |
|
os.rename(file.name, destination) |
|
else: |
|
print(f"File {destination} already exists. Skipping.") |
|
return "Index file has been successfully imported." |
|
else: |
|
return "Unsupported file type." |
|
else: |
|
return "No file has been uploaded." |
|
|
|
def import_button_click(file): |
|
return import_files(file) |
|
|
|
|
|
def get_audio_duration(audio_file_path): |
|
audio_info = sf.info(audio_file_path) |
|
duration_minutes = audio_info.duration / 60 |
|
return duration_minutes |
|
|
|
def clean(): |
|
return {"value": "", "__type__": "update"} |
|
|
|
|
|
sr_dict = { |
|
"32k": 32000, "40k": 40000, "48k": 48000, "OV2-32k": 32000, "OV2-40k": 40000, "RIN-40k": 40000, "Snowie-40k": 40000, "Snowie-48k": 48000, "SnowieV3.1-40k": 40000, "SnowieV3.1-32k": 32000, "SnowieV3.1-48k": 48000, "SnowieV3.1-RinE3-40K": 40000, |
|
} |
|
|
|
def durations(sample_rate, model_options, qualities, duration): |
|
if duration <= 350: |
|
return qualities['short'] |
|
else: |
|
if sample_rate == 32000: |
|
return model_options['32k'] |
|
elif sample_rate == 40000: |
|
return model_options['40k'] |
|
elif sample_rate == 48000: |
|
return model_options['48k'] |
|
else: |
|
return qualities['other'] |
|
|
|
def get_training_info(audio_file): |
|
if audio_file is None: |
|
return 'Please provide an audio file!' |
|
duration = get_audio_duration(audio_file) |
|
sample_rate = wave.open(audio_file, 'rb').getframerate() |
|
|
|
training_info = { |
|
(0, 2): (150, 'OV2'), |
|
(2, 3): (200, 'OV2'), |
|
(3, 5): (250, 'OV2'), |
|
(5, 10): (300, 'Normal'), |
|
(10, 25): (500, 'Normal'), |
|
(25, 45): (700, 'Normal'), |
|
(45, 60): (1000, 'Normal') |
|
} |
|
|
|
for (min_duration, max_duration), (epochs, pretrain) in training_info.items(): |
|
if min_duration <= duration < max_duration: |
|
break |
|
else: |
|
return 'Duration is not within the specified range!' |
|
|
|
return f'You should use the **{pretrain}** pretrain with **{epochs}** epochs at **{sample_rate/1000}khz** sample rate.' |
|
|
|
|
|
def if_done(done, p): |
|
while 1: |
|
if p.poll() is None: |
|
sleep(0.5) |
|
else: |
|
break |
|
done[0] = True |
|
|
|
def on_button_click(audio_file_path): |
|
return get_training_info(audio_file_path) |
|
|
|
def download_from_url(url, model): |
|
if url == '': |
|
return "URL cannot be left empty." |
|
if model == '': |
|
return "You need to name your model. For example: Ilaria" |
|
|
|
url = url.strip() |
|
zip_dirs = ["zips", "unzips"] |
|
for directory in zip_dirs: |
|
if os.path.exists(directory): |
|
shutil.rmtree(directory) |
|
|
|
os.makedirs("zips", exist_ok=True) |
|
os.makedirs("unzips", exist_ok=True) |
|
|
|
zipfile = model + '.zip' |
|
zipfile_path = './zips/' + zipfile |
|
|
|
try: |
|
if "drive.google.com" in url: |
|
subprocess.run(["gdown", url, "--fuzzy", "-O", zipfile_path]) |
|
elif "mega.nz" in url: |
|
m = Mega() |
|
m.download_url(url, './zips') |
|
else: |
|
response = requests.get(url) |
|
response.raise_for_status() |
|
with open(zipfile_path, 'wb') as file: |
|
file.write(response.content) |
|
|
|
shutil.unpack_archive(zipfile_path, "./unzips", 'zip') |
|
|
|
for root, dirs, files in os.walk('./unzips'): |
|
for file in files: |
|
file_path = os.path.join(root, file) |
|
if file.endswith(".index"): |
|
os.makedirs(f'./models/index', exist_ok=True) |
|
shutil.copy2(file_path, f'./models/index/{model}.index') |
|
elif "G_" not in file and "D_" not in file and file.endswith(".pth"): |
|
os.makedirs(f'./models/pth', exist_ok=True) |
|
shutil.copy(file_path, f'./models/pth/{model}.pth') |
|
|
|
shutil.rmtree("zips") |
|
shutil.rmtree("unzips") |
|
return "Model downloaded, you can go back to the inference page!" |
|
|
|
except subprocess.CalledProcessError as e: |
|
return f"ERROR - Download failed (gdown): {str(e)}" |
|
except requests.exceptions.RequestException as e: |
|
return f"ERROR - Download failed (requests): {str(e)}" |
|
except Exception as e: |
|
return f"ERROR - The test failed: {str(e)}" |
|
|
|
def transfer_files(filething, dataset_dir='dataset/'): |
|
file_names = [f.name for f in filething] |
|
for f in file_names: |
|
filename = os.path.basename(f) |
|
destination = os.path.join(dataset_dir, filename) |
|
shutil.copyfile(f, destination) |
|
return "Transferred files to dataset directory!" |
|
|
|
def if_done_multi(done, ps): |
|
while 1: |
|
flag = 1 |
|
for p in ps: |
|
if p.poll() is None: |
|
flag = 0 |
|
sleep(0.5) |
|
break |
|
if flag == 1: |
|
break |
|
done[0] = True |
|
|
|
|
|
def preprocess_dataset(trainset_dir, exp_dir, sr, n_p): |
|
sr = sr_dict[sr] |
|
os.makedirs("%s/logs/%s" % (now_dir, exp_dir), exist_ok=True) |
|
f = open("%s/logs/%s/preprocess.log" % (now_dir, exp_dir), "w") |
|
f.close() |
|
per = 3.0 if config.is_half else 3.7 |
|
cmd = '"%s" infer/modules/train/preprocess.py "%s" %s %s "%s/logs/%s" %s %.1f' % ( |
|
config.python_cmd, |
|
trainset_dir, |
|
sr, |
|
n_p, |
|
now_dir, |
|
exp_dir, |
|
config.noparallel, |
|
per, |
|
) |
|
logger.info(cmd) |
|
p = Popen(cmd, shell=True) |
|
done = [False] |
|
threading.Thread( |
|
target=if_done, |
|
args=( |
|
done, |
|
p, |
|
), |
|
).start() |
|
while 1: |
|
with open("%s/logs/%s/preprocess.log" % (now_dir, exp_dir), "r") as f: |
|
yield f.read() |
|
sleep(1) |
|
if done[0]: |
|
break |
|
with open("%s/logs/%s/preprocess.log" % (now_dir, exp_dir), "r") as f: |
|
log = f.read() |
|
logger.info(log) |
|
yield log |
|
|
|
|
|
def extract_f0_feature(gpus, n_p, f0method, if_f0, exp_dir, version19, gpus_rmvpe): |
|
gpus = gpus.split("-") |
|
os.makedirs("%s/logs/%s" % (now_dir, exp_dir), exist_ok=True) |
|
f = open("%s/logs/%s/extract_f0_feature.log" % (now_dir, exp_dir), "w") |
|
f.close() |
|
if if_f0: |
|
if f0method != "rmvpe_gpu": |
|
cmd = ( |
|
'"%s" infer/modules/train/extract/extract_f0_print.py "%s/logs/%s" %s %s' |
|
% ( |
|
config.python_cmd, |
|
now_dir, |
|
exp_dir, |
|
n_p, |
|
f0method, |
|
) |
|
) |
|
logger.info(cmd) |
|
p = Popen( |
|
cmd, shell=True, cwd=now_dir |
|
) |
|
done = [False] |
|
threading.Thread( |
|
target=if_done, |
|
args=( |
|
done, |
|
p, |
|
), |
|
).start() |
|
else: |
|
if gpus_rmvpe != "-": |
|
gpus_rmvpe = gpus_rmvpe.split("-") |
|
leng = len(gpus_rmvpe) |
|
ps = [] |
|
for idx, n_g in enumerate(gpus_rmvpe): |
|
cmd = ( |
|
'"%s" infer/modules/train/extract/extract_f0_rmvpe.py %s %s %s "%s/logs/%s" %s ' |
|
% ( |
|
config.python_cmd, |
|
leng, |
|
idx, |
|
n_g, |
|
now_dir, |
|
exp_dir, |
|
config.is_half, |
|
) |
|
) |
|
logger.info(cmd) |
|
p = Popen( |
|
cmd, shell=True, cwd=now_dir |
|
) |
|
ps.append(p) |
|
done = [False] |
|
threading.Thread( |
|
target=if_done_multi, |
|
args=( |
|
done, |
|
ps, |
|
), |
|
).start() |
|
else: |
|
cmd = ( |
|
config.python_cmd |
|
+ ' infer/modules/train/extract/extract_f0_rmvpe_dml.py "%s/logs/%s" ' |
|
% ( |
|
now_dir, |
|
exp_dir, |
|
) |
|
) |
|
logger.info(cmd) |
|
p = Popen( |
|
cmd, shell=True, cwd=now_dir |
|
) |
|
p.wait() |
|
done = [True] |
|
while 1: |
|
with open( |
|
"%s/logs/%s/extract_f0_feature.log" % (now_dir, exp_dir), "r" |
|
) as f: |
|
yield f.read() |
|
sleep(1) |
|
if done[0]: |
|
break |
|
with open("%s/logs/%s/extract_f0_feature.log" % (now_dir, exp_dir), "r") as f: |
|
log = f.read() |
|
logger.info(log) |
|
yield log |
|
|
|
leng = len(gpus) |
|
ps = [] |
|
for idx, n_g in enumerate(gpus): |
|
cmd = ( |
|
'"%s" infer/modules/train/extract_feature_print.py %s %s %s %s "%s/logs/%s" %s' |
|
% ( |
|
config.python_cmd, |
|
config.device, |
|
leng, |
|
idx, |
|
n_g, |
|
now_dir, |
|
exp_dir, |
|
version19, |
|
) |
|
) |
|
logger.info(cmd) |
|
p = Popen( |
|
cmd, shell=True, cwd=now_dir |
|
) |
|
ps.append(p) |
|
done = [False] |
|
threading.Thread( |
|
target=if_done_multi, |
|
args=( |
|
done, |
|
ps, |
|
), |
|
).start() |
|
while 1: |
|
with open("%s/logs/%s/extract_f0_feature.log" % (now_dir, exp_dir), "r") as f: |
|
yield f.read() |
|
sleep(1) |
|
if done[0]: |
|
break |
|
with open("%s/logs/%s/extract_f0_feature.log" % (now_dir, exp_dir), "r") as f: |
|
log = f.read() |
|
logger.info(log) |
|
yield log |
|
|
|
|
|
|
|
def change_sr2(sr2, if_f0_3, version19): |
|
path_str = "" if version19 == "v1" else "_v2" |
|
f0_str = "f0" if if_f0_3 else "" |
|
return get_pretrained_models(path_str, f0_str, sr2) |
|
|
|
|
|
def change_version19(sr2, if_f0_3, version19): |
|
path_str = "" if version19 == "v1" else "_v2" |
|
if sr2 == "32k" and version19 == "v1": |
|
sr2 = "40k" |
|
to_return_sr2 = ( |
|
{"choices": ["32k","40k", "48k"], "__type__": "update", "value": sr2} |
|
if version19 == "v1" |
|
else {"choices": ["32k", "40k", "48k", "OV2-32k", "OV2-40k", "RIN-40k","Snowie-40k","Snowie-48k"], "__type__": "update", "value": sr2} |
|
) |
|
f0_str = "f0" if if_f0_3 else "" |
|
return ( |
|
*get_pretrained_models(path_str, f0_str, sr2), |
|
to_return_sr2, |
|
) |
|
|
|
def change_f0(if_f0_3, sr2, version19): |
|
path_str = "" if version19 == "v1" else "_v2" |
|
return ( |
|
{"visible": if_f0_3, "__type__": "update"}, |
|
{"visible": if_f0_3, "__type__": "update"}, |
|
*get_pretrained_models(path_str, "f0" if if_f0_3 is True else "", sr2), |
|
) |
|
|
|
def click_train( |
|
exp_dir1, |
|
sr2, |
|
if_f0_3, |
|
spk_id5, |
|
save_epoch10, |
|
total_epoch11, |
|
batch_size12, |
|
if_save_latest13, |
|
pretrained_G14, |
|
pretrained_D15, |
|
gpus16, |
|
if_cache_gpu17, |
|
if_save_every_weights18, |
|
version19, |
|
): |
|
global f0_dir, f0nsf_dir |
|
exp_dir = "%s/logs/%s" % (now_dir, exp_dir1) |
|
os.makedirs(exp_dir, exist_ok=True) |
|
gt_wavs_dir = "%s/0_gt_wavs" % exp_dir |
|
feature_dir = ( |
|
"%s/3_feature256" % exp_dir |
|
if version19 == "v1" |
|
else "%s/3_feature768" % exp_dir |
|
) |
|
if if_f0_3: |
|
f0_dir = "%s/2a_f0" % exp_dir |
|
f0nsf_dir = "%s/2b-f0nsf" % exp_dir |
|
names = ( |
|
set([name.split(".")[0] for name in os.listdir(gt_wavs_dir)]) |
|
& set([name.split(".")[0] for name in os.listdir(feature_dir)]) |
|
& set([name.split(".")[0] for name in os.listdir(f0_dir)]) |
|
& set([name.split(".")[0] for name in os.listdir(f0nsf_dir)]) |
|
) |
|
else: |
|
names = set([name.split(".")[0] for name in os.listdir(gt_wavs_dir)]) & set( |
|
[name.split(".")[0] for name in os.listdir(feature_dir)] |
|
) |
|
opt = [] |
|
for name in names: |
|
if if_f0_3: |
|
opt.append( |
|
"%s/%s.wav|%s/%s.npy|%s/%s.wav.npy|%s/%s.wav.npy|%s" |
|
% ( |
|
gt_wavs_dir.replace("\\", "\\\\"), |
|
name, |
|
feature_dir.replace("\\", "\\\\"), |
|
name, |
|
f0_dir.replace("\\", "\\\\"), |
|
name, |
|
f0nsf_dir.replace("\\", "\\\\"), |
|
name, |
|
spk_id5, |
|
) |
|
) |
|
else: |
|
opt.append( |
|
"%s/%s.wav|%s/%s.npy|%s" |
|
% ( |
|
gt_wavs_dir.replace("\\", "\\\\"), |
|
name, |
|
feature_dir.replace("\\", "\\\\"), |
|
name, |
|
spk_id5, |
|
) |
|
) |
|
fea_dim = 256 if version19 == "v1" else 768 |
|
if if_f0_3: |
|
for _ in range(2): |
|
opt.append( |
|
"%s/logs/mute/0_gt_wavs/mute%s.wav|%s/logs/mute/3_feature%s/mute.npy|%s/logs/mute/2a_f0/mute.wav.npy" |
|
"|%s/logs/mute/2b-f0nsf/mute.wav.npy|%s" |
|
% (now_dir, sr2, now_dir, fea_dim, now_dir, now_dir, spk_id5) |
|
) |
|
else: |
|
for _ in range(2): |
|
opt.append( |
|
"%s/logs/mute/0_gt_wavs/mute%s.wav|%s/logs/mute/3_feature%s/mute.npy|%s" |
|
% (now_dir, sr2, now_dir, fea_dim, spk_id5) |
|
) |
|
shuffle(opt) |
|
with open("%s/filelist.txt" % exp_dir, "w") as f: |
|
f.write("\n".join(opt)) |
|
logger.debug("Write filelist done") |
|
logger.info("Use gpus: %s", str(gpus16)) |
|
if pretrained_G14 == "": |
|
logger.info("No pretrained Generator") |
|
if pretrained_D15 == "": |
|
logger.info("No pretrained Discriminator") |
|
if version19 == "v1" or sr2 == "40k": |
|
config_path = "v1/%s.json" % sr2 |
|
else: |
|
config_path = "v2/%s.json" % sr2 |
|
config_save_path = os.path.join(exp_dir, "config.json") |
|
if not pathlib.Path(config_save_path).exists(): |
|
with open(config_save_path, "w", encoding="utf-8") as f: |
|
json.dump( |
|
config.json_config[config_path], |
|
f, |
|
ensure_ascii=False, |
|
indent=4, |
|
sort_keys=True, |
|
) |
|
f.write("\n") |
|
if gpus16: |
|
cmd = ( |
|
'"%s" infer/modules/train/train.py -e "%s" -sr %s -f0 %s -bs %s -g %s -te %s -se %s %s %s -l %s -c %s ' |
|
"-sw %s -v %s" |
|
% ( |
|
config.python_cmd, |
|
exp_dir1, |
|
sr2, |
|
1 if if_f0_3 else 0, |
|
batch_size12, |
|
gpus16, |
|
total_epoch11, |
|
save_epoch10, |
|
"-pg %s" % pretrained_G14 if pretrained_G14 != "" else "", |
|
"-pd %s" % pretrained_D15 if pretrained_D15 != "" else "", |
|
1 if if_save_latest13 == i18n("是") else 0, |
|
1 if if_cache_gpu17 == i18n("是") else 0, |
|
1 if if_save_every_weights18 == i18n("是") else 0, |
|
version19, |
|
) |
|
) |
|
else: |
|
cmd = ( |
|
'"%s" infer/modules/train/train.py -e "%s" -sr %s -f0 %s -bs %s -te %s -se %s %s %s -l %s -c %s -sw ' |
|
"%s -v %s" |
|
% ( |
|
config.python_cmd, |
|
exp_dir1, |
|
sr2, |
|
1 if if_f0_3 else 0, |
|
batch_size12, |
|
total_epoch11, |
|
save_epoch10, |
|
"-pg %s" % pretrained_G14 if pretrained_G14 != "" else "", |
|
"-pd %s" % pretrained_D15 if pretrained_D15 != "" else "", |
|
1 if if_save_latest13 == i18n("是") else 0, |
|
1 if if_cache_gpu17 == i18n("是") else 0, |
|
1 if if_save_every_weights18 == i18n("是") else 0, |
|
version19, |
|
) |
|
) |
|
logger.info(cmd) |
|
p = Popen(cmd, shell=True, cwd=now_dir) |
|
p.wait() |
|
return "You can view console or train.log" |
|
|
|
def train_index(exp_dir1, version19): |
|
exp_dir = "logs/%s" % exp_dir1 |
|
os.makedirs(exp_dir, exist_ok=True) |
|
feature_dir = ( |
|
"%s/3_feature256" % exp_dir |
|
if version19 == "v1" |
|
else "%s/3_feature768" % exp_dir |
|
) |
|
if not os.path.exists(feature_dir): |
|
return "Please perform Feature Extraction First!" |
|
listdir_res = list(os.listdir(feature_dir)) |
|
if len(listdir_res) == 0: |
|
return "Please perform Feature Extraction First!" |
|
infos = [] |
|
npys = [] |
|
for name in sorted(listdir_res): |
|
phone = np.load("%s/%s" % (feature_dir, name)) |
|
npys.append(phone) |
|
big_npy = np.concatenate(npys, 0) |
|
big_npy_idx = np.arange(big_npy.shape[0]) |
|
np.random.shuffle(big_npy_idx) |
|
big_npy = big_npy[big_npy_idx] |
|
if big_npy.shape[0] > 2e5: |
|
infos.append("Trying doing kmeans %s shape to 10k centers." % big_npy.shape[0]) |
|
yield "\n".join(infos) |
|
try: |
|
big_npy = ( |
|
MiniBatchKMeans( |
|
n_clusters=10000, |
|
verbose=True, |
|
batch_size=256 * config.n_cpu, |
|
compute_labels=False, |
|
init="random", |
|
) |
|
.fit(big_npy) |
|
.cluster_centers_ |
|
) |
|
except: |
|
info = traceback.format_exc() |
|
logger.info(info) |
|
infos.append(info) |
|
yield "\n".join(infos) |
|
|
|
np.save("%s/total_fea.npy" % exp_dir, big_npy) |
|
n_ivf = min(int(16 * np.sqrt(big_npy.shape[0])), big_npy.shape[0] // 39) |
|
infos.append("%s,%s" % (big_npy.shape, n_ivf)) |
|
yield "\n".join(infos) |
|
index = faiss.index_factory(256 if version19 == "v1" else 768, "IVF%s,Flat" % n_ivf) |
|
infos.append("training") |
|
yield "\n".join(infos) |
|
index_ivf = faiss.extract_index_ivf(index) |
|
index_ivf.nprobe = 1 |
|
index.train(big_npy) |
|
faiss.write_index( |
|
index, |
|
"%s/trained_IVF%s_Flat_nprobe_%s_%s_%s.index" |
|
% (exp_dir, n_ivf, index_ivf.nprobe, exp_dir1, version19), |
|
) |
|
|
|
infos.append("adding") |
|
yield "\n".join(infos) |
|
batch_size_add = 8192 |
|
for i in range(0, big_npy.shape[0], batch_size_add): |
|
index.add(big_npy[i: i + batch_size_add]) |
|
faiss.write_index( |
|
index, |
|
"%s/added_IVF%s_Flat_nprobe_%s_%s_%s.index" |
|
% (exp_dir, n_ivf, index_ivf.nprobe, exp_dir1, version19), |
|
) |
|
infos.append( |
|
"Success,added_IVF%s_Flat_nprobe_%s_%s_%s.index" |
|
% (n_ivf, index_ivf.nprobe, exp_dir1, version19) |
|
) |
|
yield "\n".join(infos) |
|
|
|
F0GPUVisible = config.dml is False |
|
|
|
def change_f0_method(f0method8): |
|
if f0method8 == "rmvpe_gpu": |
|
visible = F0GPUVisible |
|
else: |
|
visible = False |
|
return {"visible": visible, "__type__": "update"} |
|
|
|
vc_output1 = gr.Textbox(label=i18n("Console")) |
|
vc_output2 = gr.Audio(label=i18n("Audio output")) |
|
|
|
with gr.Blocks(title="Ilaria RVC 💖") as app: |
|
gr.Markdown("<h1> Ilaria RVC 💖 </h1>") |
|
gr.Markdown(value=i18n("Made with 💖 by Ilaria | Support her on [Ko-Fi](https://ko-fi.com/ilariaowo)")) |
|
gr.Markdown(i18n("For voice models and support join [AI Hub](https://discord.gg/AIHUB)")) |
|
with gr.Tabs(): |
|
with gr.TabItem(i18n("Inference")): |
|
with gr.Row(): |
|
sid0= gr.Dropdown(label=i18n("Voice"), choices=sorted(names)) |
|
sid1= sid0 |
|
|
|
with gr.Column(): |
|
refresh_button = gr.Button(i18n("Refresh"), variant="primary") |
|
clean_button = gr.Button(i18n("Unload Voice from VRAM"), variant="primary") |
|
vc_transform0 = gr.inputs.Slider( |
|
label=i18n( |
|
"Pitch: -24 is lower (2 octaves) and 24 is higher (2 octaves)"), |
|
minimum=-24, |
|
maximum=24, |
|
default=0, |
|
step=1, |
|
) |
|
clean_button.click( |
|
fn=clean, inputs=[], outputs=[sid0], api_name="infer_clean" |
|
) |
|
with gr.TabItem(i18n("Inference")): |
|
with gr.Group(): |
|
with gr.Row(): |
|
with gr.Column(): |
|
input_audio1 = gr.Audio( |
|
label=i18n("Upload Audio file"), |
|
type="filepath", |
|
) |
|
record_button = gr.Audio(source="microphone", label="Use your microphone", |
|
type="filepath") |
|
|
|
input_audio0 = gr.Dropdown( |
|
label=i18n("Select a file from the audio folder"), |
|
choices=sorted(audio_paths), |
|
value='', |
|
interactive=True, |
|
) |
|
record_button.change( |
|
fn=lambda x: x, |
|
inputs=[record_button], |
|
outputs=[input_audio0], |
|
) |
|
file_index1 = gr.Textbox( |
|
label=i18n("Path of index"), |
|
placeholder=".\models\index", |
|
interactive=True, |
|
visible=False, |
|
) |
|
file_index2 = gr.Textbox( |
|
label=i18n("Auto-detect index path"), |
|
choices=sorted(index_paths), |
|
interactive=True, |
|
visible=False, |
|
) |
|
with gr.Column(): |
|
with gr.Accordion('Advanced Settings', open=False, visible=False): |
|
with gr.Column(): |
|
f0method0 = gr.Radio( |
|
label=i18n("Pitch Extraction, rmvpe is best"), |
|
choices=["harvest", "crepe", "rmvpe"] |
|
if config.dml is False |
|
else ["harvest", "rmvpe"], |
|
value="rmvpe", |
|
interactive=True, |
|
) |
|
resample_sr0 = gr.Slider( |
|
minimum=0, |
|
maximum=48000, |
|
label=i18n("Resampling, 0=none"), |
|
value=0, |
|
step=1, |
|
interactive=True, |
|
) |
|
rms_mix_rate0 = gr.Slider( |
|
minimum=0, |
|
maximum=1, |
|
label=i18n("0=Input source volume, 1=Normalized Output"), |
|
value=0.25, |
|
interactive=True, |
|
) |
|
protect0 = gr.Slider( |
|
minimum=0, |
|
maximum=0.5, |
|
label=i18n( |
|
"Protect clear consonants and breathing sounds, preventing electro-acoustic tearing and other artifacts, 0.5 does not open"), |
|
value=0.33, |
|
step=0.01, |
|
interactive=True, |
|
) |
|
filter_radius0 = gr.Slider( |
|
minimum=0, |
|
maximum=7, |
|
label=i18n(">=3 apply median filter to the harvested pitch results"), |
|
value=3, |
|
step=1, |
|
interactive=True, |
|
) |
|
index_rate1 = gr.Slider( |
|
minimum=0, |
|
maximum=1, |
|
label=i18n("Index Ratio"), |
|
value=0.40, |
|
interactive=True, |
|
) |
|
f0_file = gr.File( |
|
label=i18n("F0 curve file [optional]"), |
|
visible=False, |
|
) |
|
|
|
refresh_button.click( |
|
fn=change_choices, |
|
inputs=[], |
|
outputs=[sid0, file_index2, input_audio1], |
|
api_name="infer_refresh", |
|
) |
|
file_index1 = gr.Textbox( |
|
label=i18n("Path of index"), |
|
placeholder="%userprofile%\\Desktop\\models\\model_example.index", |
|
interactive=True, |
|
) |
|
file_index2 = gr.Dropdown( |
|
label=i18n("Auto-detect index path"), |
|
choices=sorted(index_paths), |
|
interactive=True, |
|
) |
|
spk_item = gr.Slider( |
|
minimum=0, |
|
maximum=2333, |
|
step=1, |
|
label=i18n("Speaker ID (Auto-Detected)"), |
|
value=0, |
|
visible=True, |
|
interactive=False, |
|
) |
|
|
|
with gr.Accordion('IlariaTTS', open=True): |
|
with gr.Column(): |
|
ilariaid=gr.Dropdown(label="Voice:", choices=ilariavoices, interactive=True, value="English-Jenny (Female)") |
|
ilariatext = gr.Textbox(label="Input your Text", interactive=True, value="This is a test.") |
|
ilariatts_button = gr.Button(value="Speak and Convert") |
|
ilariatts_button.click(tts_and_convert, |
|
[ilariaid, |
|
ilariatext, |
|
spk_item, |
|
vc_transform0, |
|
f0_file, |
|
f0method0, |
|
file_index1, |
|
file_index2, |
|
index_rate1, |
|
filter_radius0, |
|
resample_sr0, |
|
rms_mix_rate0, |
|
protect0] |
|
, [vc_output1, vc_output2]) |
|
|
|
|
|
with gr.Accordion('Advanced Settings', open=False, visible=True): |
|
with gr.Column(): |
|
f0method0 = gr.Radio( |
|
label=i18n("Pitch Extraction, rmvpe is best"), |
|
choices=["harvest", "crepe", "rmvpe"] |
|
if config.dml is False |
|
else ["harvest", "rmvpe"], |
|
value="rmvpe", |
|
interactive=True, |
|
) |
|
resample_sr0 = gr.Slider( |
|
minimum=0, |
|
maximum=48000, |
|
label=i18n("Resampling, 0=none"), |
|
value=0, |
|
step=1, |
|
interactive=True, |
|
) |
|
rms_mix_rate0 = gr.Slider( |
|
minimum=0, |
|
maximum=1, |
|
label=i18n("0=Input source volume, 1=Normalized Output"), |
|
value=0.25, |
|
interactive=True, |
|
) |
|
protect0 = gr.Slider( |
|
minimum=0, |
|
maximum=0.5, |
|
label=i18n( |
|
"Protect clear consonants and breathing sounds, preventing electro-acoustic tearing and other artifacts, 0.5 does not open"), |
|
value=0.33, |
|
step=0.01, |
|
interactive=True, |
|
) |
|
filter_radius0 = gr.Slider( |
|
minimum=0, |
|
maximum=7, |
|
label=i18n(">=3 apply median filter to the harvested pitch results"), |
|
value=3, |
|
step=1, |
|
interactive=True, |
|
) |
|
index_rate1 = gr.Slider( |
|
minimum=0, |
|
maximum=1, |
|
label=i18n("Index Ratio"), |
|
value=0.40, |
|
interactive=True, |
|
) |
|
f0_file = gr.File( |
|
label=i18n("F0 curve file [optional]"), |
|
visible=False, |
|
) |
|
|
|
refresh_button.click( |
|
fn=change_choices, |
|
inputs=[], |
|
outputs=[sid0, file_index2], |
|
api_name="infer_refresh", |
|
) |
|
file_index1 = gr.Textbox( |
|
label=i18n("Path of index"), |
|
placeholder="%userprofile%\\Desktop\\models\\model_example.index", |
|
interactive=True, |
|
) |
|
file_index2 = gr.Dropdown( |
|
label=i18n("Auto-detect index path"), |
|
choices=sorted(index_paths), |
|
interactive=True, |
|
) |
|
|
|
with gr.Group(): |
|
with gr.Column(): |
|
but0 = gr.Button(i18n("Convert"), variant="primary") |
|
with gr.Row(): |
|
vc_output1.render() |
|
vc_output2.render() |
|
|
|
but0.click( |
|
vc.vc_single, |
|
[ |
|
spk_item, |
|
input_audio0, |
|
input_audio1, |
|
vc_transform0, |
|
f0_file, |
|
f0method0, |
|
file_index1, |
|
file_index2, |
|
|
|
index_rate1, |
|
filter_radius0, |
|
resample_sr0, |
|
rms_mix_rate0, |
|
protect0, |
|
], |
|
[vc_output1, vc_output2], |
|
api_name="infer_convert", |
|
) |
|
with gr.TabItem("Download Voice Models"): |
|
gr.Markdown(i18n("For models found in [AI Hub](https://discord.gg/AIHUB)")) |
|
with gr.Row(): |
|
url = gr.Textbox(label="Huggingface Link:") |
|
with gr.Row(): |
|
model = gr.Textbox(label="Name of the model (without spaces):") |
|
download_button = gr.Button("Download") |
|
with gr.Row(): |
|
status_bar = gr.Textbox(label="Download Status") |
|
download_button.click(fn=download_from_url, inputs=[url, model], outputs=[status_bar]) |
|
|
|
with gr.TabItem("Import Models"): |
|
gr.Markdown(i18n("For models found on [Weights](https://weights.gg)")) |
|
file_upload = gr.File(label="Upload a .zip file containing a .pth and .index file") |
|
import_button = gr.Button("Import") |
|
import_status = gr.Textbox(label="Import Status") |
|
import_button.click(fn=import_button_click, inputs=file_upload, outputs=import_status) |
|
|
|
with gr.TabItem(i18n("Batch Inference - WIP")): |
|
gr.Markdown( |
|
value=i18n("Batch Conversion") |
|
) |
|
|
|
with gr.Row(): |
|
with gr.Column(): |
|
vc_transform1 = gr.Number( |
|
label=i18n("Pitch: 0 from man to man (or woman to woman); 12 from man to woman and -12 from woman to man."), |
|
value=0 |
|
) |
|
opt_input = gr.Textbox(label=i18n("Output"), value="InferOutput") |
|
file_index3 = gr.Textbox( |
|
label=i18n("Path to index"), |
|
value="", |
|
interactive=False, |
|
) |
|
file_index4 = gr.Dropdown( |
|
label=i18n("Auto-detect index path"), |
|
choices=sorted(index_paths), |
|
interactive=False, |
|
) |
|
f0method1 = gr.Radio( |
|
label=i18n("Pitch Extraction, rmvpe is best"), |
|
choices=["harvest", "crepe", "rmvpe"] |
|
if config.dml is False |
|
else ["harvest", "rmvpe"], |
|
value="rmvpe", |
|
interactive=False, |
|
) |
|
format1 = gr.Radio( |
|
label=i18n("Export Format"), |
|
choices=["flac", "wav", "mp3", "m4a"], |
|
value="flac", |
|
interactive=False, |
|
) |
|
|
|
refresh_button.click( |
|
fn=lambda: change_choices()[1], |
|
inputs=[], |
|
outputs=file_index4, |
|
api_name="infer_refresh_batch", |
|
) |
|
|
|
with gr.Column(): |
|
resample_sr1 = gr.Slider( |
|
minimum=0, |
|
maximum=48000, |
|
label=i18n("Resampling, 0=none"), |
|
value=0, |
|
step=1, |
|
interactive=False, |
|
) |
|
rms_mix_rate1 = gr.Slider( |
|
minimum=0, |
|
maximum=1, |
|
label=i18n("0=Input source volume, 1=Normalized Output"), |
|
value=0.25, |
|
interactive=False, |
|
) |
|
protect1 = gr.Slider( |
|
minimum=0, |
|
maximum=0.5, |
|
label=i18n( |
|
"Protect clear consonants and breathing sounds, preventing electro-acoustic tearing and other artifacts, 0.5 does not open"), |
|
value=0.33, |
|
step=0.01, |
|
interactive=False, |
|
) |
|
filter_radius1 = gr.Slider( |
|
minimum=0, |
|
maximum=7, |
|
label=i18n(">=3 apply median filter to the harvested pitch results"), |
|
value=3, |
|
step=1, |
|
interactive=False, |
|
) |
|
index_rate2 = gr.Slider( |
|
minimum=0, |
|
maximum=1, |
|
label=i18n("Index Ratio"), |
|
value=0.40, |
|
interactive=False, |
|
) |
|
with gr.Row(): |
|
dir_input = gr.Textbox( |
|
label=i18n("Enter the path to the audio folder to be processed"), |
|
placeholder="%userprofile%\\Desktop\\covers", |
|
) |
|
inputs = gr.File( |
|
file_count="multiple", label=i18n("Audio files can also be imported in batch") |
|
) |
|
|
|
with gr.Row(): |
|
but1 = gr.Button(i18n("Convert"), variant="primary") |
|
vc_output3 = gr.Textbox(label=i18n("Console")) |
|
|
|
but1.click( |
|
vc.vc_multi, |
|
[ |
|
spk_item, |
|
dir_input, |
|
opt_input, |
|
inputs, |
|
vc_transform1, |
|
f0method1, |
|
file_index3, |
|
file_index4, |
|
|
|
index_rate2, |
|
filter_radius1, |
|
resample_sr1, |
|
rms_mix_rate1, |
|
protect1, |
|
format1, |
|
], |
|
[vc_output3], |
|
api_name="infer_convert_batch", |
|
) |
|
|
|
with gr.TabItem(i18n("Extra")): |
|
with gr.Accordion('Model Info', open=False): |
|
with gr.Column(): |
|
sid1 = gr.Dropdown(label=i18n("Voice Model"), choices=sorted(names)) |
|
refresh_button = gr.Button(i18n("Refresh"), variant="primary") |
|
refresh_button.click( |
|
fn=change_choices, |
|
inputs=[], |
|
outputs=[sid1, file_index2], |
|
api_name="infer_refresh", |
|
) |
|
modelload_out = gr.Textbox(label="Model Metadata", interactive=False, lines=4) |
|
get_model_info_button = gr.Button(i18n("Get Model Info")) |
|
get_model_info_button.click( |
|
fn=vc.get_vc, |
|
inputs=[sid1, protect0, protect1], |
|
outputs=[spk_item, protect0, protect1, file_index2, file_index4, modelload_out] |
|
) |
|
|
|
|
|
with gr.Accordion('Audio Analyser', open=False): |
|
with gr.Column(): |
|
audio_input = gr.Audio(type="filepath") |
|
get_info_button = gr.Button( |
|
value=i18n("Get information about the audio"), variant="primary" |
|
) |
|
|
|
with gr.Column(): |
|
with gr.Row(): |
|
with gr.Column(): |
|
gr.Markdown( |
|
value=i18n("Information about the audio file"), |
|
visible=True, |
|
) |
|
output_markdown = gr.Markdown( |
|
value=i18n("Waiting for information..."), visible=True |
|
) |
|
image_output = gr.Image(type="filepath", interactive=False) |
|
|
|
get_info_button.click( |
|
fn=generate_spectrogram_and_get_info, |
|
inputs=[audio_input], |
|
outputs=[output_markdown, image_output], |
|
) |
|
|
|
with gr.Accordion('Training Helper', open=False): |
|
with gr.Column(): |
|
audio_input = gr.Audio(type="filepath", label="Upload your audio file") |
|
gr.Text("Please note that these results are approximate and intended to provide a general idea for beginners.", label='Notice:') |
|
training_info_output = gr.Markdown(label="Training Information:") |
|
get_info_button = gr.Button("Get Training Info") |
|
get_info_button.click( |
|
fn=on_button_click, |
|
inputs=[audio_input], |
|
outputs=[training_info_output] |
|
) |
|
|
|
with gr.Accordion('Credits', open=False): |
|
gr.Markdown(''' |
|
## All the amazing people who worked on this! |
|
|
|
### Developers |
|
|
|
- **Ilaria**: Founder, Lead Developer |
|
- **Yui**: Training feature |
|
- **GDR-**: Inference feature |
|
- **Poopmaster**: Model downloader, Model importer |
|
- **kitlemonfoot**: Ilaria TTS implementation |
|
- **eddycrack864**: UVR5 implementation |
|
- **Diablo**: Bug Fixes, UI help. |
|
- **Mikus**: Ilaria Updater & Downloader |
|
- **Mitsubachi1**: Bug Fixes, UI help |
|
|
|
### Beta Tester |
|
|
|
- **Charlotte**: Beta Tester |
|
- **RME**: Beta Tester |
|
- **Delik**: Beta Tester |
|
|
|
### Pretrains Makers |
|
|
|
- **simplcup**: Ov2Super |
|
- **mustar22**: RIN_E3 |
|
- **mustar22**: Snowie |
|
|
|
### Other |
|
|
|
- **RVC Project**: Original Developers |
|
- **yumereborn**: Ilaria RVC image |
|
|
|
### **In loving memory of JLabDX** 🕊️ |
|
''') |
|
|
|
sid0.change( |
|
fn=vc.get_vc, |
|
inputs=[sid0, protect0, protect1], |
|
outputs=[spk_item, protect0, protect1, file_index2, file_index4, modelload_out], |
|
api_name="infer_change_voice", |
|
) |
|
with gr.TabItem(i18n("")): |
|
gr.Markdown(''' |
|
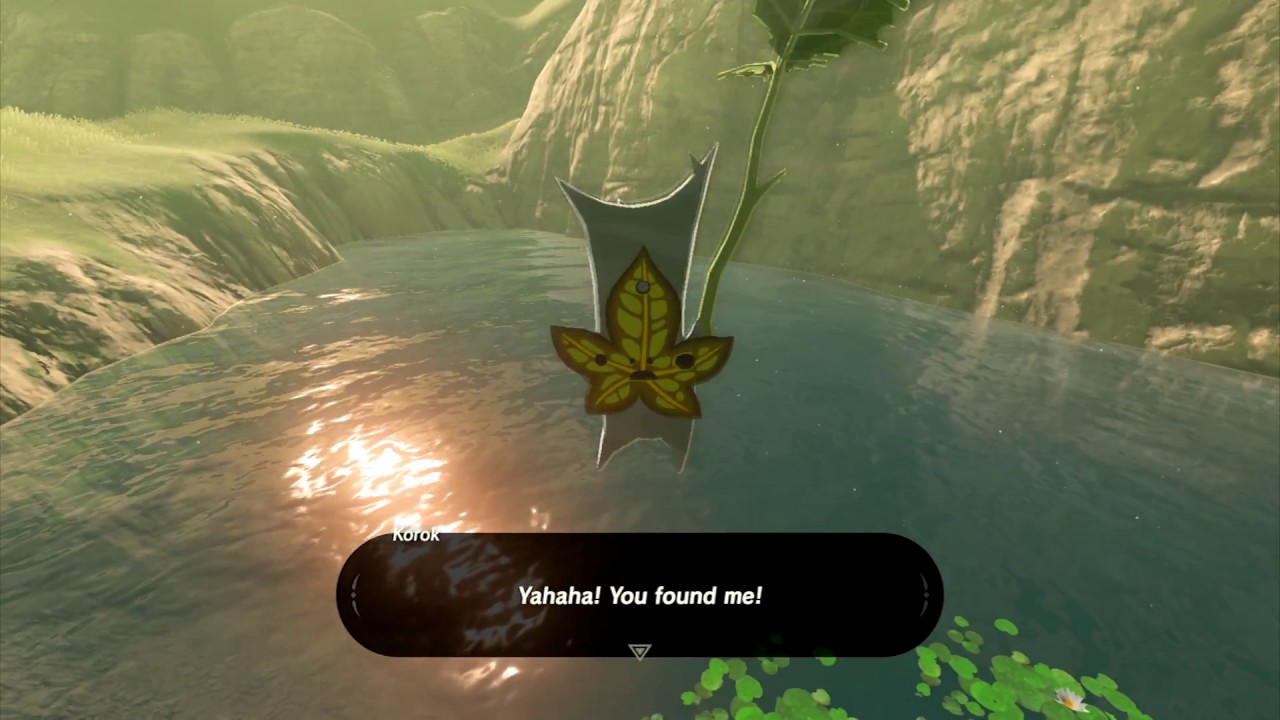 |
|
''') |
|
if config.iscolab: |
|
app.queue(concurrency_count=511, max_size=1022).launch(share=True) |
|
else: |
|
app.queue(concurrency_count=1, max_size=20).launch(share=False |
|
) |
|
|