Spaces:
Sleeping
Sleeping
File size: 8,503 Bytes
4b75840 |
1 2 3 4 5 6 7 8 9 10 11 12 13 14 15 16 17 18 19 20 21 22 23 24 25 26 27 28 29 30 31 32 33 34 35 36 37 38 39 40 41 42 43 44 45 46 47 48 49 50 51 52 53 54 55 56 57 58 59 60 61 62 63 64 65 66 67 68 69 70 71 72 73 74 75 76 77 78 79 80 81 82 83 84 85 86 87 88 89 90 91 92 93 94 95 96 97 98 99 100 101 102 103 104 105 106 107 108 109 110 111 112 113 114 115 116 117 118 119 120 121 122 123 124 125 126 127 128 129 130 131 132 133 134 135 136 137 138 139 140 141 142 143 144 145 146 147 148 149 150 151 152 153 154 155 156 157 158 159 160 161 162 163 164 165 166 167 168 169 170 171 172 173 174 175 176 177 178 179 180 181 182 183 184 185 186 187 188 189 190 191 192 193 194 195 196 197 198 199 200 201 202 203 204 205 206 207 208 209 210 211 212 213 214 215 216 217 218 219 220 221 222 223 224 225 226 227 228 229 230 231 232 233 234 235 236 237 238 239 240 241 242 243 244 245 246 247 248 249 250 |
import pandas as pd
import streamlit as st
from text_annotation import annotated_text
from streamlit_option_menu import option_menu
from sentiment_analysis import SentimentAnalysis
from keyword_extraction import KeywordExtractor
from part_of_speech_tagging import POSTagging
from emotion_detection import EmotionDetection
from named_entity_recognition import NamedEntityRecognition
hide_streamlit_style = """
<style>
#MainMenu {visibility: hidden;}
footer {visibility: hidden;}
</style>
"""
st.markdown(hide_streamlit_style, unsafe_allow_html=True)
@st.cache(allow_output_mutation=True)
def load_sentiment_model():
return SentimentAnalysis()
@st.cache(allow_output_mutation=True)
def load_keyword_model():
return KeywordExtractor()
@st.cache(allow_output_mutation=True)
def load_pos_model():
return POSTagging()
@st.cache(allow_output_mutation=True)
def load_emotion_model():
return EmotionDetection()
@st.cache(allow_output_mutation=True)
def load_ner_model():
return NamedEntityRecognition()
sentiment_analyzer = load_sentiment_model()
keyword_extractor = load_keyword_model()
pos_tagger = load_pos_model()
emotion_detector = load_emotion_model()
ner = load_ner_model()
with st.sidebar:
page = option_menu(menu_title='Menu',
menu_icon="robot",
options=["Welcome!",
"Sentiment Analysis",
"Keyword Extraction",
"Part of Speech Tagging",
"Emotion Detection",
"Named Entity Recognition"],
icons=["house-door",
"emoji-heart-eyes",
"key",
"chat-dots",
"emoji-heart-eyes",
"building"],
default_index=0
)
st.title('Open-source NLP')
if page == "Welcome!":
st.header('Welcome!')
st.write(
"""
Supercharge your workflow with this platform built using 100% open-source resources!
"""
)
st.markdown("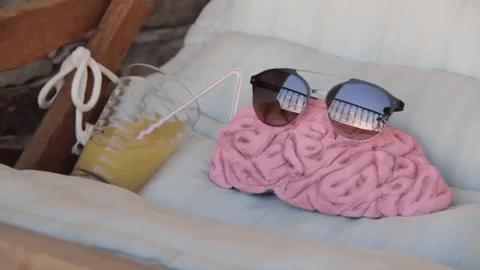")
st.write(
"""
"""
)
st.subheader("Introduction")
st.write("""
Welcome! This application is a celebration of open-source and the power that programmers have been granted today
by those who give back to the community. This tool was constructed using Streamlit, Huggingface Transformers,
Transformers-Interpret, NLTK, Spacy, amongst other open-source Python libraries and models.
Utilizing this tool you will be able to perform a multitude of Natural Language Processing Tasks on a range of
different tasks. All you need to do is paste your input, select your task, and hit the start button!
* This application currently supports:
* Sentiment Analysis
* Keyword Extraction
* Part of Speech Tagging
* Emotion Detection
* Named Entity Recognition
More features may be added in the future, depending on community feedback. Please reach out to me at
[email protected] or at my Linkedin page listed below if you have ideas or suggestions for improvement.
If you would like to contribute yourself, feel free to fork the Github repository listed below and submit a merge request.
"""
)
st.subheader("Notes")
st.write(
"""
* This dashboard was contsructed by Jacob Miesner, but every resource used is open-source! If you are interested
in his other works you can view them here:
[Project Github](https://github.com/MiesnerJacob/nlp-dashboard)
[Jacob Miesner's Github](https://github.com/MiesnerJacob)
[Jacob Miesner's Linkedin](https://www.linkedin.com/in/jacob-miesner-885050125/)
[Jacob Miesner's Website](https://www.jacobmiesner.com)
* The prediction justification for some of the tasks are printed as the model views them. For this reason the text
may contain special tokens like [CLS] or [SEP] or even hashtags splitting words. If you are knowledgeable about
language models and how they work these will be familiar, if you do not have prior experience with language models
you can ignore these characters.
"""
)
elif page == "Sentiment Analysis":
st.header('Sentiment Analysis')
st.markdown("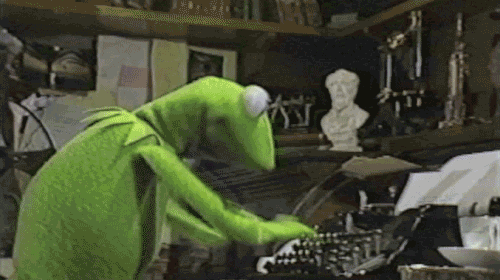")
st.write(
"""
"""
)
text = st.text_area("Paste text here", value="")
if st.button('Start!'):
with st.spinner("Loading..."):
preds, html = sentiment_analyzer.run(text)
st.success('All done!')
st.write("")
st.subheader("Sentiment Predictions")
st.bar_chart(data=preds, width=0, height=0, use_container_width=True)
st.write("")
st.subheader("Sentiment Justification")
raw_html = html._repr_html_()
st.components.v1.html(raw_html)
elif page == "Keyword Extraction":
st.header('Keyword Extraction')
st.markdown("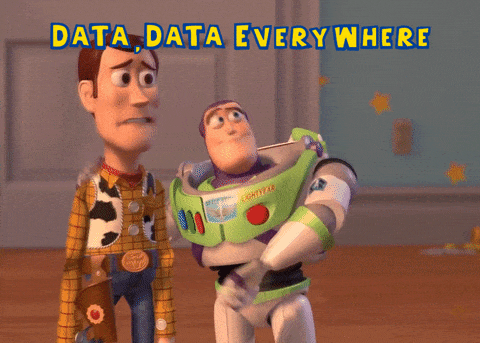")
st.write(
"""
"""
)
text = st.text_area("Paste text here", value="")
max_keywords = st.slider('# of Keywords Max Limit', min_value=1, max_value=10, value=5, step=1)
if st.button('Start!'):
with st.spinner("Loading..."):
annotation, keywords = keyword_extractor.generate(text, max_keywords)
st.success('All done!')
if annotation:
st.subheader("Keyword Annotation")
st.write("")
annotated_text(*annotation)
st.text("")
st.subheader("Extracted Keywords")
st.write("")
df = pd.DataFrame(keywords, columns=['Extracted Keywords'])
csv = df.to_csv(index=False).encode('utf-8')
st.download_button('Download Keywords to CSV', csv, file_name='news_intelligence_keywords.csv')
data_table = st.table(df)
elif page == "Part of Speech Tagging":
st.header('Part of Speech Tagging')
st.markdown("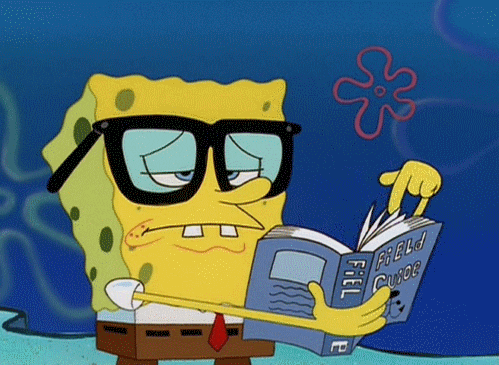")
st.write(
"""
"""
)
text = st.text_area("Paste text here", value="")
if st.button('Start!'):
with st.spinner("Loading..."):
preds = pos_tagger.classify(text)
st.success('All done!')
st.write("")
st.subheader("Part of Speech tags")
annotated_text(*preds)
st.write("")
st.components.v1.iframe('https://www.ling.upenn.edu/courses/Fall_2003/ling001/penn_treebank_pos.html', height=1000)
elif page == "Emotion Detection":
st.header('Emotion Detection')
st.markdown("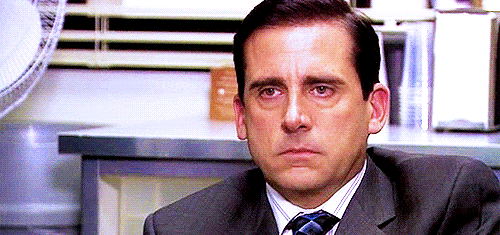")
st.write(
"""
"""
)
text = st.text_area("Paste text here", value="")
if st.button('Start!'):
with st.spinner("Loading..."):
preds, html = emotion_detector.run(text)
st.success('All done!')
st.write("")
st.subheader("Emotion Predictions")
st.bar_chart(data=preds, width=0, height=0, use_container_width=True)
raw_html = html._repr_html_()
st.write("")
st.subheader("Emotion Justification")
st.components.v1.html(raw_html, height=500)
elif page == "Named Entity Recognition":
st.header('Named Entity Recognition')
st.markdown("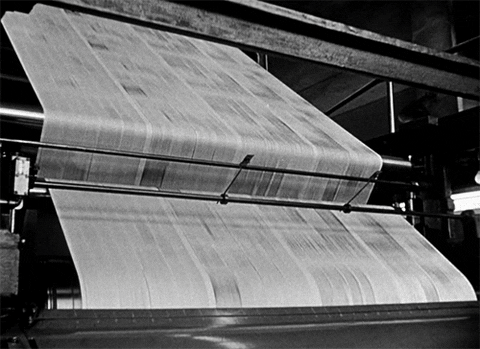")
st.write(
"""
"""
)
text = st.text_area("Paste text here", value="")
if st.button('Start!'):
with st.spinner("Loading..."):
preds, ner_annotation = ner.classify(text)
st.success('All done!')
st.write("")
st.subheader("NER Predictions")
annotated_text(*ner_annotation)
st.write("")
st.subheader("NER Prediction Metadata")
st.write(preds)
|