# Quicktour
There are many ways to launch and run your code depending on your training environment ([torchrun](https://pytorch.org/docs/stable/elastic/run.html), [DeepSpeed](https://www.deepspeed.ai/), etc.) and available hardware. Accelerate offers a unified interface for launching and training on different distributed setups, allowing you to focus on your PyTorch training code instead of the intricacies of adapting your code to these different setups. This allows you to easily scale your PyTorch code for training and inference on distributed setups with hardware like GPUs and TPUs. Accelerate also provides Big Model Inference to make loading and running inference with really large models that usually don't fit in memory more accessible.
This quicktour introduces the three main features of Accelerate:
* a unified command line launching interface for distributed training scripts
* a training library for adapting PyTorch training code to run on different distributed setups
* Big Model Inference
## Unified launch interface
Accelerate automatically selects the appropriate configuration values for any given distributed training framework (DeepSpeed, FSDP, etc.) through a unified configuration file generated from the [`accelerate config`](package_reference/cli#accelerate-config) command. You could also pass the configuration values explicitly to the command line which is helpful in certain situations like if you're using SLURM.
But in most cases, you should always run [`accelerate config`](package_reference/cli#accelerate-config) first to help Accelerate learn about your training setup.
```bash
accelerate config
```
The [`accelerate config`](package_reference/cli#accelerate-config) command creates and saves a default_config.yaml file in Accelerates cache folder. This file stores the configuration for your training environment, which helps Accelerate correctly launch your training script based on your machine.
After you've configured your environment, you can test your setup with [`accelerate test`](package_reference/cli#accelerate-test), which launches a short script to test the distributed environment.
```bash
accelerate test
```
> [!TIP]
> Add `--config_file` to the `accelerate test` or `accelerate launch` command to specify the location of the configuration file if it is saved in a non-default location like the cache.
Once your environment is setup, launch your training script with [`accelerate launch`](package_reference/cli#accelerate-launch)!
```bash
accelerate launch path_to_script.py --args_for_the_script
```
To learn more, check out the [Launch distributed code](basic_tutorials/launch) tutorial for more information about launching your scripts.
We also have a [configuration zoo](https://github.com/huggingface/accelerate/blob/main/examples/config_yaml_templates) which showcases a number of premade **minimal** example configurations for a variety of setups you can run.
## Adapt training code
The next main feature of Accelerate is the `Accelerator` class which adapts your PyTorch code to run on different distributed setups.
You only need to add a few lines of code to your training script to enable it to run on multiple GPUs or TPUs.
```diff
+ from accelerate import Accelerator
+ accelerator = Accelerator()
+ device = accelerator.device
+ model, optimizer, training_dataloader, scheduler = accelerator.prepare(
+ model, optimizer, training_dataloader, scheduler
+ )
for batch in training_dataloader:
optimizer.zero_grad()
inputs, targets = batch
- inputs = inputs.to(device)
- targets = targets.to(device)
outputs = model(inputs)
loss = loss_function(outputs, targets)
+ accelerator.backward(loss)
optimizer.step()
scheduler.step()
```
1. Import and instantiate the `Accelerator` class at the beginning of your training script. The `Accelerator` class initializes everything necessary for distributed training, and it automatically detects your training environment (a single machine with a GPU, a machine with several GPUs, several machines with multiple GPUs or a TPU, etc.) based on how the code was launched.
```python
from accelerate import Accelerator
accelerator = Accelerator()
```
2. Remove calls like `.cuda()` on your model and input data. The `Accelerator` class automatically places these objects on the appropriate device for you.
> [!WARNING]
> This step is *optional* but it is considered best practice to allow Accelerate to handle device placement. You could also deactivate automatic device placement by passing `device_placement=False` when initializing the `Accelerator`. If you want to explicitly place objects on a device with `.to(device)`, make sure you use `accelerator.device` instead. For example, if you create an optimizer before placing a model on `accelerator.device`, training fails on a TPU.
> [!WARNING]
> Accelerate does not use non-blocking transfers by default for its automatic device placement, which can result in potentially unwanted CUDA synchronizations. You can enable non-blocking transfers by passing a `DataLoaderConfiguration` with `non_blocking=True` set as the `dataloader_config` when initializing the `Accelerator`. As usual, non-blocking transfers will only work if the dataloader also has `pin_memory=True` set. Be wary that using non-blocking transfers from GPU to CPU may cause incorrect results if it results in CPU operations being performed on non-ready tensors.
```py
device = accelerator.device
```
3. Pass all relevant PyTorch objects for training (optimizer, model, dataloader(s), learning rate scheduler) to the `prepare()` method as soon as they're created. This method wraps the model in a container optimized for your distributed setup, uses Accelerates version of the optimizer and scheduler, and creates a sharded version of your dataloader for distribution across GPUs or TPUs.
```python
model, optimizer, train_dataloader, lr_scheduler = accelerator.prepare(
model, optimizer, train_dataloader, lr_scheduler
)
```
4. Replace `loss.backward()` with `backward()` to use the correct `backward()` method for your training setup.
```py
accelerator.backward(loss)
```
Read [Accelerate’s internal mechanisms](concept_guides/internal_mechanism) guide to learn more details about how Accelerate adapts your code.
### Distributed evaluation
To perform distributed evaluation, pass your validation dataloader to the `prepare()` method:
```python
validation_dataloader = accelerator.prepare(validation_dataloader)
```
Each device in your distributed setup only receives a part of the evaluation data, which means you should group your predictions together with the `gather_for_metrics()` method. This method requires all tensors to be the same size on each process, so if your tensors have different sizes on each process (for instance when dynamically padding to the maximum length in a batch), you should use the `pad_across_processes()` method to pad you tensor to the largest size across processes. Note that the tensors needs to be 1D and that we concatenate the tensors along the first dimension.
```python
for inputs, targets in validation_dataloader:
predictions = model(inputs)
# Gather all predictions and targets
all_predictions, all_targets = accelerator.gather_for_metrics((predictions, targets))
# Example of use with a *Datasets.Metric*
metric.add_batch(all_predictions, all_targets)
```
For more complex cases (e.g. 2D tensors, don't want to concatenate tensors, dict of 3D tensors), you can pass `use_gather_object=True` in `gather_for_metrics`. This will return the list of objects after gathering. Note that using it with GPU tensors is not well supported and inefficient.
> [!TIP]
> Data at the end of a dataset may be duplicated so the batch can be equally divided among all workers. The `gather_for_metrics()` method automatically removes the duplicated data to calculate a more accurate metric.
## Big Model Inference
Accelerate's Big Model Inference has two main features, `init_empty_weights()` and `load_checkpoint_and_dispatch()`, to load large models for inference that typically don't fit into memory.
> [!TIP]
> Take a look at the [Handling big models for inference](concept_guides/big_model_inference) guide for a better understanding of how Big Model Inference works under the hood.
### Empty weights initialization
The `init_empty_weights()` context manager initializes models of any size by creating a *model skeleton* and moving and placing parameters each time they're created to PyTorch's [**meta**](https://pytorch.org/docs/main/meta.html) device. This way, not all weights are immediately loaded and only a small part of the model is loaded into memory at a time.
For example, loading an empty [Mixtral-8x7B](https://huggingface.co/mistralai/Mixtral-8x7B-Instruct-v0.1) model takes significantly less memory than fully loading the models and weights on the CPU.
```py
from accelerate import init_empty_weights
from transformers import AutoConfig, AutoModelForCausalLM
config = AutoConfig.from_pretrained("mistralai/Mixtral-8x7B-Instruct-v0.1")
with init_empty_weights():
model = AutoModelForCausalLM.from_config(config)
```
### Load and dispatch weights
The `load_checkpoint_and_dispatch()` function loads full or sharded checkpoints into the empty model, and automatically distribute weights across all available devices.
The `device_map` parameter determines where to place each model layer, and specifiying `"auto"` places them on the GPU first, then the CPU, and finally the hard drive as memory-mapped tensors if there's still not enough memory. Use the `no_split_module_classes` parameter to indicate which modules shouldn't be split across devices (typically those with a residual connection).
```py
from accelerate import load_checkpoint_and_dispatch
model = load_checkpoint_and_dispatch(
model, checkpoint="mistralai/Mixtral-8x7B-Instruct-v0.1", device_map="auto", no_split_module_classes=['Block']
)
```
## Next steps
Now that you've been introduced to the main Accelerate features, your next steps could include:
* Check out the [tutorials](basic_tutorials/overview) for a gentle walkthrough of Accelerate. This is especially useful if you're new to distributed training and the library.
* Dive into the [guides](usage_guides/explore) to see how to use Accelerate for specific use-cases.
* Deepen your conceptual understanding of how Accelerate works internally by reading the [concept guides](concept_guides/internal_mechanism).
* Look up classes and commands in the [API reference](package_reference/accelerator) to see what parameters and options are available.
# Accelerate
Accelerate is a library that enables the same PyTorch code to be run across any distributed configuration by adding just four lines of code! In short, training and inference at scale made simple, efficient and adaptable.
```diff
+ from accelerate import Accelerator
+ accelerator = Accelerator()
+ model, optimizer, training_dataloader, scheduler = accelerator.prepare(
+ model, optimizer, training_dataloader, scheduler
+ )
for batch in training_dataloader:
optimizer.zero_grad()
inputs, targets = batch
inputs = inputs.to(device)
targets = targets.to(device)
outputs = model(inputs)
loss = loss_function(outputs, targets)
+ accelerator.backward(loss)
optimizer.step()
scheduler.step()
```
Built on `torch_xla` and `torch.distributed`, Accelerate takes care of the heavy lifting, so you don't have to write any custom code to adapt to these platforms.
Convert existing codebases to utilize [DeepSpeed](usage_guides/deepspeed), perform [fully sharded data parallelism](usage_guides/fsdp), and have automatic support for mixed-precision training!
To get a better idea of this process, make sure to check out the [Tutorials](basic_tutorials/overview)!
This code can then be launched on any system through Accelerate's CLI interface:
```bash
accelerate launch {my_script.py}
```
# Fully Sharded Data Parallel
To accelerate training huge models on larger batch sizes, we can use a fully sharded data parallel model.
This type of data parallel paradigm enables fitting more data and larger models by sharding the optimizer states, gradients and parameters.
To read more about it and the benefits, check out the [Fully Sharded Data Parallel blog](https://pytorch.org/blog/introducing-pytorch-fully-sharded-data-parallel-api/).
We have integrated the latest PyTorch's Fully Sharded Data Parallel (FSDP) training feature.
All you need to do is enable it through the config.
## How it works out of the box
On your machine(s) just run:
```bash
accelerate config
```
and answer the questions asked. This will generate a config file that will be used automatically to properly set the
default options when doing
```bash
accelerate launch my_script.py --args_to_my_script
```
For instance, here is how you would run `examples/nlp_example.py` (from the root of the repo) with FSDP enabled:
```bash
compute_environment: LOCAL_MACHINE
debug: false
distributed_type: FSDP
downcast_bf16: 'no'
fsdp_config:
fsdp_auto_wrap_policy: TRANSFORMER_BASED_WRAP
fsdp_backward_prefetch_policy: BACKWARD_PRE
fsdp_forward_prefetch: false
fsdp_cpu_ram_efficient_loading: true
fsdp_offload_params: false
fsdp_sharding_strategy: FULL_SHARD
fsdp_state_dict_type: SHARDED_STATE_DICT
fsdp_sync_module_states: true
fsdp_transformer_layer_cls_to_wrap: BertLayer
fsdp_use_orig_params: true
machine_rank: 0
main_training_function: main
mixed_precision: bf16
num_machines: 1
num_processes: 2
rdzv_backend: static
same_network: true
tpu_env: []
tpu_use_cluster: false
tpu_use_sudo: false
use_cpu: false
```
```bash
accelerate launch examples/nlp_example.py
```
Currently, `Accelerate` supports the following config through the CLI:
`fsdp_sharding_strategy`: [1] FULL_SHARD (shards optimizer states, gradients and parameters), [2] SHARD_GRAD_OP (shards optimizer states and gradients), [3] NO_SHARD (DDP), [4] HYBRID_SHARD (shards optimizer states, gradients and parameters within each node while each node has full copy), [5] HYBRID_SHARD_ZERO2 (shards optimizer states and gradients within each node while each node has full copy). For more information, please refer the official [PyTorch docs](https://pytorch.org/docs/stable/fsdp.html#torch.distributed.fsdp.ShardingStrategy).
`fsdp_offload_params` : Decides Whether to offload parameters and gradients to CPU
`fsdp_auto_wrap_policy`: [1] TRANSFORMER_BASED_WRAP, [2] SIZE_BASED_WRAP, [3] NO_WRAP
`fsdp_transformer_layer_cls_to_wrap`: Only applicable for Transformers. When using `fsdp_auto_wrap_policy=TRANSFORMER_BASED_WRAP`, a user may provide a comma-separated string of transformer layer class names (case-sensitive) to wrap, e.g., `BertLayer`, `GPTJBlock`, `T5Block`, `BertLayer,BertEmbeddings,BertSelfOutput`. This is important because submodules that share weights (e.g., embedding layers) should not end up in different FSDP wrapped units. Using this policy, wrapping happens for each block containing Multi-Head Attention followed by a couple of MLP layers. Remaining layers including the shared embeddings are conveniently wrapped in same outermost FSDP unit. Therefore, use this for transformer-based models. You can use the `model._no_split_modules` for Transformer models by answering `yes` to `Do you want to use the model's `_no_split_modules` to wrap. It will try to use `model._no_split_modules` when possible.
`fsdp_min_num_params`: minimum number of parameters when using `fsdp_auto_wrap_policy=SIZE_BASED_WRAP`.
`fsdp_backward_prefetch_policy`: [1] BACKWARD_PRE, [2] BACKWARD_POST, [3] NO_PREFETCH
`fsdp_forward_prefetch`: if True, then FSDP explicitly prefetches the next upcoming all-gather while executing in the forward pass. Should only be used for static-graph models since the prefetching follows the first iteration’s execution order. i.e., if the sub-modules' order changes dynamically during the model's execution do not enable this feature.
`fsdp_state_dict_type`: [1] FULL_STATE_DICT, [2] LOCAL_STATE_DICT, [3] SHARDED_STATE_DICT
`fsdp_use_orig_params`: If True, allows non-uniform `requires_grad` during init, which means support for interspersed frozen and trainable parameters. This setting is useful in cases such as parameter-efficient fine-tuning as discussed in [this post](https://dev-discuss.pytorch.org/t/rethinking-pytorch-fully-sharded-data-parallel-fsdp-from-first-principles/1019). This option also allows one to have multiple optimizer param groups. This should be `True` when creating an optimizer before preparing/wrapping the model with FSDP.
`fsdp_cpu_ram_efficient_loading`: Only applicable for Transformers models. If True, only the first process loads the pretrained model checkpoint while all other processes have empty weights. This should be set to False if you experience errors when loading the pretrained Transformers model via `from_pretrained` method. When this setting is True `fsdp_sync_module_states` also must to be True, otherwise all the processes except the main process would have random weights leading to unexpected behaviour during training. For this to work, make sure the distributed process group is initialized before calling Transformers `from_pretrained` method. When using Trainer API, the distributed process group is initialized when you create an instance of `TrainingArguments` class.
`fsdp_sync_module_states`: If True, each individually wrapped FSDP unit will broadcast module parameters from rank 0.
For additional and more nuanced control, you can specify other FSDP parameters via `FullyShardedDataParallelPlugin`.
When creating `FullyShardedDataParallelPlugin` object, pass it the parameters that weren't part of the accelerate config or if you want to override them.
The FSDP parameters will be picked based on the accelerate config file or launch command arguments and other parameters that you will pass directly through the `FullyShardedDataParallelPlugin` object will set/override that.
Below is an example:
```py
from accelerate import FullyShardedDataParallelPlugin
from torch.distributed.fsdp.fully_sharded_data_parallel import FullOptimStateDictConfig, FullStateDictConfig
fsdp_plugin = FullyShardedDataParallelPlugin(
state_dict_config=FullStateDictConfig(offload_to_cpu=False, rank0_only=False),
optim_state_dict_config=FullOptimStateDictConfig(offload_to_cpu=False, rank0_only=False),
)
accelerator = Accelerator(fsdp_plugin=fsdp_plugin)
```
## Saving and loading
The new recommended way of checkpointing when using FSDP models is to use `SHARDED_STATE_DICT` as `StateDictType` when setting up the accelerate config.
Below is the code snippet to save using `save_state` utility of accelerate.
```py
accelerator.save_state("ckpt")
```
Inspect the checkpoint folder to see model and optimizer as shards per process:
```
ls ckpt
# optimizer_0 pytorch_model_0 random_states_0.pkl random_states_1.pkl scheduler.bin
cd ckpt
ls optimizer_0
# __0_0.distcp __1_0.distcp
ls pytorch_model_0
# __0_0.distcp __1_0.distcp
```
To load them back for resuming the training, use the `load_state` utility of accelerate
```py
accelerator.load_state("ckpt")
```
When using transformers `save_pretrained`, pass `state_dict=accelerator.get_state_dict(model)` to save the model state dict.
Below is an example:
```diff
unwrapped_model.save_pretrained(
args.output_dir,
is_main_process=accelerator.is_main_process,
save_function=accelerator.save,
+ state_dict=accelerator.get_state_dict(model),
)
```
### State Dict
`accelerator.get_state_dict` will call the underlying `model.state_dict` implementation using `FullStateDictConfig(offload_to_cpu=True, rank0_only=True)` context manager to get the state dict only for rank 0 and it will be offloaded to CPU.
You can then pass `state` into the `save_pretrained` method. There are several modes for `StateDictType` and `FullStateDictConfig` that you can use to control the behavior of `state_dict`. For more information, see the [PyTorch documentation](https://pytorch.org/docs/stable/fsdp.html).
If you choose to use `StateDictType.SHARDED_STATE_DICT`, the weights of the model during `Accelerator.save_state` will be split into `n` files for each sub-split on the model. To merge them back into
a single dictionary to load back into the model later after training you can use the `merge_weights` utility:
```py
from accelerate.utils import merge_fsdp_weights
# Our weights are saved usually in a `pytorch_model_fsdp_{model_number}` folder
merge_fsdp_weights("pytorch_model_fsdp_0", "output_path", safe_serialization=True)
```
The final output will then either be saved to `model.safetensors` or `pytorch_model.bin` (if `safe_serialization=False` is passed).
This can also be called using the CLI:
```bash
accelerate merge-weights pytorch_model_fsdp_0/ output_path
```
## Mapping between FSDP sharding strategies and DeepSpeed ZeRO Stages
* `FULL_SHARD` maps to the DeepSpeed `ZeRO Stage-3`. Shards optimizer states, gradients and parameters.
* `SHARD_GRAD_OP` maps to the DeepSpeed `ZeRO Stage-2`. Shards optimizer states and gradients.
* `NO_SHARD` maps to `ZeRO Stage-0`. No sharding wherein each GPU has full copy of model, optimizer states and gradients.
* `HYBRID_SHARD` maps to `ZeRO++ Stage-3` wherein `zero_hpz_partition_size=`. Here, this will shard optimizer states, gradients and parameters within each node while each node has full copy.
## A few caveats to be aware of
- In case of multiple models, pass the optimizers to the prepare call in the same order as corresponding models else `accelerator.save_state()` and `accelerator.load_state()` will result in wrong/unexpected behaviour.
- This feature is incompatible with `--predict_with_generate` in the `run_translation.py` script of `Transformers` library.
For more control, users can leverage the `FullyShardedDataParallelPlugin`. After creating an instance of this class, users can pass it to the Accelerator class instantiation.
For more information on these options, please refer to the PyTorch [FullyShardedDataParallel](https://github.com/pytorch/pytorch/blob/0df2e863fbd5993a7b9e652910792bd21a516ff3/torch/distributed/fsdp/fully_sharded_data_parallel.py#L236) code.
For those interested in the similarities and differences between FSDP and DeepSpeed, please check out the [concept guide here](../concept_guides/fsdp_and_deepspeed)!
# Checkpointing
When training a PyTorch model with Accelerate, you may often want to save and continue a state of training. Doing so requires
saving and loading the model, optimizer, RNG generators, and the GradScaler. Inside Accelerate are two convenience functions to achieve this quickly:
- Use `save_state()` for saving everything mentioned above to a folder location
- Use `load_state()` for loading everything stored from an earlier `save_state`
To further customize where and how states are saved through `save_state()` the `ProjectConfiguration` class can be used. For example
if `automatic_checkpoint_naming` is enabled each saved checkpoint will be located then at `Accelerator.project_dir/checkpoints/checkpoint_{checkpoint_number}`.
It should be noted that the expectation is that those states come from the same training script, they should not be from two separate scripts.
- By using `register_for_checkpointing()`, you can register custom objects to be automatically stored or loaded from the two prior functions,
so long as the object has a `state_dict` **and** a `load_state_dict` functionality. This could include objects such as a learning rate scheduler.
Below is a brief example using checkpointing to save and reload a state during training:
```python
from accelerate import Accelerator
import torch
accelerator = Accelerator(project_dir="my/save/path")
my_scheduler = torch.optim.lr_scheduler.StepLR(my_optimizer, step_size=1, gamma=0.99)
my_model, my_optimizer, my_training_dataloader = accelerator.prepare(my_model, my_optimizer, my_training_dataloader)
# Register the LR scheduler
accelerator.register_for_checkpointing(my_scheduler)
# Save the starting state
accelerator.save_state()
device = accelerator.device
my_model.to(device)
# Perform training
for epoch in range(num_epochs):
for batch in my_training_dataloader:
my_optimizer.zero_grad()
inputs, targets = batch
inputs = inputs.to(device)
targets = targets.to(device)
outputs = my_model(inputs)
loss = my_loss_function(outputs, targets)
accelerator.backward(loss)
my_optimizer.step()
my_scheduler.step()
# Restore the previous state
accelerator.load_state("my/save/path/checkpointing/checkpoint_0")
```
## Restoring the state of the DataLoader
After resuming from a checkpoint, it may also be desirable to resume from a particular point in the active `DataLoader` if
the state was saved during the middle of an epoch. You can use `skip_first_batches()` to do so.
```python
from accelerate import Accelerator
accelerator = Accelerator(project_dir="my/save/path")
train_dataloader = accelerator.prepare(train_dataloader)
accelerator.load_state("my_state")
# Assume the checkpoint was saved 100 steps into the epoch
skipped_dataloader = accelerator.skip_first_batches(train_dataloader, 100)
# After the first iteration, go back to `train_dataloader`
# First epoch
for batch in skipped_dataloader:
# Do something
pass
# Second epoch
for batch in train_dataloader:
# Do something
pass
```
# DeepSpeed
[DeepSpeed](https://github.com/microsoft/DeepSpeed) implements everything described in the [ZeRO paper](https://arxiv.org/abs/1910.02054). Some of the salient optimizations are:
1. Optimizer state partitioning (ZeRO stage 1)
2. Gradient partitioning (ZeRO stage 2)
3. Parameter partitioning (ZeRO stage 3)
4. Custom mixed precision training handling
5. A range of fast CUDA-extension-based optimizers
6. ZeRO-Offload to CPU and Disk/NVMe
7. Hierarchical partitioning of model parameters (ZeRO++)
ZeRO-Offload has its own dedicated paper: [ZeRO-Offload: Democratizing Billion-Scale Model Training](https://arxiv.org/abs/2101.06840). And NVMe-support is described in the paper [ZeRO-Infinity: Breaking the GPU
Memory Wall for Extreme Scale Deep Learning](https://arxiv.org/abs/2104.07857).
DeepSpeed ZeRO-2 is primarily used only for training, as its features are of no use to inference.
DeepSpeed ZeRO-3 can be used for inference as well since it allows huge models to be loaded on multiple GPUs, which
won't be possible on a single GPU.
Accelerate integrates [DeepSpeed](https://github.com/microsoft/DeepSpeed) via 2 options:
1. Integration of the DeepSpeed features via `deepspeed config file` specification in `accelerate config` . You just supply your custom config file or use our template. Most of
this document is focused on this feature. This supports all the core features of DeepSpeed and gives user a lot of flexibility.
User may have to change a few lines of code depending on the config.
2. Integration via `deepspeed_plugin`.This supports subset of the DeepSpeed features and uses default options for the rest of the configurations.
User need not change any code and is good for those who are fine with most of the default settings of DeepSpeed.
## What is integrated?
Training:
1. Accelerate integrates all features of DeepSpeed ZeRO. This includes all the ZeRO stages 1, 2 and 3 as well as ZeRO-Offload, ZeRO-Infinity (which can offload to disk/NVMe) and ZeRO++.
Below is a short description of Data Parallelism using ZeRO - Zero Redundancy Optimizer along with diagram from this [blog post](https://www.microsoft.com/en-us/research/blog/zero-deepspeed-new-system-optimizations-enable-training-models-with-over-100-billion-parameters/)
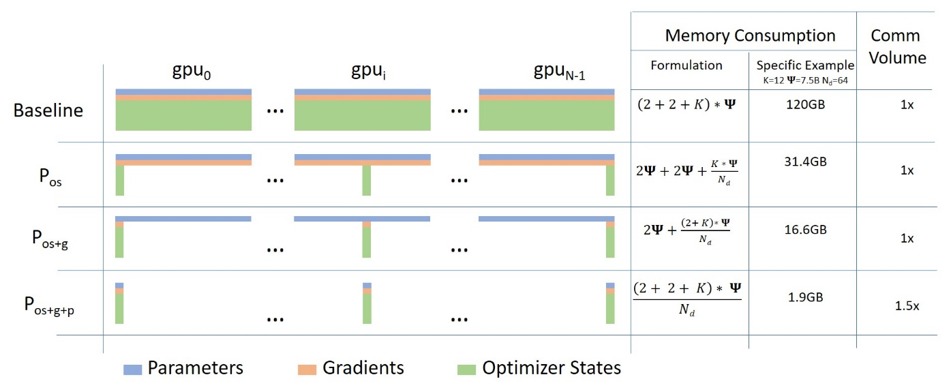
(Source: [link](https://www.microsoft.com/en-us/research/blog/zero-deepspeed-new-system-optimizations-enable-training-models-with-over-100-billion-parameters/))
a. **Stage 1** : Shards optimizer states across data parallel workers/GPUs
b. **Stage 2** : Shards optimizer states + gradients across data parallel workers/GPUs
c. **Stage 3**: Shards optimizer states + gradients + model parameters across data parallel workers/GPUs
d. **Optimizer Offload**: Offloads the gradients + optimizer states to CPU/Disk building on top of ZERO Stage 2
e. **Param Offload**: Offloads the model parameters to CPU/Disk building on top of ZERO Stage 3
f. **Hierarchical Partitioning**: Enables efficient multi-node training with data-parallel training across nodes and ZeRO-3 sharding within a node, built on top of ZeRO Stage 3.
Note: With respect to Disk Offload, the disk should be an NVME for decent speed but it technically works on any Disk
Inference:
1. DeepSpeed ZeRO Inference supports ZeRO stage 3 with ZeRO-Infinity. It uses the same ZeRO protocol as training, but
it doesn't use an optimizer and a lr scheduler and only stage 3 is relevant. For more details see:
[deepspeed-zero-inference](#deepspeed-zero-inference).
## How it works?
**Pre-Requisites**: Install DeepSpeed version >=0.6.5. Please refer to the [DeepSpeed Installation details](https://github.com/microsoft/DeepSpeed#installation)
for more information.
We will first look at easy to use integration via `accelerate config`.
Followed by more flexible and feature rich `deepspeed config file` integration.
### Accelerate DeepSpeed Plugin
On your machine(s) just run:
```bash
accelerate config
```
and answer the questions asked. It will ask whether you want to use a config file for DeepSpeed to which you should answer no. Then answer the following questions to generate a basic DeepSpeed config.
This will generate a config file that will be used automatically to properly set the
default options when doing
```bash
accelerate launch my_script.py --args_to_my_script
```
For instance, here is how you would run the NLP example `examples/nlp_example.py` (from the root of the repo) with DeepSpeed Plugin:
**ZeRO Stage-2 DeepSpeed Plugin Example**
```bash
compute_environment: LOCAL_MACHINE
deepspeed_config:
gradient_accumulation_steps: 1
gradient_clipping: 1.0
offload_optimizer_device: none
offload_param_device: none
zero3_init_flag: true
zero_stage: 2
distributed_type: DEEPSPEED
fsdp_config: {}
machine_rank: 0
main_process_ip: null
main_process_port: null
main_training_function: main
mixed_precision: fp16
num_machines: 1
num_processes: 2
use_cpu: false
```
```bash
accelerate launch examples/nlp_example.py --mixed_precision fp16
```
**ZeRO Stage-3 with CPU Offload DeepSpeed Plugin Example**
```bash
compute_environment: LOCAL_MACHINE
deepspeed_config:
gradient_accumulation_steps: 1
gradient_clipping: 1.0
offload_optimizer_device: cpu
offload_param_device: cpu
zero3_init_flag: true
zero3_save_16bit_model: true
zero_stage: 3
distributed_type: DEEPSPEED
fsdp_config: {}
machine_rank: 0
main_process_ip: null
main_process_port: null
main_training_function: main
mixed_precision: fp16
num_machines: 1
num_processes: 2
use_cpu: false
```
```bash
accelerate launch examples/nlp_example.py --mixed_precision fp16
```
Currently, `Accelerate` supports following config through the CLI:
```bash
`zero_stage`: [0] Disabled, [1] optimizer state partitioning, [2] optimizer+gradient state partitioning and [3] optimizer+gradient+parameter partitioning
`gradient_accumulation_steps`: Number of training steps to accumulate gradients before averaging and applying them.
`gradient_clipping`: Enable gradient clipping with value.
`offload_optimizer_device`: [none] Disable optimizer offloading, [cpu] offload optimizer to CPU, [nvme] offload optimizer to NVMe SSD. Only applicable with ZeRO >= Stage-2.
`offload_optimizer_nvme_path`: Decides Nvme Path to offload optimizer states. If unspecified, will default to 'none'.
`offload_param_device`: [none] Disable parameter offloading, [cpu] offload parameters to CPU, [nvme] offload parameters to NVMe SSD. Only applicable with ZeRO Stage-3.
`offload_param_nvme_path`: Decides Nvme Path to offload parameters. If unspecified, will default to 'none'.
`zero3_init_flag`: Decides whether to enable `deepspeed.zero.Init` for constructing massive models. Only applicable with ZeRO Stage-3.
`zero3_save_16bit_model`: Decides whether to save 16-bit model weights when using ZeRO Stage-3.
`mixed_precision`: `no` for FP32 training, `fp16` for FP16 mixed-precision training and `bf16` for BF16 mixed-precision training.
`deepspeed_moe_layer_cls_names`: Comma-separated list of transformer Mixture-of-Experts (MoE) layer class names (case-sensitive) to wrap ,e.g, `MixtralSparseMoeBlock`, `Qwen2MoeSparseMoeBlock`, `JetMoEAttention,JetMoEBlock` ...
`deepspeed_hostfile`: DeepSpeed hostfile for configuring multi-node compute resources.
`deepspeed_exclusion_filter`: DeepSpeed exclusion filter string when using mutli-node setup.
`deepspeed_inclusion_filter`: DeepSpeed inclusion filter string when using mutli-node setup.
`deepspeed_multinode_launcher`: DeepSpeed multi-node launcher to use. If unspecified, will default to `pdsh`.
`deepspeed_config_file`: path to the DeepSpeed config file in `json` format. See the next section for more details on this.
```
To be able to tweak more options, you will need to use a DeepSpeed config file.
### DeepSpeed Config File
On your machine(s) just run:
```bash
accelerate config
```
and answer the questions asked. It will ask whether you want to use a config file for deepspeed to which you answer yes
and provide the path to the deepspeed config file.
This will generate a config file that will be used automatically to properly set the
default options when doing
```bash
accelerate launch my_script.py --args_to_my_script
```
For instance, here is how you would run the NLP example `examples/by_feature/deepspeed_with_config_support.py` (from the root of the repo) with DeepSpeed Config File:
**ZeRO Stage-2 DeepSpeed Config File Example**
```bash
compute_environment: LOCAL_MACHINE
deepspeed_config:
deepspeed_config_file: /home/ubuntu/accelerate/examples/configs/deepspeed_config_templates/zero_stage2_config.json
zero3_init_flag: true
distributed_type: DEEPSPEED
fsdp_config: {}
machine_rank: 0
main_process_ip: null
main_process_port: null
main_training_function: main
mixed_precision: fp16
num_machines: 1
num_processes: 2
use_cpu: false
```
with the contents of `zero_stage2_config.json` being:
```json
{
"fp16": {
"enabled": true,
"loss_scale": 0,
"loss_scale_window": 1000,
"initial_scale_power": 16,
"hysteresis": 2,
"min_loss_scale": 1
},
"optimizer": {
"type": "AdamW",
"params": {
"lr": "auto",
"weight_decay": "auto",
"torch_adam": true,
"adam_w_mode": true
}
},
"scheduler": {
"type": "WarmupDecayLR",
"params": {
"warmup_min_lr": "auto",
"warmup_max_lr": "auto",
"warmup_num_steps": "auto",
"total_num_steps": "auto"
}
},
"zero_optimization": {
"stage": 2,
"allgather_partitions": true,
"allgather_bucket_size": 2e8,
"overlap_comm": true,
"reduce_scatter": true,
"reduce_bucket_size": "auto",
"contiguous_gradients": true
},
"gradient_accumulation_steps": 1,
"gradient_clipping": "auto",
"steps_per_print": 2000,
"train_batch_size": "auto",
"train_micro_batch_size_per_gpu": "auto",
"wall_clock_breakdown": false
}
```
```bash
accelerate launch examples/by_feature/deepspeed_with_config_support.py \
--config_name "gpt2-large" \
--tokenizer_name "gpt2-large" \
--dataset_name "wikitext" \
--dataset_config_name "wikitext-2-raw-v1" \
--block_size 128 \
--output_dir "./clm/clm_deepspeed_stage2_accelerate" \
--learning_rate 5e-4 \
--per_device_train_batch_size 24 \
--per_device_eval_batch_size 24 \
--num_train_epochs 3 \
--with_tracking \
--report_to "wandb"\
```
**ZeRO Stage-3 with CPU offload DeepSpeed Config File Example**
```bash
compute_environment: LOCAL_MACHINE
deepspeed_config:
deepspeed_config_file: /home/ubuntu/accelerate/examples/configs/deepspeed_config_templates/zero_stage3_offload_config.json
zero3_init_flag: true
distributed_type: DEEPSPEED
fsdp_config: {}
machine_rank: 0
main_process_ip: null
main_process_port: null
main_training_function: main
mixed_precision: fp16
num_machines: 1
num_processes: 2
use_cpu: false
```
with the contents of `zero_stage3_offload_config.json` being:
```json
{
"fp16": {
"enabled": true,
"loss_scale": 0,
"loss_scale_window": 1000,
"initial_scale_power": 16,
"hysteresis": 2,
"min_loss_scale": 1
},
"optimizer": {
"type": "AdamW",
"params": {
"lr": "auto",
"weight_decay": "auto"
}
},
"scheduler": {
"type": "WarmupDecayLR",
"params": {
"warmup_min_lr": "auto",
"warmup_max_lr": "auto",
"warmup_num_steps": "auto",
"total_num_steps": "auto"
}
},
"zero_optimization": {
"stage": 3,
"offload_optimizer": {
"device": "cpu",
"pin_memory": true
},
"offload_param": {
"device": "cpu",
"pin_memory": true
},
"overlap_comm": true,
"contiguous_gradients": true,
"reduce_bucket_size": "auto",
"stage3_prefetch_bucket_size": "auto",
"stage3_param_persistence_threshold": "auto",
"sub_group_size": 1e9,
"stage3_max_live_parameters": 1e9,
"stage3_max_reuse_distance": 1e9,
"stage3_gather_16bit_weights_on_model_save": "auto"
},
"gradient_accumulation_steps": 1,
"gradient_clipping": "auto",
"steps_per_print": 2000,
"train_batch_size": "auto",
"train_micro_batch_size_per_gpu": "auto",
"wall_clock_breakdown": false
}
```
```bash
accelerate launch examples/by_feature/deepspeed_with_config_support.py \
--config_name "gpt2-large" \
--tokenizer_name "gpt2-large" \
--dataset_name "wikitext" \
--dataset_config_name "wikitext-2-raw-v1" \
--block_size 128 \
--output_dir "./clm/clm_deepspeed_stage3_offload_accelerate" \
--learning_rate 5e-4 \
--per_device_train_batch_size 32 \
--per_device_eval_batch_size 32 \
--num_train_epochs 3 \
--with_tracking \
--report_to "wandb"\
```
**ZeRO++ Config Example**
You can use the features of ZeRO++ by using the appropriate config parameters. Note that ZeRO++ is an extension for ZeRO Stage 3. Here is how the config file can be modified, from [DeepSpeed's ZeRO++ tutorial](https://www.deepspeed.ai/tutorials/zeropp/):
```json
{
"zero_optimization": {
"stage": 3,
"reduce_bucket_size": "auto",
"zero_quantized_weights": true,
"zero_hpz_partition_size": 8,
"zero_quantized_gradients": true,
"contiguous_gradients": true,
"overlap_comm": true
}
}
```
For hierarchical partitioning, the partition size `zero_hpz_partition_size` should ideally be set to the number of GPUs per node. (For example, the above config file assumes 8 GPUs per node)
**Important code changes when using DeepSpeed Config File**
1. DeepSpeed Optimizers and Schedulers. For more information on these,
see the [DeepSpeed Optimizers](https://deepspeed.readthedocs.io/en/latest/optimizers.html) and [DeepSpeed Schedulers](https://deepspeed.readthedocs.io/en/latest/schedulers.html) documentation.
We will look at the changes needed in the code when using these.
a. DS Optim + DS Scheduler: The case when both `optimizer` and `scheduler` keys are present in the DeepSpeed config file.
In this situation, those will be used and the user has to use `accelerate.utils.DummyOptim` and `accelerate.utils.DummyScheduler` to replace the PyTorch/Custom optimizers and schedulers in their code.
Below is the snippet from `examples/by_feature/deepspeed_with_config_support.py` showing this:
```python
# Creates Dummy Optimizer if `optimizer` was specified in the config file else creates Adam Optimizer
optimizer_cls = (
torch.optim.AdamW
if accelerator.state.deepspeed_plugin is None
or "optimizer" not in accelerator.state.deepspeed_plugin.deepspeed_config
else DummyOptim
)
optimizer = optimizer_cls(optimizer_grouped_parameters, lr=args.learning_rate)
# Creates Dummy Scheduler if `scheduler` was specified in the config file else creates `args.lr_scheduler_type` Scheduler
if (
accelerator.state.deepspeed_plugin is None
or "scheduler" not in accelerator.state.deepspeed_plugin.deepspeed_config
):
lr_scheduler = get_scheduler(
name=args.lr_scheduler_type,
optimizer=optimizer,
num_warmup_steps=args.num_warmup_steps,
num_training_steps=args.max_train_steps,
)
else:
lr_scheduler = DummyScheduler(
optimizer, total_num_steps=args.max_train_steps, warmup_num_steps=args.num_warmup_steps
)
```
b. Custom Optim + Custom Scheduler: The case when both `optimizer` and `scheduler` keys are absent in the DeepSpeed config file.
In this situation, no code changes are needed from the user and this is the case when using integration via DeepSpeed Plugin.
In the above example we can see that the code remains unchanged if the `optimizer` and `scheduler` keys are absent in the DeepSpeed config file.
c. Custom Optim + DS Scheduler: The case when only `scheduler` key is present in the DeepSpeed config file.
In this situation, the user has to use `accelerate.utils.DummyScheduler` to replace the PyTorch/Custom scheduler in their code.
d. DS Optim + Custom Scheduler: The case when only `optimizer` key is present in the DeepSpeed config file.
This will result in an error because you can only use DS Scheduler when using DS Optim.
2. Notice the `auto` values in the above example DeepSpeed config files. These are automatically handled by `prepare` method
based on model, dataloaders, dummy optimizer and dummy schedulers provided to `prepare` method.
Only the `auto` fields specified in above examples are handled by `prepare` method and the rest have to be explicitly specified by the user.
The `auto` values are calculated as:
- `reduce_bucket_size`: `hidden_size * hidden_size`
- `stage3_prefetch_bucket_size`: `int(0.9 * hidden_size * hidden_size)`
- `stage3_param_persistence_threshold`: `10 * hidden_size`
For the `auto` feature to work for these 3 config entries - Accelerate will use `model.config.hidden_size` or `max(model.config.hidden_sizes)` as `hidden_size`. If neither of these is available, the launching will fail and you will have to set these 3 config entries manually. Remember the first 2 config entries are the communication buffers - the larger they are the more efficient the comms will be, and the larger they are the more GPU memory they will consume, so it's a tunable performance trade-off.
**Things to note when using DeepSpeed Config File**
Below is a sample script using `deepspeed_config_file` in different scenarios.
Code `test.py`:
```python
from accelerate import Accelerator
from accelerate.state import AcceleratorState
def main():
accelerator = Accelerator()
accelerator.print(f"{AcceleratorState()}")
if __name__ == "__main__":
main()
```
**Scenario 1**: Manually tampered accelerate config file having `deepspeed_config_file` along with other entries.
1. Content of the `accelerate` config:
```yaml
command_file: null
commands: null
compute_environment: LOCAL_MACHINE
deepspeed_config:
gradient_accumulation_steps: 1
gradient_clipping: 1.0
offload_optimizer_device: 'cpu'
offload_param_device: 'cpu'
zero3_init_flag: true
zero3_save_16bit_model: true
zero_stage: 3
deepspeed_config_file: 'ds_config.json'
distributed_type: DEEPSPEED
downcast_bf16: 'no'
dynamo_backend: 'NO'
fsdp_config: {}
gpu_ids: null
machine_rank: 0
main_process_ip: null
main_process_port: null
main_training_function: main
megatron_lm_config: {}
num_machines: 1
num_processes: 2
rdzv_backend: static
same_network: true
tpu_name: null
tpu_zone: null
use_cpu: false
```
2. `ds_config.json`:
```json
{
"bf16": {
"enabled": true
},
"zero_optimization": {
"stage": 3,
"stage3_gather_16bit_weights_on_model_save": false,
"offload_optimizer": {
"device": "none"
},
"offload_param": {
"device": "none"
}
},
"gradient_clipping": 1.0,
"train_batch_size": "auto",
"train_micro_batch_size_per_gpu": "auto",
"gradient_accumulation_steps": 10,
"steps_per_print": 2000000
}
```
3. Output of `accelerate launch test.py`:
```bash
ValueError: When using `deepspeed_config_file`, the following accelerate config variables will be ignored:
['gradient_accumulation_steps', 'gradient_clipping', 'zero_stage', 'offload_optimizer_device', 'offload_param_device',
'zero3_save_16bit_model', 'mixed_precision'].
Please specify them appropriately in the DeepSpeed config file.
If you are using an accelerate config file, remove other config variables mentioned in the above specified list.
The easiest method is to create a new config following the questionnaire via `accelerate config`.
It will only ask for the necessary config variables when using `deepspeed_config_file`.
```
**Scenario 2**: Use the solution of the error to create new accelerate config and check that no ambiguity error is now thrown.
1. Run `accelerate config`:
```bash
$ accelerate config
-------------------------------------------------------------------------------------------------------------------------------
In which compute environment are you running?
This machine
-------------------------------------------------------------------------------------------------------------------------------
Which type of machine are you using?
multi-GPU
How many different machines will you use (use more than 1 for multi-node training)? [1]:
Do you wish to optimize your script with torch dynamo?[yes/NO]:
Do you want to use DeepSpeed? [yes/NO]: yes
Do you want to specify a json file to a DeepSpeed config? [yes/NO]: yes
Please enter the path to the json DeepSpeed config file: ds_config.json
Do you want to enable `deepspeed.zero.Init` when using ZeRO Stage-3 for constructing massive models? [yes/NO]: yes
How many GPU(s) should be used for distributed training? [1]:4
accelerate configuration saved at ds_config_sample.yaml
```
2. Content of the `accelerate` config:
```yaml
compute_environment: LOCAL_MACHINE
deepspeed_config:
deepspeed_config_file: ds_config.json
zero3_init_flag: true
distributed_type: DEEPSPEED
downcast_bf16: 'no'
dynamo_backend: 'NO'
fsdp_config: {}
machine_rank: 0
main_training_function: main
megatron_lm_config: {}
num_machines: 1
num_processes: 4
rdzv_backend: static
same_network: true
use_cpu: false
```
3. Output of `accelerate launch test.py`:
```bash
Distributed environment: DEEPSPEED Backend: nccl
Num processes: 4
Process index: 0
Local process index: 0
Device: cuda:0
Mixed precision type: bf16
ds_config: {'bf16': {'enabled': True}, 'zero_optimization': {'stage': 3, 'stage3_gather_16bit_weights_on_model_save': False, 'offload_optimizer': {'device': 'none'}, 'offload_param': {'device': 'none'}}, 'gradient_clipping': 1.0, 'train_batch_size': 'auto', 'train_micro_batch_size_per_gpu': 'auto', 'gradient_accumulation_steps': 10, 'steps_per_print': inf, 'fp16': {'enabled': False}}
```
**Scenario 3**: Setting the `accelerate launch` command arguments related to DeepSpeed as `"auto"` in the DeepSpeed` configuration file and check that things work as expected.
1. New `ds_config.json` with `"auto"` for the `accelerate launch` DeepSpeed command arguments:
```json
{
"bf16": {
"enabled": "auto"
},
"zero_optimization": {
"stage": "auto",
"stage3_gather_16bit_weights_on_model_save": "auto",
"offload_optimizer": {
"device": "auto"
},
"offload_param": {
"device": "auto"
}
},
"gradient_clipping": "auto",
"train_batch_size": "auto",
"train_micro_batch_size_per_gpu": "auto",
"gradient_accumulation_steps": "auto",
"steps_per_print": 2000000
}
```
2. Output of `accelerate launch --mixed_precision="fp16" --zero_stage=3 --gradient_accumulation_steps=5 --gradient_clipping=1.0 --offload_param_device="cpu" --offload_optimizer_device="nvme" --zero3_save_16bit_model="true" test.py`:
```bash
Distributed environment: DEEPSPEED Backend: nccl
Num processes: 4
Process index: 0
Local process index: 0
Device: cuda:0
Mixed precision type: fp16
ds_config: {'bf16': {'enabled': False}, 'zero_optimization': {'stage': 3, 'stage3_gather_16bit_weights_on_model_save': True, 'offload_optimizer': {'device': 'nvme'}, 'offload_param': {'device': 'cpu'}}, 'gradient_clipping': 1.0, 'train_batch_size': 'auto', 'train_micro_batch_size_per_gpu': 'auto', 'gradient_accumulation_steps': 5, 'steps_per_print': inf, 'fp16': {'enabled': True, 'auto_cast': True}}
```
**Note**:
1. Remaining `"auto"` values are handled in `accelerator.prepare()` call as explained in point 2 of
`Important code changes when using DeepSpeed Config File`.
2. Only when `gradient_accumulation_steps` is `auto`, the value passed while creating `Accelerator` object via `Accelerator(gradient_accumulation_steps=k)` will be used. When using DeepSpeed Plugin, the value from it will be used and it will overwrite the value passed while creating Accelerator object.
## Saving and loading
1. Saving and loading of models is unchanged for ZeRO Stage-1 and Stage-2.
2. under ZeRO Stage-3, `state_dict` contains just the placeholders since the model weights are partitioned across multiple GPUs.
ZeRO Stage-3 has 2 options:
a. Saving the entire 16bit model weights to directly load later on using `model.load_state_dict(torch.load(pytorch_model.bin))`.
For this, either set `zero_optimization.stage3_gather_16bit_weights_on_model_save` to True in DeepSpeed Config file or set
`zero3_save_16bit_model` to True in DeepSpeed Plugin.
**Note that this option requires consolidation of the weights on one GPU it can be slow and memory demanding, so only use this feature when needed.**
Below is the snippet from `examples/by_feature/deepspeed_with_config_support.py` showing this:
```python
unwrapped_model = accelerator.unwrap_model(model)
# New Code #
# Saves the whole/unpartitioned fp16 model when in ZeRO Stage-3 to the output directory if
# `stage3_gather_16bit_weights_on_model_save` is True in DeepSpeed Config file or
# `zero3_save_16bit_model` is True in DeepSpeed Plugin.
# For Zero Stages 1 and 2, models are saved as usual in the output directory.
# The model name saved is `pytorch_model.bin`
unwrapped_model.save_pretrained(
args.output_dir,
is_main_process=accelerator.is_main_process,
save_function=accelerator.save,
state_dict=accelerator.get_state_dict(model),
)
```
b. To get 32bit weights, first save the model using `model.save_checkpoint()`.
Below is the snippet from `examples/by_feature/deepspeed_with_config_support.py` showing this:
```python
success = model.save_checkpoint(PATH, ckpt_id, checkpoint_state_dict)
status_msg = f"checkpointing: PATH={PATH}, ckpt_id={ckpt_id}"
if success:
logging.info(f"Success {status_msg}")
else:
logging.warning(f"Failure {status_msg}")
```
This will create ZeRO model and optimizer partitions along with `zero_to_fp32.py` script in checkpoint directory.
You can use this script to do offline consolidation.
It requires no configuration files or GPUs. Here is an example of its usage:
```bash
$ cd /path/to/checkpoint_dir
$ ./zero_to_fp32.py . pytorch_model.bin
Processing zero checkpoint at global_step1
Detected checkpoint of type zero stage 3, world_size: 2
Saving fp32 state dict to pytorch_model.bin (total_numel=60506624)
```
To get 32bit model for saving/inference, you can perform:
```python
from deepspeed.utils.zero_to_fp32 import load_state_dict_from_zero_checkpoint
unwrapped_model = accelerator.unwrap_model(model)
fp32_model = load_state_dict_from_zero_checkpoint(unwrapped_model, checkpoint_dir)
```
If you are only interested in the `state_dict`, you can do the following:
```python
from deepspeed.utils.zero_to_fp32 import get_fp32_state_dict_from_zero_checkpoint
state_dict = get_fp32_state_dict_from_zero_checkpoint(checkpoint_dir)
```
Note that all these functions require ~2x memory (general RAM) of the size of the final checkpoint.
## ZeRO Inference
DeepSpeed ZeRO Inference supports ZeRO stage 3 with ZeRO-Infinity.
It uses the same ZeRO protocol as training, but it doesn't use an optimizer and a lr scheduler and only stage 3 is relevant.
With accelerate integration, you just need to prepare the model and dataloader as shown below:
```python
model, eval_dataloader = accelerator.prepare(model, eval_dataloader)
```
## Few caveats to be aware of
1. Current integration doesn’t support Pipeline Parallelism of DeepSpeed.
2. Current integration doesn’t support `mpu`, limiting the tensor parallelism which is supported in Megatron-LM.
3. Current integration doesn’t support multiple models.
## DeepSpeed Resources
The documentation for the internals related to deepspeed can be found [here](../package_reference/deepspeed).
- [Project's github](https://github.com/microsoft/deepspeed)
- [Usage docs](https://www.deepspeed.ai/getting-started/)
- [API docs](https://deepspeed.readthedocs.io/en/latest/index.html)
- [Blog posts](https://www.microsoft.com/en-us/research/search/?q=deepspeed)
Papers:
- [ZeRO: Memory Optimizations Toward Training Trillion Parameter Models](https://arxiv.org/abs/1910.02054)
- [ZeRO-Offload: Democratizing Billion-Scale Model Training](https://arxiv.org/abs/2101.06840)
- [ZeRO-Infinity: Breaking the GPU Memory Wall for Extreme Scale Deep Learning](https://arxiv.org/abs/2104.07857)
- [ZeRO++: Extremely Efficient Collective Communication for Giant Model Training](https://arxiv.org/abs/2306.10209)
Finally, please, remember that `Accelerate` only integrates DeepSpeed, therefore if you
have any problems or questions with regards to DeepSpeed usage, please, file an issue with [DeepSpeed GitHub](https://github.com/microsoft/DeepSpeed/issues).
For those interested in the similarities and differences between FSDP and DeepSpeed, please check out the [concept guide here](../concept_guides/fsdp_and_deepspeed)!
# Distributed inference
Distributed inference can fall into three brackets:
1. Loading an entire model onto each GPU and sending chunks of a batch through each GPU's model copy at a time
2. Loading parts of a model onto each GPU and processing a single input at one time
3. Loading parts of a model onto each GPU and using what is called scheduled Pipeline Parallelism to combine the two prior techniques.
We're going to go through the first and the last bracket, showcasing how to do each as they are more realistic scenarios.
## Sending chunks of a batch automatically to each loaded model
This is the most memory-intensive solution, as it requires each GPU to keep a full copy of the model in memory at a given time.
Normally when doing this, users send the model to a specific device to load it from the CPU, and then move each prompt to a different device.
A basic pipeline using the `diffusers` library might look something like so:
```python
import torch
import torch.distributed as dist
from diffusers import DiffusionPipeline
pipe = DiffusionPipeline.from_pretrained("runwayml/stable-diffusion-v1-5", torch_dtype=torch.float16)
```
Followed then by performing inference based on the specific prompt:
```python
def run_inference(rank, world_size):
dist.init_process_group("nccl", rank=rank, world_size=world_size)
pipe.to(rank)
if torch.distributed.get_rank() == 0:
prompt = "a dog"
elif torch.distributed.get_rank() == 1:
prompt = "a cat"
result = pipe(prompt).images[0]
result.save(f"result_{rank}.png")
```
One will notice how we have to check the rank to know what prompt to send, which can be a bit tedious.
A user might then also think that with Accelerate, using the `Accelerator` to prepare a dataloader for such a task might also be
a simple way to manage this. (To learn more, check out the relevant section in the [Quick Tour](../quicktour#distributed-evaluation))
Can it manage it? Yes. Does it add unneeded extra code however: also yes.
With Accelerate, we can simplify this process by using the `Accelerator.split_between_processes()` context manager (which also exists in `PartialState` and `AcceleratorState`).
This function will automatically split whatever data you pass to it (be it a prompt, a set of tensors, a dictionary of the prior data, etc.) across all the processes (with a potential
to be padded) for you to use right away.
Let's rewrite the above example using this context manager:
```python
from accelerate import PartialState # Can also be Accelerator or AcceleratorState
from diffusers import DiffusionPipeline
pipe = DiffusionPipeline.from_pretrained("runwayml/stable-diffusion-v1-5", torch_dtype=torch.float16)
distributed_state = PartialState()
pipe.to(distributed_state.device)
# Assume two processes
with distributed_state.split_between_processes(["a dog", "a cat"]) as prompt:
result = pipe(prompt).images[0]
result.save(f"result_{distributed_state.process_index}.png")
```
And then to launch the code, we can use the Accelerate:
If you have generated a config file to be used using `accelerate config`:
```bash
accelerate launch distributed_inference.py
```
If you have a specific config file you want to use:
```bash
accelerate launch --config_file my_config.json distributed_inference.py
```
Or if don't want to make any config files and launch on two GPUs:
> Note: You will get some warnings about values being guessed based on your system. To remove these you can do `accelerate config default` or go through `accelerate config` to create a config file.
```bash
accelerate launch --num_processes 2 distributed_inference.py
```
We've now reduced the boilerplate code needed to split this data to a few lines of code quite easily.
But what if we have an odd distribution of prompts to GPUs? For example, what if we have 3 prompts, but only 2 GPUs?
Under the context manager, the first GPU would receive the first two prompts and the second GPU the third, ensuring that
all prompts are split and no overhead is needed.
*However*, what if we then wanted to do something with the results of *all the GPUs*? (Say gather them all and perform some kind of post processing)
You can pass in `apply_padding=True` to ensure that the lists of prompts are padded to the same length, with extra data being taken
from the last sample. This way all GPUs will have the same number of prompts, and you can then gather the results.
This is only needed when trying to perform an action such as gathering the results, where the data on each device
needs to be the same length. Basic inference does not require this.
For instance:
```python
from accelerate import PartialState # Can also be Accelerator or AcceleratorState
from diffusers import DiffusionPipeline
pipe = DiffusionPipeline.from_pretrained("runwayml/stable-diffusion-v1-5", torch_dtype=torch.float16)
distributed_state = PartialState()
pipe.to(distributed_state.device)
# Assume two processes
with distributed_state.split_between_processes(["a dog", "a cat", "a chicken"], apply_padding=True) as prompt:
result = pipe(prompt).images
```
On the first GPU, the prompts will be `["a dog", "a cat"]`, and on the second GPU it will be `["a chicken", "a chicken"]`.
Make sure to drop the final sample, as it will be a duplicate of the previous one.
You can find more complex examples [here](https://github.com/huggingface/accelerate/tree/main/examples/inference/distributed) such as how to use it with LLMs.
## Memory-efficient pipeline parallelism (experimental)
This next part will discuss using *pipeline parallelism*. This is an **experimental** API that utilizes [torch.distributed.pipelining](https://pytorch.org/docs/stable/distributed.pipelining.html#) as a native solution.
The general idea with pipeline parallelism is: say you have 4 GPUs and a model big enough it can be *split* on four GPUs using `device_map="auto"`. With this method you can send in 4 inputs at a time (for example here, any amount works) and each model chunk will work on an input, then receive the next input once the prior chunk finished, making it *much* more efficient **and faster** than the method described earlier. Here's a visual taken from the PyTorch repository:
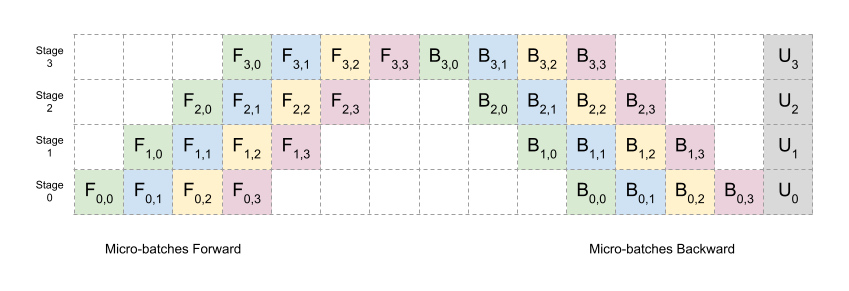
To illustrate how you can use this with Accelerate, we have created an [example zoo](https://github.com/huggingface/accelerate/tree/main/examples/inference) showcasing a number of different models and situations. In this tutorial, we'll show this method for GPT2 across two GPUs.
Before you proceed, please make sure you have the latest PyTorch version installed by running the following:
```bash
pip install torch
```
Start by creating the model on the CPU:
```{python}
from transformers import GPT2ForSequenceClassification, GPT2Config
config = GPT2Config()
model = GPT2ForSequenceClassification(config)
model.eval()
```
Next you'll need to create some example inputs to use. These help `torch.distributed.pipelining` trace the model.
However you make this example will determine the relative batch size that will be used/passed
through the model at a given time, so make sure to remember how many items there are!
```{python}
input = torch.randint(
low=0,
high=config.vocab_size,
size=(2, 1024), # bs x seq_len
device="cpu",
dtype=torch.int64,
requires_grad=False,
)
```
Next we need to actually perform the tracing and get the model ready. To do so, use the `inference.prepare_pippy()` function and it will fully wrap the model for pipeline parallelism automatically:
```{python}
from accelerate.inference import prepare_pippy
example_inputs = {"input_ids": input}
model = prepare_pippy(model, example_args=(input,))
```
There are a variety of parameters you can pass through to `prepare_pippy`:
* `split_points` lets you determine what layers to split the model at. By default we use wherever `device_map="auto" declares, such as `fc` or `conv1`.
* `num_chunks` determines how the batch will be split and sent to the model itself (so `num_chunks=1` with four split points/four GPUs will have a naive MP where a single input gets passed between the four layer split points)
From here, all that's left is to actually perform the distributed inference!
When passing inputs, we highly recommend to pass them in as a tuple of arguments. Using `kwargs` is supported, however, this approach is experimental.
```{python}
args = some_more_arguments
with torch.no_grad():
output = model(*args)
```
When finished all the data will be on the last process only:
```{python}
from accelerate import PartialState
if PartialState().is_last_process:
print(output)
```
If you pass in `gather_output=True` to `inference.prepare_pippy()`, the output will be sent
across to all the GPUs afterwards without needing the `is_last_process` check. This is
`False` by default as it incurs a communication call.
And that's it! To explore more, please check out the inference examples in the [Accelerate repo](https://github.com/huggingface/accelerate/tree/main/examples/inference/pippy) and our [documentation](../package_reference/inference) as we work to improving this integration.
# Start Here!
Please use the interactive tool below to help you get started with learning about a particular
feature of Accelerate and how to utilize it! It will provide you with a code diff, an explanation
towards what is going on, as well as provide you with some useful links to explore more within
the documentation!
Most code examples start from the following python code before integrating Accelerate in some way:
```python
for batch in dataloader:
optimizer.zero_grad()
inputs, targets = batch
inputs = inputs.to(device)
targets = targets.to(device)
outputs = model(inputs)
loss = loss_function(outputs, targets)
loss.backward()
optimizer.step()
scheduler.step()
```
# Big Model Inference
One of the biggest advancements Accelerate provides is [Big Model Inference](../concept_guides/big_model_inference), which allows you to perform inference with models that don't fully fit on your graphics card.
This tutorial will show you how to use Big Model Inference in Accelerate and the Hugging Face ecosystem.
## Accelerate
A typical workflow for loading a PyTorch model is shown below. `ModelClass` is a model that exceeds the GPU memory of your device (mps or cuda).
```py
import torch
my_model = ModelClass(...)
state_dict = torch.load(checkpoint_file)
my_model.load_state_dict(state_dict)
```
With Big Model Inference, the first step is to init an empty skeleton of the model with the `init_empty_weights` context manager. This doesn't require any memory because `my_model` is "parameterless".
```py
from accelerate import init_empty_weights
with init_empty_weights():
my_model = ModelClass(...)
```
Next, the weights are loaded into the model for inference.
The `load_checkpoint_and_dispatch()` method loads a checkpoint inside your empty model and dispatches the weights for each layer across all available devices, starting with the fastest devices (GPU, MPS, XPU, NPU, MLU, MUSA) first before moving to the slower ones (CPU and hard drive).
Setting `device_map="auto"` automatically fills all available space on the GPU(s) first, then the CPU, and finally, the hard drive (the absolute slowest option) if there is still not enough memory.
> [!TIP]
> Refer to the [Designing a device map](../concept_guides/big_model_inference#designing-a-device-map) guide for more details on how to design your own device map.
```py
from accelerate import load_checkpoint_and_dispatch
model = load_checkpoint_and_dispatch(
model, checkpoint=checkpoint_file, device_map="auto"
)
```
If there are certain “chunks” of layers that shouldn’t be split, pass them to `no_split_module_classes` (see [here](../concept_guides/big_model_inference#loading-weights) for more details).
A models weights can also be sharded into multiple checkpoints to save memory, such as when the `state_dict` doesn't fit in memory (see [here](../concept_guides/big_model_inference#sharded-checkpoints) for more details).
Now that the model is fully dispatched, you can perform inference.
```py
input = torch.randn(2,3)
input = input.to("cuda")
output = model(input)
```
Each time an input is passed through a layer, it is sent from the CPU to the GPU (or disk to CPU to GPU), the output is calculated, and the layer is removed from the GPU going back down the line. While this adds some overhead to inference, it enables you to run any size model on your system, as long as the largest layer fits on your GPU.
Multiple GPUs, or "model parallelism", can be utilized but only one GPU will be active at any given moment. This forces the GPU to wait for the previous GPU to send it the output. You should launch your script normally with Python instead of other tools like torchrun and accelerate launch.
> [!TIP]
> You may also be interested in *pipeline parallelism* which utilizes all available GPUs at once, instead of only having one GPU active at a time. This approach is less flexbile though. For more details, refer to the [Memory-efficient pipeline parallelism](./distributed_inference#memory-efficient-pipeline-parallelism-experimental) guide.
Take a look at a full example of Big Model Inference below.
```py
import torch
from accelerate import init_empty_weights, load_checkpoint_and_dispatch
with init_empty_weights():
model = MyModel(...)
model = load_checkpoint_and_dispatch(
model, checkpoint=checkpoint_file, device_map="auto"
)
input = torch.randn(2,3)
input = input.to("cuda")
output = model(input)
```
## Hugging Face ecosystem
Other libraries in the Hugging Face ecosystem, like Transformers or Diffusers, supports Big Model Inference in their [from_pretrained](https://huggingface.co/docs/transformers/main/en/main_classes/model#transformers.PreTrainedModel.from_pretrained) constructors.
You just need to add `device_map="auto"` in [from_pretrained](https://huggingface.co/docs/transformers/main/en/main_classes/model#transformers.PreTrainedModel.from_pretrained) to enable Big Model Inference.
For example, load Big Sciences T0pp 11 billion parameter model with Big Model Inference.
```py
from transformers import AutoModelForSeq2SeqLM
model = AutoModelForSeq2SeqLM.from_pretrained("bigscience/T0pp", device_map="auto")
```
After loading the model, the empty init and smart dispatch steps from before are executed and the model is fully ready to make use of all the resources in your machine. Through these constructors, you can also save more memory by specifying the `torch_dtype` parameter to load a model in a lower precision.
```py
from transformers import AutoModelForSeq2SeqLM
model = AutoModelForSeq2SeqLM.from_pretrained("bigscience/T0pp", device_map="auto", torch_dtype=torch.float16)
```
## Next steps
For a more detailed explanation of Big Model Inference, make sure to check out the [conceptual guide](../concept_guides/big_model_inference)!
# Using multiple models with DeepSpeed
This guide assumes that you have read and understood the [DeepSpeed usage guide](./deepspeed.md).
Running multiple models with Accelerate and DeepSpeed is useful for:
* Knowledge distillation
* Post-training techniques like RLHF (see the [TRL](https://github.com/huggingface/trl) library for more examples)
* Training multiple models at once
Currently, Accelerate has a **very experimental API** to help you use multiple models.
This tutorial will focus on two common use cases:
1. Knowledge distillation, where a smaller student model is trained to mimic a larger, better-performing teacher. If the student model fits on a single GPU, we can use ZeRO-2 for training and ZeRO-3 to shard the teacher for inference. This is significantly faster than using ZeRO-3 for both models.
2. Training multiple *disjoint* models at once.
## Knowledge distillation
Knowledge distillation is a good example of using multiple models, but only training one of them.
Normally, you would use a single `utils.DeepSpeedPlugin` for both models. However, in this case, there are two separate configurations. Accelerate allows you to create and use multiple plugins **if and only if** they are in a `dict` so that you can reference and enable the proper plugin when needed.
```python
from accelerate.utils import DeepSpeedPlugin
zero2_plugin = DeepSpeedPlugin(hf_ds_config="zero2_config.json")
zero3_plugin = DeepSpeedPlugin(hf_ds_config="zero3_config.json")
deepspeed_plugins = {"student": zero2_plugin, "teacher": zero3_plugin}
```
The `zero2_config.json` should be configured for full training (so specify `scheduler` and `optimizer` if you are not utilizing your own), while `zero3_config.json` should only be configured for the inference model, as shown in the example below.
```json
{
"bf16": {
"enabled": "auto"
},
"zero_optimization": {
"stage": 3,
"overlap_comm": true,
"reduce_bucket_size": "auto",
"stage3_prefetch_bucket_size": "auto",
"stage3_param_persistence_threshold": "auto",
"stage3_max_live_parameters": "auto",
"stage3_max_reuse_distance": "auto",
},
"train_micro_batch_size_per_gpu": 1
}
```
An example `zero2_config.json` configuration is shown below.
```json
{
"bf16": {
"enabled": "auto"
},
"optimizer": {
"type": "AdamW",
"params": {
"lr": "auto",
"weight_decay": "auto",
"torch_adam": true,
"adam_w_mode": true
}
},
"scheduler": {
"type": "WarmupLR",
"params": {
"warmup_min_lr": "auto",
"warmup_max_lr": "auto",
"warmup_num_steps": "auto"
}
},
"zero_optimization": {
"stage": 2,
"offload_optimizer": {
"device": "cpu",
"pin_memory": true
},
},
"gradient_accumulation_steps": 1,
"gradient_clipping": "auto",
"train_batch_size": "auto",
"train_micro_batch_size_per_gpu": "auto",
}
```
DeepSpeed will raise an error if `train_micro_batch_size_per_gpu` isn't specified, even if this particular model isn't being trained.
From here, create a single `Accelerator` and pass in both configurations.
```python
from accelerate import Accelerator
accelerator = Accelerator(deepspeed_plugins=deepspeed_plugins)
```
Now let's see how to use them.
### Student model
By default, Accelerate sets the first item in the `dict` as the default or enabled plugin (`"student"` plugin). Verify this by using the `utils.deepspeed.get_active_deepspeed_plugin()` function to see which plugin is enabled.
```python
active_plugin = get_active_deepspeed_plugin(accelerator.state)
assert active_plugin is deepspeed_plugins["student"]
```
`AcceleratorState` also keeps the active DeepSpeed plugin saved in `state.deepspeed_plugin`.
```python
assert active_plugin is accelerator.deepspeed_plugin
```
Since `student` is the currently active plugin, let's go ahead and prepare the model, optimizer, and scheduler.
```python
student_model, optimizer, scheduler = ...
student_model, optimizer, scheduler, train_dataloader = accelerator.prepare(student_model, optimizer, scheduler, train_dataloader)
```
Now it's time to deal with the teacher model.
### Teacher model
First, you need to specify in `Accelerator` that the `zero3_config.json` configuration should be used.
```python
accelerator.state.select_deepspeed_plugin("teacher")
```
This disables the `"student"` plugin and enables the `"teacher"` plugin instead. The
DeepSpeed stateful config inside of Transformers is updated, and it changes which plugin configuration gets called when using
`deepspeed.initialize()`. This allows you to use the automatic `deepspeed.zero.Init` context manager integration Transformers provides.
```python
teacher_model = AutoModel.from_pretrained(...)
teacher_model = accelerator.prepare(teacher_model)
```
Otherwise, you should manually initialize the model with `deepspeed.zero.Init`.
```python
with deepspeed.zero.Init(accelerator.deepspeed_plugin.config):
model = MyModel(...)
```
### Training
From here, your training loop can be whatever you like, as long as `teacher_model` is never being trained on.
```python
teacher_model.eval()
student_model.train()
for batch in train_dataloader:
with torch.no_grad():
output_teacher = teacher_model(**batch)
output_student = student_model(**batch)
# Combine the losses or modify it in some way
loss = output_teacher.loss + output_student.loss
accelerator.backward(loss)
optimizer.step()
scheduler.step()
optimizer.zero_grad()
```
## Train multiple disjoint models
Training multiple models is a more complicated scenario.
In its current state, we assume each model is **completely disjointed** from the other during training.
This scenario still requires two `utils.DeepSpeedPlugin`'s to be made. However, you also need a second `Accelerator`, since different `deepspeed` engines are being called at different times. A single `Accelerator` can only carry one instance at a time.
Since the `state.AcceleratorState` is a stateful object though, it is already aware of both `utils.DeepSpeedPlugin`'s available. You can just instantiate a second `Accelerator` with no extra arguments.
```python
first_accelerator = Accelerator(deepspeed_plugins=deepspeed_plugins)
second_accelerator = Accelerator()
```
You can call either `first_accelerator.state.select_deepspeed_plugin()` to enable or disable
a particular plugin, and then call `prepare`.
```python
# can be `accelerator_0`, `accelerator_1`, or by calling `AcceleratorState().select_deepspeed_plugin(...)`
first_accelerator.state.select_deepspeed_plugin("first_model")
first_model = AutoModel.from_pretrained(...)
# For this example, `get_training_items` is a nonexistent function that gets the setup we need for training
first_optimizer, first_scheduler, train_dl, eval_dl = get_training_items(model1)
first_model, first_optimizer, first_scheduler, train_dl, eval_dl = accelerator.prepare(
first_model, first_optimizer, first_scheduler, train_dl, eval_dl
)
second_accelerator.state.select_deepspeed_plugin("second_model")
second_model = AutoModel.from_pretrained(...)
# For this example, `get_training_items` is a nonexistent function that gets the setup we need for training
second_optimizer, second_scheduler, _, _ = get_training_items(model2)
second_model, second_optimizer, second_scheduler = accelerator.prepare(
second_model, second_optimizer, second_scheduler
)
```
And now you can train:
```python
for batch in dl:
outputs1 = first_model(**batch)
first_accelerator.backward(outputs1.loss)
first_optimizer.step()
first_scheduler.step()
first_optimizer.zero_grad()
outputs2 = model2(**batch)
second_accelerator.backward(outputs2.loss)
second_optimizer.step()
second_scheduler.step()
second_optimizer.zero_grad()
```
## Resources
To see more examples, please check out the [related tests](https://github.com/huggingface/accelerate/blob/main/src/accelerate/test_utils/scripts/external_deps/test_ds_multiple_model.py) currently in [Accelerate].
# Profiler
Profiler is a tool that allows the collection of performance metrics during training and inference. Profiler’s context manager API can be used to better understand what model operators are the most expensive, examine their input shapes and stack traces, study device kernel activity, and visualize the execution trace. It provides insights into the performance of your model, allowing you to optimize and improve it.
This guide explains how to use PyTorch Profiler to measure the time and memory consumption of the model’s operators and how to integrate this with Accelerate. We will cover various use cases and provide examples for each.
## Using profiler to analyze execution time
Profiler allows one to check which operators were called during the execution of a code range wrapped with a profiler context manager.
Let’s see how we can use profiler to analyze the execution time:
```python
import torch
import torchvision.models as models
from torch.profiler import profile, record_function, ProfilerActivity
model = models.resnet18()
inputs = torch.randn(5, 3, 224, 224)
with profile(activities=[ProfilerActivity.CPU], record_shapes=True) as prof:
model(inputs)
print(prof.key_averages().table(sort_by="cpu_time_total", row_limit=10))
```
```python
from accelerate import Accelerator, ProfileKwargs
import torch
import torchvision.models as models
model = models.resnet18()
inputs = torch.randn(5, 3, 224, 224)
profile_kwargs = ProfileKwargs(
activities=["cpu"],
record_shapes=True
)
accelerator = Accelerator(cpu=True, kwargs_handlers=[profile_kwargs])
model = accelerator.prepare(model)
with accelerator.profile() as prof:
with torch.no_grad():
model(inputs)
print(prof.key_averages().table(sort_by="cpu_time_total", row_limit=10))
```
The resulting table output (omitting some columns):
```
--------------------------------- ------------ ------------ ------------ ------------
Name Self CPU CPU total CPU time avg # of Calls
--------------------------------- ------------ ------------ ------------ ------------
aten::conv2d 171.000us 52.260ms 2.613ms 20
aten::convolution 227.000us 52.089ms 2.604ms 20
aten::_convolution 270.000us 51.862ms 2.593ms 20
aten::mkldnn_convolution 51.273ms 51.592ms 2.580ms 20
aten::batch_norm 118.000us 7.059ms 352.950us 20
aten::_batch_norm_impl_index 315.000us 6.941ms 347.050us 20
aten::native_batch_norm 6.305ms 6.599ms 329.950us 20
aten::max_pool2d 40.000us 4.008ms 4.008ms 1
aten::max_pool2d_with_indices 3.968ms 3.968ms 3.968ms 1
aten::add_ 780.000us 780.000us 27.857us 28
--------------------------------- ------------ ------------ ------------ ------------
Self CPU time total: 67.016ms
```
To get a finer granularity of results and include operator input shapes, pass `group_by_input_shape=True` (note: this requires running the profiler with `record_shapes=True`):
```python
print(prof.key_averages(group_by_input_shape=True).table(sort_by="cpu_time_total", row_limit=10))
```
## Using profiler to analyze memory consumption
Profiler can also show the amount of memory (used by the model’s tensors) that was allocated (or released) during the execution of the model’s operators. To enable memory profiling functionality pass `profile_memory=True`.
```python
model = models.resnet18()
inputs = torch.randn(5, 3, 224, 224)
with profile(activities=[ProfilerActivity.CPU],
profile_memory=True, record_shapes=True) as prof:
model(inputs)
print(prof.key_averages().table(sort_by="self_cpu_memory_usage", row_limit=10))
```
```python
model = models.resnet18()
inputs = torch.randn(5, 3, 224, 224)
profile_kwargs = ProfileKwargs(
activities=["cpu"],
profile_memory=True,
record_shapes=True
)
accelerator = Accelerator(cpu=True, kwargs_handlers=[profile_kwargs])
model = accelerator.prepare(model)
with accelerator.profile() as prof:
model(inputs)
print(prof.key_averages().table(sort_by="self_cpu_memory_usage", row_limit=10))
```
The resulting table output (omitting some columns):
```
--------------------------------- ------------ ------------ ------------
Name CPU Mem Self CPU Mem # of Calls
--------------------------------- ------------ ------------ ------------
aten::empty 94.85 Mb 94.85 Mb 205
aten::max_pool2d_with_indices 11.48 Mb 11.48 Mb 1
aten::addmm 19.53 Kb 19.53 Kb 1
aten::mean 10.00 Kb 10.00 Kb 1
aten::empty_strided 492 b 492 b 5
aten::cat 240 b 240 b 6
aten::abs 480 b 240 b 4
aten::masked_select 120 b 112 b 1
aten::ne 61 b 53 b 3
aten::eq 30 b 30 b 1
--------------------------------- ------------ ------------ ------------
Self CPU time total: 69.332ms
```
## Exporting chrome trace
You can examine the sequence of profiled operators and CUDA kernels in Chrome trace viewer (`chrome://tracing`):

```python
model = models.resnet18().cuda()
inputs = torch.randn(5, 3, 224, 224).cuda()
with profile(activities=[ProfilerActivity.CPU, ProfilerActivity.CUDA]) as prof:
model(inputs)
prof.export_chrome_trace("trace.json")
```
```python
profile_kwargs = ProfileKwargs(
activities=["cpu", "cuda"],
output_trace_dir="trace"
)
accelerator = Accelerator(kwargs_handlers=[profile_kwargs])
model = accelerator.prepare(model)
with accelerator.profile() as prof:
model(inputs)
# The trace will be saved to the specified directory
```
## Using Profiler to Analyze Long-Running Jobs
Profiler offers an additional API to handle long-running jobs (such as training loops). Tracing all of the execution can be slow and result in very large trace files. To avoid this, use optional arguments:
- `schedule_option`: Scheduling options allow you to control when profiling is active. This is useful for long-running jobs to avoid collecting too much data. Available keys are `wait`, `warmup`, `active`, `repeat` and `skip_first`. The profiler will skip the first `skip_first` steps, then wait for `wait` steps, then do the warmup for the next `warmup` steps, then do the active recording for the next `active` steps and then repeat the cycle starting with `wait` steps. The optional number of cycles is specified with the `repeat` parameter, the zero value means that the cycles will continue until the profiling is finished.
- `on_trace_ready`: specifies a function that takes a reference to the profiler as an input and is called by the profiler each time the new trace is ready.
To illustrate how the API works, consider the following example:
```python
from torch.profiler import schedule
my_schedule = schedule(
skip_first=10,
wait=5,
warmup=1,
active=3,
repeat=2
)
def trace_handler(p):
output = p.key_averages().table(sort_by="self_cuda_time_total", row_limit=10)
print(output)
p.export_chrome_trace("/tmp/trace_" + str(p.step_num) + ".json")
with profile(
activities=[ProfilerActivity.CPU, ProfilerActivity.CUDA],
schedule=my_schedule,
on_trace_ready=trace_handler
) as p:
for idx in range(8):
model(inputs)
p.step()
```
```python
def trace_handler(p):
output = p.key_averages().table(sort_by="self_cuda_time_total", row_limit=10)
print(output)
p.export_chrome_trace("/tmp/trace_" + str(p.step_num) + ".json")
profile_kwargs = ProfileKwargs(
activities=["cpu", "cuda"],
schedule_option={"wait": 5, "warmup": 1, "active": 3, "repeat": 2, "skip_first": 10},
on_trace_ready=trace_handler
)
accelerator = Accelerator(kwargs_handlers=[profile_kwargs])
model = accelerator.prepare(model)
with accelerator.profile() as prof:
for idx in range(8):
model(inputs)
prof.step()
```
## FLOPS
Use formula to estimate the FLOPs (floating point operations) of specific operators (matrix multiplication and 2D convolution).
To measure floating-point operations (FLOPS):
```python
with profile(
activities=[ProfilerActivity.CPU, ProfilerActivity.CUDA],
with_flops=True
) as prof:
model(inputs)
print(prof.key_averages().table(sort_by="flops", row_limit=10))
```
```python
profile_kwargs = ProfileKwargs(
with_flops=True
)
accelerator = Accelerator(kwargs_handlers=[profile_kwargs])
with accelerator.profile() as prof:
model(inputs)
print(prof.key_averages().table(sort_by="flops", row_limit=10))
```
The resulting table output (omitting some columns):
```
------------------------------------------------------- ------------ ------------ ------------
Name Self CPU Self CUDA Total FLOPs
------------------------------------------------------- ------------ ------------ ------------
aten::conv2d 197.000us 0.000us 18135613440.000
aten::addmm 103.000us 17.000us 5120000.000
aten::mul 29.000us 2.000us 30.000
aten::convolution 409.000us 0.000us --
aten::_convolution 253.000us 0.000us --
aten::cudnn_convolution 5.465ms 2.970ms --
cudaEventRecord 138.000us 0.000us --
cudaStreamIsCapturing 43.000us 0.000us --
cudaStreamGetPriority 40.000us 0.000us --
cudaDeviceGetStreamPriorityRange 10.000us 0.000us --
------------------------------------------------------- ------------ ------------ ------------
Self CPU time total: 21.938ms
Self CUDA time total: 4.165ms
```
## Conclusion and Further Information
PyTorch Profiler is a powerful tool for analyzing the performance of your models. By integrating it with Accelerate, you can easily profile your models and gain insights into their performance, helping you to optimize and improve them.
For more detailed information, refer to the [PyTorch Profiler documentation](https://pytorch.org/docs/stable/profiler.html).
# Megatron-LM
[Megatron-LM](https://github.com/NVIDIA/Megatron-LM) enables training large transformer language models at scale.
It provides efficient tensor, pipeline and sequence based model parallelism for pre-training transformer based
Language Models such as [GPT](https://arxiv.org/abs/2005.14165) (Decoder Only), [BERT](https://arxiv.org/pdf/1810.04805.pdf) (Encoder Only) and [T5](https://arxiv.org/abs/1910.10683) (Encoder-Decoder).
For detailed information and how things work behind the scene please refer the github [repo](https://github.com/NVIDIA/Megatron-LM).
## What is integrated?
Accelerate integrates following feature of Megatron-LM to enable large scale pre-training/finetuning
of BERT (Encoder), GPT (Decoder) or T5 models (Encoder and Decoder):
a. **Tensor Parallelism (TP)**: Reduces memory footprint without much additional communication on intra-node ranks.
Each tensor is split into multiple chunks with each shard residing on separate GPU. At each step, the same mini-batch of data is processed
independently and in parallel by each shard followed by syncing across all GPUs (`all-reduce` operation).
In a simple transformer layer, this leads to 2 `all-reduces` in the forward path and 2 in the backward path.
For more details, please refer research paper [Megatron-LM: Training Multi-Billion Parameter Language Models Using
Model Parallelism](https://arxiv.org/pdf/1909.08053.pdf) and
this section of blogpost [The Technology Behind BLOOM Training](https://huggingface.co/blog/bloom-megatron-deepspeed#tensor-parallelism).
b. **Pipeline Parallelism (PP)**: Reduces memory footprint and enables large scale training via inter-node parallelization.
Reduces the bubble of naive PP via PipeDream-Flush schedule/1F1B schedule and Interleaved 1F1B schedule.
Layers are distributed uniformly across PP stages. For example, if a model has `24` layers and we have `4` GPUs for
pipeline parallelism, each GPU will have `6` layers (24/4). For more details on schedules to reduce the idle time of PP,
please refer to the research paper [Efficient Large-Scale Language Model Training on GPU Clusters
Using Megatron-LM](https://arxiv.org/pdf/2104.04473.pdf) and
this section of blogpost [The Technology Behind BLOOM Training](https://huggingface.co/blog/bloom-megatron-deepspeed#pipeline-parallelism).
c. **Sequence Parallelism (SP)**: Reduces memory footprint without any additional communication. Only applicable when using TP.
It reduces activation memory required as it prevents the same copies to be on the tensor parallel ranks
post `all-reduce` by replacing then with `reduce-scatter` and `no-op` operation would be replaced by `all-gather`.
As `all-reduce = reduce-scatter + all-gather`, this saves a ton of activation memory at no added communication cost.
To put it simply, it shards the outputs of each transformer layer along sequence dimension, e.g.,
if the sequence length is `1024` and the TP size is `4`, each GPU will have `256` tokens (1024/4) for each sample.
This increases the batch size that can be supported for training. For more details, please refer to the research paper
[Reducing Activation Recomputation in Large Transformer Models](https://arxiv.org/pdf/2205.05198.pdf).
d. **Data Parallelism (DP)** via Distributed Optimizer: Reduces the memory footprint by sharding optimizer states and gradients across DP ranks
(versus the traditional method of replicating the optimizer state across data parallel ranks).
For example, when using Adam optimizer with mixed-precision training, each parameter accounts for 12 bytes of memory.
This gets distributed equally across the GPUs, i.e., each parameter would account for 3 bytes (12/4) if we have 4 GPUs.
For more details, please refer the research paper [ZeRO: Memory Optimizations Toward Training Trillion
Parameter Models](https://arxiv.org/pdf/1910.02054.pdf) and following section of blog
[The Technology Behind BLOOM Training](https://huggingface.co/blog/bloom-megatron-deepspeed#zero-data-parallelism).
e. **Selective Activation Recomputation**: Reduces the memory footprint of activations significantly via smart activation checkpointing.
It doesn't store activations occupying large memory while being fast to recompute thereby achieving great tradeoff between memory and recomputation.
For example, for GPT-3, this leads to 70% reduction in required memory for activations at the expense of
only 2.7% FLOPs overhead for recomputation of activations. For more details, please refer to the research paper
[Reducing Activation Recomputation in Large Transformer Models](https://arxiv.org/pdf/2205.05198.pdf).
f. **Fused Kernels**: Fused Softmax, Mixed Precision Fused Layer Norm and Fused gradient accumulation to weight gradient computation of linear layer.
PyTorch JIT compiled Fused GeLU and Fused Bias+Dropout+Residual addition.
g. **Support for Indexed datasets**: Efficient binary format of datasets for large scale training. Support for the `mmap`, `cached` index file and the `lazy` loader format.
h. **Checkpoint reshaping and interoperability**: Utility for reshaping Megatron-LM checkpoints of variable
tensor and pipeline parallel sizes to the beloved Transformers sharded checkpoints as it has great support with plethora of tools
such as Accelerate Big Model Inference, Megatron-DeepSpeed Inference etc.
Support is also available for converting Transformers sharded checkpoints to Megatron-LM checkpoint of variable tensor and pipeline parallel sizes
for large scale training.
## Pre-Requisites
You will need to install the latest pytorch, cuda, nccl, and NVIDIA [APEX](https://github.com/NVIDIA/apex#quick-start) releases and the nltk library.
See [documentation](https://github.com/NVIDIA/Megatron-LM#setup) for more details.
Another way to setup the environment is to pull an NVIDIA PyTorch Container that comes with all the required installations from NGC.
Below is a step-by-step method to set up the conda environment:
1. Create a virtual environment
```
conda create --name ml
```
2. Assuming that the machine has CUDA 11.3 installed, installing the corresponding PyTorch GPU Version
```
conda install pytorch torchvision torchaudio cudatoolkit=11.3 -c pytorch
```
3. Install Nvidia APEX
```
git clone https://github.com/NVIDIA/apex
cd apex
pip install -v --disable-pip-version-check --no-cache-dir --global-option="--cpp_ext" --global-option="--cuda_ext" ./
cd ..
```
4. Installing Megatron-LM
```
git clone https://github.com/NVIDIA/Megatron-LM.git
cd Megatron-LM
git checkout core_r0.5.0
pip install --no-use-pep517 -e .
```
## Accelerate Megatron-LM Plugin
Important features are directly supported via the `accelerate config` command.
An example of the corresponding questions for using Megatron-LM features is shown below:
```bash
:~$ accelerate config --config_file "megatron_gpt_config.yaml"
In which compute environment are you running? ([0] This machine, [1] AWS (Amazon SageMaker)): 0
Which type of machine are you using? ([0] No distributed training, [1] multi-CPU, [2] multi-GPU, [3] TPU): 2
How many different machines will you use (use more than 1 for multi-node training)? [1]:
Do you want to use DeepSpeed? [yes/NO]:
Do you want to use FullyShardedDataParallel? [yes/NO]:
Do you want to use Megatron-LM ? [yes/NO]: yes
What is the Tensor Parallelism degree/size? [1]:2
Do you want to enable Sequence Parallelism? [YES/no]:
What is the Pipeline Parallelism degree/size? [1]:2
What is the number of micro-batches? [1]:2
Do you want to enable selective activation recomputation? [YES/no]:
Do you want to use distributed optimizer which shards optimizer state and gradients across data parallel ranks? [YES/no]:
What is the gradient clipping value based on global L2 Norm (0 to disable)? [1.0]:
How many GPU(s) should be used for distributed training? [1]:4
Do you wish to use FP16 or BF16 (mixed precision)? [NO/fp16/bf16]: bf16
```
The resulting config is shown below:
```
~$ cat megatron_gpt_config.yaml
compute_environment: LOCAL_MACHINE
deepspeed_config: {}
distributed_type: MEGATRON_LM
downcast_bf16: 'no'
fsdp_config: {}
machine_rank: 0
main_process_ip: null
main_process_port: null
main_training_function: main
megatron_lm_config:
megatron_lm_gradient_clipping: 1.0
megatron_lm_num_micro_batches: 2
megatron_lm_pp_degree: 2
megatron_lm_recompute_activations: true
megatron_lm_sequence_parallelism: true
megatron_lm_tp_degree: 2
megatron_lm_use_distributed_optimizer: true
mixed_precision: bf16
num_machines: 1
num_processes: 4
rdzv_backend: static
same_network: true
use_cpu: false
```
We will take the example of GPT pre-training. The minimal changes required to the official `run_clm_no_trainer.py`
to use Megatron-LM are as follows:
1. As Megatron-LM uses its own implementation of Optimizer, the corresponding scheduler compatible with it needs to be used.
As such, support for only the Megatron-LM's scheduler is present. User will need to create `accelerate.utils.MegatronLMDummyScheduler`.
Example is given below:
```python
from accelerate.utils import MegatronLMDummyScheduler
if accelerator.distributed_type == DistributedType.MEGATRON_LM:
lr_scheduler = MegatronLMDummyScheduler(
optimizer=optimizer,
total_num_steps=args.max_train_steps,
warmup_num_steps=args.num_warmup_steps,
)
else:
lr_scheduler = get_scheduler(
name=args.lr_scheduler_type,
optimizer=optimizer,
num_warmup_steps=args.num_warmup_steps * args.gradient_accumulation_steps,
num_training_steps=args.max_train_steps * args.gradient_accumulation_steps,
)
```
2. Getting the details of the total batch size now needs to be cognization of tensor and pipeline parallel sizes.
Example of getting the effective total batch size is shown below:
```python
if accelerator.distributed_type == DistributedType.MEGATRON_LM:
total_batch_size = accelerator.state.megatron_lm_plugin.global_batch_size
else:
total_batch_size = args.per_device_train_batch_size * accelerator.num_processes * args.gradient_accumulation_steps
```
3. When using Megatron-LM, the losses are already averaged across the data parallel group
```python
if accelerator.distributed_type == DistributedType.MEGATRON_LM:
losses.append(loss)
else:
losses.append(accelerator.gather_for_metrics(loss.repeat(args.per_device_eval_batch_size)))
if accelerator.distributed_type == DistributedType.MEGATRON_LM:
losses = torch.tensor(losses)
else:
losses = torch.cat(losses)
```
4. For Megatron-LM, we need to save the model using `accelerator.save_state`
```python
if accelerator.distributed_type == DistributedType.MEGATRON_LM:
accelerator.save_state(args.output_dir)
else:
unwrapped_model = accelerator.unwrap_model(model)
unwrapped_model.save_pretrained(
args.output_dir, is_main_process=accelerator.is_main_process, save_function=accelerator.save
)
```
That's it! We are good to go 🚀. Please find the example script in the examples folder at the path `accelerate/examples/by_feature/megatron_lm_gpt_pretraining.py`.
Let's run it for `gpt-large` model architecture using 4 A100-80GB GPUs.
```bash
accelerate launch --config_file megatron_gpt_config.yaml \
examples/by_feature/megatron_lm_gpt_pretraining.py \
--config_name "gpt2-large" \
--tokenizer_name "gpt2-large" \
--dataset_name wikitext \
--dataset_config_name wikitext-2-raw-v1 \
--block_size 1024 \
--learning_rate 5e-5 \
--per_device_train_batch_size 24 \
--per_device_eval_batch_size 24 \
--num_train_epochs 5 \
--with_tracking \
--report_to "wandb" \
--output_dir "awesome_model"
```
Below are some important excerpts from the output logs:
```bash
Loading extension module fused_dense_cuda...
>>> done with compiling and loading fused kernels. Compilation time: 3.569 seconds
> padded vocab (size: 50257) with 175 dummy tokens (new size: 50432)
Building gpt model in the pre-training mode.
The Megatron LM model weights are initialized at random in `accelerator.prepare`. Please use `accelerator.load_checkpoint` to load a pre-trained checkpoint matching the distributed setup.
Preparing dataloader
Preparing dataloader
Preparing model
> number of parameters on (tensor, pipeline) model parallel rank (1, 0): 210753280
> number of parameters on (tensor, pipeline) model parallel rank (1, 1): 209445120
> number of parameters on (tensor, pipeline) model parallel rank (0, 0): 210753280
> number of parameters on (tensor, pipeline) model parallel rank (0, 1): 209445120
Preparing optimizer
Preparing scheduler
> learning rate decay style: linear
10/10/2022 22:57:22 - INFO - __main__ - ***** Running training *****
10/10/2022 22:57:22 - INFO - __main__ - Num examples = 2318
10/10/2022 22:57:22 - INFO - __main__ - Num Epochs = 5
10/10/2022 22:57:22 - INFO - __main__ - Instantaneous batch size per device = 24
10/10/2022 22:57:22 - INFO - __main__ - Total train batch size (w. parallel, distributed & accumulation) = 48
10/10/2022 22:57:22 - INFO - __main__ - Gradient Accumulation steps = 1
10/10/2022 22:57:22 - INFO - __main__ - Total optimization steps = 245
20%|████████████▍ | 49/245 [01:04<04:09, 1.27s/it]
10/10/2022 22:58:29 - INFO - __main__ - epoch 0: perplexity: 1222.1594275215962 eval_loss: 7.10837459564209
40%|████████████████████████▊ | 98/245 [02:10<03:07, 1.28s/it]
10/10/2022 22:59:35 - INFO - __main__ - epoch 1: perplexity: 894.5236583794557 eval_loss: 6.796291351318359
60%|████████████████████████████████████▌ | 147/245 [03:16<02:05, 1.28s/it]
10/10/2022 23:00:40 - INFO - __main__ - epoch 2: perplexity: 702.8458788508042 eval_loss: 6.555137634277344
80%|████████████████████████████████████████████████▊ | 196/245 [04:22<01:02, 1.28s/it]
10/10/2022 23:01:46 - INFO - __main__ - epoch 3: perplexity: 600.3220028695281 eval_loss: 6.39746618270874
100%|█████████████████████████████████████████████████████████████| 245/245 [05:27<00:00, 1.28s/it]
```
There are a large number of other options/features that one can set using `accelerate.utils.MegatronLMPlugin`.
## Advanced features to leverage writing custom train step and Megatron-LM Indexed Datasets
For leveraging more features, please go through below details.
1. Below is an example of changes required to customize the Train Step while using Megatron-LM.
You will implement the `accelerate.utils.AbstractTrainStep` or inherit from their corresponding children
`accelerate.utils.GPTTrainStep`, `accelerate.utils.BertTrainStep` or `accelerate.utils.T5TrainStep`.
```python
from accelerate.utils import MegatronLMDummyScheduler, GPTTrainStep, avg_losses_across_data_parallel_group
# Custom loss function for the Megatron model
class GPTTrainStepWithCustomLoss(GPTTrainStep):
def __init__(self, megatron_args, **kwargs):
super().__init__(megatron_args)
self.kwargs = kwargs
def get_loss_func(self):
def loss_func(inputs, loss_mask, output_tensor):
batch_size, seq_length = output_tensor.shape
losses = output_tensor.float()
loss_mask = loss_mask.view(-1).float()
loss = losses.view(-1) * loss_mask
# Resize and average loss per sample
loss_per_sample = loss.view(batch_size, seq_length).sum(axis=1)
loss_mask_per_sample = loss_mask.view(batch_size, seq_length).sum(axis=1)
loss_per_sample = loss_per_sample / loss_mask_per_sample
# Calculate and scale weighting
weights = torch.stack([(inputs == kt).float() for kt in self.kwargs["keytoken_ids"]]).sum(axis=[0, 2])
weights = 1.0 + self.kwargs["alpha"] * weights
# Calculate weighted average
weighted_loss = (loss_per_sample * weights).mean()
# Reduce loss across data parallel groups
averaged_loss = avg_losses_across_data_parallel_group([weighted_loss])
return weighted_loss, {"lm loss": averaged_loss[0]}
return loss_func
def get_forward_step_func(self):
def forward_step(data_iterator, model):
"""Forward step."""
# Get the batch.
tokens, labels, loss_mask, attention_mask, position_ids = self.get_batch(data_iterator)
output_tensor = model(tokens, position_ids, attention_mask, labels=labels)
return output_tensor, partial(self.loss_func, tokens, loss_mask)
return forward_step
def main():
# Custom loss function for the Megatron model
keytoken_ids = []
keywords = ["plt", "pd", "sk", "fit", "predict", " plt", " pd", " sk", " fit", " predict"]
for keyword in keywords:
ids = tokenizer([keyword]).input_ids[0]
if len(ids) == 1:
keytoken_ids.append(ids[0])
accelerator.print(f"Keytoken ids: {keytoken_ids}")
accelerator.state.megatron_lm_plugin.custom_train_step_class = GPTTrainStepWithCustomLoss
accelerator.state.megatron_lm_plugin.custom_train_step_kwargs = {
"keytoken_ids": keytoken_ids,
"alpha": 0.25,
}
```
2. For using the Megatron-LM datasets, a few more changes are required. Dataloaders for these datasets
are available only on rank 0 of each tensor parallel group. As such, there are rank where dataloader won't be
available and this requires tweaks to the training loop. Being able to do all this shows how
flexible and extensible Accelerate is. The changes required are as follows.
a. For Megatron-LM indexed datasets, we need to use `MegatronLMDummyDataLoader`
and pass the required dataset args to it such as `data_path`, `seq_length` etc.
See [here](https://github.com/NVIDIA/Megatron-LM/blob/main/megatron/arguments.py#L804) for the list of available args.
```python
from accelerate.utils import MegatronLMDummyDataLoader
megatron_dataloader_config = {
"data_path": args.data_path,
"splits_string": args.splits_string,
"seq_length": args.block_size,
"micro_batch_size": args.per_device_train_batch_size,
}
megatron_dataloader = MegatronLMDummyDataLoader(**megatron_dataloader_config)
accelerator.state.megatron_lm_plugin.megatron_dataset_flag = True
```
b. `megatron_dataloader` is repeated 3 times to get training, validation and test dataloaders
as per the `args.splits_string` proportions
```python
model, optimizer, lr_scheduler, train_dataloader, eval_dataloader, _ = accelerator.prepare(
model, optimizer, lr_scheduler, megatron_dataloader, megatron_dataloader, megatron_dataloader
)
```
c. Changes to training and evaluation loops as dataloader is only available on tensor parallel ranks 0
So, we need to iterate only if the dataloader isn't `None` else provide empty dict
As such, we loop using `while` loop and break when `completed_steps` is equal to `args.max_train_steps`
This is similar to the Megatron-LM setup wherein user has to provide `max_train_steps` when using Megaton-LM indexed datasets.
This displays how flexible and extensible Accelerate is.
```python
while completed_steps < args.max_train_steps:
model.train()
batch = next(train_dataloader) if train_dataloader is not None else {}
outputs = model(**batch)
loss = outputs.loss
...
if completed_steps % eval_interval == 0:
eval_completed_steps = 0
losses = []
while eval_completed_steps < eval_iters:
model.eval()
with torch.no_grad():
batch = next(eval_dataloader) if eval_dataloader is not None else {}
outputs = model(**batch)
```
## Utility for Checkpoint reshaping and interoperability
1. The scripts for these are present in Transformers library under respective models.
Currently, it is available for GPT model [checkpoint_reshaping_and_interoperability.py](https://github.com/huggingface/transformers/blob/main/src/transformers/models/megatron_gpt2/checkpoint_reshaping_and_interoperability.py)
2. Below is an example of conversion of checkpoint from Megatron-LM to universal Transformers sharded checkpoint.
```bash
python checkpoint_reshaping_and_interoperability.py \
--convert_checkpoint_from_megatron_to_transformers \
--load_path "gpt/iter_0005000" \
--save_path "gpt/trfs_checkpoint" \
--max_shard_size "200MB" \
--tokenizer_name "gpt2" \
--print-checkpoint-structure
```
3. Conversion of checkpoint from transformers to megatron with `tp_size=2`, `pp_size=2` and `dp_size=2`.
```bash
python checkpoint_utils/megatgron_gpt2/checkpoint_reshaping_and_interoperability.py \
--load_path "gpt/trfs_checkpoint" \
--save_path "gpt/megatron_lm_checkpoint" \
--target_tensor_model_parallel_size 2 \
--target_pipeline_model_parallel_size 2 \
--target_data_parallel_size 2 \
--target_params_dtype "bf16" \
--make_vocab_size_divisible_by 128 \
--use_distributed_optimizer \
--print-checkpoint-structure
```
## Megatron-LM GPT models support returning logits and `megatron_generate` function for text generation
1. Returning logits require setting `require_logits=True` in MegatronLMPlugin as shown below.
These would be available on the in the last stage of pipeline.
```python
megatron_lm_plugin = MegatronLMPlugin(return_logits=True)
```
2. `megatron_generate` method for Megatron-LM GPT model: This will use Tensor and Pipeline Parallelism to complete
generations for a batch of inputs when using greedy with/without top_k/top_p sampling and for individual prompt inputs when using beam search decoding.
Only a subset of features of transformers generate is supported. This will help in using large models via tensor and pipeline parallelism
for generation (already does key-value caching and uses fused kernels by default).
This requires data parallel size to be 1, sequence parallelism and activation checkpointing to be disabled.
It also requires specifying path to tokenizer's vocab file and merges file.
Below example shows how to configure and use `megatron_generate` method for Megatron-LM GPT model.
```python
# specifying tokenizer's vocab and merges file
vocab_file = os.path.join(args.resume_from_checkpoint, "vocab.json")
merge_file = os.path.join(args.resume_from_checkpoint, "merges.txt")
other_megatron_args = {"vocab_file": vocab_file, "merge_file": merge_file}
megatron_lm_plugin = MegatronLMPlugin(other_megatron_args=other_megatron_args)
# inference using `megatron_generate` functionality
tokenizer.pad_token = tokenizer.eos_token
max_new_tokens = 64
batch_texts = [
"Are you human?",
"The purpose of life is",
"The arsenal was constructed at the request of",
"How are you doing these days?",
]
batch_encodings = tokenizer(batch_texts, return_tensors="pt", padding=True)
# top-p sampling
generated_tokens = model.megatron_generate(
batch_encodings["input_ids"],
batch_encodings["attention_mask"],
max_new_tokens=max_new_tokens,
top_p=0.8,
top_p_decay=0.5,
temperature=0.9,
)
decoded_preds = tokenizer.batch_decode(generated_tokens.cpu().numpy())
accelerator.print(decoded_preds)
# top-k sampling
generated_tokens = model.megatron_generate(
batch_encodings["input_ids"],
batch_encodings["attention_mask"],
max_new_tokens=max_new_tokens,
top_k=50,
temperature=0.9,
)
decoded_preds = tokenizer.batch_decode(generated_tokens.cpu().numpy())
accelerator.print(decoded_preds)
# adding `bos` token at the start
generated_tokens = model.megatron_generate(
batch_encodings["input_ids"], batch_encodings["attention_mask"], max_new_tokens=max_new_tokens, add_BOS=True
)
decoded_preds = tokenizer.batch_decode(generated_tokens.cpu().numpy())
accelerator.print(decoded_preds)
# beam search => only takes single prompt
batch_texts = ["The purpose of life is"]
batch_encodings = tokenizer(batch_texts, return_tensors="pt", padding=True)
generated_tokens = model.megatron_generate(
batch_encodings["input_ids"],
batch_encodings["attention_mask"],
max_new_tokens=max_new_tokens,
num_beams=20,
length_penalty=1.5,
)
decoded_preds = tokenizer.batch_decode(generated_tokens.cpu().numpy())
accelerator.print(decoded_preds)
```
3. An end-to-end example of using `megatron_generate` method for Megatron-LM GPT model is available at
[megatron_gpt2_generation.py](https://github.com/pacman100/accelerate-megatron-test/blob/main/src/inference/megatron_gpt2_generation.py) with
config file [megatron_lm_gpt_generate_config.yaml](https://github.com/pacman100/accelerate-megatron-test/blob/main/src/Configs/megatron_lm_gpt_generate_config.yaml).
The bash script with accelerate launch command is available at [megatron_lm_gpt_generate.sh](https://github.com/pacman100/accelerate-megatron-test/blob/main/megatron_lm_gpt_generate.sh).
The output logs of the script are available at [megatron_lm_gpt_generate.log](https://github.com/pacman100/accelerate-megatron-test/blob/main/output_logs/megatron_lm_gpt_generate.log).
## Support for ROPE and ALiBi Positional embeddings and Multi-Query Attention
1. For ROPE/ALiBi attention, pass `position_embedding_type` with `("absolute" | "rotary" | "alibi")` to `MegatronLMPlugin` as shown below.
```python
other_megatron_args = {"position_embedding_type": "alibi"}
megatron_lm_plugin = MegatronLMPlugin(other_megatron_args=other_megatron_args)
```
2. For Multi-Query Attention, pass `attention_head_type` with `("multihead" | "multiquery")` to `MegatronLMPlugin` as shown below.
```python
other_megatron_args = {"attention_head_type": "multiquery"}
megatron_lm_plugin = MegatronLMPlugin(other_megatron_args=other_megatron_args)
```
## Caveats
1. Supports Transformers GPT2, Megatron-BERT and T5 models.
This covers Decoder only, Encode only and Encoder-Decoder model classes.
2. Only loss is returned from model forward pass as
there is quite complex interplay of pipeline, tensor and data parallelism behind the scenes.
The `model(**batch_data)` call return loss(es) averaged across the data parallel ranks.
This is fine for most cases wherein pre-training jobs are run using Megatron-LM features and
you can easily compute the `perplexity` using the loss.
For GPT model, returning logits in addition to loss(es) is supported.
These logits aren't gathered across data parallel ranks. Use `accelerator.utils.gather_across_data_parallel_groups`
to gather logits across data parallel ranks. These logits along with labels can be used for computing various
performance metrics.
3. The main process is the last rank as the losses/logits are available in the last stage of pipeline.
`accelerator.is_main_process` and `accelerator.is_local_main_process` return `True` for last rank when using
Megatron-LM integration.
4. In `accelerator.prepare` call, a Megatron-LM model corresponding to a given Transformers model is created
with random weights. Please use `accelerator.load_state` to load the Megatron-LM checkpoint with matching TP, PP and DP partitions.
5. Currently, checkpoint reshaping and interoperability support is only available for GPT.
Soon it will be extended to BERT and T5.
6. `gradient_accumulation_steps` needs to be 1. When using Megatron-LM, micro batches in pipeline parallelism
setting is synonymous with gradient accumulation.
7. When using Megatron-LM, use `accelerator.save_state` and `accelerator.load_state` for saving and loading checkpoints.
8. Below are the mapping from Megatron-LM model architectures to the the equivalent transformers model architectures.
Only these transformers model architectures are supported.
a. Megatron-LM [BertModel](https://github.com/NVIDIA/Megatron-LM/blob/main/megatron/model/bert_model.py) :
transformers models with `megatron-bert` in config's model type, e.g.,
[MegatronBERT](https://huggingface.co/docs/transformers/model_doc/megatron-bert)
b. Megatron-LM [GPTModel](https://github.com/NVIDIA/Megatron-LM/blob/main/megatron/model/gpt_model.py) :
transformers models with `gpt2` in config's model type, e.g.,
[OpenAI GPT2](https://huggingface.co/docs/transformers/model_doc/gpt2)
c. Megatron-LM [T5Model](https://github.com/NVIDIA/Megatron-LM/blob/main/megatron/model/t5_model.py) :
transformers models with `t5` in config's model type, e.g.,
[T5](https://huggingface.co/docs/transformers/model_doc/t5) and
[MT5](https://huggingface.co/docs/transformers/model_doc/mt5)
# Amazon SageMaker
Hugging Face and Amazon introduced new [Hugging Face Deep Learning Containers (DLCs)](https://github.com/aws/deep-learning-containers/blob/master/available_images.md#huggingface-training-containers) to
make it easier than ever to train Hugging Face Transformer models in [Amazon SageMaker](https://aws.amazon.com/sagemaker/).
## Getting Started
### Setup & Installation
Before you can run your Accelerate scripts on Amazon SageMaker you need to sign up for an AWS account. If you do not
have an AWS account yet learn more [here](https://docs.aws.amazon.com/sagemaker/latest/dg/gs-set-up.html).
After you have your AWS Account you need to install the `sagemaker` sdk for Accelerate with:
```bash
pip install "accelerate[sagemaker]" --upgrade
```
Accelerate currently uses the DLCs, with `transformers`, `datasets` and `tokenizers` pre-installed. Accelerate is not in the DLC yet (will soon be added!) so to use it within Amazon SageMaker you need to create a
`requirements.txt` in the same directory where your training script is located and add it as dependency:
```
accelerate
```
You should also add any other dependencies you have to this `requirements.txt`.
### Configure Accelerate
You can configure the launch configuration for Amazon SageMaker the same as you do for non SageMaker training jobs with
the Accelerate CLI:
```bash
accelerate config
# In which compute environment are you running? ([0] This machine, [1] AWS (Amazon SageMaker)): 1
```
Accelerate will go through a questionnaire about your Amazon SageMaker setup and create a config file you can edit.
Accelerate is not saving any of your credentials.
### Prepare a Accelerate fine-tuning script
The training script is very similar to a training script you might run outside of SageMaker, but to save your model
after training you need to specify either `/opt/ml/model` or use `os.environ["SM_MODEL_DIR"]` as your save
directory. After training, artifacts in this directory are uploaded to S3:
```diff
- torch.save('/opt/ml/model`)
+ accelerator.save('/opt/ml/model')
```
SageMaker doesn’t support argparse actions. If you want to use, for example, boolean hyperparameters, you need to
specify type as bool in your script and provide an explicit True or False value for this hyperparameter. [[REF]](https://sagemaker.readthedocs.io/en/stable/frameworks/pytorch/using_pytorch.html#prepare-a-pytorch-training-script).
### Launch Training
You can launch your training with Accelerate CLI with:
```
accelerate launch path_to_script.py --args_to_the_script
```
This will launch your training script using your configuration. The only thing you have to do is provide all the
arguments needed by your training script as named arguments.
**Examples**
If you run one of the example scripts, don't forget to add `accelerator.save('/opt/ml/model')` to it.
```bash
accelerate launch ./examples/sagemaker_example.py
```
Outputs:
```
Configuring Amazon SageMaker environment
Converting Arguments to Hyperparameters
Creating Estimator
2021-04-08 11:56:50 Starting - Starting the training job...
2021-04-08 11:57:13 Starting - Launching requested ML instancesProfilerReport-1617883008: InProgress
.........
2021-04-08 11:58:54 Starting - Preparing the instances for training.........
2021-04-08 12:00:24 Downloading - Downloading input data
2021-04-08 12:00:24 Training - Downloading the training image..................
2021-04-08 12:03:39 Training - Training image download completed. Training in progress..
........
epoch 0: {'accuracy': 0.7598039215686274, 'f1': 0.8178438661710037}
epoch 1: {'accuracy': 0.8357843137254902, 'f1': 0.882249560632689}
epoch 2: {'accuracy': 0.8406862745098039, 'f1': 0.8869565217391304}
........
2021-04-08 12:05:40 Uploading - Uploading generated training model
2021-04-08 12:05:40 Completed - Training job completed
Training seconds: 331
Billable seconds: 331
You can find your model data at: s3://your-bucket/accelerate-sagemaker-1-2021-04-08-11-56-47-108/output/model.tar.gz
```
## Advanced Features
### Distributed Training: Data Parallelism
Set up the accelerate config by running `accelerate config` and answer the SageMaker questions and set it up.
To use SageMaker DDP, select it when asked
`What is the distributed mode? ([0] No distributed training, [1] data parallelism):`.
Example config below:
```yaml
base_job_name: accelerate-sagemaker-1
compute_environment: AMAZON_SAGEMAKER
distributed_type: DATA_PARALLEL
ec2_instance_type: ml.p3.16xlarge
iam_role_name: xxxxx
image_uri: null
mixed_precision: fp16
num_machines: 1
profile: xxxxx
py_version: py38
pytorch_version: 1.10.2
region: us-east-1
transformers_version: 4.17.0
use_cpu: false
```
### Distributed Training: Model Parallelism
*currently in development, will be supported soon.*
### Python packages and dependencies
Accelerate currently uses the DLCs, with `transformers`, `datasets` and `tokenizers` pre-installed. If you
want to use different/other Python packages you can do this by adding them to the `requirements.txt`. These packages
will be installed before your training script is started.
### Local Training: SageMaker Local mode
The local mode in the SageMaker SDK allows you to run your training script locally inside the HuggingFace DLC (Deep Learning container)
or using your custom container image. This is useful for debugging and testing your training script inside the final container environment.
Local mode uses Docker compose (*Note: Docker Compose V2 is not supported yet*). The SDK will handle the authentication against ECR
to pull the DLC to your local environment. You can emulate CPU (single and multi-instance) and GPU (single instance) SageMaker training jobs.
To use local mode, you need to set your `ec2_instance_type` to `local`.
```yaml
ec2_instance_type: local
```
### Advanced configuration
The configuration allows you to override parameters for the [Estimator](https://sagemaker.readthedocs.io/en/stable/api/training/estimators.html).
These settings have to be applied in the config file and are not part of `accelerate config`. You can control many additional aspects of the training job, e.g. use Spot instances, enable network isolation and many more.
```yaml
additional_args:
# enable network isolation to restrict internet access for containers
enable_network_isolation: True
```
You can find all available configuration [here](https://sagemaker.readthedocs.io/en/stable/api/training/estimators.html).
### Use Spot Instances
You can use Spot Instances e.g. using (see [Advanced configuration](#advanced-configuration)):
```yaml
additional_args:
use_spot_instances: True
max_wait: 86400
```
*Note: Spot Instances are subject to be terminated and training to be continued from a checkpoint. This is not handled in Accelerate out of the box. Contact us if you would like this feature.*
### Remote scripts: Use scripts located on Github
*undecided if feature is needed. Contact us if you would like this feature.*
# Using Local SGD with Accelerate
Local SGD is a technique for distributed training where gradients are not synchronized every step. Thus, each process updates its own version of the model weights and after a given number of steps these weights are synchronized by averaging across all processes. This improves communication efficiency and can lead to substantial training speed up especially when a computer lacks a faster interconnect such as NVLink.
Unlike gradient accumulation (where improving communication efficiency requires increasing the effective batch size), Local SGD does not require changing a batch size or a learning rate / schedule. However, if necessary, Local SGD can be combined with gradient accumulation as well.
In this tutorial you will see how to quickly setup Local SGD Accelerate. Compared to a standard Accelerate setup, this requires only two extra lines of code.
This example will use a very simplistic PyTorch training loop that performs gradient accumulation every two batches:
```python
device = "cuda"
model.to(device)
gradient_accumulation_steps = 2
for index, batch in enumerate(training_dataloader):
inputs, targets = batch
inputs = inputs.to(device)
targets = targets.to(device)
outputs = model(inputs)
loss = loss_function(outputs, targets)
loss = loss / gradient_accumulation_steps
loss.backward()
if (index + 1) % gradient_accumulation_steps == 0:
optimizer.step()
scheduler.step()
optimizer.zero_grad()
```
## Converting it to Accelerate
First the code shown earlier will be converted to use Accelerate with neither a LocalSGD or a gradient accumulation helper:
```diff
+ from accelerate import Accelerator
+ accelerator = Accelerator()
+ model, optimizer, training_dataloader, scheduler = accelerator.prepare(
+ model, optimizer, training_dataloader, scheduler
+ )
for index, batch in enumerate(training_dataloader):
inputs, targets = batch
- inputs = inputs.to(device)
- targets = targets.to(device)
outputs = model(inputs)
loss = loss_function(outputs, targets)
loss = loss / gradient_accumulation_steps
+ accelerator.backward(loss)
if (index+1) % gradient_accumulation_steps == 0:
optimizer.step()
scheduler.step()
```
## Letting Accelerate handle model synchronization
All that is left now is to let Accelerate handle model parameter synchronization **and** the gradient accumulation for us. For simplicity let us assume we need to synchronize every 8 steps. This is
achieved by adding one `with LocalSGD` statement and one call `local_sgd.step()` after every optimizer step:
```diff
+local_sgd_steps=8
+with LocalSGD(accelerator=accelerator, model=model, local_sgd_steps=8, enabled=True) as local_sgd:
for batch in training_dataloader:
with accelerator.accumulate(model):
inputs, targets = batch
outputs = model(inputs)
loss = loss_function(outputs, targets)
accelerator.backward(loss)
optimizer.step()
scheduler.step()
optimizer.zero_grad()
+ local_sgd.step()
```
Under the hood, the Local SGD code **disables** automatic gradient synchronization (but accumulation still works as expected!). Instead it averages model parameters every `local_sgd_steps` steps (as well as at the end of the training loop).
## Limitations
The current implementation works only with basic multi-GPU (or multi-CPU) training without, e.g., [DeepSpeed.](https://github.com/microsoft/DeepSpeed).
## References
Although we are not aware of the true origins of this simple approach, the idea of local SGD is quite old and goes
back to at least:
Zhang, J., De Sa, C., Mitliagkas, I., & Ré, C. (2016). [Parallel SGD: When does averaging help?. arXiv preprint
arXiv:1606.07365.](https://arxiv.org/abs/1606.07365)
We credit the term Local SGD to the following paper (but there might be earlier references we are not aware of).
Stich, Sebastian Urban. ["Local SGD Converges Fast and Communicates Little." ICLR 2019-International Conference on
Learning Representations. No. CONF. 2019.](https://arxiv.org/abs/1805.09767)
# Model quantization
## `bitsandbytes` Integration
Accelerate brings `bitsandbytes` quantization to your model. You can now load any pytorch model in 8-bit or 4-bit with a few lines of code.
If you want to use Transformers models with `bitsandbytes`, you should follow this [documentation](https://huggingface.co/docs/transformers/main_classes/quantization).
To learn more about how the `bitsandbytes` quantization works, check out the blog posts on [8-bit quantization](https://huggingface.co/blog/hf-bitsandbytes-integration) and [4-bit quantization](https://huggingface.co/blog/4bit-transformers-bitsandbytes).
### Pre-Requisites
You will need to install the following requirements:
- Install `bitsandbytes` library
```bash
pip install bitsandbytes
```
- Install latest `accelerate` from source
```bash
pip install git+https://github.com/huggingface/accelerate.git
```
- Install `minGPT` and `huggingface_hub` to run examples
```bash
git clone https://github.com/karpathy/minGPT.git
pip install minGPT/
pip install huggingface_hub
```
### How it works
First, we need to initialize our model. To save memory, we can initialize an empty model using the context manager `init_empty_weights()`.
Let's take the GPT2 model from minGPT library.
```py
from accelerate import init_empty_weights
from mingpt.model import GPT
model_config = GPT.get_default_config()
model_config.model_type = 'gpt2-xl'
model_config.vocab_size = 50257
model_config.block_size = 1024
with init_empty_weights():
empty_model = GPT(model_config)
```
Then, we need to get the path to the weights of your model. The path can be the state_dict file (e.g. "pytorch_model.bin") or a folder containing the sharded checkpoints.
```py
from huggingface_hub import snapshot_download
weights_location = snapshot_download(repo_id="marcsun13/gpt2-xl-linear-sharded")
```
Finally, you need to set your quantization configuration with `BnbQuantizationConfig`.
Here's an example for 8-bit quantization:
```py
from accelerate.utils import BnbQuantizationConfig
bnb_quantization_config = BnbQuantizationConfig(load_in_8bit=True, llm_int8_threshold = 6)
```
Here's an example for 4-bit quantization:
```py
from accelerate.utils import BnbQuantizationConfig
bnb_quantization_config = BnbQuantizationConfig(load_in_4bit=True, bnb_4bit_compute_dtype=torch.bfloat16, bnb_4bit_use_double_quant=True, bnb_4bit_quant_type="nf4")
```
To quantize your empty model with the selected configuration, you need to use `load_and_quantize_model()`.
```py
from accelerate.utils import load_and_quantize_model
quantized_model = load_and_quantize_model(empty_model, weights_location=weights_location, bnb_quantization_config=bnb_quantization_config, device_map = "auto")
```
### Saving and loading 8-bit model
You can save your 8-bit model with accelerate using `save_model()`.
```py
from accelerate import Accelerator
accelerate = Accelerator()
new_weights_location = "path/to/save_directory"
accelerate.save_model(quantized_model, new_weights_location)
quantized_model_from_saved = load_and_quantize_model(empty_model, weights_location=new_weights_location, bnb_quantization_config=bnb_quantization_config, device_map = "auto")
```
Note that 4-bit model serialization is currently not supported.
### Offload modules to cpu and disk
You can offload some modules to cpu/disk if you don't have enough space on the GPU to store the entire model on your GPUs.
This uses big model inference under the hood. Check this [documentation](https://huggingface.co/docs/accelerate/usage_guides/big_modeling) for more details.
For 8-bit quantization, the selected modules will be converted to 8-bit precision.
For 4-bit quantization, the selected modules will be kept in `torch_dtype` that the user passed in `BnbQuantizationConfig`. We will add support to convert these offloaded modules in 4-bit when 4-bit serialization will be possible.
You just need to pass a custom `device_map` in order to offload modules on cpu/disk. The offload modules will be dispatched on the GPU when needed. Here's an example :
```py
device_map = {
"transformer.wte": 0,
"transformer.wpe": 0,
"transformer.drop": 0,
"transformer.h": "cpu",
"transformer.ln_f": "disk",
"lm_head": "disk",
}
```
### Fine-tune a quantized model
It is not possible to perform pure 8bit or 4bit training on these models. However, you can train these models by leveraging parameter efficient fine tuning methods (PEFT) and train for example adapters on top of them. Please have a look at [peft](https://github.com/huggingface/peft) library for more details.
Currently, you can't add adapters on top of any quantized model. However, with the official support of adapters with Transformers models, you can fine-tune quantized models. If you want to finetune a Transformers model , follow this [documentation](https://huggingface.co/docs/transformers/main_classes/quantization) instead. Check out this [demo](https://colab.research.google.com/drive/1VoYNfYDKcKRQRor98Zbf2-9VQTtGJ24k?usp=sharing) on how to fine-tune a 4-bit Transformers model.
Note that you don’t need to pass `device_map` when loading the model for training. It will automatically load your model on your GPU. Please note that `device_map=auto` should be used for inference only.
### Example demo - running GPT2 1.5b on a Google Colab
Check out the Google Colab [demo](https://colab.research.google.com/drive/1T1pOgewAWVpR9gKpaEWw4orOrzPFb3yM?usp=sharing) for running quantized models on a GTP2 model. The GPT2-1.5B model checkpoint is in FP32 which uses 6GB of memory. After quantization, it uses 1.6GB with 8-bit modules and 1.2GB with 4-bit modules.
# Intel® Extension for PyTorch
[IPEX](https://github.com/intel/intel-extension-for-pytorch) is optimized for CPUs with AVX-512 or above, and functionally works for CPUs with only AVX2. So, it is expected to bring performance benefit for Intel CPU generations with AVX-512 or above while CPUs with only AVX2 (e.g., AMD CPUs or older Intel CPUs) might result in a better performance under IPEX, but not guaranteed. IPEX provides performance optimizations for CPU training with both Float32 and BFloat16. The usage of BFloat16 is the main focus of the following sections.
Low precision data type BFloat16 has been natively supported on the 3rd Generation Xeon® Scalable Processors (aka Cooper Lake) with AVX512 instruction set and will be supported on the next generation of Intel® Xeon® Scalable Processors with Intel® Advanced Matrix Extensions (Intel® AMX) instruction set with further boosted performance. The Auto Mixed Precision for CPU backend has been enabled since PyTorch-1.10. At the same time, the support of Auto Mixed Precision with BFloat16 for CPU and BFloat16 optimization of operators has been massively enabled in Intel® Extension for PyTorch, and partially upstreamed to PyTorch master branch. Users can get better performance and user experience with IPEX Auto Mixed Precision.
## IPEX installation:
IPEX release is following PyTorch, to install via pip:
| PyTorch Version | IPEX version |
| :---------------: | :----------: |
| 2.0 | 2.0.0 |
| 1.13 | 1.13.0 |
| 1.12 | 1.12.300 |
| 1.11 | 1.11.200 |
| 1.10 | 1.10.100 |
```
pip install intel_extension_for_pytorch== -f https://developer.intel.com/ipex-whl-stable-cpu
```
Check more approaches for [IPEX installation](https://intel.github.io/intel-extension-for-pytorch/cpu/latest/tutorials/installation.html).
## How It Works For Training optimization in CPU
Accelerate has integrated [IPEX](https://github.com/intel/intel-extension-for-pytorch), all you need to do is enabling it through the config.
**Scenario 1**: Acceleration of No distributed CPU training
Run accelerate config on your machine:
```bash
$ accelerate config
-----------------------------------------------------------------------------------------------------------------------------------------------------------
In which compute environment are you running?
This machine
-----------------------------------------------------------------------------------------------------------------------------------------------------------
Which type of machine are you using?
No distributed training
Do you want to run your training on CPU only (even if a GPU / Apple Silicon device is available)? [yes/NO]:yes
Do you want to use Intel PyTorch Extension (IPEX) to speed up training on CPU? [yes/NO]:yes
Do you wish to optimize your script with torch dynamo?[yes/NO]:NO
Do you want to use DeepSpeed? [yes/NO]: NO
-----------------------------------------------------------------------------------------------------------------------------------------------------------
Do you wish to use FP16 or BF16 (mixed precision)?
bf16
```
This will generate a config file that will be used automatically to properly set the
default options when doing
```bash
accelerate launch my_script.py --args_to_my_script
```
For instance, here is how you would run the NLP example `examples/nlp_example.py` (from the root of the repo) with IPEX enabled.
default_config.yaml that is generated after `accelerate config`
```bash
compute_environment: LOCAL_MACHINE
distributed_type: 'NO'
downcast_bf16: 'no'
ipex_config:
ipex: true
machine_rank: 0
main_training_function: main
mixed_precision: bf16
num_machines: 1
num_processes: 1
rdzv_backend: static
same_network: true
tpu_env: []
tpu_use_cluster: false
tpu_use_sudo: false
use_cpu: true
```
```bash
accelerate launch examples/nlp_example.py
```
**Scenario 2**: Acceleration of distributed CPU training
we use Intel oneCCL for communication, combined with Intel® MPI library to deliver flexible, efficient, scalable cluster messaging on Intel® architecture. you could refer the [here](https://huggingface.co/docs/transformers/perf_train_cpu_many) for the installation guide
Run accelerate config on your machine(node0):
```bash
$ accelerate config
-----------------------------------------------------------------------------------------------------------------------------------------------------------
In which compute environment are you running?
This machine
-----------------------------------------------------------------------------------------------------------------------------------------------------------
Which type of machine are you using?
multi-CPU
How many different machines will you use (use more than 1 for multi-node training)? [1]: 4
-----------------------------------------------------------------------------------------------------------------------------------------------------------
What is the rank of this machine?
0
What is the IP address of the machine that will host the main process? 36.112.23.24
What is the port you will use to communicate with the main process? 29500
Are all the machines on the same local network? Answer `no` if nodes are on the cloud and/or on different network hosts [YES/no]: yes
Do you want to use Intel PyTorch Extension (IPEX) to speed up training on CPU? [yes/NO]:yes
Do you want accelerate to launch mpirun? [yes/NO]: yes
Please enter the path to the hostfile to use with mpirun [~/hostfile]: ~/hostfile
Enter the number of oneCCL worker threads [1]: 1
Do you wish to optimize your script with torch dynamo?[yes/NO]:NO
How many processes should be used for distributed training? [1]:16
-----------------------------------------------------------------------------------------------------------------------------------------------------------
Do you wish to use FP16 or BF16 (mixed precision)?
bf16
```
For instance, here is how you would run the NLP example `examples/nlp_example.py` (from the root of the repo) with IPEX enabled for distributed CPU training.
default_config.yaml that is generated after `accelerate config`
```bash
compute_environment: LOCAL_MACHINE
distributed_type: MULTI_CPU
downcast_bf16: 'no'
ipex_config:
ipex: true
machine_rank: 0
main_process_ip: 36.112.23.24
main_process_port: 29500
main_training_function: main
mixed_precision: bf16
mpirun_config:
mpirun_ccl: '1'
mpirun_hostfile: /home/user/hostfile
num_machines: 4
num_processes: 16
rdzv_backend: static
same_network: true
tpu_env: []
tpu_use_cluster: false
tpu_use_sudo: false
use_cpu: true
```
Set following env and using intel MPI to launch the training
In node0, you need to create a configuration file which contains the IP addresses of each node (for example hostfile) and pass that configuration file path as an argument.
If you selected to have Accelerate launch `mpirun`, ensure that the location of your hostfile matches the path in the config.
```bash
$ cat hostfile
xxx.xxx.xxx.xxx #node0 ip
xxx.xxx.xxx.xxx #node1 ip
xxx.xxx.xxx.xxx #node2 ip
xxx.xxx.xxx.xxx #node3 ip
```
When Accelerate is launching `mpirun`, source the oneCCL bindings setvars.sh to get your Intel MPI environment, and then
run your script using `accelerate launch`. Note that the python script and environment needs to exist on all of the
machines being used for multi-CPU training.
```bash
oneccl_bindings_for_pytorch_path=$(python -c "from oneccl_bindings_for_pytorch import cwd; print(cwd)")
source $oneccl_bindings_for_pytorch_path/env/setvars.sh
accelerate launch examples/nlp_example.py
```
Otherwise, if you selected not to have Accelerate launch `mpirun`, run the following command in node0 and **16DDP** will
be enabled in node0,node1,node2,node3 with BF16 mixed precision. When using this method, the python script, python
environment, and accelerate config file need to be present on all of the machines used for multi-CPU training.
```bash
oneccl_bindings_for_pytorch_path=$(python -c "from oneccl_bindings_for_pytorch import cwd; print(cwd)")
source $oneccl_bindings_for_pytorch_path/env/setvars.sh
export CCL_WORKER_COUNT=1
export MASTER_ADDR=xxx.xxx.xxx.xxx #node0 ip
export CCL_ATL_TRANSPORT=ofi
mpirun -f hostfile -n 16 -ppn 4 accelerate launch examples/nlp_example.py
```
## Related Resources
- [Project's github](https://github.com/intel/intel-extension-for-pytorch)
- [API docs](https://intel.github.io/intel-extension-for-pytorch/cpu/latest/tutorials/api_doc.html)
- [Tuning guide](https://intel.github.io/intel-extension-for-pytorch/cpu/latest/tutorials/performance_tuning/tuning_guide.html)
- [Blogs & Publications](https://intel.github.io/intel-extension-for-pytorch/cpu/latest/tutorials/blogs_publications.html)
# Low Precision Training Methods
Accelerate provides integrations to train on lower precision methods using specified supported hardware through the `TransformersEngine` and `MS-AMP` packages. This documentation will help guide you through what hardware is supported, how to configure your `Accelerator` to leverage the low precision methods, and what you can expect when training.
## What training on FP8 means
To explore more of the nitty-gritty in training in FP8 with PyTorch and Accelerate, check out the [concept_guide](../concept_guides/low_precision_training) on why this can be difficult. But essentially rather than training in BF16, some (or all) aspects of training a model can be performed using 8 bits instead of 16. The challenge is doing so without degrading final performance.
This is only enabled on specific NVIDIA hardware, namely:
* Anything after the 3000 series consumer graphics cards (such as the 4090)
* Hopper-based GPU architectures (such as the `H100` and `H200`)
What this will result in is some gain in the memory used (as we've cut the needed memory in half for some parts of training) and an increase in throughput *should* be seen as well for larger models that can replace certain layers with FP8-enabled ones.
## Configuring the Accelerator
Currently two different backends for FP8 are supported (`TransformersEngine` and `MS-AMP`), each with different capabilities and configurations.
To use either, the same core API is used. Just pass `mixed_precision="fp8"` to either the `Accelerator`, during `accelerate config` when prompted about mixed precision, or as part of your `config.yaml` file in the `mixed_precision` key:
```{python}
from accelerate import Accelerator
accelerator = Accelerator(mixed_precision="fp8")
```
By default, if `MS-AMP` is available in your environment, Accelerate will automatically utilize it as a backend. To specify it yourself (and customize other parts of the FP8 mixed precision setup), you can utilize the `utils.FP8RecipeKwargs` or clarify it in your config `yaml`/during `accelerate launch`:
```{python}
from accelerate import Accelerator
from accelerate.utils import FP8RecipeKwargs
kwargs = [FP8RecipeKwargs(backend="msamp")]
# Or to specify the backend as `TransformersEngine` even if MS-AMP is installed
# kwargs = [FP8RecipeKwargs(backend="te")]
accelerator = Accelerator(mixed_precision="fp8", kwarg_handlers=kwargs)
```
```{yaml}
mixed_precision: fp8
fp8_config:
amax_compute_algorithm: max
amax_history_length: 1024
backend: TE
fp8_format: HYBRID
interval: 1
margin: 0
override_linear_precision: false
use_autocast_during_eval: false
```
## Configuring MS-AMP
Of the two, `MS-AMP` is traditionally the easier one to configure as there is only a single argument: the optimization level.
Currently two levels of optimization are supported in the Accelerate integration, `"O1"` and `"O2"` (using the letter 'o', not zero).
* `"O1"` will cast the weight gradients and `all_reduce` communications to happen in 8-bit, while the rest are done in 16 bit. This reduces the general GPU memory usage and speeds up communication bandwidths.
* `"O2"` will also cast first-order optimizer states into 8 bit, while the second order states are in FP16. (Currently just the `Adam` optimizer is supported). This tries its best to minimize final accuracy degradation and will save the highest potential memory.
To specify an optimization level, pass it to the `FP8KwargsHandler` by setting the `optimization_level` argument:
```{python}
from accelerate import Accelerator
from accelerate.utils import FP8RecipeKwargs
kwargs = [FP8RecipeKwargs(backend="msamp", optimization_level="O2")]
accelerator = Accelerator(mixed_precision="fp8", kwarg_handlers=kwargs)
```
Or during `accelerate launch` via `--fp8_backend=msamp --fp8_opt_level=O2`
Similarly this can be set in your `config.yaml`:
```{yaml}
mixed_precision: fp8
fp8_config:
backend: MSAMP
opt_level: O2
```
## Configuring TransformersEngine
TransformersEngine has much more available for customizing how and what FP8 calculations are performed. A full list of supported arguments and what they mean are available in [NVIDIA's documentation](https://docs.nvidia.com/deeplearning/transformer-engine/user-guide/api/common.html), however they are restated as part of `FP8KwargsHandler`'s docstring for your convenience.
Accelerate tries to set sensible defaults, but exploring and tweaking the various parameters yourself can lead to better performance potentially.
To use it, specify `backend="te"` and modify any of the arguments you want as part of your kwarg handler:
```{python}
from accelerate import Accelerator
from accelerate.utils import FP8RecipeKwargs
kwargs = [FP8RecipeKwargs(backend="te", ...)]
accelerator = Accelerator(mixed_precision="fp8", kwarg_handlers=kwargs)
```
Or during `accelerate launch` via `--fp8_backend=te ...`. Use `accelerate launch --fp8_backend=te -h` to see relevent arguments.
Similarly this can be set in your `config.yaml`:
```{yaml}
mixed_precision: fp8
fp8_config:
amax_compute_algorithm: max
amax_history_length: 1024
backend: TE
fp8_format: HYBRID
interval: 1
margin: 0
override_linear_precision: false
use_autocast_during_eval: false
```
## Example Zoo
We have examples showcasing training with FP8 both with accelerate and its underlying implementation available in the accelerate repo.
Currently we support scripts showcasing:
* Single GPU
* Distributed Data Parallelism (Multi-GPU)
* Fully Sharded Data Parallelism
* DeepSpeed ZeRO 1 through 3
Find out more [here](https://github.com/huggingface/accelerate/tree/main/benchmarks/fp8)
## Further Reading
To learn more about training in FP8 please check out the following resources:
* [Our concept guide](../concept_guides/low_precision_training) detailing into more about both TransformersEngine and MS-AMP
* [The `transformers-engine` documentation](https://docs.nvidia.com/deeplearning/transformer-engine/user-guide/api/common.html)
* [The `MS-AMP` documentation](https://azure.github.io/MS-AMP/docs/)
# Accelerated PyTorch Training on Mac
With PyTorch v1.12 release, developers and researchers can take advantage of Apple silicon GPUs for significantly faster model training.
This unlocks the ability to perform machine learning workflows like prototyping and fine-tuning locally, right on Mac.
Apple's Metal Performance Shaders (MPS) as a backend for PyTorch enables this and can be used via the new `"mps"` device.
This will map computational graphs and primitives on the MPS Graph framework and tuned kernels provided by MPS.
For more information please refer official documents [Introducing Accelerated PyTorch Training on Mac](https://pytorch.org/blog/introducing-accelerated-pytorch-training-on-mac/)
and [MPS BACKEND](https://pytorch.org/docs/stable/notes/mps.html).
### Benefits of Training and Inference using Apple Silicon Chips
1. Enables users to train larger networks or batch sizes locally
2. Reduces data retrieval latency and provides the GPU with direct access to the full memory store due to unified memory architecture.
Therefore, improving end-to-end performance.
3. Reduces costs associated with cloud-based development or the need for additional local GPUs.
**Pre-requisites**: To install torch with mps support,
please follow this nice medium article [GPU-Acceleration Comes to PyTorch on M1 Macs](https://medium.com/towards-data-science/gpu-acceleration-comes-to-pytorch-on-m1-macs-195c399efcc1).
## How it works out of the box
It is enabled by default on MacOs machines with MPS enabled Apple Silicon GPUs.
To disable it, pass `--cpu` flag to `accelerate launch` command or answer the corresponding question when answering the `accelerate config` questionnaire.
You can directly run the following script to test it out on MPS enabled Apple Silicon machines:
```bash
accelerate launch /examples/cv_example.py --data_dir images
```
## A few caveats to be aware of
1. We strongly recommend to install PyTorch >= 1.13 (nightly version at the time of writing) on your MacOS machine.
It has major fixes related to model correctness and performance improvements for transformer based models.
Please refer to https://github.com/pytorch/pytorch/issues/82707 for more details.
2. Distributed setups `gloo` and `nccl` are not working with `mps` device.
This means that currently only single GPU of `mps` device type can be used.
Finally, please, remember that, `Accelerate` only integrates MPS backend, therefore if you
have any problems or questions with regards to MPS backend usage, please, file an issue with [PyTorch GitHub](https://github.com/pytorch/pytorch/issues).
# Model memory estimator
One very difficult aspect when exploring potential models to use on your machine is knowing just how big of a model will *fit* into memory with your current graphics card (such as loading the model onto CUDA).
To help alleviate this, Accelerate has a CLI interface through `accelerate estimate-memory`. This tutorial will
help walk you through using it, what to expect, and at the end link to the interactive demo hosted on the Hub which will
even let you post those results directly on the model repo!
Currently we support searching for models that can be used in `timm` and `transformers`.
This API will load the model into memory on the `meta` device, so we are not actually downloading
and loading the full weights of the model into memory, nor do we need to. As a result it's
perfectly fine to measure 8 billion parameter models (or more), without having to worry about
if your CPU can handle it!
## Gradio Demos
Below are a few gradio demos related to what was described above. The first is the official Hugging Face memory estimation space, utilizing Accelerate directly:
A community member has taken the idea and expanded it further, allowing you to filter models directly and see if you can run a particular LLM given GPU constraints and LoRA configurations. To play with it, see [here](https://huggingface.co/spaces/Vokturz/can-it-run-llm) for more details.
## The Command
When using `accelerate estimate-memory`, you need to pass in the name of the model you want to use, potentially the framework
that model utilizing (if it can't be found automatically), and the data types you want the model to be loaded in with.
For example, here is how we can calculate the memory footprint for `bert-base-cased`:
```bash
accelerate estimate-memory bert-base-cased
```
This will download the `config.json` for `bert-based-cased`, load the model on the `meta` device, and report back how much space
it will use:
Memory Usage for loading `bert-base-cased`:
| dtype | Largest Layer | Total Size | Training using Adam |
|---------|---------------|------------|---------------------|
| float32 | 84.95 MB | 418.18 MB | 1.61 GB |
| float16 | 42.47 MB | 206.59 MB | 826.36 MB |
| int8 | 21.24 MB | 103.29 MB | 413.18 MB |
| int4 | 10.62 MB | 51.65 MB | 206.59 MB |
By default it will return all the supported dtypes (`int4` through `float32`), but if you are interested in specific ones these can be filtered.
### Specific libraries
If the source library cannot be determined automatically (like it could in the case of `bert-base-cased`), a library name can
be passed in.
```bash
accelerate estimate-memory HuggingFaceM4/idefics-80b-instruct --library_name transformers
```
Memory Usage for loading `HuggingFaceM4/idefics-80b-instruct`:
| dtype | Largest Layer | Total Size | Training using Adam |
|---------|---------------|------------|---------------------|
| float32 | 3.02 GB | 297.12 GB | 1.16 TB |
| float16 | 1.51 GB | 148.56 GB | 594.24 GB |
| int8 | 772.52 MB | 74.28 GB | 297.12 GB |
| int4 | 386.26 MB | 37.14 GB | 148.56 GB |
```bash
accelerate estimate-memory timm/resnet50.a1_in1k --library_name timm
```
Memory Usage for loading `timm/resnet50.a1_in1k`:
| dtype | Largest Layer | Total Size | Training using Adam |
|---------|---------------|------------|---------------------|
| float32 | 9.0 MB | 97.7 MB | 390.78 MB |
| float16 | 4.5 MB | 48.85 MB | 195.39 MB |
| int8 | 2.25 MB | 24.42 MB | 97.7 MB |
| int4 | 1.12 MB | 12.21 MB | 48.85 MB |
### Specific dtypes
As mentioned earlier, while we return `int4` through `float32` by default, any dtype can be used from `float32`, `float16`, `int8`, and `int4`.
To do so, pass them in after specifying `--dtypes`:
```bash
accelerate estimate-memory bert-base-cased --dtypes float32 float16
```
Memory Usage for loading `bert-base-cased`:
| dtype | Largest Layer | Total Size | Training using Adam |
|---------|---------------|------------|---------------------|
| float32 | 84.95 MB | 413.18 MB | 1.61 GB |
| float16 | 42.47 MB | 206.59 MB | 826.36 MB |
## Caveats with this calculator
This calculator will tell you how much memory is needed to purely load the model in, *not* to perform inference.
This calculation is accurate within a few % of the actual value, so it is a very good view of just how much memory it will take. For instance loading `bert-base-cased` actually takes `413.68 MB` when loaded on CUDA in full precision, and the calculator estimates `413.18 MB`.
When performing inference you can expect to add up to an additional 20% as found by [EleutherAI](https://blog.eleuther.ai/transformer-math/). We'll be conducting research into finding a more accurate estimate to these values, and will update
this calculator once done.
# Performing gradient accumulation with Accelerate
Gradient accumulation is a technique where you can train on bigger batch sizes than
your machine would normally be able to fit into memory. This is done by accumulating gradients over
several batches, and only stepping the optimizer after a certain number of batches have been performed.
While technically standard gradient accumulation code would work fine in a distributed setup, it is not the most efficient
method for doing so and you may experience considerable slowdowns!
In this tutorial you will see how to quickly setup gradient accumulation and perform it with the utilities provided in Accelerate,
which can total to adding just one new line of code!
This example will use a very simplistic PyTorch training loop that performs gradient accumulation every two batches:
```python
device = "cuda"
model.to(device)
gradient_accumulation_steps = 2
for index, batch in enumerate(training_dataloader):
inputs, targets = batch
inputs = inputs.to(device)
targets = targets.to(device)
outputs = model(inputs)
loss = loss_function(outputs, targets)
loss = loss / gradient_accumulation_steps
loss.backward()
if (index + 1) % gradient_accumulation_steps == 0:
optimizer.step()
scheduler.step()
optimizer.zero_grad()
```
## Converting it to Accelerate
First the code shown earlier will be converted to utilize Accelerate without the special gradient accumulation helper:
```diff
+ from accelerate import Accelerator
+ accelerator = Accelerator()
+ model, optimizer, training_dataloader, scheduler = accelerator.prepare(
+ model, optimizer, training_dataloader, scheduler
+ )
for index, batch in enumerate(training_dataloader):
inputs, targets = batch
- inputs = inputs.to(device)
- targets = targets.to(device)
outputs = model(inputs)
loss = loss_function(outputs, targets)
loss = loss / gradient_accumulation_steps
+ accelerator.backward(loss)
if (index+1) % gradient_accumulation_steps == 0:
optimizer.step()
scheduler.step()
optimizer.zero_grad()
```
In its current state, this code is not going to perform gradient accumulation efficiently due to a process called gradient synchronization. Read more about that in the [Concepts tutorial](../concept_guides/gradient_synchronization)!
## Letting Accelerate handle gradient accumulation
All that is left now is to let Accelerate handle the gradient accumulation for us. To do so you should pass in a `gradient_accumulation_steps` parameter to `Accelerator`, dictating the number
of steps to perform before each call to `step()` and how to automatically adjust the loss during the call to `backward()`:
```diff
from accelerate import Accelerator
- accelerator = Accelerator()
+ accelerator = Accelerator(gradient_accumulation_steps=2)
```
Alternatively, you can pass in a `gradient_accumulation_plugin` parameter to the `Accelerator` object's `__init__`, which will allow you to further customize the gradient accumulation behavior.
Read more about that in the [GradientAccumulationPlugin](../package_reference/accelerator#accelerate.utils.GradientAccumulationPlugin) docs.
From here you can use the `accumulate()` context manager from inside your training loop to automatically perform the gradient accumulation for you!
You just wrap it around the entire training part of our code:
```diff
- for index, batch in enumerate(training_dataloader):
+ for batch in training_dataloader:
+ with accelerator.accumulate(model):
inputs, targets = batch
outputs = model(inputs)
```
You can remove all the special checks for the step number and the loss adjustment:
```diff
- loss = loss / gradient_accumulation_steps
accelerator.backward(loss)
- if (index+1) % gradient_accumulation_steps == 0:
optimizer.step()
scheduler.step()
optimizer.zero_grad()
```
As you can see the `Accelerator` is able to keep track of the batch number you are on and it will automatically know whether to step through the prepared optimizer and how to adjust the loss.
Typically with gradient accumulation, you would need to adjust the number of steps to reflect the change in total batches you are
training on. Accelerate automagically does this for you by default. Behind the scenes we instantiate a `GradientAccumulationPlugin` configured to do this.
The `state.GradientState` is sync'd with the active dataloader being iterated upon. As such it assumes naively that when we have reached the end of the dataloader everything will sync and a step will be performed. To disable this, set `sync_with_dataloader` to be `False` in the `GradientAccumulationPlugin`:
```{python}
from accelerate import Accelerator
from accelerate.utils import GradientAccumulationPlugin
plugin = GradientAccumulationPlugin(sync_with_dataloader=False)
accelerator = Accelerator(..., gradient_accumulation_plugin=plugin)
```
## The finished code
Below is the finished implementation for performing gradient accumulation with Accelerate
```python
from accelerate import Accelerator
accelerator = Accelerator(gradient_accumulation_steps=2)
model, optimizer, training_dataloader, scheduler = accelerator.prepare(
model, optimizer, training_dataloader, scheduler
)
for batch in training_dataloader:
with accelerator.accumulate(model):
inputs, targets = batch
outputs = model(inputs)
loss = loss_function(outputs, targets)
accelerator.backward(loss)
optimizer.step()
scheduler.step()
optimizer.zero_grad()
```
It's important that **only one forward/backward** should be done inside the context manager `with accelerator.accumulate(model)`.
To learn more about what magic this wraps around, read the [Gradient Synchronization concept guide](../concept_guides/gradient_synchronization)
## Self-contained example
Here is a self-contained example that you can run to see gradient accumulation in action with Accelerate:
```python
import torch
import copy
from accelerate import Accelerator
from accelerate.utils import set_seed
from torch.utils.data import TensorDataset, DataLoader
# seed
set_seed(0)
# define toy inputs and labels
x = torch.tensor([1., 2., 3., 4., 5., 6., 7., 8.])
y = torch.tensor([2., 4., 6., 8., 10., 12., 14., 16.])
gradient_accumulation_steps = 4
batch_size = len(x) // gradient_accumulation_steps
# define dataset and dataloader
dataset = TensorDataset(x, y)
dataloader = DataLoader(dataset, batch_size=batch_size)
# define model, optimizer and loss function
class SimpleLinearModel(torch.nn.Module):
def __init__(self):
super(SimpleLinearModel, self).__init__()
self.weight = torch.nn.Parameter(torch.zeros((1, 1)))
def forward(self, inputs):
return inputs @ self.weight
model = SimpleLinearModel()
model_clone = copy.deepcopy(model)
criterion = torch.nn.MSELoss()
model_optimizer = torch.optim.SGD(model.parameters(), lr=0.02)
accelerator = Accelerator(gradient_accumulation_steps=gradient_accumulation_steps)
model, model_optimizer, dataloader = accelerator.prepare(model, model_optimizer, dataloader)
model_clone_optimizer = torch.optim.SGD(model_clone.parameters(), lr=0.02)
print(f"initial model weight is {model.weight.mean().item():.5f}")
print(f"initial model weight is {model_clone.weight.mean().item():.5f}")
for i, (inputs, labels) in enumerate(dataloader):
with accelerator.accumulate(model):
inputs = inputs.view(-1, 1)
print(i, inputs.flatten())
labels = labels.view(-1, 1)
outputs = model(inputs)
loss = criterion(outputs, labels)
accelerator.backward(loss)
model_optimizer.step()
model_optimizer.zero_grad()
loss = criterion(x.view(-1, 1) @ model_clone.weight, y.view(-1, 1))
model_clone_optimizer.zero_grad()
loss.backward()
model_clone_optimizer.step()
print(f"w/ accumulation, the final model weight is {model.weight.mean().item():.5f}")
print(f"w/o accumulation, the final model weight is {model_clone.weight.mean().item():.5f}")
```
```
initial model weight is 0.00000
initial model weight is 0.00000
0 tensor([1., 2.])
1 tensor([3., 4.])
2 tensor([5., 6.])
3 tensor([7., 8.])
w/ accumulation, the final model weight is 2.04000
w/o accumulation, the final model weight is 2.04000
```
# Experiment trackers
There are a large number of experiment tracking API's available, however getting them all to work with in a multi-processing environment can oftentimes be complex.
Accelerate provides a general tracking API that can be used to log useful items during your script through `Accelerator.log()`
## Integrated Trackers
Currently `Accelerate` supports seven trackers out-of-the-box:
- TensorBoard
- WandB
- CometML
- Aim
- MLFlow
- ClearML
- DVCLive
To use any of them, pass in the selected type(s) to the `log_with` parameter in `Accelerate`:
```python
from accelerate import Accelerator
from accelerate.utils import LoggerType
accelerator = Accelerator(log_with="all") # For all available trackers in the environment
accelerator = Accelerator(log_with="wandb")
accelerator = Accelerator(log_with=["wandb", LoggerType.TENSORBOARD])
```
At the start of your experiment `Accelerator.init_trackers()` should be used to setup your project, and potentially add any experiment hyperparameters to be logged:
```python
hps = {"num_iterations": 5, "learning_rate": 1e-2}
accelerator.init_trackers("my_project", config=hps)
```
When you are ready to log any data, `Accelerator.log()` should be used.
A `step` can also be passed in to correlate the data with a particular step in the training loop.
```python
accelerator.log({"train_loss": 1.12, "valid_loss": 0.8}, step=1)
```
Once you've finished training, make sure to run `Accelerator.end_training()` so that all the trackers can run their finish functionalities if they have any.
```python
accelerator.end_training()
```
A full example is below:
```python
from accelerate import Accelerator
accelerator = Accelerator(log_with="all")
config = {
"num_iterations": 5,
"learning_rate": 1e-2,
"loss_function": str(my_loss_function),
}
accelerator.init_trackers("example_project", config=config)
my_model, my_optimizer, my_training_dataloader = accelerate.prepare(my_model, my_optimizer, my_training_dataloader)
device = accelerator.device
my_model.to(device)
for iteration in config["num_iterations"]:
for step, batch in my_training_dataloader:
my_optimizer.zero_grad()
inputs, targets = batch
inputs = inputs.to(device)
targets = targets.to(device)
outputs = my_model(inputs)
loss = my_loss_function(outputs, targets)
accelerator.backward(loss)
my_optimizer.step()
accelerator.log({"training_loss": loss}, step=step)
accelerator.end_training()
```
If a tracker requires a directory to save data to, such as `TensorBoard`, then pass the directory path to `project_dir`. The `project_dir` parameter is useful
when there are other configurations to be combined with in the `ProjectConfiguration` data class. For example, you can save the TensorBoard data to `project_dir` and everything else can be logged in the `logging_dir` parameter of [`~utils.ProjectConfiguration`:
```python
accelerator = Accelerator(log_with="tensorboard", project_dir=".")
# use with ProjectConfiguration
config = ProjectConfiguration(project_dir=".", logging_dir="another/directory")
accelerator = Accelerator(log_with="tensorboard", project_config=config)
```
## Implementing Custom Trackers
To implement a new tracker to be used in `Accelerator`, a new one can be made through implementing the `GeneralTracker` class.
Every tracker must implement three functions and have three properties:
- `__init__`:
- Should store a `run_name` and initialize the tracker API of the integrated library.
- If a tracker stores their data locally (such as TensorBoard), a `logging_dir` parameter can be added.
- `store_init_configuration`:
- Should take in a `values` dictionary and store them as a one-time experiment configuration
- `log`:
- Should take in a `values` dictionary and a `step`, and should log them to the run
- `name` (`str`):
- A unique string name for the tracker, such as `"wandb"` for the wandb tracker.
- This will be used for interacting with this tracker specifically
- `requires_logging_directory` (`bool`):
- Whether a `logging_dir` is needed for this particular tracker and if it uses one.
- `tracker`:
- This should be implemented as a `@property` function
- Should return the internal tracking mechanism the library uses, such as the `run` object for `wandb`.
Each method should also utilize the `state.PartialState` class if the logger should only be executed on the main process for instance.
A brief example can be seen below with an integration with Weights and Biases, containing only the relevant information and logging just on
the main process:
```python
from accelerate.tracking import GeneralTracker, on_main_process
from typing import Optional
import wandb
class MyCustomTracker(GeneralTracker):
name = "wandb"
requires_logging_directory = False
@on_main_process
def __init__(self, run_name: str):
self.run_name = run_name
run = wandb.init(self.run_name)
@property
def tracker(self):
return self.run.run
@on_main_process
def store_init_configuration(self, values: dict):
wandb.config(values)
@on_main_process
def log(self, values: dict, step: Optional[int] = None):
wandb.log(values, step=step)
```
When you are ready to build your `Accelerator` object, pass in an **instance** of your tracker to `Accelerator.log_with` to have it automatically
be used with the API:
```python
tracker = MyCustomTracker("some_run_name")
accelerator = Accelerator(log_with=tracker)
```
These also can be mixed with existing trackers, including with `"all"`:
```python
tracker = MyCustomTracker("some_run_name")
accelerator = Accelerator(log_with=[tracker, "all"])
```
## Accessing the internal tracker
If some custom interactions with a tracker might be wanted directly, you can quickly access one using the
`Accelerator.get_tracker()` method. Just pass in the string corresponding to a tracker's `.name` attribute
and it will return that tracker on the main process.
This example shows doing so with wandb:
```python
wandb_tracker = accelerator.get_tracker("wandb")
```
From there you can interact with `wandb`'s `run` object like normal:
```python
wandb_run.log_artifact(some_artifact_to_log)
```
Trackers built in Accelerate will automatically execute on the correct process,
so if a tracker is only meant to be ran on the main process it will do so
automatically.
If you want to truly remove Accelerate's wrapping entirely, you can
achieve the same outcome with:
```python
wandb_tracker = accelerator.get_tracker("wandb", unwrap=True)
if accelerator.is_main_process:
wandb_tracker.log_artifact(some_artifact_to_log)
```
## When a wrapper cannot work
If a library has an API that does not follow a strict `.log` with an overall dictionary such as Neptune.AI, logging can be done manually under an `if accelerator.is_main_process` statement:
```diff
from accelerate import Accelerator
+ import neptune
accelerator = Accelerator()
+ run = neptune.init_run(...)
my_model, my_optimizer, my_training_dataloader = accelerate.prepare(my_model, my_optimizer, my_training_dataloader)
device = accelerator.device
my_model.to(device)
for iteration in config["num_iterations"]:
for batch in my_training_dataloader:
my_optimizer.zero_grad()
inputs, targets = batch
inputs = inputs.to(device)
targets = targets.to(device)
outputs = my_model(inputs)
loss = my_loss_function(outputs, targets)
total_loss += loss
accelerator.backward(loss)
my_optimizer.step()
+ if accelerator.is_main_process:
+ run["logs/training/batch/loss"].log(loss)
```
# Example Zoo
Below contains a non-exhaustive list of tutorials and scripts showcasing Accelerate.
## Official Accelerate Examples:
### Basic Examples
These examples showcase the base features of Accelerate and are a great starting point
- [Barebones NLP example](https://github.com/huggingface/accelerate/blob/main/examples/nlp_example.py)
- [Barebones distributed NLP example in a Jupyter Notebook](https://github.com/huggingface/notebooks/blob/main/examples/accelerate_examples/simple_nlp_example.ipynb)
- [Barebones computer vision example](https://github.com/huggingface/accelerate/blob/main/examples/cv_example.py)
- [Barebones distributed computer vision example in a Jupyter Notebook](https://github.com/huggingface/notebooks/blob/main/examples/accelerate_examples/simple_cv_example.ipynb)
- [Using Accelerate in Kaggle](https://www.kaggle.com/code/muellerzr/multi-gpu-and-accelerate)
### Feature Specific Examples
These examples showcase specific features that the Accelerate framework offers
- [Automatic memory-aware gradient accumulation](https://github.com/huggingface/accelerate/blob/main/examples/by_feature/automatic_gradient_accumulation.py)
- [Checkpointing states](https://github.com/huggingface/accelerate/blob/main/examples/by_feature/checkpointing.py)
- [Cross validation](https://github.com/huggingface/accelerate/blob/main/examples/by_feature/cross_validation.py)
- [DeepSpeed](https://github.com/huggingface/accelerate/blob/main/examples/by_feature/deepspeed_with_config_support.py)
- [Fully Sharded Data Parallelism](https://github.com/huggingface/accelerate/blob/main/examples/by_feature/fsdp_with_peak_mem_tracking.py)
- [Gradient accumulation](https://github.com/huggingface/accelerate/blob/main/examples/by_feature/gradient_accumulation.py)
- [Memory-aware batch size finder](https://github.com/huggingface/accelerate/blob/main/examples/by_feature/memory.py)
- [Metric Computation](https://github.com/huggingface/accelerate/blob/main/examples/by_feature/multi_process_metrics.py)
- [Using Trackers](https://github.com/huggingface/accelerate/blob/main/examples/by_feature/tracking.py)
- [Using Megatron-LM](https://github.com/huggingface/accelerate/blob/main/examples/by_feature/megatron_lm_gpt_pretraining.py)
### Full Examples
These examples showcase every feature in Accelerate at once that was shown in "Feature Specific Examples"
- [Complete NLP example](https://github.com/huggingface/accelerate/blob/main/examples/complete_nlp_example.py)
- [Complete computer vision example](https://github.com/huggingface/accelerate/blob/main/examples/complete_cv_example.py)
- [Very complete and extensible vision example showcasing SLURM, hydra, and a very extensible usage of the framework](https://github.com/yuvalkirstain/PickScore)
- [Causal language model fine-tuning example](https://github.com/huggingface/transformers/blob/main/examples/pytorch/language-modeling/run_clm_no_trainer.py)
- [Masked language model fine-tuning example](https://github.com/huggingface/transformers/blob/main/examples/pytorch/language-modeling/run_mlm_no_trainer.py)
- [Speech pretraining example](https://github.com/huggingface/transformers/blob/main/examples/pytorch/speech-pretraining/run_wav2vec2_pretraining_no_trainer.py)
- [Translation fine-tuning example](https://github.com/huggingface/transformers/blob/main/examples/pytorch/translation/run_translation_no_trainer.py)
- [Text classification fine-tuning example](https://github.com/huggingface/transformers/blob/main/examples/pytorch/text-classification/run_glue_no_trainer.py)
- [Semantic segmentation fine-tuning example](https://github.com/huggingface/transformers/blob/main/examples/pytorch/semantic-segmentation/run_semantic_segmentation_no_trainer.py)
- [Question answering fine-tuning example](https://github.com/huggingface/transformers/blob/main/examples/pytorch/question-answering/run_qa_no_trainer.py)
- [Beam search question answering fine-tuning example](https://github.com/huggingface/transformers/blob/main/examples/pytorch/question-answering/run_qa_beam_search_no_trainer.py)
- [Multiple choice question answering fine-tuning example](https://github.com/huggingface/transformers/blob/main/examples/pytorch/multiple-choice/run_swag_no_trainer.py)
- [Named entity recognition fine-tuning example](https://github.com/huggingface/transformers/blob/main/examples/pytorch/token-classification/run_ner_no_trainer.py)
- [Image classification fine-tuning example](https://github.com/huggingface/transformers/blob/main/examples/pytorch/image-classification/run_image_classification_no_trainer.py)
- [Summarization fine-tuning example](https://github.com/huggingface/transformers/blob/main/examples/pytorch/summarization/run_summarization_no_trainer.py)
- [End-to-end examples on how to use AWS SageMaker integration of Accelerate](https://github.com/huggingface/notebooks/blob/main/sagemaker/22_accelerate_sagemaker_examples/README.md)
- [Megatron-LM examples for various NLp tasks](https://github.com/pacman100/accelerate-megatron-test)
## Integration Examples
These are tutorials from libraries that integrate with Accelerate:
> Don't find your integration here? Make a PR to include it!
### Amphion
- [Training Text-to-Speech Models with Amphion](https://github.com/open-mmlab/Amphion/blob/main/egs/tts/README.md)
- [Training Singing Voice Conversion Models with Amphion](https://github.com/open-mmlab/Amphion/blob/main/egs/svc/README.md)
- [Training Vocoders with Amphion](https://github.com/open-mmlab/Amphion/blob/main/egs/vocoder/README.md)
### Catalyst
- [Distributed training tutorial with Catalyst](https://catalyst-team.github.io/catalyst/tutorials/ddp.html)
### DALLE2-pytorch
- [Fine-tuning DALLE2](https://github.com/lucidrains/DALLE2-pytorch#usage)
### Diffusers
- [Performing textual inversion with diffusers](https://github.com/huggingface/diffusers/tree/main/examples/textual_inversion)
- [Training DreamBooth with diffusers](https://github.com/huggingface/diffusers/tree/main/examples/dreambooth)
### fastai
- [Distributed training from Jupyter Notebooks with fastai](https://docs.fast.ai/tutorial.distributed.html)
- [Basic distributed training examples with fastai](https://docs.fast.ai/examples/distributed_app_examples.html)
### GradsFlow
- [Auto Image Classification with GradsFlow](https://docs.gradsflow.com/en/latest/examples/nbs/01-ImageClassification/)
### imagen-pytorch
- [Fine-tuning Imagen](https://github.com/lucidrains/imagen-pytorch#usage)
### Kornia
- [Fine-tuning vision models with Kornia's Trainer](https://kornia.readthedocs.io/en/latest/get-started/training.html)
### PyTorch Accelerated
- [Quickstart distributed training tutorial with PyTorch Accelerated](https://pytorch-accelerated.readthedocs.io/en/latest/quickstart.html)
### PyTorch3D
- [Perform Deep Learning with 3D data](https://pytorch3d.org/tutorials/)
### Stable-Dreamfusion
- [Training with Stable-Dreamfusion to convert text to a 3D model](https://colab.research.google.com/drive/1MXT3yfOFvO0ooKEfiUUvTKwUkrrlCHpF?usp=sharing)
### Tez
- [Leaf disease detection with Tez and Accelerate](https://www.kaggle.com/code/abhishek/tez-faster-and-easier-training-for-leaf-detection/notebook)
### trlx
- [How to implement a sentiment learning task with trlx](https://github.com/CarperAI/trlx#example-how-to-add-a-task)
### Comfy-UI
- [Enabling using large Stable Diffusion Models in low-vram settings using Accelerate](https://github.com/comfyanonymous/ComfyUI/blob/master/comfy/model_management.py#L291-L296)
## In Science
Below contains a non-exhaustive list of papers utilizing Accelerate.
> Don't find your paper here? Make a PR to include it!
* Yuval Kirstain, Adam Polyak, Uriel Singer, Shahbuland Matiana, Joe Penna, Omer Levy: “Pick-a-Pic: An Open Dataset of User Preferences for Text-to-Image Generation”, 2023; [arXiv:2305.01569](http://arxiv.org/abs/2305.01569).
* Lei Wang, Wanyu Xu, Yihuai Lan, Zhiqiang Hu, Yunshi Lan, Roy Ka-Wei Lee, Ee-Peng Lim: “Plan-and-Solve Prompting: Improving Zero-Shot Chain-of-Thought Reasoning by Large Language Models”, 2023; [arXiv:2305.04091](http://arxiv.org/abs/2305.04091).
* Arthur Câmara, Claudia Hauff: “Moving Stuff Around: A study on efficiency of moving documents into memory for Neural IR models”, 2022; [arXiv:2205.08343](http://arxiv.org/abs/2205.08343).
* Ying Sheng, Lianmin Zheng, Binhang Yuan, Zhuohan Li, Max Ryabinin, Daniel Y. Fu, Zhiqiang Xie, Beidi Chen, Clark Barrett, Joseph E. Gonzalez, Percy Liang, Christopher Ré, Ion Stoica, Ce Zhang: “High-throughput Generative Inference of Large Language Models with a Single GPU”, 2023; [arXiv:2303.06865](http://arxiv.org/abs/2303.06865).
* Peter Melchior, Yan Liang, ChangHoon Hahn, Andy Goulding: “Autoencoding Galaxy Spectra I: Architecture”, 2022; [arXiv:2211.07890](http://arxiv.org/abs/2211.07890).
* Jiaao Chen, Aston Zhang, Mu Li, Alex Smola, Diyi Yang: “A Cheaper and Better Diffusion Language Model with Soft-Masked Noise”, 2023; [arXiv:2304.04746](http://arxiv.org/abs/2304.04746).
* Ayaan Haque, Matthew Tancik, Alexei A. Efros, Aleksander Holynski, Angjoo Kanazawa: “Instruct-NeRF2NeRF: Editing 3D Scenes with Instructions”, 2023; [arXiv:2303.12789](http://arxiv.org/abs/2303.12789).
* Luke Melas-Kyriazi, Christian Rupprecht, Iro Laina, Andrea Vedaldi: “RealFusion: 360° Reconstruction of Any Object from a Single Image”, 2023; [arXiv:2302.10663](http://arxiv.org/abs/2302.10663).
* Xiaoshi Wu, Keqiang Sun, Feng Zhu, Rui Zhao, Hongsheng Li: “Better Aligning Text-to-Image Models with Human Preference”, 2023; [arXiv:2303.14420](http://arxiv.org/abs/2303.14420).
* Yongliang Shen, Kaitao Song, Xu Tan, Dongsheng Li, Weiming Lu, Yueting Zhuang: “HuggingGPT: Solving AI Tasks with ChatGPT and its Friends in HuggingFace”, 2023; [arXiv:2303.17580](http://arxiv.org/abs/2303.17580).
* Yue Yang, Wenlin Yao, Hongming Zhang, Xiaoyang Wang, Dong Yu, Jianshu Chen: “Z-LaVI: Zero-Shot Language Solver Fueled by Visual Imagination”, 2022; [arXiv:2210.12261](http://arxiv.org/abs/2210.12261).
* Sheng-Yen Chou, Pin-Yu Chen, Tsung-Yi Ho: “How to Backdoor Diffusion Models?”, 2022; [arXiv:2212.05400](http://arxiv.org/abs/2212.05400).
* Junyoung Seo, Wooseok Jang, Min-Seop Kwak, Jaehoon Ko, Hyeonsu Kim, Junho Kim, Jin-Hwa Kim, Jiyoung Lee, Seungryong Kim: “Let 2D Diffusion Model Know 3D-Consistency for Robust Text-to-3D Generation”, 2023; [arXiv:2303.07937](http://arxiv.org/abs/2303.07937).
* Or Patashnik, Daniel Garibi, Idan Azuri, Hadar Averbuch-Elor, Daniel Cohen-Or: “Localizing Object-level Shape Variations with Text-to-Image Diffusion Models”, 2023; [arXiv:2303.11306](http://arxiv.org/abs/2303.11306).
* Dídac Surís, Sachit Menon, Carl Vondrick: “ViperGPT: Visual Inference via Python Execution for Reasoning”, 2023; [arXiv:2303.08128](http://arxiv.org/abs/2303.08128).
* Chenyang Qi, Xiaodong Cun, Yong Zhang, Chenyang Lei, Xintao Wang, Ying Shan, Qifeng Chen: “FateZero: Fusing Attentions for Zero-shot Text-based Video Editing”, 2023; [arXiv:2303.09535](http://arxiv.org/abs/2303.09535).
* Sean Welleck, Jiacheng Liu, Ximing Lu, Hannaneh Hajishirzi, Yejin Choi: “NaturalProver: Grounded Mathematical Proof Generation with Language Models”, 2022; [arXiv:2205.12910](http://arxiv.org/abs/2205.12910).
* Elad Richardson, Gal Metzer, Yuval Alaluf, Raja Giryes, Daniel Cohen-Or: “TEXTure: Text-Guided Texturing of 3D Shapes”, 2023; [arXiv:2302.01721](http://arxiv.org/abs/2302.01721).
* Puijin Cheng, Li Lin, Yijin Huang, Huaqing He, Wenhan Luo, Xiaoying Tang: “Learning Enhancement From Degradation: A Diffusion Model For Fundus Image Enhancement”, 2023; [arXiv:2303.04603](http://arxiv.org/abs/2303.04603).
* Shun Shao, Yftah Ziser, Shay Cohen: “Erasure of Unaligned Attributes from Neural Representations”, 2023; [arXiv:2302.02997](http://arxiv.org/abs/2302.02997).
* Seonghyeon Ye, Hyeonbin Hwang, Sohee Yang, Hyeongu Yun, Yireun Kim, Minjoon Seo: “In-Context Instruction Learning”, 2023; [arXiv:2302.14691](http://arxiv.org/abs/2302.14691).
* Shikun Liu, Linxi Fan, Edward Johns, Zhiding Yu, Chaowei Xiao, Anima Anandkumar: “Prismer: A Vision-Language Model with An Ensemble of Experts”, 2023; [arXiv:2303.02506](http://arxiv.org/abs/2303.02506).
* Haoyu Chen, Zhihua Wang, Yang Yang, Qilin Sun, Kede Ma: “Learning a Deep Color Difference Metric for Photographic Images”, 2023; [arXiv:2303.14964](http://arxiv.org/abs/2303.14964).
* Van-Hoang Le, Hongyu Zhang: “Log Parsing with Prompt-based Few-shot Learning”, 2023; [arXiv:2302.07435](http://arxiv.org/abs/2302.07435).
* Keito Kudo, Yoichi Aoki, Tatsuki Kuribayashi, Ana Brassard, Masashi Yoshikawa, Keisuke Sakaguchi, Kentaro Inui: “Do Deep Neural Networks Capture Compositionality in Arithmetic Reasoning?”, 2023; [arXiv:2302.07866](http://arxiv.org/abs/2302.07866).
* Ruoyao Wang, Peter Jansen, Marc-Alexandre Côté, Prithviraj Ammanabrolu: “Behavior Cloned Transformers are Neurosymbolic Reasoners”, 2022; [arXiv:2210.07382](http://arxiv.org/abs/2210.07382).
* Martin Wessel, Tomáš Horych, Terry Ruas, Akiko Aizawa, Bela Gipp, Timo Spinde: “Introducing MBIB -- the first Media Bias Identification Benchmark Task and Dataset Collection”, 2023; [arXiv:2304.13148](http://arxiv.org/abs/2304.13148). DOI: [https://dx.doi.org/10.1145/3539618.3591882 10.1145/3539618.3591882].
* Hila Chefer, Yuval Alaluf, Yael Vinker, Lior Wolf, Daniel Cohen-Or: “Attend-and-Excite: Attention-Based Semantic Guidance for Text-to-Image Diffusion Models”, 2023; [arXiv:2301.13826](http://arxiv.org/abs/2301.13826).
* Marcio Fonseca, Yftah Ziser, Shay B. Cohen: “Factorizing Content and Budget Decisions in Abstractive Summarization of Long Documents”, 2022; [arXiv:2205.12486](http://arxiv.org/abs/2205.12486).
* Elad Richardson, Gal Metzer, Yuval Alaluf, Raja Giryes, Daniel Cohen-Or: “TEXTure: Text-Guided Texturing of 3D Shapes”, 2023; [arXiv:2302.01721](http://arxiv.org/abs/2302.01721).
* Tianxing He, Jingyu Zhang, Tianle Wang, Sachin Kumar, Kyunghyun Cho, James Glass, Yulia Tsvetkov: “On the Blind Spots of Model-Based Evaluation Metrics for Text Generation”, 2022; [arXiv:2212.10020](http://arxiv.org/abs/2212.10020).
* Ori Ram, Yoav Levine, Itay Dalmedigos, Dor Muhlgay, Amnon Shashua, Kevin Leyton-Brown, Yoav Shoham: “In-Context Retrieval-Augmented Language Models”, 2023; [arXiv:2302.00083](http://arxiv.org/abs/2302.00083).
* Dacheng Li, Rulin Shao, Hongyi Wang, Han Guo, Eric P. Xing, Hao Zhang: “MPCFormer: fast, performant and private Transformer inference with MPC”, 2022; [arXiv:2211.01452](http://arxiv.org/abs/2211.01452).
* Baolin Peng, Michel Galley, Pengcheng He, Chris Brockett, Lars Liden, Elnaz Nouri, Zhou Yu, Bill Dolan, Jianfeng Gao: “GODEL: Large-Scale Pre-Training for Goal-Directed Dialog”, 2022; [arXiv:2206.11309](http://arxiv.org/abs/2206.11309).
* Egil Rønningstad, Erik Velldal, Lilja Øvrelid: “Entity-Level Sentiment Analysis (ELSA): An exploratory task survey”, 2023, Proceedings of the 29th International Conference on Computational Linguistics, 2022, pages 6773-6783; [arXiv:2304.14241](http://arxiv.org/abs/2304.14241).
* Charlie Snell, Ilya Kostrikov, Yi Su, Mengjiao Yang, Sergey Levine: “Offline RL for Natural Language Generation with Implicit Language Q Learning”, 2022; [arXiv:2206.11871](http://arxiv.org/abs/2206.11871).
* Zhiruo Wang, Shuyan Zhou, Daniel Fried, Graham Neubig: “Execution-Based Evaluation for Open-Domain Code Generation”, 2022; [arXiv:2212.10481](http://arxiv.org/abs/2212.10481).
* Minh-Long Luu, Zeyi Huang, Eric P. Xing, Yong Jae Lee, Haohan Wang: “Expeditious Saliency-guided Mix-up through Random Gradient Thresholding”, 2022; [arXiv:2212.04875](http://arxiv.org/abs/2212.04875).
* Jun Hao Liew, Hanshu Yan, Daquan Zhou, Jiashi Feng: “MagicMix: Semantic Mixing with Diffusion Models”, 2022; [arXiv:2210.16056](http://arxiv.org/abs/2210.16056).
* Yaqing Wang, Subhabrata Mukherjee, Xiaodong Liu, Jing Gao, Ahmed Hassan Awadallah, Jianfeng Gao: “LiST: Lite Prompted Self-training Makes Parameter-Efficient Few-shot Learners”, 2021; [arXiv:2110.06274](http://arxiv.org/abs/2110.06274).
# DDP Communication Hooks
Distributed Data Parallel (DDP) communication hooks provide a generic interface to control how gradients are communicated across workers by overriding the vanilla allreduce in `DistributedDataParallel`. A few built-in communication hooks are provided, and users can easily apply any of these hooks to optimize communication.
- **FP16 Compression Hook**: Compresses gradients by casting them to half-precision floating-point format (`torch.float16`), reducing communication overhead.
- **BF16 Compression Hook**: Similar to FP16, but uses the Brain Floating Point format (`torch.bfloat16`), which can be more efficient on certain hardware.
- **PowerSGD Hook**: An advanced gradient compression algorithm that provides high compression rates and can accelerate bandwidth-bound distributed training.
In this tutorial, you will see how to quickly set up DDP communication hooks and perform training with the utilities provided in Accelerate, which can be as simple as adding just one new line of code! This demonstrates how to use DDP communication hooks to optimize gradient communication in distributed training with the Accelerate library.
## FP16 Compression Hook
```python
import torch
from torch.nn.parallel import DistributedDataParallel as DDP
from torch.distributed.algorithms.ddp_comm_hooks import default_hooks
class MyModel(torch.nn.Module):
def __init__(self):
super().__init__()
self.layer = torch.nn.Linear(10, 10)
def forward(self, x):
return self.layer(x)
model = MyModel()
model = DDP(model, device_ids=[torch.cuda.current_device()])
model.register_comm_hook(state=None, hook=default_hooks.fp16_compress_hook)
# Training loop
for data, targets in data_loader:
outputs = model(data)
loss = criterion(outputs, targets)
loss.backward()
optimizer.step()
optimizer.zero_grad()
```
```python
from accelerate import Accelerator, DDPCommunicationHookType, DistributedDataParallelKwargs
import torch
class MyModel(torch.nn.Module):
def __init__(self):
super().__init__()
self.layer = torch.nn.Linear(10, 10)
def forward(self, x):
return self.layer(x)
# DDP Communication Hook setup
ddp_kwargs = DistributedDataParallelKwargs(comm_hook=DDPCommunicationHookType.FP16)
accelerator = Accelerator(kwargs_handlers=[ddp_kwargs])
model = MyModel()
optimizer = torch.optim.Adam(model.parameters())
data_loader = DataLoader(dataset, batch_size=16)
model, optimizer, data_loader = accelerator.prepare(model, optimizer, data_loader)
# Training loop
for data, targets in data_loader:
outputs = model(data)
loss = criterion(outputs, targets)
accelerator.backward(loss)
optimizer.step()
optimizer.zero_grad()
```
### BF16 Compression Hook
BF16 Compression Hook API is experimental, and it requires NCCL version later than 2.9.6.
```python
import torch
from torch.nn.parallel import DistributedDataParallel as DDP
from torch.distributed.algorithms.ddp_comm_hooks import default_hooks
class MyModel(torch.nn.Module):
def __init__(self):
super().__init__()
self.layer = torch.nn.Linear(10, 10)
def forward(self, x):
return self.layer(x)
model = MyModel()
model = DDP(model, device_ids=[torch.cuda.current_device()])
model.register_comm_hook(state=None, hook=default_hooks.bf16_compress_hook)
# Training loop
for data, targets in data_loader:
outputs = model(data)
loss = criterion(outputs, targets)
loss.backward()
optimizer.step()
optimizer.zero_grad()
```
```python
from accelerate import Accelerator, DDPCommunicationHookType, DistributedDataParallelKwargs
import torch
class MyModel(torch.nn.Module):
def __init__(self):
super().__init__()
self.layer = torch.nn.Linear(10, 10)
def forward(self, x):
return self.layer(x)
# DDP Communication Hook setup
ddp_kwargs = DistributedDataParallelKwargs(comm_hook=DDPCommunicationHookType.BF16)
accelerator = Accelerator(kwargs_handlers=[ddp_kwargs])
model = MyModel()
optimizer = torch.optim.Adam(model.parameters())
data_loader = DataLoader(dataset, batch_size=16)
model, optimizer, data_loader = accelerator.prepare(model, optimizer, data_loader)
# Training loop
for data, targets in data_loader:
outputs = model(data)
loss = criterion(outputs, targets)
accelerator.backward(loss)
optimizer.step()
optimizer.zero_grad()
```
### PowerSGD Hook
PowerSGD typically requires extra memory of the same size as the model’s gradients to enable error feedback, which can compensate for biased compressed communication and improve accuracy.
```python
import torch
from torch.nn.parallel import DistributedDataParallel as DDP
from torch.distributed.algorithms.ddp_comm_hooks import powerSGD_hook
class MyModel(torch.nn.Module):
def __init__(self):
super().__init__()
self.layer = torch.nn.Linear(10, 10)
def forward(self, x):
return self.layer(x)
model = MyModel()
model = DDP(model, device_ids=[torch.cuda.current_device()])
state = powerSGD_hook.PowerSGDState(process_group=None)
model.register_comm_hook(state=state, hook=powerSGD_hook.powerSGD_hook)
# Training loop
for data, targets in data_loader:
outputs = model(data)
loss = criterion(outputs, targets)
loss.backward()
optimizer.step()
optimizer.zero_grad()
```
```python
from accelerate import Accelerator, DDPCommunicationHookType, DistributedDataParallelKwargs
import torch
class MyModel(torch.nn.Module):
def __init__(self):
super().__init__()
self.layer = torch.nn.Linear(10, 10)
def forward(self, x):
return self.layer(x)
# DDP Communication Hook setup
ddp_kwargs = DistributedDataParallelKwargs(comm_hook=DDPCommunicationHookType.POWER_SGD)
accelerator = Accelerator(kwargs_handlers=[ddp_kwargs])
model = MyModel()
optimizer = torch.optim.Adam(model.parameters())
data_loader = DataLoader(dataset, batch_size=16)
model, optimizer, data_loader = accelerator.prepare(model, optimizer, data_loader)
# Training loop
for data, targets in data_loader:
outputs = model(data)
loss = criterion(outputs, targets)
accelerator.backward(loss)
optimizer.step()
optimizer.zero_grad()
```
## DDP Communication Hooks utilities
There are two additional utilities for supporting optional functionalities with the communication hooks.
### comm_wrapper
`comm_wrapper` is an option to wrap a communication hook with additional functionality. For example, it can be used to combine FP16 compression with other communication strategies. Currently supported wrappers are `no`, `fp16`, and `bf16`.
```python
from accelerate import Accelerator, DDPCommunicationHookType, DistributedDataParallelKwargs
import torch
class MyModel(torch.nn.Module):
def __init__(self):
super().__init__()
self.layer = torch.nn.Linear(10, 10)
def forward(self, x):
return self.layer(x)
# DDP Communication Hook setup
ddp_kwargs = DistributedDataParallelKwargs(
comm_hook=DDPCommunicationHookType.POWER_SGD,
comm_wrapper=DDPCommunicationHookType.FP16
)
accelerator = Accelerator(kwargs_handlers=[ddp_kwargs])
model = MyModel()
optimizer = torch.optim.Adam(model.parameters())
data_loader = DataLoader(dataset, batch_size=16)
model, optimizer, data_loader = accelerator.prepare(model, optimizer, data_loader)
# Training loop
for data, targets in data_loader:
outputs = model(data)
loss = criterion(outputs, targets)
accelerator.backward(loss)
optimizer.step()
optimizer.zero_grad()
```
### comm_state_option
`comm_state_option` allows you to pass additional state information required by certain communication hooks. This is particularly useful for stateful hooks like `PowerSGD`, which require maintaining hyperparameters and internal states across training steps. Below is an example showcasing the use of `comm_state_option` with the `PowerSGD` hook.
```python
from accelerate import Accelerator, DDPCommunicationHookType, DistributedDataParallelKwargs
import torch
class MyModel(torch.nn.Module):
def __init__(self):
super().__init__()
self.layer = torch.nn.Linear(10, 10)
def forward(self, x):
return self.layer(x)
# DDP Communication Hook setup
ddp_kwargs = DistributedDataParallelKwargs(
comm_hook=DDPCommunicationHookType.POWER_SGD,
comm_state_option={"matrix_approximation_rank": 2}
)
accelerator = Accelerator(kwargs_handlers=[ddp_kwargs])
model = MyModel()
optimizer = torch.optim.Adam(model.parameters())
data_loader = DataLoader(dataset, batch_size=16)
model, optimizer, data_loader = accelerator.prepare(model, optimizer, data_loader)
# Training loop
for data, targets in data_loader:
outputs = model(data)
loss = criterion(outputs, targets)
accelerator.backward(loss)
optimizer.step()
optimizer.zero_grad()
```
For more advanced usage and additional hooks, refer to the [PyTorch DDP Communication Hooks documentation](https://pytorch.org/docs/stable/ddp_comm_hooks.html).
# Loading big models into memory
When loading a pre-trained model in PyTorch, the usual workflow looks like this:
```py
import torch
my_model = ModelClass(...)
state_dict = torch.load(checkpoint_file)
my_model.load_state_dict(state_dict)
```
In plain English, those steps are:
1. Create the model with randomly initialized weights
2. Load the model weights (in a dictionary usually called a state dict) from the disk
3. Load those weights inside the model
While this works very well for regularly sized models, this workflow has some clear limitations when we deal with a huge model: in step 1, we load a full version of the model in RAM, and spend some time randomly initializing the weights (which will be discarded in step 3). In step 2, we load another full version of the model in RAM, with the pre-trained weights. If you're loading a model with 6 billion parameters, this means you will need 24GB of RAM for each copy of the model, so 48GB in total (half of it to load the model in FP16).
This API is quite new and still in its experimental stage. While we strive to provide a stable API, it's possible some small parts of the public API will change in the future.
## How the Process Works: A Quick Overview
## How the Process Works: Working with Code
### Instantiating an empty model
The first tool Accelerate introduces to help with big models is a context manager `init_empty_weights()` that helps you initialize a model without using any RAM so that step 1 can be done on models of any size. Here is how it works:
```py
from accelerate import init_empty_weights
with init_empty_weights():
my_model = ModelClass(...)
```
For instance:
```py
with init_empty_weights():
model = nn.Sequential(*[nn.Linear(10000, 10000) for _ in range(1000)])
```
initializes an empty model with a bit more than 100B parameters. Behind the scenes, this relies on the meta device introduced in PyTorch 1.9. During the initialization under the context manager, each time a parameter is created, it is instantly moved to that device.
You can't move a model initialized like this on CPU or another device directly, since it doesn't have any data. It's also very likely that a forward pass with that empty model will fail, as not all operations are supported on the meta device.
### Sharded checkpoints
It's possible your model is so big that even a single copy won't fit in RAM. That doesn't mean it can't be loaded: if you have one or several GPUs, this is more memory available to store your model. In this case, it's better if your checkpoint is split into several smaller files that we call checkpoint shards.
Accelerate will handle sharded checkpoints as long as you follow the following format: your checkpoint should be in a folder, with several files containing the partial state dicts, and there should be an index in the JSON format that contains a dictionary mapping parameter names to the file containing their weights. You can easily shard your model with `save_model()`. For instance, we could have a folder containing:
```bash
first_state_dict.bin
index.json
second_state_dict.bin
```
with index.json being the following file:
```
{
"linear1.weight": "first_state_dict.bin",
"linear1.bias": "first_state_dict.bin",
"linear2.weight": "second_state_dict.bin",
"linear2.bias": "second_state_dict.bin"
}
```
and `first_state_dict.bin` containing the weights for `"linear1.weight"` and `"linear1.bias"`, `second_state_dict.bin` the ones for `"linear2.weight"` and `"linear2.bias"`
### Loading weights
The second tool Accelerate introduces is a function `load_checkpoint_and_dispatch()`, that will allow you to load a checkpoint inside your empty model. This supports full checkpoints (a single file containing the whole state dict) as well as sharded checkpoints. It will also automatically dispatch those weights across the devices you have available (GPUs, CPU RAM), so if you are loading a sharded checkpoint, the maximum RAM usage will be the size of the biggest shard.
If you want to use big model inference with Transformers models, check out this [documentation](https://huggingface.co/docs/transformers/main/en/main_classes/model#large-model-loading).
Here is how we can use this to load the [GPT2-1.5B](https://huggingface.co/marcsun13/gpt2-xl-linear-sharded) model.
Let's download the sharded version of this model.
```bash
pip install huggingface_hub
```
```py
from huggingface_hub import snapshot_download
checkpoint = "marcsun13/gpt2-xl-linear-sharded"
weights_location = snapshot_download(repo_id=checkpoint)
```
In order to initialize the model, we will use the library minGPT.
```bash
git clone https://github.com/karpathy/minGPT.git
pip install minGPT/
```
```py
from accelerate import init_empty_weights
from mingpt.model import GPT
model_config = GPT.get_default_config()
model_config.model_type = 'gpt2-xl'
model_config.vocab_size = 50257
model_config.block_size = 1024
with init_empty_weights():
model = GPT(model_config)
```
Then, load the checkpoint we just downloaded with:
```py
from accelerate import load_checkpoint_and_dispatch
model = load_checkpoint_and_dispatch(
model, checkpoint=weights_location, device_map="auto", no_split_module_classes=['Block']
)
```
By passing `device_map="auto"`, we tell Accelerate to determine automatically where to put each layer of the model depending on the available resources:
- first, we use the maximum space available on the GPU(s)
- if we still need space, we store the remaining weights on the CPU
- if there is not enough RAM, we store the remaining weights on the hard drive as memory-mapped tensors
#### `no_split_module_classes`
This parameter will indicate that some of the modules with the name `"Block"` should not be split across different devices. You should set here all blocks that
include a residual connection of some kind.
#### The `device_map`
You can see the `device_map` that Accelerate picked by accessing the `hf_device_map` attribute of your model:
```py
model.hf_device_map
```
```python out
{'transformer.wte': 0,
'transformer.wpe': 0,
'transformer.drop': 0,
'transformer.h.0': 0,
...
'transformer.h.21': 0,
'transformer.h.22': 1,
'transformer.h.23': 1,
'transformer.h.24': 1,
...
'transformer.h.47': 1,
'transformer.ln_f': 1,
'lm_head': 1}
```
It's fully possible to create your own device map for the layers to use as well, specifying the GPU device to use (a number), `"cpu"`, or `"disk"` and pass this in:
```python
device_map = {
"transformer.wte": "cpu",
"transformer.wpe": 0,
"transformer.drop": "cpu",
"transformer.h.0": "disk"
}
model = load_checkpoint_and_dispatch(
model, checkpoint=weights_location, device_map=device_map
)
```
### Run the model
Now that we have done this, our model lies across several devices, and maybe the hard drive. But it can still be used as a regular PyTorch model:
```py
from mingpt.bpe import BPETokenizer
tokenizer = BPETokenizer()
inputs = tokenizer("Hello, my name is").to(0)
outputs = model.generate(x1, max_new_tokens=10, do_sample=False)[0]
tokenizer.decode(outputs.cpu().squeeze())
```
Behind the scenes, Accelerate added hooks to the model, so that:
- at each layer, the inputs are put on the right device (so even if your model is spread across several GPUs, it works)
- for the weights offloaded on the CPU, they are put on a GPU just before the forward pass and cleaned up just after
- for the weights offloaded on the hard drive, they are loaded in RAM then put on a GPU just before the forward pass and cleaned up just after
This way, your model can run for inference even if it doesn't fit on one of the GPUs or the CPU RAM!
This only supports the inference of your model, not training. Most of the computation happens behind `torch.no_grad()` context managers to avoid spending some GPU memory with intermediate activations.
### Designing a device map
You can let Accelerate handle the device map computation by setting `device_map` to one of the supported options (`"auto"`, `"balanced"`, `"balanced_low_0"`, `"sequential"`) or create one yourself if you want more control over where each layer should go.
You can derive all sizes of the model (and thus compute a `device_map`) on a model that is on the meta device.
All the options will produce the same result when you don't have enough GPU memory to accommodate the whole model (which is to fit everything that can on the GPU, then offload weights on the CPU or even on the disk if there is not enough RAM).
When you have more GPU memory available than the model size, here is the difference between each option:
- `"auto"` and `"balanced"` evenly split the model on all available GPUs, making it possible for you to use a batch size greater than 1.
- `"balanced_low_0"` evenly splits the model on all GPUs except the first one, and only puts on GPU 0 what does not fit on the others. This option is great when you need to use GPU 0 for some processing of the outputs, like when using the `generate` function for Transformers models
- `"sequential"` will fit what it can on GPU 0, then move on GPU 1 and so forth (so won't use the last GPUs if it doesn't need to).
The options `"auto"` and `"balanced"` produce the same results for now, but the behavior of `"auto"` might change in the future if we find a strategy that makes more sense, while `"balanced"` will stay stable.
First note that you can limit the memory used on each GPU by using the `max_memory` argument (available in `infer_auto_device_map()` and in all functions using it). When setting `max_memory`, you should pass along a dictionary containing the GPU identifiers (for instance `0`, `1` etc.) and the `"cpu"` key for the maximum RAM you want to use for CPU offload. The values can either be an integer (in bytes) or a string representing a number with its unit, such as `"10GiB"` or `"10GB"`.
Here is an example where we don't want to use more than 10GiB on each of the two GPUs and no more than 30GiB of CPU RAM for the model weights:
```python
from accelerate import infer_auto_device_map
device_map = infer_auto_device_map(my_model, max_memory={0: "10GiB", 1: "10GiB", "cpu": "30GiB"})
```
When a first allocation happens in PyTorch, it loads CUDA kernels which take about 1-2GB of memory depending on the GPU. Therefore you always have less usable memory than the actual size of the GPU. To see how much memory is actually used do `torch.ones(1).cuda()` and look at the memory usage.
Therefore when you create memory maps with `max_memory` make sure to adjust the available memory accordingly to avoid out-of-memory errors.
Additionally, if you do some additional operations with your outputs without placing them back on the CPU (for instance inside the `generate` method of Transformers) and if you placed your inputs on a GPU, that GPU will consume more memory than the others (Accelerate always place the output back to the device of the input). Therefore if you would like to optimize the maximum batch size and you have many GPUs, give the first GPU less memory. For example, with BLOOM-176B on 8x80 A100 setup, the close-to-ideal map is:
```python
max_memory = {0: "30GIB", 1: "46GIB", 2: "46GIB", 3: "46GIB", 4: "46GIB", 5: "46GIB", 6: "46GIB", 7: "46GIB"}
```
as you can see we gave the remaining 7 GPUs ~50% more memory than GPU 0.
If you opt to fully design the `device_map` yourself, it should be a dictionary with keys being module names of your model and values being a valid device identifier (for instance an integer for the GPUs) or `"cpu"` for CPU offload, `"disk"` for disk offload. The keys need to cover the whole model, you can then define your device map as you wish: for instance, if your model has two blocks (let's say `block1` and `block2`) which each contain three linear layers (let's say `linear1`, `linear2` and `linear3`), a valid device map can be:
```python
device_map = {"block1": 0, "block2": 1}
```
another one that is valid could be:
```python
device_map = {"block1": 0, "block2.linear1": 0, "block2.linear2": 1, "block2.linear3": 1}
```
On the other hand, this one is not valid as it does not cover every parameter of the model:
```python
device_map = {"block1": 0, "block2.linear1": 1, "block2.linear2": 1}
```
To be the most efficient, make sure your device map puts the parameters on the GPUs in a sequential manner (e.g. don't put one of the first weights on GPU 0, then weights on GPU 1 and the last weight back to GPU 0) to avoid making many transfers of data between the GPUs.
## CPU offload only
If you want to offload your model on CPU, you can use `cpu_offload()`. As a result, all parameters of the model will be offloaded and only one copy of the state dict of the model will be kept. During the forward pass, parameters will be extracted from that state dict and put on the execution device and passed as they are needed, then offloaded again.
```python
cpu_offload(model, execution_device)
```
You can also use `cpu_offload_with_hook()`. This function will offloads a model on the CPU and puts it back to an execution device when executed. The difference with `cpu_offload()` is that the model stays on the execution device after the forward and is only offloaded again when the `offload` method of the returned `hook` is called. Furthermore, `cpu_offload_with_hook()` is more performant but less memory saving. It is useful for pipelines running a model in a loop:
```python
model_1, hook_1 = cpu_offload_with_hook(model_1, execution_device)
model_2, hook_2 = cpu_offload_with_hook(model_2, execution_device, prev_module_hook=hook_1)
model_3, hook_3 = cpu_offload_with_hook(model_3, execution_device, prev_module_hook=hook_2)
hid_1 = model_1(input)
for i in range(50):
# model1 is offloaded on the CPU at the first iteration, model 2 stays on the GPU for this whole loop.
hid_2 = model_2(hid_1)
# model2 is offloaded to the CPU just before this forward.
hid_3 = model_3(hid_3)
# For model3, you need to manually call the hook offload method.
hook_3.offload()
```
## Disk offload only
To perform disk offload, you can use `disk_offload()`. As a result, all parameters of the model will be offloaded as memory-mapped array in a given folder. During the forward pass, parameters will be accessed from that folder and put on the execution device passed as they are needed, then offloaded again.
```python
disk_offload(model, offload_dir, execution_device)
```
## Limits and further development
We are aware of the current limitations in the API:
- `infer_auto_device_map()` (or `device_map="auto"` in `load_checkpoint_and_dispatch()`) tries to maximize GPU and CPU RAM it sees available when you execute it. While PyTorch is very good at managing GPU RAM efficiently (and giving it back when not needed), it's not entirely true with Python and CPU RAM. Therefore, an automatically computed device map might be too intense on the CPU. Move a few modules to the disk device if you get crashes due to a lack of RAM.
- `infer_auto_device_map()` (or `device_map="auto"` in `load_checkpoint_and_dispatch()`) attributes devices sequentially (to avoid moving things back and forth) so if your first layer is bigger than the size of the GPU you have, it will end up with everything on the CPU/Disk.
- `load_checkpoint_and_dispatch()` and `load_checkpoint_in_model()` do not perform any check on the correctness of your state dict compared to your model at the moment (this will be fixed in a future version), so you may get some weird errors if trying to load a checkpoint with mismatched or missing keys.
- The model parallelism used when your model is split on several GPUs is naive and not optimized, meaning that only one GPU works at a given time and the other sits idle.
- When weights are offloaded on the CPU/hard drive, there is no pre-fetching (yet, we will work on this for future versions) which means the weights are put on the GPU when they are needed and not before.
- Hard-drive offloading might be very slow if the hardware you run on does not have fast communication between disk and CPU (like NVMes).
# Training on TPUs
Training on TPUs can be slightly different from training on multi-gpu, even with Accelerate. This guide aims to show you
where you should be careful and why, as well as the best practices in general.
## Training in a Notebook
The main carepoint when training on TPUs comes from the `notebook_launcher()`. As mentioned in the [notebook tutorial](../usage_guides/notebook), you need to
restructure your training code into a function that can get passed to the `notebook_launcher()` function and be careful about not declaring any tensors on the GPU.
While on a TPU that last part is not as important, a critical part to understand is that when you launch code from a notebook you do so through a process called **forking**.
When launching from the command-line, you perform **spawning**, where a python process is not currently running and you *spawn* a new process in. Since your Jupyter notebook is already
utilizing a python process, you need to *fork* a new process from it to launch your code.
Where this becomes important is in regard to declaring your model. On forked TPU processes, it is recommended that you instantiate your model *once* and pass this into your
training function. This is different than training on GPUs where you create `n` models that have their gradients synced and back-propagated at certain moments. Instead, one
model instance is shared between all the nodes and it is passed back and forth. This is important especially when training on low-resource TPUs such as those provided in Kaggle kernels or
on Google Colaboratory.
Below is an example of a training function passed to the `notebook_launcher()` if training on CPUs or GPUs:
This code snippet is based off the one from the `simple_nlp_example` notebook found [here](https://github.com/huggingface/notebooks/blob/main/examples/accelerate_examples/simple_nlp_example.ipynb) with slight
modifications for the sake of simplicity
```python
def training_function():
# Initialize accelerator
accelerator = Accelerator()
model = AutoModelForSequenceClassification.from_pretrained("bert-base-cased", num_labels=2)
train_dataloader, eval_dataloader = create_dataloaders(
train_batch_size=hyperparameters["train_batch_size"], eval_batch_size=hyperparameters["eval_batch_size"]
)
# Instantiate optimizer
optimizer = AdamW(params=model.parameters(), lr=hyperparameters["learning_rate"])
# Prepare everything
# There is no specific order to remember, we just need to unpack the objects in the same order we gave them to the
# prepare method.
model, optimizer, train_dataloader, eval_dataloader = accelerator.prepare(
model, optimizer, train_dataloader, eval_dataloader
)
num_epochs = hyperparameters["num_epochs"]
# Now we train the model
for epoch in range(num_epochs):
model.train()
for step, batch in enumerate(train_dataloader):
outputs = model(**batch)
loss = outputs.loss
accelerator.backward(loss)
optimizer.step()
optimizer.zero_grad()
```
```python
from accelerate import notebook_launcher
notebook_launcher(training_function)
```
The `notebook_launcher` will default to 8 processes if Accelerate has been configured for a TPU
If you use this example and declare the model *inside* the training loop, then on a low-resource system you will potentially see an error
like:
```
ProcessExitedException: process 0 terminated with signal SIGSEGV
```
This error is *extremely* cryptic but the basic explanation is you ran out of system RAM. You can avoid this entirely by reconfiguring the training function to
accept a single `model` argument, and declare it in an outside cell:
```python
# In another Jupyter cell
model = AutoModelForSequenceClassification.from_pretrained("bert-base-cased", num_labels=2)
```
```diff
+ def training_function(model):
# Initialize accelerator
accelerator = Accelerator()
- model = AutoModelForSequenceClassification.from_pretrained("bert-base-cased", num_labels=2)
train_dataloader, eval_dataloader = create_dataloaders(
train_batch_size=hyperparameters["train_batch_size"], eval_batch_size=hyperparameters["eval_batch_size"]
)
...
```
And finally calling the training function with:
```diff
from accelerate import notebook_launcher
- notebook_launcher(training_function)
+ notebook_launcher(training_function, (model,))
```
The above workaround is only needed when launching a TPU instance from a Jupyter Notebook on a low-resource server such as Google Colaboratory or Kaggle. If
using a script or launching on a much beefier server declaring the model beforehand is not needed.
## Mixed Precision and Global Variables
As mentioned in the [mixed precision tutorial](../usage_guides/mixed_precision), Accelerate supports fp16 and bf16, both of which can be used on TPUs.
That being said, ideally `bf16` should be utilized as it is extremely efficient to use.
There are two "layers" when using `bf16` and Accelerate on TPUs, at the base level and at the operation level.
At the base level, this is enabled when passing `mixed_precision="bf16"` to `Accelerator`, such as:
```python
accelerator = Accelerator(mixed_precision="bf16")
```
By default, this will cast `torch.float` and `torch.double` to `bfloat16` on TPUs.
The specific configuration being set is an environmental variable of `XLA_USE_BF16` is set to `1`.
There is a further configuration you can perform which is setting the `XLA_DOWNCAST_BF16` environmental variable. If set to `1`, then
`torch.float` is `bfloat16` and `torch.double` is `float32`.
This is performed in the `Accelerator` object when passing `downcast_bf16=True`:
```python
accelerator = Accelerator(mixed_precision="bf16", downcast_bf16=True)
```
Using downcasting instead of bf16 everywhere is good for when you are trying to calculate metrics, log values, and more where raw bf16 tensors would be unusable.
## Training Times on TPUs
As you launch your script, you may notice that training seems exceptionally slow at first. This is because TPUs
first run through a few batches of data to see how much memory to allocate before finally utilizing this configured
memory allocation extremely efficiently.
If you notice that your evaluation code to calculate the metrics of your model takes longer due to a larger batch size being used,
it is recommended to keep the batch size the same as the training data if it is too slow. Otherwise the memory will reallocate to this
new batch size after the first few iterations.
Just because the memory is allocated does not mean it will be used or that the batch size will increase when going back to your training dataloader.
# Gradient synchronization
PyTorch's distributed module operates by communicating back and forth between all of the GPUs in your system.
This communication takes time, and ensuring all processes know the states of each other happens at particular triggerpoints
when using the `ddp` module.
These triggerpoints are added to the PyTorch model, specifically their `forward()` and `backward()` methods.
This happens when the model is wrapped with `DistributedDataParallel`:
```python
import torch.nn as nn
from torch.nn.parallel import DistributedDataParallel
model = nn.Linear(10, 10)
ddp_model = DistributedDataParallel(model)
```
In Accelerate this conversion happens automatically when calling `prepare()` and passing in your model.
```diff
+ from accelerate import Accelerator
+ accelerator = Accelerator()
import torch.nn as nn
- from torch.nn.parallel import DistributedDataParallel
model = nn.Linear(10,10)
+ model = accelerator.prepare(model)
```
## The slowdown in gradient accumulation
You now understand that PyTorch adds hooks to the `forward` and `backward` method of your PyTorch model when
training in a distributed setup. But how does this risk slowing down your code?
In DDP (distributed data parallel), the specific order in which processes are performed and ran are expected
at specific points and these must also occur at roughly the same time before moving on.
The most direct example is when you update model parameters through
`optimizer.step()`.
Without gradient accumulation, all instances of the model need to have updated
their gradients computed, collated, and updated before moving on to the next
batch of data.
When performing gradient accumulation, you accumulate `n` loss gradients and
skip `optimizer.step()` until `n` batches have been reached. As all training
processes only need to synchronize by the time `optimizer.step()` is called,
without any modification to your training step, this needless inter-process
communication can cause a significant slowdown.
How can you avoid this overhead?
## Solving the slowdown problem
Since you are skipping model parameter updates when training on these batches, their gradients do not need to be synchronized until the point where `optimizer.step()` is actually called.
PyTorch cannot automagically tell when you need to do this, but they do provide a tool to help through the [`no_sync`](https://pytorch.org/docs/stable/generated/torch.nn.parallel.DistributedDataParallel.html#torch.nn.parallel.DistributedDataParallel.no_sync) context manager
that is added to your model after converting it to DDP.
Under this context manager, PyTorch will skip synchronizing the gradients when
`.backward()` is called, and the first call to `.backward()` outside this
context manager will trigger the synchronization. See an example below:
```python
ddp_model, dataloader, optimizer = accelerator.prepare(model, dataloader, optimizer)
for index, batch in enumerate(dataloader):
inputs, targets = batch
# Trigger gradient synchronization on the last batch
if index != (len(dataloader) - 1):
with ddp_model.no_sync():
# Gradients only accumulate
outputs = ddp_model(inputs)
loss = loss_func(outputs)
accelerator.backward(loss)
else:
# Gradients finally sync
outputs = ddp_model(inputs)
loss = loss_func(outputs)
accelerator.backward(loss)
optimizer.step()
```
In Accelerate to make this an API that can be called no matter the training device (though it may not do anything if you are not in a distributed system!),
`ddp_model.no_sync` gets replaced with `no_sync()` and operates the same way:
```diff
ddp_model, dataloader, optimizer = accelerator.prepare(model, dataloader, optimizer)
for index, batch in enumerate(dataloader):
inputs, targets = batch
# Trigger gradient synchronization on the last batch
if index != (len(dataloader)-1):
- with ddp_model.no_sync():
+ with accelerator.no_sync(model):
# Gradients only accumulate
outputs = ddp_model(inputs)
loss = loss_func(outputs, targets)
accelerator.backward(loss)
else:
# Gradients finally sync
outputs = ddp_model(inputs)
loss = loss_func(outputs)
accelerator.backward(loss)
optimizer.step()
optimizer.zero_grad()
```
As you may expect, the `accumulate()` function wraps around this conditional check by keeping track of the current batch number, leaving you with the final
gradient accumulation API:
```python
ddp_model, dataloader, optimizer = accelerator.prepare(model, dataloader, optimizer)
for batch in dataloader:
with accelerator.accumulate(model):
optimizer.zero_grad()
inputs, targets = batch
outputs = model(inputs)
loss = loss_function(outputs, targets)
accelerator.backward(loss)
optimizer.step()
optimizer.zero_grad()
```
As a result, you should either use *`accelerator.accumulate` or `accelerator.no_sync`* when it comes to API choice.
## Just how much of a slowdown is there, and easy mistakes you can make
To set up a realistic example, consider the following setup:
* Two single-GPU T4 nodes and one node with two GPUs
* Each GPU is a T4, and are hosted on GCP
* The script used is a modification of the [NLP Example](https://github.com/muellerzr/timing_experiments/blob/main/baseline.py) script
* Batch size per GPU is 16, and gradients are accumulated every 4 steps
All scripts are available in [this repository](https://github.com/muellerzr/timing_experiments).
If not careful about gradient synchronization and GPU communication, a *large* amount of time can be wasted
from when these GPUs communicate to each other during unnecessary periods.
By how much?
Reference:
- Baseline: uses no synchronization practices discussed here
- `no_sync` improperly: `no_sync` only around the `backward` call, not the `forward`
- `no_sync`: using the `no_sync` pattern properly
- `accumulate`: using `accumulate()` properly
Below are the average seconds per batch iterating over 29 batches of data for each setup on both a single node and on the dual-node setup:
| | Baseline | `no_sync` improperly | `no_sync` | `accumulate`|
| :---------: | :-------: | :------------------: | :-------: | :---------: |
| Multi-Node | 2±0.01s | 2.13±0.08s | **0.91±0.11s** | **0.91±0.11s** |
| Single Node | 0.50±0.01s | 0.50±0.01s | **0.41±0.015s** | **0.41±0.015s** |
As you can see, if you are not careful about how you set up your gradient synchronization, you can get upwards of more than a 2x slowdown during training!
If you are worried about making sure everything is done properly, we highly recommend utilizing the `accumulate()` function and passing in
`gradient_accumulation_steps` or `gradient_accumulation_plugin` to the `Accelerator` object so Accelerate can handle this for you.
### `no_sync` requires additional GPU memory when using FSDP
Be aware that not syncing gradients can have adverse effects while performing FSDP training. As it has been warned in `torch`, the [`no_sync` context manager for FSDP](https://pytorch.org/docs/stable/fsdp.html#torch.distributed.fsdp.FullyShardedDataParallel.no_sync) will require additional memory.
Therefore in memory intensive situations while using FSDP, we recommend to set `sync_each_batch` to `True` in the `GradientAccumulationPlugin` to disable `no_sync`.
See the example below where we fine-tune Mixtral (47B parameters) on 8 A100-80GB GPUs. We see that even for a modest `gradient_accumulation_steps=2` we quickly go out-of-memory (OOM) if `no_sync` is enabled. Again, this is due to additional memory overheads due to FSDP's `no_sync`. However, if `no_sync` is disabled via `sync_each_batch=True`, then the memory consumption for `gradient_accumulation_steps=16` reverts to that of `gradient_accumulation_steps=1`.
| Model | `no_sync` (accum=1) | `no_sync` (accum=2) | `no_sync` disabled (accum=16)
| :-------------: | :-----------------: | :-----------------: | :-----------------:
mixtral 8x7B | 69G | OOM | 69G
> [!WARNING]
> Disabling `no_sync` means there _will be slowdown_ due the extra data syncs, as explained by the earlier sections of this guide.
# DExecuting and deferring jobs
When you run your usual script, instructions are executed in order. Using Accelerate to deploy your script on several
GPUs at the same time introduces a complication: while each process executes all instructions in order, some may be
faster than others.
You might need to wait for all processes to have reached a certain point before executing a given instruction. For
instance, you shouldn't save a model before being sure every process is done with training, and you wouldn't want to
continue training before all the model weights have been loaded in. To do this, just write the following line in your code:
```
accelerator.wait_for_everyone()
```
This instruction will block all the processes that arrive first until all the other processes have reached that
point (if you run your script on just one GPU or CPU, this won't do anything).
A few example cases of when to use this utility are listed below:
Some of these are utilized with the `main_process_first()` context manager, which utilizes `wait_for_everyone()` to
run a particular set of code on the main process beforehand before triggering and launching the other processes
## Downloading a Dataset
When downloading a dataset, you should download it first on the main process and then load the cached dataset afterward
`load_dataset` will perform a lock under the hood to stop multiple downloads from happening at once, but if you are downloading something
not using this library you should use this method.
```python
with accelerator.main_process_first():
datasets = load_dataset("glue", "mrpc")
```
Under the hood this is the same as calling:
```python
# First do something on the main process
if accelerator.is_main_process:
datasets = load_dataset("glue", "mrpc")
else:
accelerator.wait_for_everyone()
# And then send it to the rest of them
if not accelerator.is_main_process:
datasets = load_dataset("glue", "mrpc")
else:
accelerator.wait_for_everyone()
```
## Saving the `state_dict`
When saving the `state_dict` of the model, since you would normally save one file on just the main process
you should specify that:
```python
if accelerator.is_main_process:
model = accelerator.unwrap_model(model)
torch.save(model.state_dict(), "weights.pth")
```
## Loading in the `state_dict`
When loading in the `state_dict` to a model, optimizer, or scheduler, you should wait
for all workers to have the weights loaded in before moving on to training
```python
with accelerator.main_process_first():
state = torch.load("weights.pth")
model.load_state_dict(state)
```
## Applying a multi-worker CPU operation
Applying a `map()` operation on multiple workers, such as tokenizing should be done on the
main process first, and then propagated to each one.
```python
datasets = load_dataset("glue", "mrpc")
with accelerator.main_process_first():
tokenized_datasets = datasets.map(
tokenize_function,
batched=True,
remove_columns=["idx", "sentence1", "sentence2"],
)
```
## Applying checks such as Early Stopping
To have a check that works with a flag set by a particular process, the `set_trigger` and `check_trigger` API should be used. Useful examples
for doing so can include situations such as using early stopping and monitoring the loss (as each loss slightly differs on each process).
Call `Accelerator.set_trigger()` when your condition has been met, and `Accelerator.check_trigger()` when checking if that condition has been met in any process:
```python
for (x,y) in data_loader:
logits = model(x)
loss = loss_func(logits, y)
# Assume `should_do_early_stopping` is a custom defined function that returns a conditional
if should_do_early_stopping(loss):
accelerator.set_trigger()
# Later in the training script when we need to check for the breakpoint
if accelerator.check_trigger():
break
```
# Comparing performance across distributed setups
Evaluating and comparing the performance from different setups can be quite tricky if you don't know what to look for.
For example, you cannot run the same script with the same batch size across TPU, multi-GPU, and single-GPU with Accelerate
and expect your results to line up.
But why?
There are three reasons for this that this tutorial will cover:
1. **Setting the right seeds**
2. **Observed Batch Sizes**
3. **Learning Rates**
## Setting the Seed
While this issue has not come up as much, make sure to use `utils.set_seed()` to fully set the seed in all distributed cases so training will be reproducible:
```python
from accelerate.utils import set_seed
set_seed(42)
```
Why is this important? Under the hood this will set **5** different seed settings:
```python
random.seed(seed)
np.random.seed(seed)
torch.manual_seed(seed)
torch.cuda.manual_seed_all(seed)
# ^^ safe to call this function even if cuda is not available
if is_torch_xla_available():
xm.set_rng_state(seed)
```
The random state, numpy's state, torch, torch's cuda state, and if TPUs are available torch_xla's cuda state.
## Observed Batch Sizes
When training with Accelerate, the batch size passed to the dataloader is the **batch size per GPU**. What this entails is
a batch size of 64 on two GPUs is truly a batch size of 128. As a result, when testing on a single GPU this needs to be accounted for,
as well as similarly for TPUs.
The below table can be used as a quick reference to try out different batch sizes:
In this example, there are two GPUs for "Multi-GPU" and a TPU pod with 8 workers
| Single GPU Batch Size | Multi-GPU Equivalent Batch Size | TPU Equivalent Batch Size |
|-----------------------|---------------------------------|---------------------------|
| 256 | 128 | 32 |
| 128 | 64 | 16 |
| 64 | 32 | 8 |
| 32 | 16 | 4 |
## Learning Rates
As noted in multiple sources[[1](https://aws.amazon.com/blogs/machine-learning/scalable-multi-node-deep-learning-training-using-gpus-in-the-aws-cloud/)][[2](https://docs.nvidia.com/clara/clara-train-sdk/pt/model.html#classification-models-multi-gpu-training)], the learning rate should be scaled *linearly* based on the number of devices present. The below
snippet shows doing so with Accelerate:
Since users can have their own learning rate schedulers defined, we leave this up to the user to decide if they wish to scale their
learning rate or not.
```python
learning_rate = 1e-3
accelerator = Accelerator()
learning_rate *= accelerator.num_processes
optimizer = AdamW(params=model.parameters(), lr=learning_rate)
```
You will also find that `accelerate` will step the learning rate based on the number of processes being trained on. This is because
of the observed batch size noted earlier. So in the case of 2 GPUs, the learning rate will be stepped twice as often as a single GPU
to account for the batch size being twice as large (if no changes to the batch size on the single GPU instance are made).
## Gradient Accumulation and Mixed Precision
When using gradient accumulation and mixed precision, due to how gradient averaging works (accumulation) and the precision loss (mixed precision),
some degradation in performance is expected. This will be explicitly seen when comparing the batch-wise loss between different compute
setups. However, the overall loss, metric, and general performance at the end of training should be _roughly_ the same.
# Low precision training methods
The release of new kinds of hardware led to the emergence of new training paradigms that better utilize them. Currently, this is in the form of training
in 8-bit precision using packages such as [TransformersEngine](https://github.com/NVIDIA/TransformerEngine) (TE) or [MS-AMP](https://github.com/Azure/MS-AMP/tree/main).
For an introduction to the topics discussed today, we recommend reviewing the [low-precision usage guide](../usage_guides/low_precision_training) as this documentation will reference it regularly.
## A Quick Chart
Below is a quick chart from the MS-AMP documentation showing the different bit-precisions for each solution during training:
Optimization Level | Computation(GEMM) | Comm | Weight | Master Weight | Weight Gradient | Optimizer States
-- | -- | -- | -- | -- | -- | --
FP16 AMP | FP16 | FP32 | FP32 | N/A | FP32 | FP32+FP32
Nvidia TE | FP8 | FP32 | FP32 | N/A | FP32 | FP32+FP32
MS-AMP O1 | FP8 | FP8 | FP16 | N/A | FP8 | FP32+FP32
MS-AMP O2 | FP8 | FP8 | FP16 | N/A | FP8 | FP8+FP16
MS-AMP O3 | FP8 | FP8 | FP8 | FP16 | FP8 | FP8+FP16
## `TransformersEngine`
`TransformersEngine` is the first solution to trying to train in 8-bit floating point. It works by using drop-in replacement layers for certain ones in a model that utilizes their FP8-engine to reduce the number of bits (such as 32 to 8) without degrading the final accuracy of the model.
Specifically, Accelerate will find and replace the following layers with `TransformersEngine` versions:
* `nn.LayerNorm` for `te.LayerNorm`
* `nn.Linear` for `te.Linear`
As a result we wind up with a model that has most of its layers in BF16, while some layers are in FP8 reducing some of the memory.
Anecdotally, we have noticed that performance gains don't really start showing when using `TransformerEngine` until a large majority of the layers
in the model are made up of those two layers to replace. As a result, only larger models have shown performance improvements when the number of parameters is around and upwards of a few billion.
The `TransformerEngine` can receive many different arguments that customize how it performs FP8 calculations and what they do. A full list of the arguments is available below:
* `margin`: The margin to use for the gradient scaling.
* `interval`: The interval to use for how often the scaling factor is recomputed.
* `fp8_format``: The format to use for the FP8 recipe. Must be one of `HYBRID` or `E4M3`. (Generally `HYBRID` for training, `E4M3` for evaluation)
* `amax_history_len`: The length of the history to use for the scaling factor computation
* `amax_compute_algo`: The algorithm to use for the scaling factor computation. Must be one of `max` or `most_recent`.
* `override_linear_precision`: Whether or not to execute `fprop`, `dgrad`, and `wgrad` GEMMS in higher precision.
You can customize each of these as part of `utils.FP8RecipeKwargs` to help optimize performance of your models.
If we notice in the chart mentioned earlier, TE simply casts the computation layers into FP8, while everything else is in FP32. As a result this winds up utilizing the most memory but does so with the benefit of guaranteeing the least amount of loss in end accuracy during training.
## `MS-AMP`
MS-AMP takes a different approach to `TransformersEngine` by providing three different optimization levels to convert more operations in FP8 or FP16.
* The base optimization level (`O1`), passes communications of the weights (such as in DDP) in FP8, stores the weights of the model in FP16, and leaves the optimizer states in FP32. The main benefit of this optimization level is that we can reduce the communication bandwidth by essentially half. Additionally, more GPU memory is saved due to 1/2 of everything being cast in FP8, and the weights being cast to FP16. Notably, both the optimizer states remain in FP32.
* The second optimization level (`O2`) improves upon this by also reducing the precision of the optimizer states. One is in FP8 while the other is in FP16. Generally it's been shown that this will only provide a net-gain of no degraded end accuracy, increased training speed, and reduced memory as now every state is either in FP16 or FP8.
* Finally, MS-AMP has a third optimization level (`O3`) which helps during DDP scenarios such as DeepSpeed. The weights of the model in memory are fully cast to FP8, and the master weights are now stored in FP16. This fully reduces memory by the highest factor as now not only is almost everything in FP8, only two states are left in FP16. Currently, only DeepSpeed versions up through 0.9.2 are supported, so this capability is not included in the Accelerate integration
## Combining the two
More experiments need to be performed but it's been noted that combining both MS-AMP and TransformersEngine can lead to the highest throughput by relying on NVIDIA's optimized FP8 operators and utilizing how MS-AMP reduces the memory overhead.
# FSDP vs DeepSpeed
Accelerate offers flexibilty of training frameworks, by integrating two extremely powerful tools for distributed training, namely [Pytorch FSDP](../usage_guides/fsdp) and [Microsoft DeepSpeed](../usage_guides/deepspeed). The aim of this tutorial is to draw parallels, as well as to outline potential differences, to empower the user to switch seamlessly between these two frameworks.
To switch between the frameworks, we recommend launching code `accelerate launch` passing in the correct config file with `--config_file`, or passing in the respective arguments directly for [FSDP and DeepSpeed](../package_reference/cli#accelerate-launch) .
Example Accelerate configurations can be found here for [DeepSpeed](../usage_guides/deepspeed#accelerate-deepspeed-plugin) and [FSDP](../usage_guides/fsdp#how-it-works-out-of-the-box), or in the [example zoo under "Launch Configurations"](../usage_guides/explore)
This tutorial is for single-node, multi-GPU, scenarios only.
## Configuring Functionalities
Model tensors are split into different GPUs in an attempt to scale up model sizes; this is termed *sharding* in FSDP, and *partitioning* in DeepSpeed. FSDP sharding and DeepSpeed ZeRO (partitioning) stages are configured by `--fsdp_sharding_strategy`, and `--zero_stage`, respectively. In particular, FSDP `FULL_SHARD` maps to DeepSpeed ZeRO stage `3`; see this [comprehensive mapping between FSDP sharding and DeepSpeed ZeRO settings](../usage_guides/fsdp#mapping-between-fsdp-sharding-strategies-and-deepspeed-zero-stages). The below table summarizes and groups similar settings:
Group | Framework | Configuration | Example | Restrictions (if any)
--|--|--|--|--
sharding / partitioning | FSDP
DeepSpeed | `--fsdp_sharding_strategy`
`--zero_stage` | `1` (`FULL_SHARD`)
`3` |
offload | FSDP
DeepSpeed | `--fsdp_offload_params`
`--offload_param_device`
`--offload_optimizer_device` | `true`
`cpu`
`cpu` | all or nothing
model loading | FSDP
DeepSpeed | `--fsdp_cpu_ram_efficient_loading`
`--zero3_init_flag` | `true`
`true` |
only ZeRO 3
efficient checkpointing | FSDP
DeepSpeed | `--fsdp_state_dict_type`
`--zero3_save_16bit_model` | `SHARDED_STATE_DICT`
`true` |
only ZeRO 3
weights prefetching | FSDP
DeepSpeed | `--fsdp_forward_prefetch`
`--fsdp_backward_prefetch`
None | `true`
`BACKWARD_PRE` |
model | FSDP
DeepSpeed | `--fsdp_auto_wrap_policy`
`--fsdp_transformer_layer_cls_to_wrap`
None | `TRANSFORMER_BASED_WRAP`
|
Usually not needed
Transparent to user.
parameters summoning | FSDP
DeepSpeed | `--fsdp_use_orig_params`
None | `true` | required for `torch.compile`
Transparent to user
parameters syncing | FSDP
DeepSpeed | `--fsdp_sync_module_states`
None | `true` |
training | FSDP
DeepSpeed | None
`--gradient_accumulation_steps`
`--gradient_clipping` |
`auto`
`auto` | Transparent to user
For detailed descriptions of the above, refer to [`Accelerate` launch documentation](../package_reference/cli#accelerate-launch).
To access other DeepSpeed configurations, such as mixed precision settings,
you need to pass in a `--deepspeed_config_file`, see the [documentation](../usage_guides/deepspeed#deepspeed-config-file).
DeepSpeed can be also configured via `DeepSpeedPlugin`, e.g., `DeepSpeedPlugin.zero_stage` is equivalent of `--zero_stage`, and `DeepSpeedPlugin.hf_ds_config` can be used to pass `--deepeed_config_file.`
FSDP can be also configured via `FullyShardedDataParallelPlugin`, e.g., `FullyShardedDataParallelPlugin.sharding_strategy` is equivalent of `--fsdp_sharding_strategy`.
### Checkpointing
Do note that while FSDP can be configured via `--fsdp_state_dict_type` to save either full / sharded checkpoints.
For DeepSpeed Zero3, one could pass a `--zero3_save_16bit_model true`, which conveniently consolidates the model to a single rank and saves; this is the FSDP equivalent of `fsdp_state_dict_type: FULL_STATE_DICT`.
For large models, consolidating the model to a single rank can be very slow.
For quicker checkpointing, for FSDP use `fsdp_state_dict_type: SHARDED_STATE_DICT`, and for DeepSpeed Zero3 [use the `zero_to_fp32.py` script to post-convert sharded checkpoints](https://www.deepspeed.ai/tutorials/zero/#extracting-weights).
### Offloading
FSDP only allows *all-or-nothing* offload (i.e., either offload parameters, gradients, and optimizer, or keep them all in GPU), but DeepSpeed can offload parameters and optimizer differently. Furthermore, DeepSpeed also supports [offloading to NVME](https://www.deepspeed.ai/docs/config-json/#parameter-offloading).
### Prefetching
FSDP allows two prefetching configurations `--fsdp_forward_prefetch` and `--fsdp_backward_prefetch` to improve overlap of comms / computation at a cost of extra memory, see [FSDP documentation](https://pytorch.org/docs/stable/fsdp.html).
For DeepSpeed, the prefetching will be turned on when needed, and it turns on depending on certain hyper-params like `stage3_param_persistence_threshold`, `stage3_max_reuse_distance`, etc, [that can be configured for Zero3](https://www.deepspeed.ai/docs/config-json/#parameter-offloading); `accelerate` may set these hyper-params automatically if you don't set those explicitly in the deepspeed config file.
For FSDP set `fsdp_backward_prefetch: BACKWARD_PRE` for improved throughputs if memory allows.
### Model Loading
While FSDP require an explicit `--fsdp_cpu_ram_efficient_loading true` to activate efficient model loading, `transformers` will activate the similar feature whenever DeepSpeed Zero3 is used.
For FSDP, whenever setting `--fsdp_cpu_ram_efficient_loading true`, `accelerate` will automatically set `sync_module_states` to true.
For RAM efficient loading the weights will be loaded only in a singe rank, and thus requires `sync_module_states` to broadcast weights to other ranks.
### Model
FSDP requires an explicit `--fsdp_auto_wrap_policy` for the algorithm to decide how to schedule the all-gather and reduce-scatter operations. But for DeepSpeed this is transparent to the user.
For FSDP, simply set `fsdp_auto_wrap_policy: TRANSFORMER_BASED_WRAP`. With the latest `transformers` versions, we try our best to figure out the suitable `fsdp_transformer_layer_cls_to_wrap` for HF transformers models. However, if you get an error regarding it, please specify this.
### Parameters Summoning
FSDP requires an explicit `--fsdp_use_orig_params` flag if using `torch.compile`, see [the pytorch documenation](https://pytorch.org/docs/stable/fsdp.html#module-torch.distributed.fsdp). For DeepSpeed this is transparent to the user.
For FSDP, when using `torch.compile` please set `fsdp_use_orig_params: True`.
## Training
Deepspeed requires explicit `--gradient_accumulation_steps` and `--gradient_clipping` flags. For FSDP this is transparent to the user.
When using DeepSpeed, set `gradient_accumulation_steps: "auto"` and `gradient_clipping: "auto"` to automatically pick up values set in the `Accelerator` or `TrainingArguments` (if using `transformers`).
## On Differences in Data Precision Handling
To discuss the how data precision is handled in both FSDP and Deepspeed, it is instructive to first give an overview of how model parameters are handled in these frameworks. Before the model / optimizer parameters are distributed across GPUs, parameter preparation is involved to first "flatten" them to one-dimensional [`torch.Tensor`](https://pytorch.org/docs/stable/tensors.html#torch-tensor). The implementation of FSDP / DeepSpeed varies in the respect of the `dtype` in which these "flattened" parameters are stored, and there are ramifications with regards to how [`torch.Optimizer`](https://pytorch.org/docs/stable/optim.html#module-torch.optim) allocate their `dtype`s. The table below outlines the processes for both frameworks; the "Local" column indicates the process occurring at a per-gpu level, therefore any memory overheads by upcasting should be understood to be amortized by the number of gpus used.
As a rule of thumb, for stable training with automatic mixed precision, all the trainable parameters have to be in `torch.float32`.
Process | Local | Framework | Details
--|--|--|--
Loading, i.e., `AutoModel.from_pretrained(..., torch_dtype=torch_dtype)` |
Preparation, i.e., creation of "flat params" | ✅ | FSDP
DeepSpeed | created in `torch_dtype`.
disregards `torch_dtype`, created in `float32`.
Optimizer initialization | ✅ | FSDP
DeepSpeed | creates parameters in `torch_dtype`
creates parameters in `float32`
Training Step, i.e, forward, backward, reduction | | FSDP
DeepSpeed | follows [`MixedPrecision`](https://pytorch.org/docs/stable/fsdp.html#torch.distributed.fsdp.MixedPrecision)
follows `deepspeed_config_file` mixed precision settings.
Optimizer (Pre-Step) | ✅ | FSDP
DeepSpeed | upcasting (if any) to `torch_dtype`
upcasted to `float32`
Optimizer (Actual Step) | ✅ | FSDP
DeepSpeed | occurs in `torch_dtype`
occurs in `float32`.
Therefore when using DeepSpeed a small number of GPUs, be aware of potentially significant memory overheads due to the upcasting during preperation.
With FSDP, in the absence of mixed precision, it is possible to operate the [`torch.Optimizer`](https://pytorch.org/docs/stable/optim.html#module-torch.optim) in low precision `torch_dtype`, which may be helpful when using small number of GPUs.
With mixed precision, FSDP and DeepSpeed will upcast in the model preparation step (c.f. table above). But do note that FSDP will then save checkpoints in the upcasted precision; Deepspeed may still save low precision checkpoints if `--zero3_save_16bit_model` is specified.
To clarify the above table consider the concrete examples below; the optimizer pre- and actual step combined for brevity. With FSDP it is possible to operate in the two modes shown below, but DeepSpeed can only operate in one.
Framework | Model Loading (`torch_dtype`) | Mixed Precision | Preparation (Local) | Training | Optimizer (Local)
--|--|--|--|--|--
FSDP | bf16 | default (none) | bf16 | bf16 | bf16
FSDP | bf16 | bf16 | fp32 | bf16 | fp32
DeepSpeed | bf16 | bf16 | fp32 | bf16 | fp32
# Accelerate's internal mechanisms
Internally, Accelerate works by first analyzing the environment in which the script is launched to determine which
kind of distributed setup is used, how many different processes there are and which one the current script is in. All
that information is stored in the `~AcceleratorState`.
This class is initialized the first time you instantiate an `~Accelerator` as well as performing any
specific initialization your distributed setup needs. Its state is then uniquely shared through all instances of
`AcceleratorState`. (The same can also be done with the `PartialState`, a more barebones version it inherits)
Then, when calling `prepare()`, the library:
- wraps your model(s) in the container adapted for the distributed setup,
- wraps your optimizer(s) in an `AcceleratedOptimizer`,
- wraps your scheduler(s) in an `AcceleratedScheduler`
- creates a new version of your dataloader(s) in a `DataLoaderShard` or `DataLoaderDispatcher`
While the model(s), optimizer(s), and scheduler(s) are just put in simple wrappers, the dataloader(s) are re-created. This is mostly
because PyTorch does not let the user change the `batch_sampler` of a dataloader once it's been created and the
library handles the sharding of your data between processes by changing that `batch_sampler` to yield every other
`num_processes` batches (if enabled).
The `DataLoaderShard` subclasses `DataLoader` to add the following functionality:
- it synchronizes the appropriate random number generator of all processes at each new iteration, to ensure any
randomization (like shuffling) is done the exact same way across processes.
- it puts the batches on the proper device before yielding them (unless you have opted out of
`device_placement=True`).
The `DataLoaderDispatcher` subclasses differs from the `DataLoaderShard` in that when iterating through the `DataLoader`, the data is all starting from process 0 and *then* split and sent off to each process rather than it happening at the dataset level.
The random number generator synchronization will by default synchronize:
- the `generator` attribute of a given sampler (like the PyTorch `RandomSampler`) for PyTorch >= 1.6
- the main random number generator in PyTorch <=1.5.1
You can choose which random number generator(s) to synchronize with the `rng_types` argument of the main
`Accelerator`. In PyTorch >= 1.6, it is recommended to rely on a local `generator` to avoid
setting the same seed in the main random number generator in all processes.
Synchronization of the main torch (or CUDA or XLA) random number generator will affect any other potential random
artifacts you could have in your dataset (like random data augmentation) in the sense that all processes will get
the same random numbers from the torch random modules (so will apply the same random data augmentation if it's
controlled by torch).
The randomization part of your custom sampler, batch sampler or iterable dataset should be done using a local
`torch.Generator` object (in PyTorch >= 1.6), see the traditional `RandomSampler`, as an example.
If you have [`torchdata>=0.8.0`](https://github.com/pytorch/data/tree/main) installed, and you have passed `use_stateful_dataloader=True` into your `DataLoaderConfiguration`, these classes will directly inherit from `StatefulDataLoader` instead, and maintain a `state_dict`.
For more details about the internals, see the [Internals page](package_reference/torch_wrappers).
# Add Accelerate to your code
Each distributed training framework has their own way of doing things which can require writing a lot of custom code to adapt it to your PyTorch training code and training environment. Accelerate offers a friendly way to interface with these distributed training frameworks without having to learn the specific details of each one. Accelerate takes care of those details for you, so you can focus on the training code and scale it to any distributed training environment.
In this tutorial, you'll learn how to adapt your existing PyTorch code with Accelerate and get you on your way toward training on distributed systems with ease! You'll start with a basic PyTorch training loop (it assumes all the training objects like `model` and `optimizer` have been setup already) and progressively integrate Accelerate into it.
```python
device = "cuda"
model.to(device)
for batch in training_dataloader:
optimizer.zero_grad()
inputs, targets = batch
inputs = inputs.to(device)
targets = targets.to(device)
outputs = model(inputs)
loss = loss_function(outputs, targets)
loss.backward()
optimizer.step()
scheduler.step()
```
## Accelerator
The `Accelerator` is the main class for adapting your code to work with Accelerate. It knows about the distributed setup you're using such as the number of different processes and your hardware type. This class also provides access to many of the necessary methods for enabling your PyTorch code to work in any distributed training environment and for managing and executing processes across devices.
That's why you should always start by importing and creating an `Accelerator` instance in your script.
```python
from accelerate import Accelerator
accelerator = Accelerator()
```
The `Accelerator` also knows which device to move your PyTorch objects to, so it is recommended to let Accelerate handle this for you.
```diff
- device = "cuda"
+ device = accelerator.device
model.to(device)
```
## Prepare PyTorch objects
Next, you need to prepare your PyTorch objects (model, optimizer, scheduler, etc.) for distributed training. The `prepare()` method takes care of placing your model in the appropriate container (like single GPU or multi-GPU) for your training setup, adapting the optimizer and scheduler to use Accelerate's `AcceleratedOptimizer` and `AcceleratedScheduler`, and creating a new dataloader that can be sharded across processes.
> [!TIP]
> Accelerate only prepares objects that inherit from their respective PyTorch classes such as `torch.optim.Optimizer`.
The PyTorch objects are returned in the same order they're sent.
```py
model, optimizer, training_dataloader, scheduler = accelerator.prepare(
model, optimizer, training_dataloader, scheduler
)
```
## Training loop
Finally, remove the `to(device)` calls to the inputs and targets in the training loop because Accelerate's DataLoader classes automatically places them on the right device. You should also replace the usual `backward()` pass with Accelerate's `backward()` method which scales the gradients for you and uses the appropriate `backward()` method depending on your distributed setup (for example, DeepSpeed or Megatron).
```diff
- inputs = inputs.to(device)
- targets = targets.to(device)
outputs = model(inputs)
loss = loss_function(outputs, targets)
- loss.backward()
+ accelerator.backward(loss)
```
Put everything together and your new Accelerate training loop should now look like this!
```python
from accelerate import Accelerator
accelerator = Accelerator()
device = accelerator.device
model, optimizer, training_dataloader, scheduler = accelerator.prepare(
model, optimizer, training_dataloader, scheduler
)
for batch in training_dataloader:
optimizer.zero_grad()
inputs, targets = batch
outputs = model(inputs)
loss = loss_function(outputs, targets)
accelerator.backward(loss)
optimizer.step()
scheduler.step()
```
## Training features
Accelerate offers additional features - like gradient accumulation, gradient clipping, mixed precision training and more - you can add to your script to improve your training run. Let's explore these three features.
### Gradient accumulation
Gradient accumulation enables you to train on larger batch sizes by accumulating the gradients over multiple batches before updating the weights. This can be useful for getting around memory limitations. To enable this feature in Accelerate, specify the `gradient_accumulation_steps` parameter in the `Accelerator` class and add the `accumulate()` context manager to your script.
```diff
+ accelerator = Accelerator(gradient_accumulation_steps=2)
model, optimizer, training_dataloader = accelerator.prepare(model, optimizer, training_dataloader)
for input, label in training_dataloader:
+ with accelerator.accumulate(model):
predictions = model(input)
loss = loss_function(predictions, label)
accelerator.backward(loss)
optimizer.step()
scheduler.step()
optimizer.zero_grad()
```
### Gradient clipping
Gradient clipping is a technique to prevent "exploding gradients", and Accelerate offers:
* `clip_grad_value_()` to clip gradients to a minimum and maximum value
* `clip_grad_norm_()` for normalizing gradients to a certain value
### Mixed precision
Mixed precision accelerates training by using a lower precision data type like fp16 (half-precision) to calculate the gradients. For the best performance with Accelerate, the loss should be computed inside your model (like in Transformers models) because computations outside of the model are computed in full precision.
Set the mixed precision type to use in the `Accelerator`, and then use the `autocast()` context manager to automatically cast the values to the specified data type.
> [!WARNING]
> Accelerate enables automatic mixed precision, so `autocast()` is only needed if there are other mixed precision operations besides those performed on loss by `backward()` which already handles the scaling.
```diff
+ accelerator = Accelerator(mixed_precision="fp16")
+ with accelerator.autocast():
loss = complex_loss_function(outputs, target):
```
## Save and load
Accelerate can also save and load a *model* once training is complete or you can also save the model and optimizer *state* which could be useful for resuming training.
### Model
Once all processes are complete, unwrap the model with the `unwrap_model()` method before saving it because the `prepare()` method wrapped your model into the proper interface for distributed training. If you don't unwrap the model, saving the model state dictionary also saves any potential extra layers from the larger model and you won't be able to load the weights back into your base model.
You should use the `save_model()` method to unwrap and save the model state dictionary. This method can also save a model into sharded checkpoints or into the [safetensors](https://hf.co/docs/safetensors/index) format.
```py
accelerator.wait_for_everyone()
accelerator.save_model(model, save_directory)
```
For models from the [Transformers](https://hf.co/docs/transformers/index) library, save the model with the [save_pretrained](https://huggingface.co/docs/transformers/main/en/main_classes/model#transformers.PreTrainedModel.save_pretrained) method so that it can be reloaded with the [from_pretrained](https://huggingface.co/docs/transformers/main/en/main_classes/model#transformers.PreTrainedModel.from_pretrained) method.
```py
from transformers import AutoModel
unwrapped_model = accelerator.unwrap_model(model)
unwrapped_model.save_pretrained(
"path/to/my_model_directory",
is_main_process=accelerator.is_main_process,
save_function=accelerator.save,
)
model = AutoModel.from_pretrained("path/to/my_model_directory")
```
To load your weights, use the `unwrap_model()` method to unwrap the model first before loading the weights. All model parameters are references to tensors, so this loads your weights inside `model`.
```py
unwrapped_model = accelerator.unwrap_model(model)
path_to_checkpoint = os.path.join(save_directory,"pytorch_model.bin")
unwrapped_model.load_state_dict(torch.load(path_to_checkpoint))
```
Set `safe_serialization=True` to save the model in the safetensor format.
```py
accelerator.wait_for_everyone()
accelerator.save_model(model, save_directory, max_shard_size="1GB", safe_serialization=True)
```
To load a sharded checkpoint or a safetensor formatted checkpoint, use the `load_checkpoint_in_model()` method. This method allows you to load a checkpoint onto a specific device.
```py
load_checkpoint_in_model(unwrapped_model, save_directory, device_map={"":device})
```
### State
During training, you may want to save the current state of the model, optimizer, random generators, and potentially learning rate schedulers so they can be restored in the *same script*. You should add the `save_state()` and `load_state()` methods to your script to save and load states.
To further customize where and how states are saved through `save_state()`, use the `ProjectConfiguration` class. For example, if `automatic_checkpoint_naming` is enabled, each saved checkpoint is stored at `Accelerator.project_dir/checkpoints/checkpoint_{checkpoint_number}`.
Any other stateful items to be stored should be registered with the `register_for_checkpointing()` method so they can be saved and loaded. Every object passed to this method to be stored must have a `load_state_dict` and `state_dict` function.
> [!TIP]
> If you have [`torchdata>=0.8.0`](https://github.com/pytorch/data/tree/main) installed, you can additionally pass `use_stateful_dataloader=True` into your `DataLoaderConfiguration`. This extends Accelerate's DataLoader classes with a `load_state_dict` and `state_dict` function, and makes it so `Accelerator.save_state` and `Accelerator.load_state` also track how far into the training dataset it has read when persisting the model.
# Launching Accelerate scripts
In the previous tutorial, you were introduced to how to modify your current training script to use Accelerate.
The final version of that code is shown below:
```python
from accelerate import Accelerator
accelerator = Accelerator()
model, optimizer, training_dataloader, scheduler = accelerator.prepare(
model, optimizer, training_dataloader, scheduler
)
for batch in training_dataloader:
optimizer.zero_grad()
inputs, targets = batch
outputs = model(inputs)
loss = loss_function(outputs, targets)
accelerator.backward(loss)
optimizer.step()
scheduler.step()
```
But how do you run this code and have it utilize the special hardware available to it?
First, you should rewrite the above code into a function, and make it callable as a script. For example:
```diff
from accelerate import Accelerator
+ def main():
accelerator = Accelerator()
model, optimizer, training_dataloader, scheduler = accelerator.prepare(
model, optimizer, training_dataloader, scheduler
)
for batch in training_dataloader:
optimizer.zero_grad()
inputs, targets = batch
outputs = model(inputs)
loss = loss_function(outputs, targets)
accelerator.backward(loss)
optimizer.step()
scheduler.step()
+ if __name__ == "__main__":
+ main()
```
Next, you need to launch it with `accelerate launch`.
It's recommended you run `accelerate config` before using `accelerate launch` to configure your environment to your liking.
Otherwise Accelerate will use very basic defaults depending on your system setup.
## Using accelerate launch
Accelerate has a special CLI command to help you launch your code in your system through `accelerate launch`.
This command wraps around all of the different commands needed to launch your script on various platforms, without you having to remember what each of them is.
If you are familiar with launching scripts in PyTorch yourself such as with `torchrun`, you can still do this. It is not required to use `accelerate launch`.
You can launch your script quickly by using:
```bash
accelerate launch {script_name.py} --arg1 --arg2 ...
```
Just put `accelerate launch` at the start of your command, and pass in additional arguments and parameters to your script afterward like normal!
Since this runs the various torch spawn methods, all of the expected environment variables can be modified here as well.
For example, here is how to use `accelerate launch` with a single GPU:
```bash
# for cuda device:
CUDA_VISIBLE_DEVICES="0" accelerate launch {script_name.py} --arg1 --arg2 ...
# for xpu device:
ZE_AFFINITY_MASK="0" accelerate launch {script_name.py} --arg1 --arg2 ...
```
You can also use `accelerate launch` without performing `accelerate config` first, but you may need to manually pass in the right configuration parameters.
In this case, Accelerate will make some hyperparameter decisions for you, e.g., if GPUs are available, it will use all of them by default without the mixed precision.
Here is how you would use all GPUs and train with mixed precision disabled:
```bash
accelerate launch --multi_gpu {script_name.py} {--arg1} {--arg2} ...
```
Or by specifying a number of GPUs to use:
```bash
accelerate launch --num_processes=2 {script_name.py} {--arg1} {--arg2} ...
```
To get more specific you should pass in the needed parameters yourself. For instance, here is how you
would also launch that same script on two GPUs using mixed precision while avoiding all of the warnings:
```bash
accelerate launch --multi_gpu --mixed_precision=fp16 --num_processes=2 {script_name.py} {--arg1} {--arg2} ...
```
For a complete list of parameters you can pass in, run:
```bash
accelerate launch -h
```
Even if you are not using Accelerate in your code, you can still use the launcher for starting your scripts!
For a visualization of this difference, that earlier `accelerate launch` on multi-gpu would look something like so with `torchrun`:
```bash
MIXED_PRECISION="fp16" torchrun --nproc_per_node=2 --nnodes=1 {script_name.py} {--arg1} {--arg2} ...
```
You can also launch your script utilizing the launch CLI as a python module itself, enabling the ability to pass in other python-specific
launching behaviors. To do so, use `accelerate.commands.launch` instead of `accelerate launch`:
```bash
python -m accelerate.commands.launch --num_processes=2 {script_name.py} {--arg1} {--arg2}
```
If you want to execute the script with any other python flags, you can pass them in as well similar to `-m`, such as
the below example enabling unbuffered stdout and stderr:
```bash
python -u -m accelerate.commands.launch --num_processes=2 {script_name.py} {--arg1} {--arg2}
```
You can run your code on CPU as well! This is helpful for debugging and testing purposes on toy models and datasets.
```bash
accelerate launch --cpu {script_name.py} {--arg1} {--arg2}
```
## Why you should always use `accelerate config`
Why is it useful to the point you should **always** run `accelerate config`?
Remember that earlier call to `accelerate launch` as well as `torchrun`?
Post configuration, to run that script with the needed parts you just need to use `accelerate launch` outright, without passing anything else in:
```bash
accelerate launch {script_name.py} {--arg1} {--arg2} ...
```
## Custom Configurations
As briefly mentioned earlier, `accelerate launch` should be mostly used through combining set configurations
made with the `accelerate config` command. These configs are saved to a `default_config.yaml` file in your cache folder for Accelerate.
This cache folder is located at (with decreasing order of priority):
- The content of your environment variable `HF_HOME` suffixed with `accelerate`.
- If it does not exist, the content of your environment variable `XDG_CACHE_HOME` suffixed with
`huggingface/accelerate`.
- If this does not exist either, the folder `~/.cache/huggingface/accelerate`.
To have multiple configurations, the flag `--config_file` can be passed to the `accelerate launch` command paired
with the location of the custom yaml.
An example yaml may look something like the following for two GPUs on a single machine using `fp16` for mixed precision:
```yaml
compute_environment: LOCAL_MACHINE
deepspeed_config: {}
distributed_type: MULTI_GPU
fsdp_config: {}
machine_rank: 0
main_process_ip: null
main_process_port: null
main_training_function: main
mixed_precision: fp16
num_machines: 1
num_processes: 2
use_cpu: false
```
Launching a script from the location of that custom yaml file looks like the following:
```bash
accelerate launch --config_file {path/to/config/my_config_file.yaml} {script_name.py} {--arg1} {--arg2} ...
```
## Multi-node training
Multi-node training with Accelerate is similar to [multi-node training with torchrun](https://pytorch.org/tutorials/intermediate/ddp_series_multinode.html). The simplest way to launch a multi-node training run is to do the following:
- Copy your codebase and data to all nodes. (or place them on a shared filesystem)
- Setup your python packages on all nodes.
- Run `accelerate config` on the main single node first. After specifying the number of nodes, you will be asked to specify the rank of each node (this will be 0 for the main/master node), along with the IP address and port for the main process. This is required for the worker nodes to communicate with the main process. Afterwards, you can copy or send this config file across all of your nodes, changing the `machine_rank` to 1, 2,3, etc. to avoid having to run the command (or just follow their directions directly for launching with `torchrun` as well)
Once you have done this, you can start your multi-node training run by running `accelerate launch` (or `torchrun`) on all nodes.
It is required that the command be ran on all nodes for everything to start, not just running it from the main node. You can use something like SLURM or a different process executor to wrap around this requirement and call everything from a single command.
It is recommended to use the intranet IP of your main node over the public IP for better latency. This is the `192.168.x.x` or the `172.x.x.x` address you see when you run `hostname -I` on the main node.
To get a better idea about multi-node training, check out our example for [multi-node training with FSDP](https://huggingface.co/blog/ram-efficient-pytorch-fsdp).
# Execution process
When working with distributed training systems, it is important to manage how and when processes are executed across GPUs. Some processes are completed faster than others, and some processes shouldn't begin if others haven't finished yet. Accelerate provides tools for orchestrating when processes are executed to ensure everything remains synchronized across all devices.
This tutorial will teach you how to execute a process on only one machine and how to delay execution until all processes have reached a certain point.
## Execute on one process
Certain code only needs to be run once on a given machine, such as printing a log statement or only displaying one progress bar on the local main process.
You should use `accelerator.is_local_main_process` to indicate code that should only be executed once.
```py
from tqdm.auto import tqdm
progress_bar = tqdm(range(args.max_train_steps), disable=not accelerator.is_local_main_process)
```
You could also wrap a statement with `accelerator.is_local_main_process`.
> [!TIP]
> For standalone `print` statements that aren't wrapped in `accelerator.is_local_main_process`, replace `print` with Accelerate's `print()` method to only print once per process.
```py
if accelerator.is_local_main_process:
print("Accelerate is the best")
```
For a function that should only be executed once, use `on_local_main_process()`.
```py
@accelerator.on_local_main_process
def do_my_thing():
"Something done once per server"
do_thing_once_per_server()
```
You could also direct Accelerate to execute code once across *all processes* regardless of the number of machines. This is useful if you're uploading a final model to the Hub.
You should use `accelerator.is_main_process` to indicate code that should only be executed once across all processes.
```py
if accelerator.is_main_process:
repo.push_to_hub()
```
For a function that should only be executed once across all processes, use `on_main_process()`.
```py
@accelerator.on_main_process
def do_my_thing():
"Something done once per server"
do_thing_once()
```
## Execute on a specific process
Accelerate can also help you execute functions that should only be executed on a specific process or a local process index.
Use the `on_process()` method and specify the process index to execute a function on.
```py
@accelerator.on_process(process_index=0)
def do_my_thing():
"Something done on process index 0"
do_thing_on_index_zero()
```
Use the `on_local_process()` method and specify the local process index to execute a function on.
```py
@accelerator.on_local_process(local_process_idx=0)
def do_my_thing():
"Something done on process index 0 on each server"
do_thing_on_index_zero_on_each_server()
```
## Defer execution
When you run your script on several GPUs at the same time, some code may be executed faster than others. You might need to wait for all processes to reach a certain point before executing the next set of instructions. For instance, you shouldn’t save a model before making sure every process is done with training.
To do this, add `wait_for_everyone()` in your code. This blocks all processes that have finished first from continuing until all remaining processes have reached the same point (this has no effect if you're running on a single GPU or CPU).
```py
accelerator.wait_for_everyone()
```
# Launching distributed training from Jupyter Notebooks
This tutorial teaches you how to fine tune a computer vision model with 🤗 Accelerate from a Jupyter Notebook on a distributed system.
You will also learn how to setup a few requirements needed for ensuring your environment is configured properly, your data has been prepared properly, and finally how to launch training.
This tutorial is also available as a Jupyter Notebook [here](https://github.com/huggingface/notebooks/blob/main/examples/accelerate_examples/simple_cv_example.ipynb)
## Configuring the Environment
Before any training can be performed, a Accelerate config file must exist in the system. Usually this can be done by running the following in a terminal and answering the prompts:
```bash
accelerate config
```
However, if general defaults are fine and you are *not* running on a TPU, Accelerate has a utility to quickly write your GPU configuration into a config file via `utils.write_basic_config()`.
The following code will restart Jupyter after writing the configuration, as CUDA code was called to perform this.
CUDA can't be initialized more than once on a multi-GPU system. It's fine to debug in the notebook and have calls to CUDA, but in order to finally train a full cleanup and restart will need to be performed.
```python
import os
from accelerate.utils import write_basic_config
write_basic_config() # Write a config file
os._exit(00) # Restart the notebook
```
## Preparing the Dataset and Model
Next you should prepare your dataset. As mentioned at earlier, great care should be taken when preparing the `DataLoaders` and model to make sure that **nothing** is put on *any* GPU.
If you do, it is recommended to put that specific code into a function and call that from within the notebook launcher interface, which will be shown later.
Make sure the dataset is downloaded based on the directions [here](https://github.com/huggingface/accelerate/tree/main/examples#simple-vision-example)
```python
import os, re, torch, PIL
import numpy as np
from torch.optim.lr_scheduler import OneCycleLR
from torch.utils.data import DataLoader, Dataset
from torchvision.transforms import Compose, RandomResizedCrop, Resize, ToTensor
from accelerate import Accelerator
from accelerate.utils import set_seed
from timm import create_model
```
First you need to create a function to extract the class name based on a filename:
```python
import os
data_dir = "../../images"
fnames = os.listdir(data_dir)
fname = fnames[0]
print(fname)
```
```python out
beagle_32.jpg
```
In the case here, the label is `beagle`. Using regex you can extract the label from the filename:
```python
import re
def extract_label(fname):
stem = fname.split(os.path.sep)[-1]
return re.search(r"^(.*)_\d+\.jpg$", stem).groups()[0]
```
```python
extract_label(fname)
```
And you can see it properly returned the right name for our file:
```python out
"beagle"
```
Next a `Dataset` class should be made to handle grabbing the image and the label:
```python
class PetsDataset(Dataset):
def __init__(self, file_names, image_transform=None, label_to_id=None):
self.file_names = file_names
self.image_transform = image_transform
self.label_to_id = label_to_id
def __len__(self):
return len(self.file_names)
def __getitem__(self, idx):
fname = self.file_names[idx]
raw_image = PIL.Image.open(fname)
image = raw_image.convert("RGB")
if self.image_transform is not None:
image = self.image_transform(image)
label = extract_label(fname)
if self.label_to_id is not None:
label = self.label_to_id[label]
return {"image": image, "label": label}
```
Now to build the dataset. Outside the training function you can find and declare all the filenames and labels and use them as references inside the
launched function:
```python
fnames = [os.path.join("../../images", fname) for fname in fnames if fname.endswith(".jpg")]
```
Next gather all the labels:
```python
all_labels = [extract_label(fname) for fname in fnames]
id_to_label = list(set(all_labels))
id_to_label.sort()
label_to_id = {lbl: i for i, lbl in enumerate(id_to_label)}
```
Next, you should make a `get_dataloaders` function that will return your built dataloaders for you. As mentioned earlier, if data is automatically
sent to the GPU or a TPU device when building your `DataLoaders`, they must be built using this method.
```python
def get_dataloaders(batch_size: int = 64):
"Builds a set of dataloaders with a batch_size"
random_perm = np.random.permutation(len(fnames))
cut = int(0.8 * len(fnames))
train_split = random_perm[:cut]
eval_split = random_perm[cut:]
# For training a simple RandomResizedCrop will be used
train_tfm = Compose([RandomResizedCrop((224, 224), scale=(0.5, 1.0)), ToTensor()])
train_dataset = PetsDataset([fnames[i] for i in train_split], image_transform=train_tfm, label_to_id=label_to_id)
# For evaluation a deterministic Resize will be used
eval_tfm = Compose([Resize((224, 224)), ToTensor()])
eval_dataset = PetsDataset([fnames[i] for i in eval_split], image_transform=eval_tfm, label_to_id=label_to_id)
# Instantiate dataloaders
train_dataloader = DataLoader(train_dataset, shuffle=True, batch_size=batch_size, num_workers=4)
eval_dataloader = DataLoader(eval_dataset, shuffle=False, batch_size=batch_size * 2, num_workers=4)
return train_dataloader, eval_dataloader
```
Finally, you should import the scheduler to be used later:
```python
from torch.optim.lr_scheduler import CosineAnnealingLR
```
## Writing the Training Function
Now you can build the training loop. `notebook_launcher()` works by passing in a function to call that will be ran across the distributed system.
Here is a basic training loop for the animal classification problem:
The code has been split up to allow for explanations on each section. A full version that can be copy and pasted will be available at the end
```python
def training_loop(mixed_precision="fp16", seed: int = 42, batch_size: int = 64):
set_seed(seed)
accelerator = Accelerator(mixed_precision=mixed_precision)
```
First you should set the seed and create an `Accelerator` object as early in the training loop as possible.
If training on the TPU, your training loop should take in the model as a parameter and it should be instantiated
outside of the training loop function. See the [TPU best practices](../concept_guides/training_tpu)
to learn why
Next you should build your dataloaders and create your model:
```python
train_dataloader, eval_dataloader = get_dataloaders(batch_size)
model = create_model("resnet50d", pretrained=True, num_classes=len(label_to_id))
```
You build the model here so that the seed also controls the new weight initialization
As you are performing transfer learning in this example, the encoder of the model starts out frozen so the head of the model can be
trained only initially:
```python
for param in model.parameters():
param.requires_grad = False
for param in model.get_classifier().parameters():
param.requires_grad = True
```
Normalizing the batches of images will make training a little faster:
```python
mean = torch.tensor(model.default_cfg["mean"])[None, :, None, None]
std = torch.tensor(model.default_cfg["std"])[None, :, None, None]
```
To make these constants available on the active device, you should set it to the Accelerator's device:
```python
mean = mean.to(accelerator.device)
std = std.to(accelerator.device)
```
Next instantiate the rest of the PyTorch classes used for training:
```python
optimizer = torch.optim.Adam(params=model.parameters(), lr=3e-2 / 25)
lr_scheduler = OneCycleLR(optimizer=optimizer, max_lr=3e-2, epochs=5, steps_per_epoch=len(train_dataloader))
```
Before passing everything to `prepare()`.
There is no specific order to remember, you just need to unpack the objects in the same order you gave them to the prepare method.
```python
model, optimizer, train_dataloader, eval_dataloader, lr_scheduler = accelerator.prepare(
model, optimizer, train_dataloader, eval_dataloader, lr_scheduler
)
```
Now train the model:
```python
for epoch in range(5):
model.train()
for batch in train_dataloader:
inputs = (batch["image"] - mean) / std
outputs = model(inputs)
loss = torch.nn.functional.cross_entropy(outputs, batch["label"])
accelerator.backward(loss)
optimizer.step()
lr_scheduler.step()
optimizer.zero_grad()
```
The evaluation loop will look slightly different compared to the training loop. The number of elements passed as well as the overall
total accuracy of each batch will be added to two constants:
```python
model.eval()
accurate = 0
num_elems = 0
```
Next you have the rest of your standard PyTorch loop:
```python
for batch in eval_dataloader:
inputs = (batch["image"] - mean) / std
with torch.no_grad():
outputs = model(inputs)
predictions = outputs.argmax(dim=-1)
```
Before finally the last major difference.
When performing distributed evaluation, the predictions and labels need to be passed through
`gather()` so that all of the data is available on the current device and a properly calculated metric can be achieved:
```python
accurate_preds = accelerator.gather(predictions) == accelerator.gather(batch["label"])
num_elems += accurate_preds.shape[0]
accurate += accurate_preds.long().sum()
```
Now you just need to calculate the actual metric for this problem, and you can print it on the main process using `print()`:
```python
eval_metric = accurate.item() / num_elems
accelerator.print(f"epoch {epoch}: {100 * eval_metric:.2f}")
```
A full version of this training loop is available below:
```python
def training_loop(mixed_precision="fp16", seed: int = 42, batch_size: int = 64):
set_seed(seed)
# Initialize accelerator
accelerator = Accelerator(mixed_precision=mixed_precision)
# Build dataloaders
train_dataloader, eval_dataloader = get_dataloaders(batch_size)
# Instantiate the model (you build the model here so that the seed also controls new weight initaliziations)
model = create_model("resnet50d", pretrained=True, num_classes=len(label_to_id))
# Freeze the base model
for param in model.parameters():
param.requires_grad = False
for param in model.get_classifier().parameters():
param.requires_grad = True
# You can normalize the batches of images to be a bit faster
mean = torch.tensor(model.default_cfg["mean"])[None, :, None, None]
std = torch.tensor(model.default_cfg["std"])[None, :, None, None]
# To make these constants available on the active device, set it to the accelerator device
mean = mean.to(accelerator.device)
std = std.to(accelerator.device)
# Instantiate the optimizer
optimizer = torch.optim.Adam(params=model.parameters(), lr=3e-2 / 25)
# Instantiate the learning rate scheduler
lr_scheduler = OneCycleLR(optimizer=optimizer, max_lr=3e-2, epochs=5, steps_per_epoch=len(train_dataloader))
# Prepare everything
# There is no specific order to remember, you just need to unpack the objects in the same order you gave them to the
# prepare method.
model, optimizer, train_dataloader, eval_dataloader, lr_scheduler = accelerator.prepare(
model, optimizer, train_dataloader, eval_dataloader, lr_scheduler
)
# Now you train the model
for epoch in range(5):
model.train()
for batch in train_dataloader:
inputs = (batch["image"] - mean) / std
outputs = model(inputs)
loss = torch.nn.functional.cross_entropy(outputs, batch["label"])
accelerator.backward(loss)
optimizer.step()
lr_scheduler.step()
optimizer.zero_grad()
model.eval()
accurate = 0
num_elems = 0
for batch in eval_dataloader:
inputs = (batch["image"] - mean) / std
with torch.no_grad():
outputs = model(inputs)
predictions = outputs.argmax(dim=-1)
accurate_preds = accelerator.gather(predictions) == accelerator.gather(batch["label"])
num_elems += accurate_preds.shape[0]
accurate += accurate_preds.long().sum()
eval_metric = accurate.item() / num_elems
# Use accelerator.print to print only on the main process.
accelerator.print(f"epoch {epoch}: {100 * eval_metric:.2f}")
```
## Using the notebook_launcher
All that's left is to use the `notebook_launcher()`.
You pass in the function, the arguments (as a tuple), and the number of processes to train on. (See the [documentation](../package_reference/launchers) for more information)
```python
from accelerate import notebook_launcher
```
```python
args = ("fp16", 42, 64)
notebook_launcher(training_loop, args, num_processes=2)
```
In the case of running on multiple nodes, you need to set up a Jupyter session at each node and run the launching cell at the same time.
For an environment containing 2 nodes (computers) with 8 GPUs each and the main computer with an IP address of "172.31.43.8", it would look like so:
```python
notebook_launcher(training_loop, args, master_addr="172.31.43.8", node_rank=0, num_nodes=2, num_processes=8)
```
And in the second Jupyter session on the other machine:
Notice how the `node_rank` has changed
```python
notebook_launcher(training_loop, args, master_addr="172.31.43.8", node_rank=1, num_nodes=2, num_processes=8)
```
In the case of running on the TPU, it would look like so:
```python
model = create_model("resnet50d", pretrained=True, num_classes=len(label_to_id))
args = (model, "fp16", 42, 64)
notebook_launcher(training_loop, args, num_processes=8)
```
To launch the training process with elasticity, enabling fault tolerance, you can use the `elastic_launch` feature provided by PyTorch. This requires setting additional parameters such as `rdzv_backend` and `max_restarts`. Here is an example of how to use `notebook_launcher` with elastic capabilities:
```python
notebook_launcher(
training_loop,
args,
num_processes=2,
max_restarts=3
)
```
As it's running it will print the progress as well as state how many devices you ran on. This tutorial was ran with two GPUs:
```python out
Launching training on 2 GPUs.
epoch 0: 88.12
epoch 1: 91.73
epoch 2: 92.58
epoch 3: 93.90
epoch 4: 94.71
```
And that's it!
Please note that `notebook_launcher()` ignores the Accelerate config file, to launch based on the config use:
```bash
accelerate launch
```
## Debugging
A common issue when running the `notebook_launcher` is receiving a CUDA has already been initialized issue. This usually stems
from an import or prior code in the notebook that makes a call to the PyTorch `torch.cuda` sublibrary. To help narrow down what went wrong,
you can launch the `notebook_launcher` with `ACCELERATE_DEBUG_MODE=yes` in your environment and an additional check
will be made when spawning that a regular process can be created and utilize CUDA without issue. (Your CUDA code can still be ran afterwards).
## Conclusion
This notebook showed how to perform distributed training from inside of a Jupyter Notebook. Some key notes to remember:
- Make sure to save any code that use CUDA (or CUDA imports) for the function passed to `notebook_launcher()`
- Set the `num_processes` to be the number of devices used for training (such as number of GPUs, CPUs, TPUs, etc)
- If using the TPU, declare your model outside the training loop function
# Overview
Welcome to the Accelerate tutorials! These introductory guides will help catch you up to speed on working with Accelerate.
You'll learn how to modify your code to have it work with the API seamlessly, how to launch your script properly,
and more!
These tutorials assume some basic knowledge of Python and familiarity with the PyTorch framework.
If you have any questions about Accelerate, feel free to join and ask the community on our [forum](/static-proxy?url=https%3A%2F%2Fdiscuss.huggingface.co%2Fc%2Faccelerate%2F18).
# TPU training
A [TPU (Tensor Processing Unit)](https://cloud.google.com/tpu/docs/intro-to-tpu) is a type of hardware specifically designed for training models efficiently. Accelerate supports TPU training, but there are a few things you should be aware of, namely graph compilation. This tutorial briefly discusses compilation, and for more details, take a look at the [Training on TPUs with Accelerate](../concept_guides/training_tpu) guide.
## Compilation
A TPU creates a graph of all the operations in the training step such as the forward pass, backward pass and optimizer step. This is why the first training step always takes a while because building and compiling this graph takes time. But once compilation is complete, it is cached and all subsequent steps are much faster.
The key is to avoid compiling your code again or else training is super slow. This means all your operations must be exactly the same:
* all tensors in your batches must have the same length (for example, no dynamic padding for NLP tasks)
* your code must be static (for example, no layers with for loops that have different lengths depending on the input such as a LSTM)
## Weight tying
A common language model design is to tie the weights of the embedding and softmax layers. However, moving the model to a TPU (either yourself or passing it to the `prepare()` method) breaks the weight tying and you'll need to retie the weights.
To add special behavior (like weight tying) in your script for TPUs, set `distributed_type` to `DistributedType.TPU` first. Then you can use the [tie_weights](https://huggingface.co/docs/transformers/main/en/main_classes/model#transformers.PreTrainedModel.tie_weights) method to tie the weights.
```py
if accelerator.distributed_type == DistributedType.TPU:
model.tie_weights()
```
# Troubleshoot
This guide provides solutions to some issues you might encounter when using Accelerate. Not all errors are covered because Accelerate is an active library that is continuously evolving and there are many different use cases and distributed training setups. If the solutions described here don't help with your specific error, please take a look at the [Ask for help](#ask-for-help) section to learn where and how to get help.
## Logging
Logging can help you identify where an error is coming from. In a distributed setup with multiple processes, logging can be a challenge, but Accelerate provides the `logging()` utility to ensure logs are synchronized.
To troubleshoot an issue, use `logging()` instead of the standard Python [`logging`](https://docs.python.org/3/library/logging.html#module-logging) module. Set the verbosity level (`INFO`, `DEBUG`, `WARNING`, `ERROR`, `CRITICAL`) with the `log_level` parameter, and then you can either:
1. Export the `log_level` as the `ACCELERATE_LOG_LEVEL` environment variable.
2. Pass the `log_level` directly to `get_logger`.
For example, to set `log_level="INFO"`:
```py
from accelerate.logging import get_logger
logger = get_logger(__name__, log_level="DEBUG")
```
By default, the log is called on main processes only. To call it on all processes, pass `main_process_only=False`.
If a log should be called on all processes and in order, also pass `in_order=True`.
```py
from accelerate.logging import get_logger
logger = get_logger(__name__, log_level="DEBUG")
# log all processes
logger.debug("thing_to_log", main_process_only=False)
# log all processes in order
logger.debug("thing_to_log", main_process_only=False, in_order=True)
```
## Hanging code and timeout errors
There can be many reasons why your code is hanging. Let's take a look at how to solve some of the most common issues that can cause your code to hang.
### Mismatched tensor shapes
Mismatched tensor shapes is a common issue that can cause your code to hang for a significant amount of time on a distributed setup.
When running scripts in a distributed setup, functions such as `Accelerator.gather()` and `Accelerator.reduce()` are necessary to grab tensors across devices to collectively perform operations on them. These (and other) functions rely on `torch.distributed` to perform a `gather` operation, which requires tensors to have the **exact same shape** across all processes. When the tensor shapes don't match, your code hangs and you'll eventually hit a timeout exception.
You can use Accelerate's operational debug mode to immediately catch this issue. We recommend enabling this mode during the `accelerate config` setup, but you can also enable it from the CLI, as an environment variable, or by manually editing the `config.yaml` file.
```bash
accelerate launch --debug {my_script.py} --arg1 --arg2
```
If enabling debug mode as an environment variable, you don't need to call `accelerate launch`.
```bash
ACCELERATE_DEBUG_MODE="1" torchrun {my_script.py} --arg1 --arg2
```
Add `debug: true` to your `config.yaml` file.
```yaml
compute_environment: LOCAL_MACHINE
debug: true
```
Once you enable debug mode, you should get a traceback that points to the tensor shape mismatch issue.
```py
Traceback (most recent call last):
File "/home/zach_mueller_huggingface_co/test.py", line 18, in
main()
File "/home/zach_mueller_huggingface_co/test.py", line 15, in main
broadcast_tensor = broadcast(tensor)
File "/home/zach_mueller_huggingface_co/accelerate/src/accelerate/utils/operations.py", line 303, in wrapper
accelerate.utils.operations.DistributedOperationException:
Cannot apply desired operation due to shape mismatches. All shapes across devices must be valid.
Operation: `accelerate.utils.operations.broadcast`
Input shapes:
- Process 0: [1, 5]
- Process 1: [1, 2, 5]
```
### Early stopping
For early stopping in distributed training, if each process has a specific stopping condition (e.g. validation loss), it may not be synchronized across all processes. As a result, a break can happen on process 0 but not on process 1 which will cause your code to hang indefinitely until a timeout occurs.
If you have early stopping conditionals, use the `set_breakpoint` and `check_breakpoint` methods to make sure all the processes
are ended correctly.
```py
# Assume `should_do_breakpoint` is a custom defined function that returns a conditional,
# and that conditional might be true only on process 1
if should_do_breakpoint(loss):
accelerator.set_breakpoint()
# Later in the training script when we need to check for the breakpoint
if accelerator.check_breakpoint():
break
```
### Low kernel versions on Linux
On Linux with kernel version < 5.5, hanging processes have been reported. To avoid this problem, upgrade your system to a later kernel version.
### MPI
If your distributed CPU training job using MPI is hanging, ensure that you have
[passwordless SSH](https://www.open-mpi.org/faq/?category=rsh#ssh-keys) setup (using keys) between the nodes. This means
that for all nodes in your hostfile, you should to be able to SSH from one node to another without being prompted for a password.
Next, try to run the `mpirun` command as a sanity check. For example, the command below should print out the
hostnames for each of the nodes.
```bash
mpirun -f hostfile -n {number of nodes} -ppn 1 hostname
```
## CUDA Out-of-Memory
One of the most frustrating errors when it comes to running training scripts is hitting "CUDA Out-of-Memory". The entire script needs to be restarted and any progress is lost.
To address this problem, Accelerate provides the `find_executable_batch_size()` utility that is heavily based on [toma](https://github.com/BlackHC/toma).
This utility retries code that fails due to OOM (out-of-memory) conditions and automatically lowers batch sizes. For each OOM condition, the algorithm decreases the batch size by half and retries the code until it succeeds.
To use `find_executable_batch_size()`, restructure your training function to include an inner function with `find_executable_batch_size` and build your dataloaders inside it. At a minimum, this only takes 4 new lines of code.
The inner function **must** take batch size as the first parameter, but we do not pass one to it when called. The wrapper will handles this for you. Any object (models, optimizers) that consumes CUDA memory and is passed to the `Accelerator` also **must** be declared inside the inner function.
```diff
def training_function(args):
accelerator = Accelerator()
+ @find_executable_batch_size(starting_batch_size=args.batch_size)
+ def inner_training_loop(batch_size):
+ nonlocal accelerator # Ensure they can be used in our context
+ accelerator.free_memory() # Free all lingering references
model = get_model()
model.to(accelerator.device)
optimizer = get_optimizer()
train_dataloader, eval_dataloader = get_dataloaders(accelerator, batch_size)
lr_scheduler = get_scheduler(
optimizer,
num_training_steps=len(train_dataloader)*num_epochs
)
model, optimizer, train_dataloader, eval_dataloader, lr_scheduler = accelerator.prepare(
model, optimizer, train_dataloader, eval_dataloader, lr_scheduler
)
train(model, optimizer, train_dataloader, lr_scheduler)
validate(model, eval_dataloader)
+ inner_training_loop()
```
## Non-reproducible results between device setups
If you changed the device setup and observe different model performance, it is likely you didn't update your script when moving from one setup to another. Even if you're using the same script with the same batch size, the results will still be different on a TPU, multi-GPU, and single GPU.
For example, if you were training on a single GPU with a batch size of 16 and you move to a dual GPU setup, you need to change the batch size to 8 to have the same effective batch size. This is because when training with Accelerate, the batch size passed to the dataloader is the **batch size per GPU**.
To make sure you can reproduce the results between the setups, make sure to use the same seed, adjust the batch size accordingly, and consider scaling the learning rate.
For more details and a quick reference for batch sizes, check out the [Comparing performance between different device setups](../concept_guides/performance) guide.
## Performance issues on different GPUs
If your multi-GPU setup consists of different GPUs, you may encounter some performance issues:
- There may be an imbalance in GPU memory between the GPUs. In this case, the GPU with the smaller memory will limit the batch size or the size of the model that can be loaded onto the GPUs.
- If you are using GPUs with different performance profiles, the performance will be driven by the slowest GPU you are using because the other GPUs will have to wait for it to complete its workload.
Vastly different GPUs within the same setup can lead to performance bottlenecks.
## Ask for help
If none of the solutions and advice here helped resolve your issue, you can always reach out to the community and Accelerate team for help.
- Ask for help on the Hugging Face forums by posting your question in the [Accelerate category](/static-proxy?url=https%3A%2F%2Fdiscuss.huggingface.co%2Fc%2Faccelerate%2F18). Make sure to write a descriptive post with relevant context about your setup and reproducible code to maximize the likelihood that your problem is solved!
- Post a question on [Discord](http://hf.co/join/discord), and let the team and the community help you.
- Create an Issue on the Accelerate [GitHub repository](https://github.com/huggingface/accelerate/issues) if you think you've found a bug related to the library. Include context regarding the bug and details about your distributed setup to help us better figure out what's wrong and how we can fix it.
# Installation
Before you start, you will need to setup your environment, install the appropriate packages, and configure Accelerate. Accelerate is tested on **Python 3.8+**.
Accelerate is available on pypi and conda, as well as on GitHub. Details to install from each are below:
## pip
To install Accelerate from pypi, perform:
```bash
pip install accelerate
```
## conda
Accelerate can also be installed with conda with:
```bash
conda install -c conda-forge accelerate
```
## Source
New features are added every day that haven't been released yet. To try them out yourself, install
from the GitHub repository:
```bash
pip install git+https://github.com/huggingface/accelerate
```
If you're working on contributing to the library or wish to play with the source code and see live
results as you run the code, an editable version can be installed from a locally-cloned version of the
repository:
```bash
git clone https://github.com/huggingface/accelerate
cd accelerate
pip install -e .
```
## Configuration
After installing, you need to configure Accelerate for how the current system is setup for training.
To do so run the following and answer the questions prompted to you:
```bash
accelerate config
```
To write a barebones configuration that doesn't include options such as DeepSpeed configuration or running on TPUs, you can quickly run:
```bash
python -c "from accelerate.utils import write_basic_config; write_basic_config(mixed_precision='fp16')"
```
Accelerate will automatically utilize the maximum number of GPUs available and set the mixed precision mode.
To check that your configuration looks fine, run:
```bash
accelerate env
```
An example output is shown below, which describes two GPUs on a single machine with no mixed precision being used:
```bash
- `Accelerate` version: 0.11.0.dev0
- Platform: Linux-5.10.0-15-cloud-amd64-x86_64-with-debian-11.3
- Python version: 3.7.12
- Numpy version: 1.19.5
- PyTorch version (GPU?): 1.12.0+cu102 (True)
- `Accelerate` default config:
- compute_environment: LOCAL_MACHINE
- distributed_type: MULTI_GPU
- mixed_precision: no
- use_cpu: False
- num_processes: 2
- machine_rank: 0
- num_machines: 1
- main_process_ip: None
- main_process_port: None
- main_training_function: main
- deepspeed_config: {}
- fsdp_config: {}
```
# Fully Sharded Data Parallel utilities
## enable_fsdp_ram_efficient_loading
## disable_fsdp_ram_efficient_loading
## merge_fsdp_weights
## FullyShardedDataParallelPlugin
[[autodoc]] utils.enable_fsdp_ram_efficient_loading
[[autodoc]] utils.disable_fsdp_ram_efficient_loading
[[autodoc]] utils.merge_fsdp_weights
[[autodoc]] utils.FullyShardedDataParallelPlugin
# Logging
Refer to the [Troubleshooting guide](../usage_guides/troubleshooting#logging) or to the example below to learn
how to use Accelerate's logger.
[[autodoc]] logging.get_logger
# Launchers
Functions for launching training on distributed processes.
## notebook_launcher
## debug_launcher
[[autodoc]] accelerate.notebook_launcher
[[autodoc]] accelerate.debug_launcher
# DataLoaders, Optimizers, and Schedulers
The internal classes Accelerate uses to prepare objects for distributed training
when calling `prepare()`.
## DataLoader utilities
## BatchSamplerShard
## IterableDatasetShard
## DataLoaderShard
## DataLoaderDispatcher
## AcceleratedOptimizer
## AcceleratedScheduler
[[autodoc]] data_loader.prepare_data_loader
[[autodoc]] data_loader.skip_first_batches
[[autodoc]] data_loader.BatchSamplerShard
[[autodoc]] data_loader.IterableDatasetShard
[[autodoc]] data_loader.DataLoaderShard
[[autodoc]] data_loader.DataLoaderDispatcher
[[autodoc]] optimizer.AcceleratedOptimizer
[[autodoc]] scheduler.AcceleratedScheduler
# DeepSpeed utilities
## DeepSpeedPlugin
## get_active_deepspeed_plugin
## DeepSpeedEnginerWrapper
## DeepSpeedOptimizerWrapper
## DeepSpeedSchedulerWrapper
## DummyOptim
## DummyScheduler
[[autodoc]] utils.get_active_deepspeed_plugin
[[autodoc]] utils.DeepSpeedPlugin
[[autodoc]] utils.deepspeed.DummyScheduler
[[autodoc]] utils.deepspeed.DeepSpeedEngineWrapper
[[autodoc]] utils.deepspeed.DeepSpeedOptimizerWrapper
[[autodoc]] utils.deepspeed.DeepSpeedSchedulerWrapper
[[autodoc]] utils.deepspeed.DummyOptim
# Working with large models
## Dispatch and offload
### init_empty_weights
### cpu_offload
### cpu_offload_with_hook
### disk_offload
### dispatch_model
### load_checkpoint_and_dispatch
### load_checkpoint_in_model
### infer_auto_device_map
## Hooks
### ModelHook
### AlignDevicesHook
### SequentialHook
## Adding Hooks
### add_hook_to_module
### attach_execution_device_hook
### attach_align_device_hook
### attach_align_device_hook_on_blocks
## Removing Hooks
### remove_hook_from_module
### remove_hook_from_submodules
## Utilities
### has_offloaded_params
### align_module_device
[[autodoc]] big_modeling.init_empty_weights
[[autodoc]] big_modeling.cpu_offload
[[autodoc]] big_modeling.cpu_offload_with_hook
[[autodoc]] big_modeling.disk_offload
[[autodoc]] big_modeling.dispatch_model
[[autodoc]] big_modeling.load_checkpoint_and_dispatch
[[autodoc]] big_modeling.load_checkpoint_in_model
[[autodoc]] utils.infer_auto_device_map
[[autodoc]] hooks.ModelHook
[[autodoc]] hooks.AlignDevicesHook
[[autodoc]] hooks.SequentialHook
[[autodoc]] hooks.add_hook_to_module
[[autodoc]] hooks.attach_execution_device_hook
[[autodoc]] hooks.attach_align_device_hook
[[autodoc]] hooks.attach_align_device_hook_on_blocks
[[autodoc]] hooks.remove_hook_from_module
[[autodoc]] hooks.remove_hook_from_submodules
[[autodoc]] utils.has_offloaded_params
[[autodoc]] utils.align_module_device
# The Command Line
Below is a list of all the available commands 🤗 Accelerate with their parameters
## accelerate config
**Command**:
`accelerate config` or `accelerate-config`
Launches a series of prompts to create and save a `default_config.yml` configuration file for your training system. Should
always be ran first on your machine.
**Usage**:
```bash
accelerate config [arguments]
```
**Optional Arguments**:
* `--config_file CONFIG_FILE` (`str`) -- The path to use to store the config file. Will default to a file named default_config.yaml in the cache location, which is the content
of the environment `HF_HOME` suffixed with 'accelerate', or if you don't have such an environment variable, your cache directory
(`~/.cache` or the content of `XDG_CACHE_HOME`) suffixed with `huggingface`.
* `-h`, `--help` (`bool`) -- Show a help message and exit
## accelerate config default
**Command**:
`accelerate config default` or `accelerate-config default`
Create a default config file for Accelerate with only a few flags set.
**Usage**:
```bash
accelerate config default [arguments]
```
**Optional Arguments**:
* `--config_file CONFIG_FILE` (`str`) -- The path to use to store the config file. Will default to a file named default_config.yaml in the cache location, which is the content
of the environment `HF_HOME` suffixed with 'accelerate', or if you don't have such an environment variable, your cache directory
(`~/.cache` or the content of `XDG_CACHE_HOME`) suffixed with `huggingface`.
* `-h`, `--help` (`bool`) -- Show a help message and exit
* `--mixed_precision {no,fp16,bf16}` (`str`) -- Whether or not to use mixed precision training. Choose between FP16 and BF16 (bfloat16) training. BF16 training is only supported on Nvidia Ampere GPUs and PyTorch 1.10 or later.
## accelerate config update
**Command**:
`accelerate config update` or `accelerate-config update`
Update an existing config file with the latest defaults while maintaining the old configuration.
**Usage**:
```bash
accelerate config update [arguments]
```
**Optional Arguments**:
* `--config_file CONFIG_FILE` (`str`) -- The path to the config file to update. Will default to a file named default_config.yaml in the cache location, which is the content
of the environment `HF_HOME` suffixed with 'accelerate', or if you don't have such an environment variable, your cache directory
(`~/.cache` or the content of `XDG_CACHE_HOME`) suffixed with `huggingface`.
* `-h`, `--help` (`bool`) -- Show a help message and exit
## accelerate env
**Command**:
`accelerate env` or `accelerate-env` or `python -m accelerate.commands.env`
Lists the contents of the passed 🤗 Accelerate configuration file. Should always be used when opening an issue on the [GitHub repository](https://github.com/huggingface/accelerate).
**Usage**:
```bash
accelerate env [arguments]
```
**Optional Arguments**:
* `--config_file CONFIG_FILE` (`str`) -- The path to use to store the config file. Will default to a file named default_config.yaml in the cache location, which is the content
of the environment `HF_HOME` suffixed with 'accelerate', or if you don't have such an environment variable, your cache directory
(`~/.cache` or the content of `XDG_CACHE_HOME`) suffixed with `huggingface`.
* `-h`, `--help` (`bool`) -- Show a help message and exit
## accelerate launch
**Command**:
`accelerate launch` or `accelerate-launch` or `python -m accelerate.commands.launch`
Launches a specified script on a distributed system with the right parameters.
**Usage**:
```bash
accelerate launch [arguments] {training_script} --{training_script-argument-1} --{training_script-argument-2} ...
```
**Positional Arguments**:
- `{training_script}` -- The full path to the script to be launched in parallel
- `--{training_script-argument-1}` -- Arguments of the training script
**Optional Arguments**:
* `-h`, `--help` (`bool`) -- Show a help message and exit
* `--config_file CONFIG_FILE` (`str`)-- The config file to use for the default values in the launching script.
* `-m`, `--module` (`bool`) -- Change each process to interpret the launch script as a Python module, executing with the same behavior as 'python -m'.
* `--no_python` (`bool`) -- Skip prepending the training script with 'python' - just execute it directly. Useful when the script is not a Python script.
* `--debug` (`bool`) -- Whether to print out the torch.distributed stack trace when something fails.
* `-q`, `--quiet` (`bool`) -- Silence subprocess errors from the launch stack trace to only show the relevant tracebacks. (Only applicable to DeepSpeed and single-process configurations).
The rest of these arguments are configured through `accelerate config` and are read in from the specified `--config_file` (or default configuration) for their
values. They can also be passed in manually.
**Hardware Selection Arguments**:
* `--cpu` (`bool`) -- Whether or not to force the training on the CPU.
* `--multi_gpu` (`bool`) -- Whether or not this should launch a distributed GPU training.
* `--tpu` (`bool`) -- Whether or not this should launch a TPU training.
* `--ipex` (`bool`) -- Whether or not this should launch an Intel Pytorch Extension (IPEX) training.
**Resource Selection Arguments**:
The following arguments are useful for fine-tuning how available hardware should be used
* `--mixed_precision {no,fp16,bf16,fp8}` (`str`) -- Whether or not to use mixed precision training. Choose between FP16 and BF16 (bfloat16) training. BF16 training is only supported on Nvidia Ampere GPUs and PyTorch 1.10 or later.
* `--num_processes NUM_PROCESSES` (`int`) -- The total number of processes to be launched in parallel.
* `--num_machines NUM_MACHINES` (`int`) -- The total number of machines used in this training.
* `--num_cpu_threads_per_process NUM_CPU_THREADS_PER_PROCESS` (`int`) -- The number of CPU threads per process. Can be tuned for optimal performance.
* `--enable_cpu_affinity` (`bool`) -- Whether or not CPU affinity and balancing should be enabled. Currently only supported on NVIDIA hardware.
**Training Paradigm Arguments**:
The following arguments are useful for selecting which training paradigm to use.
* `--use_deepspeed` (`bool`) -- Whether or not to use DeepSpeed for training.
* `--use_fsdp` (`bool`) -- Whether or not to use FullyShardedDataParallel for training.
* `--use_megatron_lm` (`bool`) -- Whether or not to use Megatron-LM for training.
* `--use_xpu` (`bool`) -- Whether to use IPEX plugin to speed up training on XPU specifically.
**Distributed GPU Arguments**:
The following arguments are only useful when `multi_gpu` is passed or multi-gpu training is configured through `accelerate config`:
* `--gpu_ids` (`str`) -- What GPUs (by id) should be used for training on this machine as a comma-seperated list
* `--same_network` (`bool`) -- Whether all machines used for multinode training exist on the same local network.
* `--machine_rank` (`int`) -- The rank of the machine on which this script is launched.
* `--main_process_ip` (`str`) -- The IP address of the machine of rank 0.
* `--main_process_port` (`int`) -- The port to use to communicate with the machine of rank 0.
* `-t`, `--tee` (`str`) -- Tee std streams into a log file and also to console.
* `--log_dir` (`str`) -- Base directory to use for log files when using torchrun/torch.distributed.run as launcher. Use with --tee to redirect std streams info log files.
* `--role` (`str`) -- User-defined role for the workers.
* `--rdzv_backend` (`str`) -- The rendezvous method to use, such as 'static' (the default) or 'c10d'
* `--rdzv_conf` (`str`) -- Additional rendezvous configuration (=,=,...).
* `--max_restarts` (`int`) -- Maximum number of worker group restarts before failing.
* `--monitor_interval` (`int`) -- Interval, in seconds, to monitor the state of workers.
**TPU Arguments**:
The following arguments are only useful when `tpu` is passed or TPU training is configured through `accelerate config`:
* `--tpu_cluster` (`bool`) -- Whether to use a GCP TPU pod for training.
* `--tpu_use_sudo` (`bool`) -- Whether to use `sudo` when running the TPU training script in each pod.
* `--vm` (`str`) -- List of single Compute VM instance names. If not provided we assume usage of instance groups. For TPU pods.
* `--env` (`str`) -- List of environment variables to set on the Compute VM instances. For TPU pods.
* `--main_training_function` (`str`) -- The name of the main function to be executed in your script (only for TPU training).
* `--downcast_bf16` (`bool`) -- Whether when using bf16 precision on TPUs if both float and double tensors are cast to bfloat16 or if double tensors remain as float32.
**DeepSpeed Arguments**:
The following arguments are only useful when `use_deepspeed` is passed or `deepspeed` is configured through `accelerate config`:
* `--deepspeed_config_file` (`str`) -- DeepSpeed config file.
* `--zero_stage` (`int`) -- DeepSpeed's ZeRO optimization stage.
* `--offload_optimizer_device` (`str`) -- Decides where (none|cpu|nvme) to offload optimizer states.
* `--offload_param_device` (`str`) -- Decides where (none|cpu|nvme) to offload parameters.
* `--offload_optimizer_nvme_path` (`str`) -- Decides Nvme Path to offload optimizer states.
* `--gradient_accumulation_steps` (`int`) -- No of gradient_accumulation_steps used in your training script.
* `--gradient_clipping` (`float`) -- Gradient clipping value used in your training script.
* `--zero3_init_flag` (`str`) -- Decides Whether (true|false) to enable `deepspeed.zero.Init` for constructing massive models. Only applicable with DeepSpeed ZeRO Stage-3.
* `--zero3_save_16bit_model` (`str`) -- Decides Whether (true|false) to save 16-bit model weights when using ZeRO Stage-3. Only applicable with DeepSpeed ZeRO Stage-3.
* `--deepspeed_hostfile` (`str`) -- DeepSpeed hostfile for configuring multi-node compute resources.
* `--deepspeed_exclusion_filter` (`str`) -- DeepSpeed exclusion filter string when using mutli-node setup.
* `--deepspeed_inclusion_filter` (`str`) -- DeepSpeed inclusion filter string when using mutli-node setup.
* `--deepspeed_multinode_launcher` (`str`) -- DeepSpeed multi-node launcher to use.
* `--deepspeed_moe_layer_cls_names` (`str`) -- comma-separated list of transformer MoE layer class names (case-sensitive) to wrap, e.g, `MixtralSparseMoeBlock` `Qwen2MoeSparseMoeBlock`, `JetMoEAttention,JetMoEBlock`
**Fully Sharded Data Parallelism Arguments**:
The following arguments are only useful when `use_fsdp` is passed or Fully Sharded Data Parallelism is configured through `accelerate config`:
* `--fsdp_offload_params` (`str`) -- Decides Whether (true|false) to offload parameters and gradients to CPU.
* `--fsdp_min_num_params` (`int`) -- FSDP's minimum number of parameters for Default Auto Wrapping.
* `--fsdp_sharding_strategy` (`int`) -- FSDP's Sharding Strategy.
* `--fsdp_auto_wrap_policy` (`str`) -- FSDP's auto wrap policy.
* `--fsdp_transformer_layer_cls_to_wrap` (`str`) -- Transformer layer class name (case-sensitive) to wrap, e.g, `BertLayer`, `GPTJBlock`, `T5Block` ...
* `--fsdp_backward_prefetch_policy` (`str`) -- FSDP's backward prefetch policy.
* `--fsdp_state_dict_type` (`str`) -- FSDP's state dict type.
* `--fsdp_forward_prefetch` (`str`) -- FSDP forward prefetch.
* `--fsdp_use_orig_params` (`str`) -- If True, allows non-uniform `requires_grad` mixed in a FSDP unit.
* `--fsdp_cpu_ram_efficient_loading` (`str`) -- If true, only the first process loads the pretrained model checkoint while all other processes have empty weights. When using this, `--fsdp_sync_module_states` needs to True.
* `--fsdp_sync_module_states` (`str`) -- If true, each individually wrapped FSDP unit will broadcast module parameters from rank 0.
* `--fsdp_activation_checkpointing` (`bool`) -- Decides Whether intermediate activations are freed during the forward pass, and a checkpoint is left as a placeholder
**Megatron-LM Arguments**:
The following arguments are only useful when `use_megatron_lm` is passed or Megatron-LM is configured through `accelerate config`:
* `--megatron_lm_tp_degree` (``) -- Megatron-LM's Tensor Parallelism (TP) degree.
* `--megatron_lm_pp_degree` (``) -- Megatron-LM's Pipeline Parallelism (PP) degree.
* `--megatron_lm_num_micro_batches` (``) -- Megatron-LM's number of micro batches when PP degree > 1.
* `--megatron_lm_sequence_parallelism` (``) -- Decides Whether (true|false) to enable Sequence Parallelism when TP degree > 1.
* `--megatron_lm_recompute_activations` (``) -- Decides Whether (true|false) to enable Selective Activation Recomputation.
* `--megatron_lm_use_distributed_optimizer` (``) -- Decides Whether (true|false) to use distributed optimizer which shards optimizer state and gradients across Data Parallel (DP) ranks.
* `--megatron_lm_gradient_clipping` (``) -- Megatron-LM's gradient clipping value based on global L2 Norm (0 to disable).
**FP8 Arguments**:
* `--fp8_backend` (`str`) -- Choose a backend to train with FP8 (`te` or `msamp`)
* `--fp8_use_autocast_during_eval` (`bool`) -- Whether to use FP8 autocast during eval mode (useful only when `--fp8_backend=te` is passed). Generally better metrics are found when this is not passed.
* `--fp8_margin` (`int`) -- The margin to use for the gradient scaling (useful only when `--fp8_backend=te` is passed).
* `--fp8_interval` (`int`) -- The interval to use for how often the scaling factor is recomputed (useful only when `--fp8_backend=te` is passed).
* `--fp8_format` (`str`) -- The format to use for the FP8 recipe (useful only when `--fp8_backend=te` is passed).
* `--fp8_amax_history_len` (`int`) -- The length of the history to use for the scaling factor computation (useful only when `--fp8_backend=te` is passed).
* `--fp8_amax_compute_algo` (`str`) -- The algorithm to use for the scaling factor computation. (useful only when `--fp8_backend=te` is passed).
* `--fp8_override_linear_precision` (`Tuple[bool, bool, bool]`) -- Whether or not to execute `fprop`, `dgrad`, and `wgrad` GEMMS in higher precision.
* `--fp8_opt_level` (`str`) -- What level of 8-bit collective communication should be used with MS-AMP (useful only when `--fp8_backend=msamp` is passed)
**AWS SageMaker Arguments**:
The following arguments are only useful when training in SageMaker
* `--aws_access_key_id AWS_ACCESS_KEY_ID` (`str`) -- The AWS_ACCESS_KEY_ID used to launch the Amazon SageMaker training job
* `--aws_secret_access_key AWS_SECRET_ACCESS_KEY` (`str`) -- The AWS_SECRET_ACCESS_KEY used to launch the Amazon SageMaker training job
## accelerate estimate-memory
**Command**:
`accelerate estimate-memory` or `accelerate-estimate-memory` or `python -m accelerate.commands.estimate`
Estimates the total vRAM a particular model hosted on the Hub needs to be loaded in with an estimate for training. Requires that `huggingface_hub` be installed.
When performing inference, typically add ≤20% to the result as overall allocation [as referenced here](https://blog.eleuther.ai/transformer-math/). We will have more extensive estimations in the future that will automatically be included in the calculation.
**Usage**:
```bash
accelerate estimate-memory {MODEL_NAME} --library_name {LIBRARY_NAME} --dtypes {dtype_1} {dtype_2} ...
```
**Required Arguments**:
* `MODEL_NAME` (`str`)-- The model name on the Hugging Face Hub
**Optional Arguments**:
* `--library_name {timm,transformers}` (`str`) -- The library the model has an integration with, such as `transformers`, needed only if this information is not stored on the Hub
* `--dtypes {float32,float16,int8,int4}` (`[{float32,float16,int8,int4} ...]`) -- The dtypes to use for the model, must be one (or many) of `float32`, `float16`, `int8`, and `int4`
* `--trust_remote_code` (`bool`) -- Whether or not to allow for custom models defined on the Hub in their own modeling files. This option should only be passed for repositories you trust and in which you have read the code, as it will execute code present on the Hub on your local machine.
## accelerate tpu-config
`accelerate tpu-config`
**Usage**:
```bash
accelerate tpu-config [arguments]
```
**Optional Arguments**:
* `-h`, `--help` (`bool`) -- Show a help message and exit
**Config Arguments**:
Arguments that can be configured through `accelerate config`.
* `--config_file` (`str`) -- Path to the config file to use for accelerate.
* `--tpu_name` (`str`) -- The name of the TPU to use. If not specified, will use the TPU specified in the config file.
* `--tpu_zone` (`str`) -- The zone of the TPU to use. If not specified, will use the zone specified in the config file.
**TPU Arguments**:
Arguments for options ran inside the TPU.
* `--command_file` (`str`) -- The path to the file containing the commands to run on the pod on startup.
* `--command` (`str`) -- A command to run on the pod. Can be passed multiple times.
* `--install_accelerate` (`bool`) -- Whether to install accelerate on the pod. Defaults to False.
* `--accelerate_version` (`str`) -- The version of accelerate to install on the pod. If not specified, will use the latest pypi version. Specify 'dev' to install from GitHub.
* `--debug` (`bool`) -- If set, will print the command that would be run instead of running it.
## accelerate test
`accelerate test` or `accelerate-test`
Runs `accelerate/test_utils/test_script.py` to verify that 🤗 Accelerate has been properly configured on your system and runs.
**Usage**:
```bash
accelerate test [arguments]
```
**Optional Arguments**:
* `--config_file CONFIG_FILE` (`str`) -- The path to use to store the config file. Will default to a file named default_config.yaml in the cache location, which is the content
of the environment `HF_HOME` suffixed with 'accelerate', or if you don't have such an environment variable, your cache directory
(`~/.cache` or the content of `XDG_CACHE_HOME`) suffixed with `huggingface`.
* `-h`, `--help` (`bool`) -- Show a help message and exit
# Stateful Classes
Below are variations of a [singleton class](https://en.wikipedia.org/wiki/Singleton_pattern) in the sense that all
instances share the same state, which is initialized on the first instantiation.
These classes are immutable and store information about certain configurations or
states.
## PartialState
## AcceleratorState
## GradientState
[[autodoc]] state.PartialState
[[autodoc]] state.AcceleratorState
[[autodoc]] state.GradientState
# Megatron-LM utilities
## MegatronLMPlugin
## MegatronLMDummyScheduler
## MegatronLMDummyDataLoader
## AbstractTrainStep
## GPTTrainStep
## BertTrainStep
## T5TrainStep
## avg_losses_across_data_parallel_group
[[autodoc]] utils.MegatronLMPlugin
[[autodoc]] utils.MegatronLMDummyScheduler
[[autodoc]] utils.MegatronLMDummyDataLoader
[[autodoc]] utils.AbstractTrainStep
[[autodoc]] utils.GPTTrainStep
[[autodoc]] utils.BertTrainStep
[[autodoc]] utils.T5TrainStep
[[autodoc]] utils.avg_losses_across_data_parallel_group
# Kwargs handlers
The following objects can be passed to the main `Accelerator` to customize how some PyTorch objects
related to distributed training or mixed precision are created.
## AutocastKwargs
## DistributedDataParallelKwargs
## FP8RecipeKwargs
## ProfileKwargs
## GradScalerKwargs
## InitProcessGroupKwargs
## KwargsHandler
[[autodoc]] AutocastKwargs
[[autodoc]] DistributedDataParallelKwargs
[[autodoc]] utils.FP8RecipeKwargs
[[autodoc]] utils.ProfileKwargs
[[autodoc]] GradScalerKwargs
[[autodoc]] InitProcessGroupKwargs
[[autodoc]] utils.KwargsHandler
# Pipeline parallelism
Accelerate supports pipeline parallelism for large-scale training with the PyTorch [torch.distributed.pipelining](https://pytorch.org/docs/stable/distributed.pipelining.html) API.
## prepare_pippy
[[autodoc]] inference.prepare_pippy
# FP8
Below are functions and classes relative to the underlying FP8 implementation
## FP8RecipeKwargs
## convert_model
## has_transformer_engine_layers
## contextual_fp8_autocast
## apply_fp8_autowrap
[[autodoc]] utils.FP8RecipeKwargs
[[autodoc]] utils.convert_model
[[autodoc]] utils.has_transformer_engine_layers
[[autodoc]] utils.contextual_fp8_autocast
[[autodoc]] utils.apply_fp8_autowrap
# Utility functions and classes
Below are a variety of utility functions that 🤗 Accelerate provides, broken down by use-case.
## Constants
Constants used throughout 🤗 Accelerate for reference
The following are constants used when utilizing `Accelerator.save_state()`
`utils.MODEL_NAME`: `"pytorch_model"`
`utils.OPTIMIZER_NAME`: `"optimizer"`
`utils.RNG_STATE_NAME`: `"random_states"`
`utils.SCALER_NAME`: `"scaler.pt`
`utils.SCHEDULER_NAME`: `"scheduler`
The following are constants used when utilizing `Accelerator.save_model()`
`utils.WEIGHTS_NAME`: `"pytorch_model.bin"`
`utils.SAFE_WEIGHTS_NAME`: `"model.safetensors"`
`utils.WEIGHTS_INDEX_NAME`: `"pytorch_model.bin.index.json"`
`utils.SAFE_WEIGHTS_INDEX_NAME`: `"model.safetensors.index.json"`
## Data Classes
These are basic dataclasses used throughout 🤗 Accelerate and they can be passed in as parameters.
### Standalone
These are standalone dataclasses used for checks, such as the type of distributed system being used
### Kwargs
These are configurable arguments for specific interactions throughout the PyTorch ecosystem that Accelerate handles under the hood.
## Plugins
These are plugins that can be passed to the `Accelerator` object. While they are defined elsewhere in the documentation,
for convenience all of them are available to see here:
## Configurations
These are classes which can be configured and passed through to the appropriate integration
## Environmental Variables
These are environmental variables that can be enabled for different use cases
* `ACCELERATE_DEBUG_MODE` (`str`): Whether to run accelerate in debug mode. More info available [here](../usage_guides/debug.md).
## Data Manipulation and Operations
These include data operations that mimic the same `torch` ops but can be used on distributed processes.
## Environment Checks
These functionalities check the state of the current working environment including information about the operating system itself, what it can support, and if particular dependencies are installed.
## Environment Manipulation
When setting up 🤗 Accelerate for the first time, rather than running `accelerate config` [~utils.write_basic_config] can be used as an alternative for quick configuration.
## Memory
## Modeling
These utilities relate to interacting with PyTorch models
## Parallel
These include general utilities that should be used when working in parallel.
## Random
These utilities relate to setting and synchronizing of all the random states.
## PyTorch XLA
These include utilities that are useful while using PyTorch with XLA.
## Loading model weights
These include utilities that are useful to load checkpoints.
## Quantization
These include utilities that are useful to quantize model.
[[autodoc]] utils.ComputeEnvironment
[[autodoc]] utils.DistributedType
[[autodoc]] utils.DynamoBackend
[[autodoc]] utils.LoggerType
[[autodoc]] utils.PrecisionType
[[autodoc]] utils.RNGType
[[autodoc]] utils.SageMakerDistributedType
[[autodoc]] utils.AutocastKwargs
[[autodoc]] utils.DistributedDataParallelKwargs
[[autodoc]] utils.FP8RecipeKwargs
[[autodoc]] utils.GradScalerKwargs
[[autodoc]] utils.InitProcessGroupKwargs
[[autodoc]] utils.KwargsHandler
[[autodoc]] utils.DeepSpeedPlugin
[[autodoc]] utils.FullyShardedDataParallelPlugin
[[autodoc]] utils.GradientAccumulationPlugin
[[autodoc]] utils.MegatronLMPlugin
[[autodoc]] utils.TorchDynamoPlugin
[[autodoc]] utils.BnbQuantizationConfig
[[autodoc]] utils.DataLoaderConfiguration
[[autodoc]] utils.ProjectConfiguration
[[autodoc]] utils.broadcast
[[autodoc]] utils.broadcast_object_list
[[autodoc]] utils.concatenate
[[autodoc]] utils.convert_outputs_to_fp32
[[autodoc]] utils.convert_to_fp32
[[autodoc]] utils.gather
[[autodoc]] utils.gather_object
[[autodoc]] utils.get_grad_scaler
[[autodoc]] utils.get_mixed_precision_context_manager
[[autodoc]] utils.listify
[[autodoc]] utils.pad_across_processes
[[autodoc]] utils.recursively_apply
[[autodoc]] utils.reduce
[[autodoc]] utils.send_to_device
[[autodoc]] utils.slice_tensors
[[autodoc]] utils.is_bf16_available
[[autodoc]] utils.is_ipex_available
[[autodoc]] utils.is_mps_available
[[autodoc]] utils.is_npu_available
[[autodoc]] utils.is_torch_version
[[autodoc]] utils.is_torch_xla_available
[[autodoc]] utils.is_xpu_available
[[autodoc]] utils.patch_environment
[[autodoc]] utils.clear_environment
[[autodoc]] utils.write_basic_config
[[autodoc]] utils.set_numa_affinity
[[autodoc]] utils.environment.override_numa_affinity
[[autodoc]] utils.find_executable_batch_size
[[autodoc]] utils.calculate_maximum_sizes
[[autodoc]] utils.compute_module_sizes
[[autodoc]] utils.extract_model_from_parallel
[[autodoc]] utils.get_balanced_memory
[[autodoc]] utils.get_max_layer_size
[[autodoc]] utils.infer_auto_device_map
[[autodoc]] utils.load_checkpoint_in_model
[[autodoc]] utils.load_offloaded_weights
[[autodoc]] utils.load_state_dict
[[autodoc]] utils.offload_state_dict
[[autodoc]] utils.retie_parameters
[[autodoc]] utils.set_module_tensor_to_device
[[autodoc]] utils.extract_model_from_parallel
[[autodoc]] utils.save
[[autodoc]] utils.load
[[autodoc]] utils.wait_for_everyone
[[autodoc]] utils.set_seed
[[autodoc]] utils.synchronize_rng_state
[[autodoc]] utils.synchronize_rng_states
[[autodoc]] utils.install_xla
[[autodoc]] utils.load_checkpoint_in_model
[[autodoc]] utils.load_and_quantize_model
# Accelerator
The `Accelerator` is the main class for enabling distributed training on any type of training setup. Read the [Add Accelerator to your code](../basic_tutorials/migration) tutorial to learn more about how to add the `Accelerator` to your script.
## Accelerator[[api]]
## Utilities
[[autodoc]] Accelerator
[[autodoc]] accelerate.utils.gather_object
# Experiment Trackers
## GeneralTracker
## TensorBoardTracker
## WandBTracker
## CometMLTracker
## AimTracker
## MLflowTracker
## ClearMLTracker
[[autodoc]] tracking.GeneralTracker
[[autodoc]] tracking.TensorBoardTracker
- __init__
[[autodoc]] tracking.WandBTracker
- __init__
[[autodoc]] tracking.CometMLTracker
- __init__
[[autodoc]] tracking.AimTracker
- __init__
[[autodoc]] tracking.MLflowTracker
- __init__
[[autodoc]] tracking.ClearMLTracker
- __init__