File size: 4,446 Bytes
f4b3fa4 27127ad f4b3fa4 27127ad f4b3fa4 27127ad f4b3fa4 27127ad f4b3fa4 |
1 2 3 4 5 6 7 8 9 10 11 12 13 14 15 16 17 18 19 20 21 22 23 24 25 26 27 28 29 30 31 32 33 34 35 36 37 38 39 40 41 42 43 44 45 46 47 48 49 50 51 52 53 54 55 56 57 58 59 60 61 62 63 64 65 66 67 68 69 70 71 72 73 74 75 76 77 78 79 80 81 82 83 84 85 86 87 88 89 90 91 92 93 94 95 96 97 98 99 100 101 102 103 104 105 106 107 108 109 110 111 112 113 114 115 116 117 118 119 120 121 122 123 124 125 126 127 128 129 130 131 132 133 134 |
---
datasets:
- lmms-lab/LLaVA-OneVision-Data
language:
- en
- zh
library_name: transformers
license: apache-2.0
metrics:
- accuracy
tags:
- multimodal
---
# LLaVA-OneVision
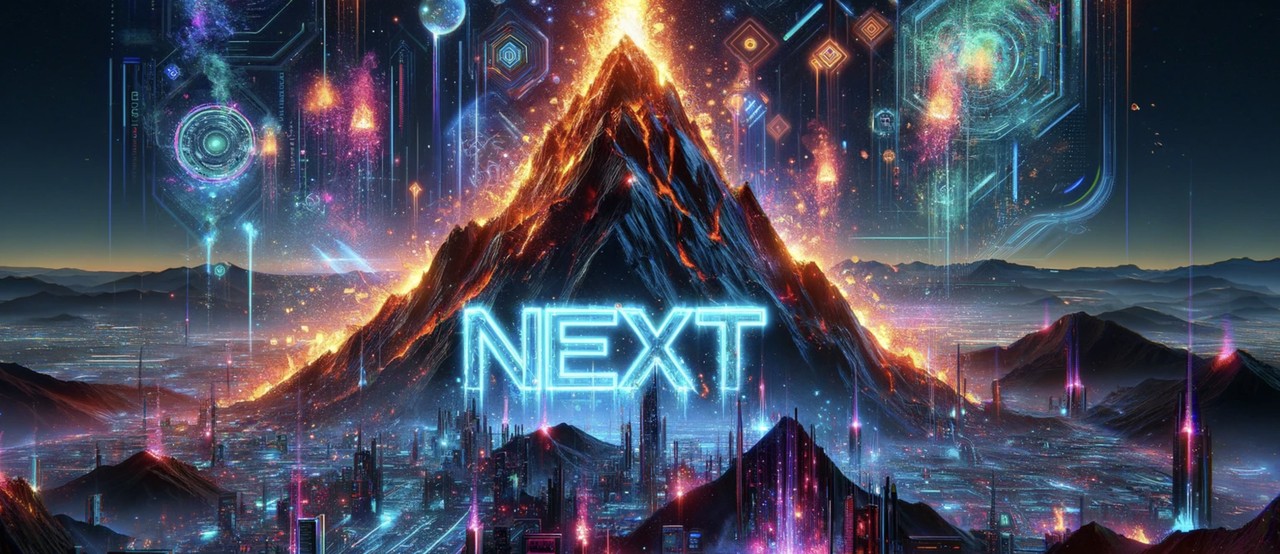
Play with the model on the [LLaVA OneVision Chat](https://llava-onevision.lmms-lab.com/).
## Table of Contents
1. [Model Summary](##model-summary)
2. [Use](##use)
3. [Limitations](##limitations)
4. [Training](##training)
5. [License](##license)
6. [Citation](##citation)
## Model Summary
`llava-onevision-72b-ov-chat` is our latest model specifically designed for chat scenarios. It is built upon `llava-onevision-72b-ov` and has undergone iterative DPO training with human preference, making it well-suited for chat applications.
Research by [Tianyi Xiong](https://tyxiong23.github.io/) indicates that our iterative DPO training method enhances the model's chat capabilities while preserving its instruction-following abilities.
For further details, please refer to our upcoming blog or paper.
- **Repository:** [LLaVA-VL/LLaVA-NeXT](https://github.com/LLaVA-VL/LLaVA-NeXT?tab=readme-ov-file)
- **Project Website:** [llava-onevision.lmms-lab.com](llava-onevision.lmms-lab.com)
- **Paper:** [LLaVA-OneVision](arxiv.org/abs/2408.03326)
- **Point of Contact:** [Tianyi Xiong](https://tyxiong23.github.io/), [Bo Li](mailto:[email protected])
- **Languages:** English, Chinese
## Benchmark Performance
To be released
## Use
### Intended use
The model was trained on [LLaVA-OneVision Dataset](https://huggingface.co/datasets/lmms-lab/LLaVA-OneVision-Data) and have the ability to interact with images, multi-image and videos.
**Feel free to share your generations in the Community tab!**
### Generation
```python
# pip install git+https://github.com/LLaVA-VL/LLaVA-NeXT.git
from llava.model.builder import load_pretrained_model
from llava.mm_utils import get_model_name_from_path, process_images, tokenizer_image_token
from llava.constants import IMAGE_TOKEN_INDEX, DEFAULT_IMAGE_TOKEN, DEFAULT_IM_START_TOKEN, DEFAULT_IM_END_TOKEN, IGNORE_INDEX
from llava.conversation import conv_templates, SeparatorStyle
from PIL import Image
import requests
import copy
import torch
import sys
import warnings
warnings.filterwarnings("ignore")
pretrained = "lmms-lab/llava-onevision-qwen2-0.5b-si"
model_name = "llava_qwen"
device = "cuda"
device_map = "auto"
tokenizer, model, image_processor, max_length = load_pretrained_model(pretrained, None, model_name, device_map=device_map) # Add any other thing you want to pass in llava_model_args
model.eval()
url = "https://github.com/haotian-liu/LLaVA/blob/1a91fc274d7c35a9b50b3cb29c4247ae5837ce39/images/llava_v1_5_radar.jpg?raw=true"
image = Image.open(requests.get(url, stream=True).raw)
image_tensor = process_images([image], image_processor, model.config)
image_tensor = [_image.to(dtype=torch.float16, device=device) for _image in image_tensor]
conv_template = "qwen_1_5" # Make sure you use correct chat template for different models
question = DEFAULT_IMAGE_TOKEN + "\nWhat is shown in this image?"
conv = copy.deepcopy(conv_templates[conv_template])
conv.append_message(conv.roles[0], question)
conv.append_message(conv.roles[1], None)
prompt_question = conv.get_prompt()
input_ids = tokenizer_image_token(prompt_question, tokenizer, IMAGE_TOKEN_INDEX, return_tensors="pt").unsqueeze(0).to(device)
image_sizes = [image.size]
cont = model.generate(
input_ids,
images=image_tensor,
image_sizes=image_sizes,
do_sample=False,
temperature=0,
max_new_tokens=4096,
)
text_outputs = tokenizer.batch_decode(cont, skip_special_tokens=True)
print(text_outputs)
```
# Training
## Model
- **Architecture:** SO400M + Qwen2
- **Pretraining Stage:** LCS-558K, 1 epoch, projector
- **Mid Stage:** A mixture of 4.7M high-quality synthetic data, 1 epoch, full model
- **Final-Image Stage:** A mixture of 3.6M single-image data, 1 epoch, full model
- **OneVision Stage:** A mixture of 1.6M single-image/multi-image/video data, 1 epoch, full model
- **Precision:** bfloat16
## Hardware & Software
- **GPUs:** 256 \* Nvidia Tesla A100 (for whole model series training)
- **Orchestration:** [Huggingface Trainer](https://huggingface.co/docs/transformers/main_classes/trainer)
- **Neural networks:** [PyTorch](https://github.com/pytorch/pytorch)
# Citation
```
@article{li2024llavaonevision,
title={LLaVA-OneVision},
}
```
|