Update image
Browse files
README.md
CHANGED
@@ -26,7 +26,7 @@ The DETR model is an encoder-decoder transformer with a convolutional backbone.
|
|
26 |
|
27 |
The model is trained using a "bipartite matching loss": one compares the predicted classes + bounding boxes of each of the N = 100 object queries to the ground truth annotations, padded up to the same length N (so if an image only contains 4 objects, 96 annotations will just have a "no object" as class and "no bounding box" as bounding box). The Hungarian matching algorithm is used to create an optimal one-to-one mapping between each of the N queries and each of the N annotations. Next, standard cross-entropy (for the classes) and a linear combination of the L1 and generalized IoU loss (for the bounding boxes) are used to optimize the parameters of the model.
|
28 |
|
29 |
-
, FOS: Computer and information sciences, FOS: Computer and information sciences},
|
91 |
-
|
92 |
title = {Deformable DETR: Deformable Transformers for End-to-End Object Detection},
|
93 |
-
|
94 |
publisher = {arXiv},
|
95 |
-
|
96 |
year = {2020},
|
97 |
-
|
98 |
copyright = {arXiv.org perpetual, non-exclusive license}
|
99 |
}
|
100 |
```
|
|
|
26 |
|
27 |
The model is trained using a "bipartite matching loss": one compares the predicted classes + bounding boxes of each of the N = 100 object queries to the ground truth annotations, padded up to the same length N (so if an image only contains 4 objects, 96 annotations will just have a "no object" as class and "no bounding box" as bounding box). The Hungarian matching algorithm is used to create an optimal one-to-one mapping between each of the N queries and each of the N annotations. Next, standard cross-entropy (for the classes) and a linear combination of the L1 and generalized IoU loss (for the bounding boxes) are used to optimize the parameters of the model.
|
28 |
|
29 |
+
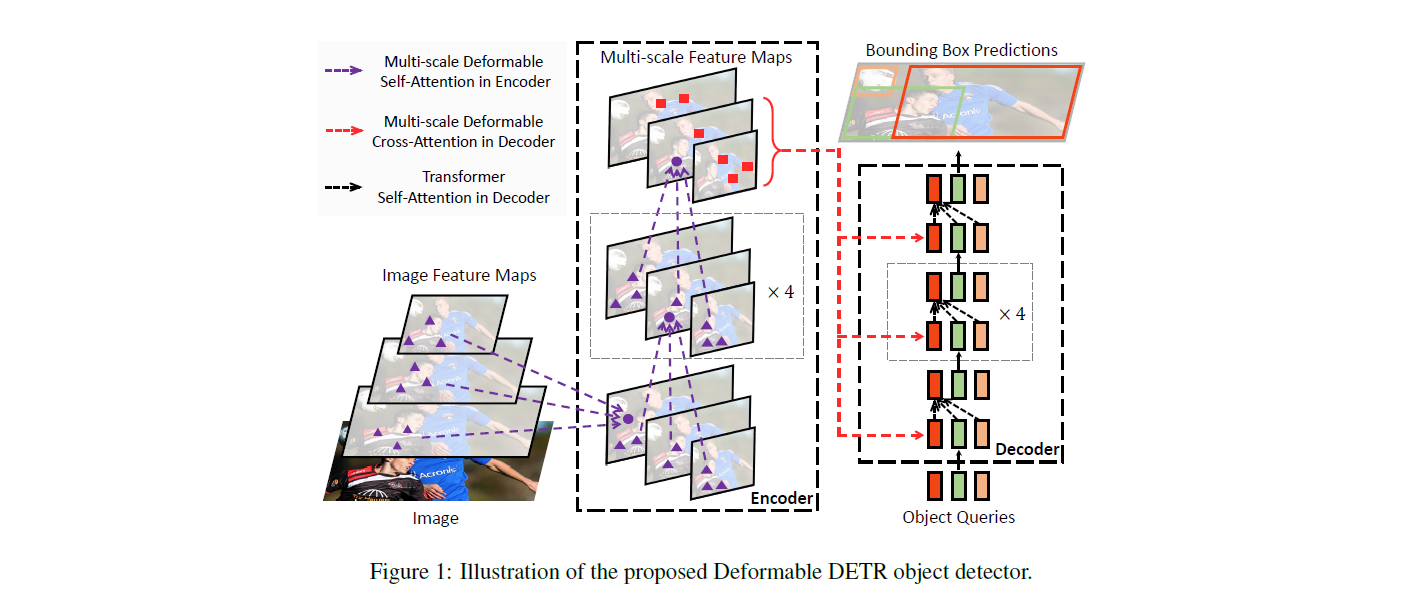
|
30 |
|
31 |
## Intended uses & limitations
|
32 |
|
|
|
82 |
```bibtex
|
83 |
@misc{https://doi.org/10.48550/arxiv.2010.04159,
|
84 |
doi = {10.48550/ARXIV.2010.04159},
|
85 |
+
url = {https://arxiv.org/abs/2010.04159},
|
|
|
|
|
86 |
author = {Zhu, Xizhou and Su, Weijie and Lu, Lewei and Li, Bin and Wang, Xiaogang and Dai, Jifeng},
|
|
|
87 |
keywords = {Computer Vision and Pattern Recognition (cs.CV), FOS: Computer and information sciences, FOS: Computer and information sciences},
|
|
|
88 |
title = {Deformable DETR: Deformable Transformers for End-to-End Object Detection},
|
|
|
89 |
publisher = {arXiv},
|
|
|
90 |
year = {2020},
|
|
|
91 |
copyright = {arXiv.org perpetual, non-exclusive license}
|
92 |
}
|
93 |
```
|